restarted the space, and regarding the speed I found forgot to offload the model to gpu :D
try now
Csaba Kecskemeti PRO
AI & ML interests
Recent Activity
Organizations
csabakecskemeti's activity
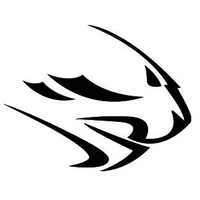
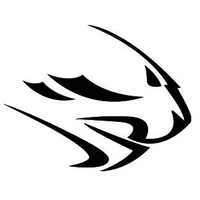
Here you can try
https://huggingface.co/spaces/DevQuasar/Mi50
Bust something seems off with my network or with HF everything is very slow.
When llama benched the model I've get 60t/s on the mi50.
Anyway you can try it.
ROCR_VISIBLE_DEVICES=0 build/bin/llama-bench -m ~/Downloads/DevQuasar-R1-Uncensored-Llama-8B.Q8_0.gguf
ggml_cuda_init: GGML_CUDA_FORCE_MMQ: no
ggml_cuda_init: GGML_CUDA_FORCE_CUBLAS: no
ggml_cuda_init: found 1 ROCm devices:
Device 0: AMD Radeon VII, compute capability 9.0, VMM: no
model | size | params | backend | ngl | test | t/s |
---|---|---|---|---|---|---|
llama 8B Q8_0 | 7.95 GiB | 8.03 B | ROCm | 99 | pp512 | 416.30 ± 0.07 |
llama 8B Q8_0 | 7.95 GiB | 8.03 B | ROCm | 99 | tg128 | 60.13 ± 0.02 |
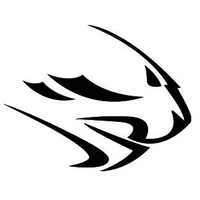
Tested with lm-evaluation-harness on standard open llm leaderboard tests + hellaswag. Scores are improved in most. Details on the model card.
Model:
DevQuasar/DevQuasar-R1-Uncensored-Llama-8B
Quants:
DevQuasar/DevQuasar-R1-Uncensored-Llama-8B-GGUF
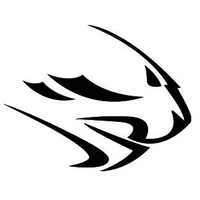
Here is the full result or the re-executed evaluation on deepseek-ai/DeepSeek-R1-Distill-Llama-8B with the suggested gen args.
I see some marginal changes in the scores but not much. If this is true the original Llama 3.1 8B wins more test than the Deepseek R1 distilled. I'm not sure what is going on. If anyone can perform the eval, please share your results.
Again I can be totally wrong here.
Full result data (results with 2025-01-26 date)
https://github.com/csabakecskemeti/lm_eval_results/blob/main/deepseek-ai__DeepSeek-R1-Distill-Llama-8B/results_2025-01-26T22-29-00.931915.json
Eval command:accelerate launch -m lm_eval --model hf --model_args pretrained=deepseek-ai/DeepSeek-R1-Distill-Llama-8B,parallelize=True,dtype="float16" --tasks hellaswag,leaderboard_gpqa,leaderboard_ifeval,leaderboard_math_hard,leaderboard_mmlu_pro,leaderboard_musr,leaderboard_bbh --batch_size auto:4 --log_samples --output_path eval_results --gen_kwargs temperature=0.6,top_p=0.95,do_sample=True
Eval output:
hf (pretrained=deepseek-ai/DeepSeek-R1-Distill-Llama-8B,parallelize=True,dtype=float16), gen_kwargs: (temperature=0.6,top_p=0.95,do_sample=True), limit: None, num_fewshot: None, batch_size: auto:4 (1,16,64,64)
Tasks | Version | Filter | n-shot | Metric | Value | Stderr | ||
---|---|---|---|---|---|---|---|---|
hellaswag | 1 | none | 0 | acc | ↑ | 0.5559 | ± | 0.0050 |
none | 0 | acc_norm | ↑ | 0.7436 | ± | 0.0044 | ||
leaderboard_bbh | N/A | |||||||
