--- base_model: - Qwen/Qwen2-VL-7B language: - en library_name: transformers license: apache-2.0 pipeline_tag: visual-document-retrieval tags: - multimodal --- ```markdown # UGround-V1-7B (Qwen2-VL-Based) UGround is a strong GUI visual grounding model trained with a simple recipe. Check our homepage and paper for more details. This work is a collaboration between [OSUNLP](https://x.com/osunlp) and [Orby AI](https://www.orby.ai/). 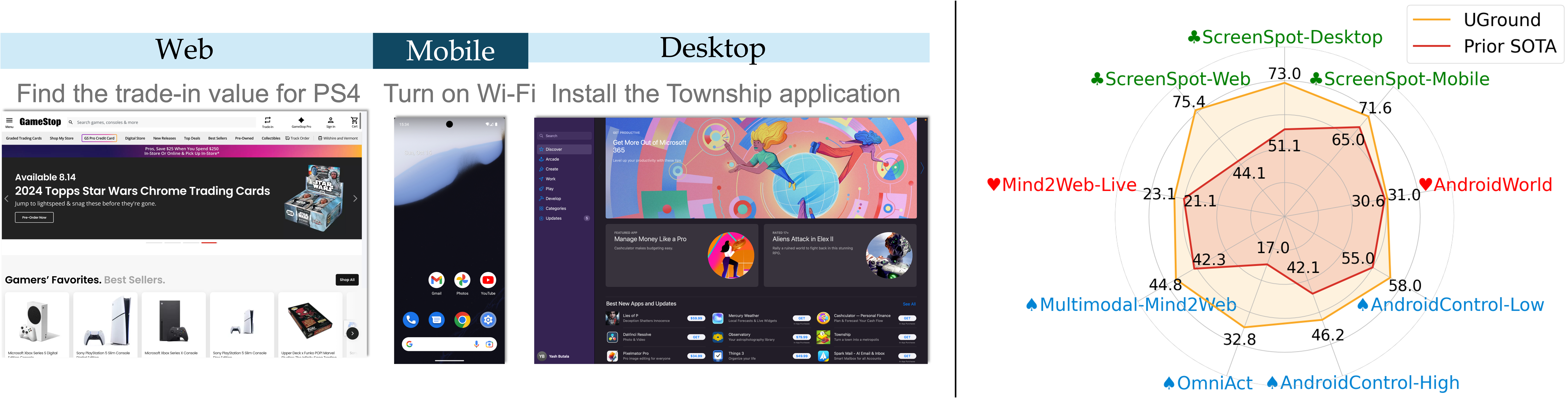 - **Homepage:** https://osu-nlp-group.github.io/UGround/ - **Repository:** https://github.com/OSU-NLP-Group/UGround - **Paper (ICLR'25 Oral):** https://arxiv.org/abs/2504.04716 - **Demo:** https://huggingface.co/spaces/orby-osu/UGround - **Point of Contact:** [Boyu Gou](mailto:gou.43@osu.edu) ## Models - Model-V1: - [Initial UGround](https://huggingface.co/osunlp/UGround): - [UGround-V1-2B (Qwen2-VL)](https://huggingface.co/osunlp/UGround-V1-2B) - [UGround-V1-7B (Qwen2-VL)](https://huggingface.co/osunlp/UGround-V1-7B) - [UGround-V1-72B (Qwen2-VL)](https://huggingface.co/osunlp/UGround-V1-72B) - [Training Data](https://huggingface.co/osunlp/UGround) ## Release Plan - [x] [Model Weights](https://huggingface.co/collections/osunlp/uground-677824fc5823d21267bc9812) - [x] Initial Version (the one used in the paper) - [x] Qwen2-VL-Based V1 - [x] 2B - [x] 7B - [x] 72B - [x] Code - [x] Inference Code of UGround (Initial & Qwen2-VL-Based - [x] Offline Experiments (Code, Results, and Useful Resources) - [x] ScreenSpot (along with referring expressions generated by GPT-4/4o) - [x] Multimodal-Mind2Web - [x] OmniAct - [x] Android Control - [x] Online Experiments - [x] Mind2Web-Live-SeeAct-V - [x] [AndroidWorld-SeeAct-V](https://github.com/boyugou/android_world_seeact_v) - [ ] Data Synthesis Pipeline (Coming Soon) - [x] Training-Data (V1) - [x] Online Demo (HF Spaces) ## Main Results ### GUI Visual Grounding: ScreenSpot (Standard Setting)  | ScreenSpot (Standard) | Arch | SFT data | Mobile-Text | Mobile-Icon | Desktop-Text | Desktop-Icon | Web-Text | Web-Icon | Avg | | ------------------------------- | ---------------- | ------------------ | ----------- | ----------- | ------------ | ------------ | -------- | -------- | -------- | | InternVL-2-4B | InternVL-2 | | 9.2 | 4.8 | 4.6 | 4.3 | 0.9 | 0.1 | 4.0 | | Groma | Groma | | 10.3 | 2.6 | 4.6 | 4.3 | 5.7 | 3.4 | 5.2 | | Qwen-VL | Qwen-VL | | 9.5 | 4.8 | 5.7 | 5.0 | 3.5 | 2.4 | 5.2 | | MiniGPT-v2 | MiniGPT-v2 | | 8.4 | 6.6 | 6.2 | 2.9 | 6.5 | 3.4 | 5.7 | | GPT-4 | | | 22.6 | 24.5 | 20.2 | 11.8 | 9.2 | 8.8 | 16.2 | | GPT-4o | | | 20.2 | 24.9 | 21.1 | 23.6 | 12.2 | 7.8 | 18.3 | | Fuyu | Fuyu | | 41.0 | 1.3 | 33.0 | 3.6 | 33.9 | 4.4 | 19.5 | | Qwen-GUI | Qwen-VL | GUICourse | 52.4 | 10.9 | 45.9 | 5.7 | 43.0 | 13.6 | 28.6 | | Ferret-UI-Llama8b | Ferret-UI | | 64.5 | 32.3 | 45.9 | 11.4 | 28.3 | 11.7 | 32.3 | | Qwen2-VL | Qwen2-VL | | 61.3 | 39.3 | 52.0 | 45.0 | 33.0 | 21.8 | 42.1 | | CogAgent | CogAgent | | 67.0 | 24.0 | 74.2 | 20.0 | 70.4 | 28.6 | 47.4 | | SeeClick | Qwen-VL | SeeClick | 78.0 | 52.0 | 72.2 | 30.0 | 55.7 | 32.5 | 53.4 | | OS-Atlas-Base-4B | InternVL-2 | OS-Atlas | 85.7 | 58.5 | 72.2 | 45.7 | 82.6 | 63.1 | 68.0 | | OmniParser | | | 93.9 | 57.0 | 91.3 | 63.6 | 81.3 | 51.0 | 73.0 | | **UGround** | LLaVA-UGround-V1 | UGround-V1 | 82.8 | **60.3** | 82.5 | **63.6** | 80.4 | **70.4** | **73.3** | | Iris | Iris | SeeClick | 85.3 | 64.2 | 86.7 | 57.5 | 82.6 | 71.2 | 74.6 | | ShowUI-G | ShowUI | ShowUI | 91.6 | 69.0 | 81.8 | 59.0 | 83.0 | 65.5 | 75.0 | | ShowUI | ShowUI | ShowUI | 92.3 | 75.5 | 76.3 | 61.1 | 81.7 | 63.6 | 75.1 | | Molmo-7B-D | | | 85.4 | 69.0 | 79.4 | 70.7 | 81.3 | 65.5 | 75.2 | | **UGround-V1-2B (Qwen2-VL)** | Qwen2-VL | UGround-V1 | 89.4 | 72.0 | 88.7 | 65.7 | 81.3 | 68.9 | 77.7 | | Molmo-72B | | | 92.7 | 79.5 | 86.1 | 64.3 | 83.0 | 66.0 | 78.6 | | Aguvis-G-7B | Qwen2-VL | Aguvis-Stage-1 | 88.3 | 78.2 | 88.1 | 70.7 | 85.7 | 74.8 | 81.0 | | OS-Atlas-Base-7B | Qwen2-VL | OS-Atlas | 93.0 | 72.9 | 91.8 | 62.9 | 90.9 | 74.3 | 81.0 | | Aria-UI | Aria | Aria-UI | 92.3 | 73.8 | 93.3 | 64.3 | 86.5 | 76.2 | 81.1 | | Claude (Computer-Use) | | | **98.2** | **85.6** | 79.9 | 57.1 | **92.2** | **84.5** | 82.9 | | Aguvis-7B | Qwen2-VL | Aguvis-Stage-1&2 | 95.6 | 77.7 | **93.8** | 67.1 | 88.3 | 75.2 | 83.0 | | Project Mariner | | | | | | | | | 84.0 | | **UGround-V1-7B (Qwen2-VL)** | Qwen2-VL | UGround-V1 | 93.0 | 79.9 | **93.8** | **76.4** | 90.9 | 84.0 | **86.3** | | *AGUVIS-72B* | *Qwen2-VL* | *Aguvis-Stage-1&2* | *94.5* | *85.2* | *95.4* | *77.9* | *91.3* | *85.9* | *88.4* | | ***UGround-V1-72B (Qwen2-VL)*** | *Qwen2-VL* | *UGround-V1* | *94.1* | *83.4* | *94.9* | *85.7* | *90.4* | *87.9* | *89.4* | ### GUI Visual Grounding: ScreenSpot (Agent Setting) | Planner | Agent-Screenspot | arch | SFT data | Mobile-Text | Mobile-Icon | Desktop-Text | Desktop-Icon | Web-Text | Web-Icon | Avg | | ------- | ---------------------------- | ---------------- | ---------------- | ----------- | ----------- | ------------ | ------------ | -------- | -------- | -------- | | GPT-4o | Qwen-VL | Qwen-VL | | 21.3 | 21.4 | 18.6 | 10.7 | 9.1 | 5.8 | 14.5 | | GPT-4o | Qwen-GUI | Qwen-VL | GUICourse | 67.8 | 24.5 | 53.1 | 16.4 | 50.4 | 18.5 | 38.5 | | GPT-4o | SeeClick | Qwen-VL | SeeClick | 81.0 | 59.8 | 69.6 | 33.6 | 43.9 | 26.2 | 52.4 | | GPT-4o | OS-Atlas-Base-4B | InternVL-2 | OS-Atlas | **94.1** | 73.8 | 77.8 | 47.1 | 86.5 | 65.3 | 74.1 | | GPT-4o | OS-Atlas-Base-7B | Qwen2-VL | OS-Atlas | 93.8 | **79.9** | 90.2 | 66.4 | **92.6** | **79.1** | 83.7 | | GPT-4o | **UGround-V1** | LLaVA-UGround-V1 | UGround-V1 | 93.4 | 76.9 | 92.8 | 67.9 | 88.7 | 68.9 | 81.4 | | GPT-4o | **UGround-V1-2B (Qwen2-VL)** | Qwen2-VL | UGround-V1 | **94.1** | 77.7 | 92.8 | 63.6 | 90.0 | 70.9 | 81.5 | | GPT-4o | **UGround-V1-7B (Qwen2-VL)** | Qwen2-VL | UGround-V1 | **94.1** | **79.9** | **93.3** | **73.6** | 89.6 | 73.3 | **84.0** | ## Inference ### vLLM server ```bash vllm serve osunlp/UGround-V1-7B --api-key token-abc123 --dtype float16 ``` or ```bash python -m vllm.entrypoints.openai.api_server --served-model-name osunlp/UGround-V1-7B --model osunlp/UGround-V1-7B --dtype float16 ``` You can find more instruction about training and inference in [Qwen2-VL's Official Repo](https://github.com/QwenLM/Qwen2-VL). ### Visual Grounding Prompt ```python def format_openai_template(description: str, base64_image): return [ { "role": "user", "content": [ { "type": "image_url", "image_url": {"url": f"data:image/jpeg;base64,{base64_image}"}, }, { "type": "text", "text": f""" Your task is to help the user identify the precise coordinates (x, y) of a specific area/element/object on the screen based on a description. - Your response should aim to point to the center or a representative point within the described area/element/object as accurately as possible. - If the description is unclear or ambiguous, infer the most relevant area or element based on its likely context or purpose. - Your answer should be a single string (x, y) corresponding to the point of the interest. Description: {description} Answer:""" }, ], }, ] messages = format_openai_template(description, base64_image) completion = await client.chat.completions.create( model=args.model_path, messages=messages, temperature=0 # REMEMBER to set temperature to ZERO! # REMEMBER to set temperature to ZERO! # REMEMBER to set temperature to ZERO! ) # The output will be in the range of [0,1000), which is compatible with the original Qwen2-VL # So the actual coordinates