Update README.md
Browse files
README.md
CHANGED
@@ -29,6 +29,28 @@ We introduce AceCoder, the first work to propose a fully automated pipeline for
|
|
29 |
|
30 |
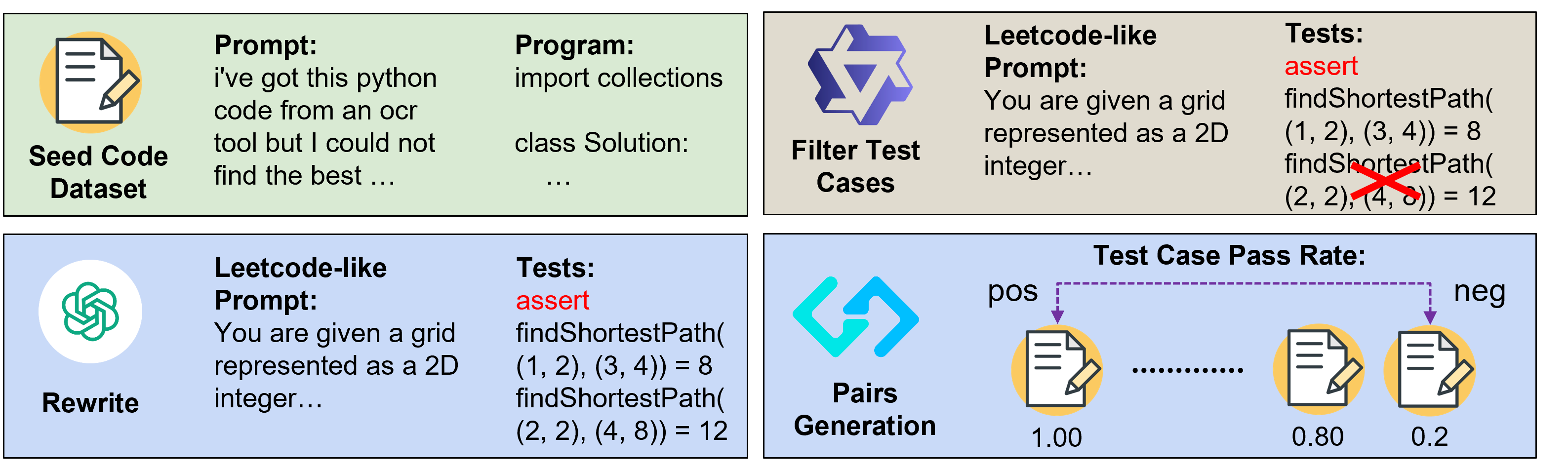
|
31 |
|
|
|
|
|
|
|
|
|
|
|
|
|
|
|
|
|
|
|
|
|
|
|
|
|
|
|
|
|
|
|
|
|
|
|
|
|
|
|
|
|
|
|
|
|
32 |
|
33 |
## Performance on Best-of-N sampling
|
34 |
|
@@ -40,11 +62,11 @@ We introduce AceCoder, the first work to propose a fully automated pipeline for
|
|
40 |
|
41 |
```python
|
42 |
"""pip install git+https://github.com/TIGER-AI-Lab/AceCoder"""
|
43 |
-
from acecoder import
|
44 |
from transformers import AutoTokenizer
|
45 |
|
46 |
model_path = "TIGER-Lab/AceCodeRM-7B"
|
47 |
-
model =
|
48 |
tokenizer = AutoTokenizer.from_pretrained(model_path, trust_remote_code=True)
|
49 |
|
50 |
question = """\
|
@@ -114,17 +136,12 @@ input_tokens = tokenizer.apply_chat_template(
|
|
114 |
return_tensors="pt",
|
115 |
).to(model.device)
|
116 |
|
117 |
-
|
118 |
**input_tokens,
|
119 |
output_hidden_states=True,
|
120 |
return_dict=True,
|
121 |
use_cache=False,
|
122 |
)
|
123 |
-
masks = input_tokens["attention_mask"]
|
124 |
-
rm_scores = values.gather(
|
125 |
-
dim=-1, index=(masks.sum(dim=-1, keepdim=True) - 1)
|
126 |
-
) # find the last token (eos) in each sequence, a
|
127 |
-
rm_scores = rm_scores.squeeze()
|
128 |
|
129 |
print("RM Scores:", rm_scores)
|
130 |
print("Score of program with 3 errors:", rm_scores[0].item())
|
|
|
29 |
|
30 |
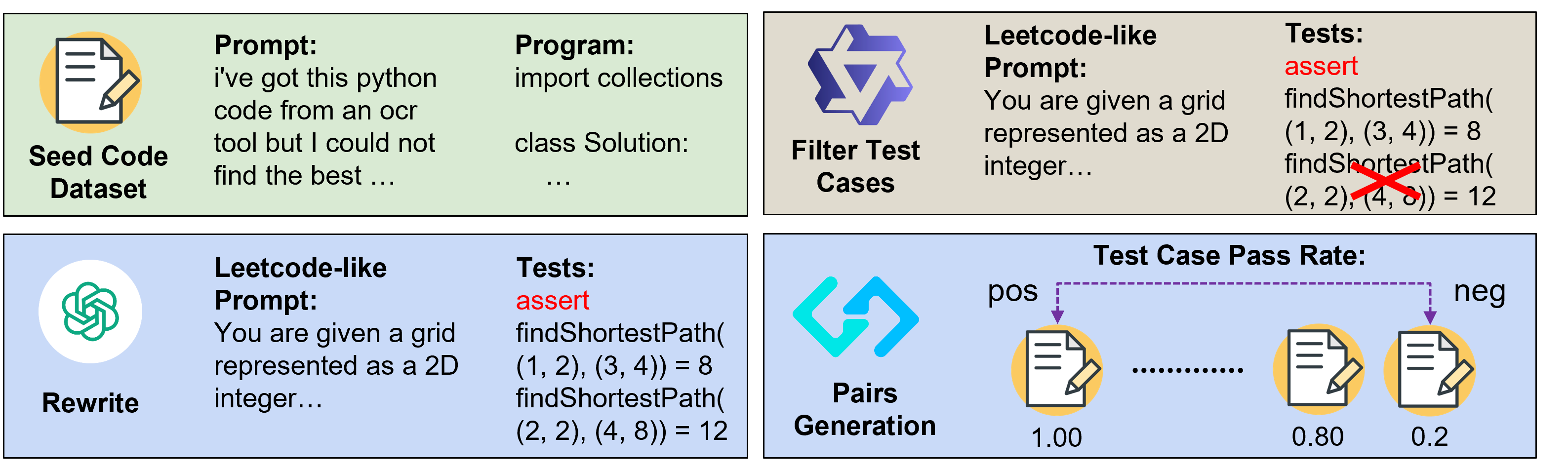
|
31 |
|
32 |
+
## Performance on RM Bench
|
33 |
+
|
34 |
+
```markdown
|
35 |
+
| Model | Code | Chat | Math | Safety | Easy | Normal | Hard | Avg |
|
36 |
+
| ------------------------------------ | ---- | ----- | ----- | ------ | ----- | ------ | ---- | ---- |
|
37 |
+
| Skywork/Skywork-Reward-Llama-3.1-8B | 54.5 | 69.5 | 60.6 | 95.7 | 89 | 74.7 | 46.6 | 70.1 |
|
38 |
+
| LxzGordon/URM-LLaMa-3.1-8B | 54.1 | 71.2 | 61.8 | 93.1 | 84 | 73.2 | 53 | 70 |
|
39 |
+
| NVIDIA/Nemotron-340B-Reward | 59.4 | 71.2 | 59.8 | 87.5 | 81 | 71.4 | 56.1 | 69.5 |
|
40 |
+