- leaderboard_bbh_boolean_expressions | 1 | none | 3 | acc_norm | ↑ | 0.8080 | ± | 0.0250 |
- leaderboard_bbh_causal_judgement | 1 | none | 3 | acc_norm | ↑ | 0.5508 | ± | 0.0365 |
- leaderboard_bbh_date_understanding | 1 | none | 3 | acc_norm | ↑ | 0.4240 | ± | 0.0313 |
- leaderboard_bbh_disambiguation_qa | 1 | none | 3 | acc_norm | ↑ | 0.2240 | ± | 0.0264 |
- leaderboard_bbh_formal_fallacies | 1 | none | 3 | acc_norm | ↑ | 0.5200 | ± | 0.0317 |
- leaderboard_bbh_geometric_shapes | 1 | none | 3 | acc_norm | ↑ | 0.2360 | ± | 0.0269 |
- leaderboard_bbh_hyperbaton | 1 | none | 3 | acc_norm | ↑ | 0.4840 | ± | 0.0317 |
- leaderboard_bbh_logical_deduction_five_objects | 1 | none | 3 | acc_norm | ↑ | 0.3240 | ± | 0.0297 |
- leaderboard_bbh_logical_deduction_seven_objects | 1 | none | 3 | acc_norm | ↑ | 0.4200 | ± | 0.0313 |
- leaderboard_bbh_logical_deduction_three_objects | 1 | none | 3 | acc_norm | ↑ | 0.4040 | ± | 0.0311 |
- leaderboard_bbh_movie_recommendation | 1 | none | 3 | acc_norm | ↑ | 0.6880 | ± | 0.0294 |
- leaderboard_bbh_navigate | 1 | none | 3 | acc_norm | ↑ | 0.6240 | ± | 0.0307 |
- leaderboard_bbh_object_counting | 1 | none | 3 | acc_norm | ↑ | 0.4040 | ± | 0.0311 |
- leaderboard_bbh_penguins_in_a_table | 1 | none | 3 | acc_norm | ↑ | 0.2945 | ± | 0.0379 |
- leaderboard_bbh_reasoning_about_colored_objects | 1 | none | 3 | acc_norm | ↑ | 0.4120 | ± | 0.0312 |
- leaderboard_bbh_ruin_names | 1 | none | 3 | acc_norm | ↑ | 0.4600 | ± | 0.0316 |
- leaderboard_bbh_salient_translation_error_detection | 1 | none | 3 | acc_norm | ↑ | 0.3440 | ± | 0.0301 |
- leaderboard_bbh_snarks | 1 | none | 3 | acc_norm | ↑ | 0.5112 | ± | 0.0376 |
- leaderboard_bbh_sports_understanding | 1 | none | 3 | acc_norm | ↑ | 0.4880 | ± | 0.0317 |
- leaderboard_bbh_temporal_sequences | 1 | none | 3 | acc_norm | ↑ | 0.2080 | ± | 0.0257 |
- leaderboard_bbh_tracking_shuffled_objects_five_objects | 1 | none | 3 | acc_norm | ↑ | 0.1800 | ± | 0.0243 |
- leaderboard_bbh_tracking_shuffled_objects_seven_objects | 1 | none | 3 | acc_norm | ↑ | 0.1040 | ± | 0.0193 |
- leaderboard_bbh_tracking_shuffled_objects_three_objects | 1 | none | 3 | acc_norm | ↑ | 0.3400 | ± | 0.0300 |
- leaderboard_bbh_web_of_lies | 1 | none | 3 | acc_norm | ↑ | 0.4880 | ± | 0.0317 |
leaderboard_gpqa | N/A | |||||||
- leaderboard_gpqa_diamond | 1 | none | 0 | acc_norm | ↑ | 0.2879 | ± | 0.0323 |
- leaderboard_gpqa_extended | 1 | none | 0 | acc_norm | ↑ | 0.3004 | ± | 0.0196 |
- leaderboard_gpqa_main | 1 | none | 0 | acc_norm | ↑ | 0.3036 | ± | 0.0217 |
leaderboard_ifeval | 3 | none | 0 | inst_level_loose_acc | ↑ | 0.4556 | ± | N/A |
none | 0 | inst_level_strict_acc | ↑ | 0.4400 | ± | N/A | ||
none | 0 | prompt_level_loose_acc | ↑ | 0.3087 | ± | 0.0199 | ||