should be (x/1000*width, y/1000*height) ```  ## Citation Information If you find this work useful, please consider citing our papers: ``` @article{gou2024uground, title={Navigating the Digital World as Humans Do: Universal Visual Grounding for GUI Agents}, author={Boyu Gou and Ruohan Wang and Boyuan Zheng and Yanan Xie and Cheng Chang and Yiheng Shu and Huan Sun and Yu Su}, journal={arXiv preprint arXiv:2410.05243}, year={2024}, url={https://arxiv.org/abs/2410.05243}, } @article{zheng2023seeact, title={GPT-4V(ision) is a Generalist Web Agent, if Grounded}, author={Boyuan Zheng and Boyu Gou and Jihyung Kil and Huan Sun and Yu Su}, journal={arXiv preprint arXiv:2401.01614}, year={2024}, } ``` # Qwen2-VL-7B-Instruct ## Introduction We're excited to unveil **Qwen2-VL**, the latest iteration of our Qwen-VL model, representing nearly a year of innovation. ### What’s New in Qwen2-VL? #### Key Enhancements: * **SoTA understanding of images of various resolution & ratio**: Qwen2-VL achieves state-of-the-art performance on visual understanding benchmarks, including MathVista, DocVQA, RealWorldQA, MTVQA, etc. * **Understanding videos of 20min+**: Qwen2-VL can understand videos over 20 minutes for high-quality video-based question answering, dialog, content creation, etc. * **Agent that can operate your mobiles, robots, etc.**: with the abilities of complex reasoning and decision making, Qwen2-VL can be integrated with devices like mobile phones, robots, etc., for automatic operation based on visual environment and text instructions. * **Multilingual Support**: to serve global users, besides English and Chinese, Qwen2-VL now supports the understanding of texts in different languages inside images, including most European languages, Japanese, Korean, Arabic, Vietnamese, etc. #### Model Architecture Updates: * **Naive Dynamic Resolution**: Unlike before, Qwen2-VL can handle arbitrary image resolutions, mapping them into a dynamic number of visual tokens, offering a more human-like visual processing experience.
* **Multimodal Rotary Position Embedding (M-ROPE)**: Decomposes positional embedding into parts to capture 1D textual, 2D visual, and 3D video positional information, enhancing its multimodal processing capabilities.
We have three models with 2, 7 and 72 billion parameters. This repo contains the instruction-tuned 7B Qwen2-VL model. For more information, visit our [Blog](https://qwenlm.github.io/blog/qwen2-vl/) and [GitHub](https://github.com/QwenLM/Qwen2-VL).