| NCSOFT/Llama-3-OffsetBias-RM-8B | 53.2 | 71.3 | 61.9 | 89.6 | 84.6 | 72.2 | 50.2 | 69 |
|
41 |
+
| internlm/internlm2-20b-reward | 56.7 | 63.1 | 66.8 | 86.5 | 82.6 | 71.6 | 50.7 | 68.3 |
|
42 |
+
| Ray2333/GRM-llama3-8B-sftreg | 57.8 | 62.7 | 62.5 | 90 | 83.5 | 72.7 | 48.6 | 68.2 |
|
43 |
+
| Ray2333/GRM-llama3-8B-distill | 56.9 | 62.4 | 62.1 | 88.1 | 82.2 | 71.5 | 48.4 | 67.4 |
|
44 |
+
| Ray2333/GRM-Llama3-8B-rewardmodel-ft | 52.1 | 66.8 | 58.8 | 91.4 | 86.2 | 70.6 | 45.1 | 67.3 |
|
45 |
+
| LxzGordon/URM-LLLaMa-3-8B | 52.3 | 68.5 | 57.6 | 90.3 | 80.2 | 69.9 | 51.5 | 67.2 |
|
46 |
+
| internlm/internlm2-7b-reward | 49.7 | 61.7 | 71.4 | 85.5 | 85.4 | 70.7 | 45.1 | 67.1 |
|
47 |
+
| Skywork-Reward-Llama-3.1-8B-v0.2 | 53.4 | 69.2 | 62.1 | 96 | 88.5 | 74 | 47.9 | 70.1 |
|
48 |
+
| Skywork-Reward-Gemma-2-27B-v0.2 | 45.8 | 49.4 | 50.7 | 48.2 | 50.3 | 48.2 | 47 | 48.5 |
|
49 |
+
| AceCoder-RM-7B | 66.9 | 66.7 | 65.3 | 89.9 | 79.9 | 74.4 | 62.2 | 72.2 |
|
50 |
+
| AceCoder-RM-32B | 72.1 | 73.7 | 70.5 | 88 | 84.5 | 78.3 | 65.5 | 76.1 |
|
51 |
+
| Delta (AceCoder 7B - Others) | 7.5 | \-4.6 | \-6.1 | \-6.1 | \-9.1 | \-0.3 | 6.1 | 2.1 |
|
52 |
+
| Delta (AceCoder 32B - Others) | 12.7 | 2.4 | \-0.9 | \-8 | \-4.5 | 3.6 | 9.4 | 6 |
|
53 |
+
```
|
54 |
|
55 |
## Performance on Best-of-N sampling
|
56 |
|
|
|
62 |
|
63 |
```python
|
64 |
"""pip install git+https://github.com/TIGER-AI-Lab/AceCoder"""
|
65 |
+
from acecoder import AceCoderRM
|
66 |
from transformers import AutoTokenizer
|
67 |
|
68 |
model_path = "TIGER-Lab/AceCodeRM-7B"
|
69 |
+
model = AceCoderRM.from_pretrained(model_path, device_map="auto")
|
70 |
tokenizer = AutoTokenizer.from_pretrained(model_path, trust_remote_code=True)
|
71 |
|
72 |
question = """\
|
|
|
136 |
return_tensors="pt",
|
137 |
).to(model.device)
|
138 |
|
139 |
+
rm_scores = model(
|
140 |
**input_tokens,
|
141 |
output_hidden_states=True,
|
142 |
return_dict=True,
|
143 |
use_cache=False,
|
144 |
)
|
|
|
|
|
|
|
|
|
|
|
145 |
|
146 |
print("RM Scores:", rm_scores)
|
147 |
print("Score of program with 3 errors:", rm_scores[0].item())
|