none | 0 | prompt_level_strict_acc | ↑ | 0.2957 | ± | 0.0196 | ||
leaderboard_math_hard | N/A | |||||||
- leaderboard_math_algebra_hard | 2 | none | 4 | exact_match | ↑ | 0.4821 | ± | 0.0286 |
- leaderboard_math_counting_and_prob_hard | 2 | none | 4 | exact_match | ↑ | 0.2033 | ± | 0.0364 |
- leaderboard_math_geometry_hard | 2 | none | 4 | exact_match | ↑ | 0.2197 | ± | 0.0362 |
- leaderboard_math_intermediate_algebra_hard | 2 | none | 4 | exact_match | ↑ | 0.0750 | ± | 0.0158 |
- leaderboard_math_num_theory_hard | 2 | none | 4 | exact_match | ↑ | 0.4026 | ± | 0.0396 |
- leaderboard_math_prealgebra_hard | 2 | none | 4 | exact_match | ↑ | 0.4508 | ± | 0.0359 |
- leaderboard_math_precalculus_hard | 2 | none | 4 | exact_match | ↑ | 0.0963 | ± | 0.0255 |
leaderboard_mmlu_pro | 0.1 | none | 5 | acc | ↑ | 0.2741 | ± | 0.0041 |
leaderboard_musr | N/A | |||||||
- leaderboard_musr_murder_mysteries | 1 | none | 0 | acc_norm | ↑ | 0.5200 | ± | 0.0317 |
- leaderboard_musr_object_placements | 1 | none | 0 | acc_norm | ↑ | 0.3086 | ± | 0.0289 |
- leaderboard_musr_team_allocation | 1 | none | 0 | acc_norm | ↑ | 0.3120 | ± | 0.0294 |
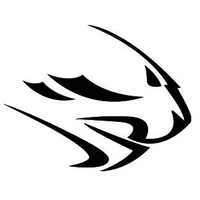
I've rerun hellaswag with the suggested config, the results haven't improved:
Tasks | Version | Filter | n-shot | Metric | Value | Stderr | ||
---|---|---|---|---|---|---|---|---|
hellaswag | 1 | none | 0 | acc | ↑ | 0.5559 | ± | 0.0050 |
none | 0 | acc_norm | ↑ | 0.7436 | ± | 0.0044 |
command:accelerate launch -m lm_eval --model hf --model_args pretrained=deepseek-ai/DeepSeek-R1-Distill-Llama-8B,parallelize=True,dtype="float16" --tasks hellaswag --batch_size auto:4 --log_samples --output_path eval_results --gen_kwargs temperature=0.6,top_p=0.95,generate_until=64,do_sample=True
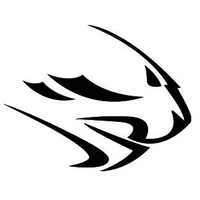
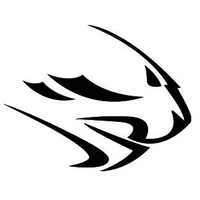
Thx, will try
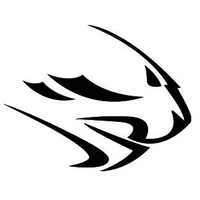
Thx, will try
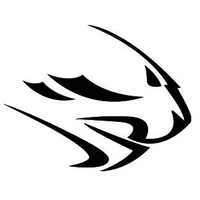
If anyone wants to double check the results are posted here:
https://github.com/csabakecskemeti/lm_eval_results
Am I made some mistake, or (at least this distilled version) not as good/better than the competition?
I'll run the same on the Qwen 7B distilled version too.
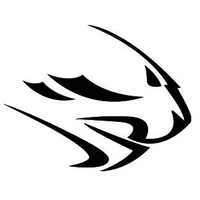
DevQuasar/nvidia-aceinstruct-and-acemath-678d716f736603ddc8d7cbd4
(some still uploading please be patient)
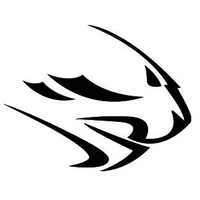
How minimalistic can I go with on device AI with behemoth models - here I'm running DeepSeek V3 MoE on a single A6000 GPU.
Not great, not terrible, for this minimalistic setup. I love the Mixture of Experts architectures. Typically I'm running my core LLM distributed over the 4 GPUs.
Make sure you own your AI. AI in the cloud is not aligned with you; it's aligned with the company that owns it.