## Evaluation
### Image Benchmarks
| Benchmark | InternVL2-8B | MiniCPM-V 2.6 | GPT-4o-mini | **Qwen2-VL-7B** |
| :--- | :---: | :---: | :---: | :---: |
| MMMUval | 51.8 | 49.8 | **60**| 54.1 |
| DocVQAtest | 91.6 | 90.8 | - | **94.5** |
| InfoVQAtest | 74.8 | - | - |**76.5** |
| ChartQAtest | **83.3** | - |- | 83.0 |
| TextVQAval | 77.4 | 80.1 | -| **84.3** |
| OCRBench | 794 | **852** | 785 | 845 |
| MTVQA | - | - | -| **26.3** |
| VCRen easy | - | 73.88 | 83.60 | **89.70** |
| VCRzh easy | - | 10.18| 1.10 | **59.94** |
| RealWorldQA | 64.4 | - | - | **70.1** |
| MMEsum | 2210.3 | **2348.4** | 2003.4| 2326.8 |
| MMBench-ENtest | 81.7 | - | - | **83.0** |
| MMBench-CNtest | **81.2** | - | - | 80.5 |
| MMBench-V1.1test | 79.4 | 78.0 | 76.0| **80.7** |
| MMT-Benchtest | - | - | - |**63.7** |
| MMStar | **61.5** | 57.5 | 54.8 | 60.7 |
| MMVetGPT-4-Turbo | 54.2 | 60.0 | **66.9** | 62.0 |
| HallBenchavg | 45.2 | 48.1 | 46.1| **50.6** |
| MathVistatestmini | 58.3 | **60.6** | 52.4 | 58.2 |
| MathVision | - | - | - | **16.3** |
### Video Benchmarks
| Benchmark | Internvl2-8B | LLaVA-OneVision-7B | MiniCPM-V 2.6 | **Qwen2-VL-7B** |
| :--- | :---: | :---: | :---: | :---: |
| MVBench | 66.4 | 56.7 | - | **67.0** |
| PerceptionTesttest | - | 57.1 | - | **62.3** |
| EgoSchematest | - | 60.1 | - | **66.7** |
| Video-MMEwo/w subs | 54.0/56.9 | 58.2/- | 60.9/63.6 | **63.3**/**69.0** |
## Requirements
The code of Qwen2-VL has been in the latest Hugging face transformers and we advise you to build from source with command `pip install git+https://github.com/huggingface/transformers`, or you might encounter the following error:
```
KeyError: 'qwen2_vl'
```
## Quickstart
We offer a toolkit to help you handle various types of visual input more conveniently. This includes base64, URLs, and interleaved images and videos. You can install it using the following command:
```bash
pip install qwen-vl-utils
```
Here we show a code snippet to show you how to use the chat model with `transformers` and `qwen_vl_utils`:
```python
from transformers import Qwen2VLForConditionalGeneration, AutoTokenizer, AutoProcessor
from qwen_vl_utils import process_vision_info
# default: Load the model on the available device(s)
model = Qwen2VLForConditionalGeneration.from_pretrained(
"Qwen/Qwen2-VL-7B-Instruct", torch_dtype="auto", device_map="auto"
)
# We recommend enabling flash_attention_2 for better acceleration and memory saving, especially in multi-image and video scenarios.
# model = Qwen2VLForConditionalGeneration.from_pretrained(
# "Qwen/Qwen2-VL-7B-Instruct",
# torch_dtype=torch.bfloat16,
# attn_implementation="flash_attention_2",
# device_map="auto",
# )
# default processer
processor = AutoProcessor.from_pretrained("Qwen/Qwen2-VL-7B-Instruct")
# The default range for the number of visual tokens per image in the model is 4-16384. You can set min_pixels and max_pixels according to your needs, such as a token count range of 256-1280, to balance speed and memory usage.