Deepseek-V3-Base Q2_K
AMD Ryzen™ Threadripper™ 3970X × 64
ASUS ROG ZENITH II EXTREME ALPHA
256.0 GiB
NVIDIA GeForce RTX™ 3090 / NVIDIA GeForce RTX™ 3090 / NVIDIA GeForce RTX™ 4080
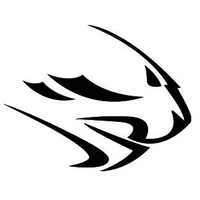
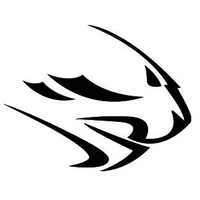
and there is the paper:
https://www.alphaxiv.org/abs/2412.18004
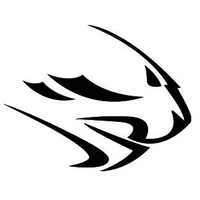
Fascinating new research from L3S Research Center, University of Amsterdam, and TU Delft reveals a critical insight into Retrieval Augmented Generation (RAG) systems. The study exposes that up to 57% of citations in RAG systems could be unfaithful, despite being technically correct.
>> Key Technical Insights:
Post-rationalization Problem
The researchers discovered that RAG systems often engage in "post-rationalization" - where models first generate answers from their parametric memory and then search for supporting evidence afterward. This means that while citations may be correct, they don't reflect the actual reasoning process.
Experimental Design
The team used Command-R+ (104B parameters) with 4-bit quantization on NVIDIA A100 GPU, testing on the NaturalQuestions dataset. They employed BM25 for initial retrieval and ColBERT v2 for reranking.
Attribution Framework
The research introduces a comprehensive framework for evaluating RAG systems across multiple dimensions:
- Citation Correctness: Whether cited documents support the claims
- Citation Faithfulness: Whether citations reflect actual model reasoning
- Citation Appropriateness: Relevance and meaningfulness of citations
- Citation Comprehensiveness: Coverage of key points
Under the Hood
The system processes involve:
1. Document relevance prediction
2. Citation prediction
3. Answer generation without citations
4. Answer generation with citations
This work fundamentally challenges our understanding of RAG systems and highlights the need for more robust evaluation metrics in AI systems that claim to provide verifiable information.
I had the same hesitation but had to settle with something, so I went with '.' :D
Basically the '.' as separata has resemble me the domain name structure which has made sense for me
Hi,
I've switched to author.model-name like 1-2 month back. Would be nice to have a standard across all quantizers.
How much you've settled with '_' as the separator?
I've carried over the convention to the filename too, that can help those just download particular files if the model name is the same.
example: https://huggingface.co/DevQuasar/tarscaleai.Llama-3.2-1B-Instruct-Product-Description-GGUF
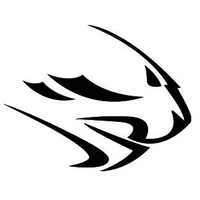
Researchers from National Chengchi University and Academia Sinica have introduced a paradigm-shifting approach that challenges the conventional wisdom of Retrieval-Augmented Generation (RAG).
Instead of the traditional retrieve-then-generate pipeline, their innovative Cache-Augmented Generation (CAG) framework preloads documents and precomputes key-value caches, eliminating the need for real-time retrieval during inference.
Technical Deep Dive:
- CAG preloads external knowledge and precomputes KV caches, storing them for future use
- The system processes documents only once, regardless of subsequent query volume
- During inference, it loads the precomputed cache alongside user queries, enabling rapid response generation
- The cache reset mechanism allows efficient handling of multiple inference sessions through strategic token truncation
Performance Highlights:
- Achieved superior BERTScore metrics compared to both sparse and dense retrieval RAG systems
- Demonstrated up to 40x faster generation times compared to traditional approaches
- Particularly effective with both SQuAD and HotPotQA datasets, showing robust performance across different knowledge tasks
Why This Matters:
The approach significantly reduces system complexity, eliminates retrieval latency, and mitigates common RAG pipeline errors. As LLMs continue evolving with expanded context windows, this methodology becomes increasingly relevant for knowledge-intensive applications.
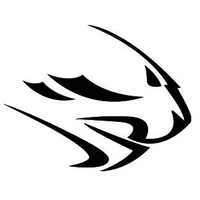
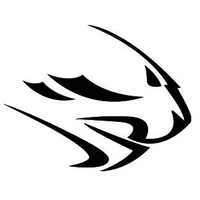
The quants are uploading (probably ~10-12hrs) here: DevQuasar/deepseek-ai.DeepSeek-V3-Base-GGUF