# min_pixels = 256*28*28
# max_pixels = 1280*28*28
# processor = AutoProcessor.from_pretrained("Qwen/Qwen2-VL-7B-Instruct", min_pixels=min_pixels, max_pixels=max_pixels)
messages = [
{
"role": "user",
"content": [
{
"type": "image",
"image": "https://qianwen-res.oss-cn-beijing.aliyuncs.com/Qwen-VL/assets/demo.jpeg",
},
{"type": "text", "text": "Describe this image."},
],
}
]
# Preparation for inference
text = processor.apply_chat_template(
messages, tokenize=False, add_generation_prompt=True
)
image_inputs, video_inputs = process_vision_info(messages)
inputs = processor(
text=[text],
images=image_inputs,
videos=video_inputs,
padding=True,
return_tensors="pt",
)
inputs = inputs.to("cuda")
# Inference: Generation of the output
generated_ids = model.generate(**inputs, max_new_tokens=128)
generated_ids_trimmed = [
out_ids[len(in_ids) :] for in_ids, out_ids in zip(inputs.input_ids, generated_ids)
]
output_text = processor.batch_decode(
generated_ids_trimmed, skip_special_tokens=True, clean_up_tokenization_spaces=False
)
print(output_text)
```
Without qwen_vl_utils
```python
from PIL import Image
import requests
import torch
from torchvision import io
from typing import Dict
from transformers import Qwen2VLForConditionalGeneration, AutoTokenizer, AutoProcessor
# Load the model in half-precision on the available device(s)
model = Qwen2VLForConditionalGeneration.from_pretrained(
"Qwen/Qwen2-VL-7B-Instruct", torch_dtype="auto", device_map="auto"
)
processor = AutoProcessor.from_pretrained("Qwen/Qwen2-VL-7B-Instruct")
# Image
url = "https://qianwen-res.oss-cn-beijing.aliyuncs.com/Qwen-VL/assets/demo.jpeg"
image = Image.open(requests.get(url, stream=True).raw)
conversation = [
{
"role": "user",
"content": [
{
"type": "image",
},
{"type": "text", "text": "Describe this image."},
],
}
]
# Preprocess the inputs
text_prompt = processor.apply_chat_template(conversation, add_generation_prompt=True)
# Excepted output: '<|im_start|>system
You are a helpful assistant.<|im_end|>
<|im_start|>user
<|vision_start|><|image_pad|><|vision_end|>Describe this image.<|im_end|>
<|im_start|>assistant
'
inputs = processor(
text=[text_prompt], images=[image], padding=True, return_tensors="pt"
)
inputs = inputs.to("cuda")
# Inference: Generation of the output
output_ids = model.generate(**inputs, max_new_tokens=128)
generated_ids = [
out_ids[len(input_ids) :]
for input_ids, output_ids in zip(inputs.input_ids, output_ids)
]
output_text = processor.batch_decode(
generated_ids, skip_special_tokens=True, clean_up_tokenization_spaces=True
)
print(output_text)
```
Multi image inference
```python
# Messages containing multiple images and a text query
messages = [
{
"role": "user",
"content": [
{"type": "image", "image": "file:///path/to/image1.jpg"},
{"type": "image", "image": "file:///path/to/image2.jpg"},
{"type": "text", "text": "What are the common elements in these pictures?"},
],
}
]
# Preparation for inference
text = processor.apply_chat_template(
messages, tokenize=False, add_generation_prompt=True
)
image_inputs, video_inputs = process_vision_info(messages)
inputs = processor(
text=[text],
images=image_inputs,
videos=video_inputs,
padding=True,
return_tensors="pt",
)
inputs = inputs.to("cuda")
# Inference
generated_ids = model.generate(**inputs, max_new_tokens=128)
generated_ids_trimmed = [
out_ids[len(in_ids) :] for in_ids, out_ids in zip(inputs.input_ids, generated_ids)
]
output_text = processor.batch_decode(
generated_ids_trimmed, skip_special_tokens=True, clean_up_tokenization_spaces=False
)
print(output_text)
```
Video inference
```python
# Messages containing a images list as a video and a text query
messages = [
{
"role": "user",
"content": [
{
"type": "video",
"video": [
"file:///path/to/frame1.jpg",
"file:///path/to/frame2.jpg",
"file:///path/to/frame3.jpg",
"file:///path/to/frame4.jpg",
],
"fps": 1.0,
},
{"type": "text", "text": "Describe this video."},
],
}
]
# Messages containing a video and a text query
messages = [
{
"role": "user",
"content": [
{
"type": "video",
"video": "file:///path/to/video1.mp4",
"max_pixels": 360 * 420,
"fps": 1.0,
},
{"type": "text", "text": "Describe this video."},
],
}
]
# Preparation for inference
text = processor.apply_chat_template(
messages, tokenize=False, add_generation_prompt=True
)
image_inputs, video_inputs = process_vision_info(messages)
inputs = processor(
text=[text],
images=image_inputs,
videos=video_inputs,
padding=True,
return_tensors="pt",
)
inputs = inputs.to("cuda")
# Inference
generated_ids = model.generate(**inputs, max_new_tokens=128)
generated_ids_trimmed = [
out_ids[len(in_ids) :] for in_ids, out_ids in zip(inputs.input_ids, generated_ids)
]
output_text = processor.