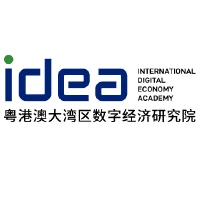
Zero-Shot Object Detection
Zero-shot object detection is a computer vision task to detect objects and their classes in images, without any prior training or knowledge of the classes. Zero-shot object detection models receive an image as input, as well as a list of candidate classes, and output the bounding boxes and labels where the objects have been detected.
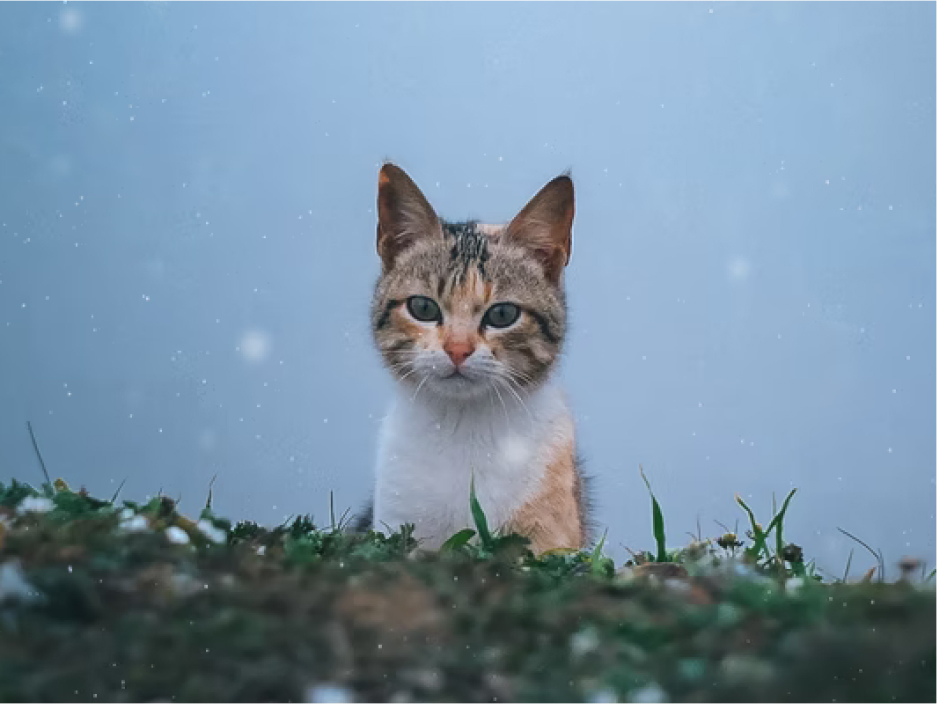
Classes
cat, dog, bird
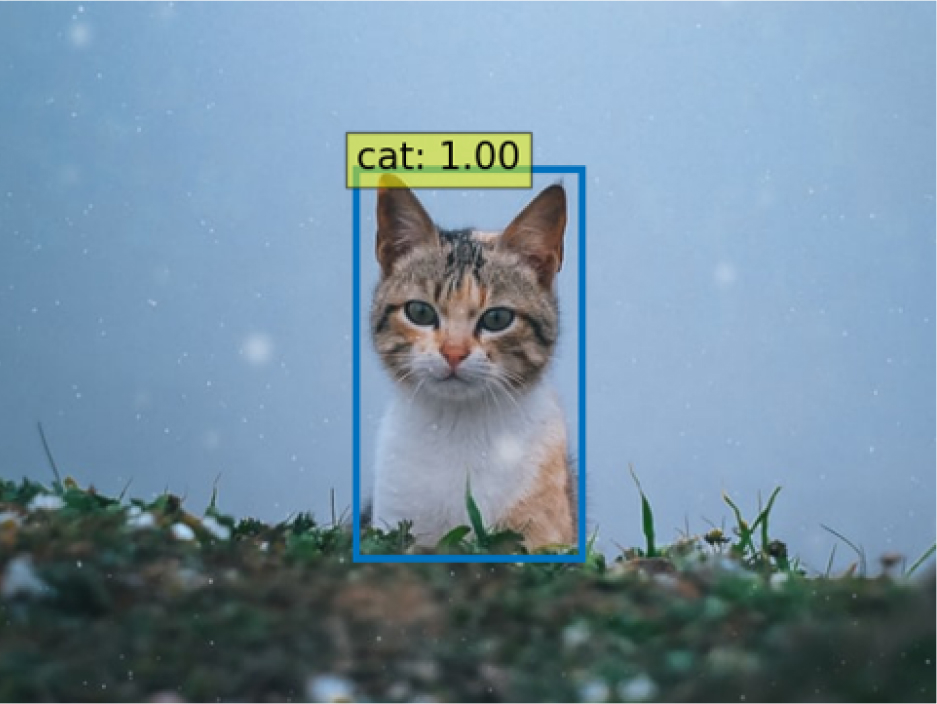
About Zero-Shot Object Detection
Use Cases
Zero-shot object detection models can be used in any object detection application where the detection involves text queries for objects of interest.
Object Search
Zero-shot object detection models can be used in image search. Smartphones, for example, use zero-shot object detection models to detect entities (such as specific places or objects) and allow the user to search for the entity on the internet.
Object Counting
Zero-shot object detection models are used to count instances of objects in a given image. This can include counting the objects in warehouses or stores or the number of visitors in a store. They are also used to manage crowds at events to prevent disasters.
Object Tracking
Zero-shot object detectors can track objects in videos.
Inference
You can infer with zero-shot object detection models through the zero-shot-object-detection
pipeline. When calling the pipeline, you just need to specify a path or HTTP link to an image and the candidate labels.
from transformers import pipeline
from PIL import Image
image = Image.open("my-image.png").convert("RGB")
detector = pipeline(model="google/owlvit-base-patch32", task="zero-shot-object-detection")
predictions = detector(
image,
candidate_labels=["a photo of a cat", "a photo of a dog"],
)
# [{'score': 0.95,
# 'label': 'a photo of a cat',
# 'box': {'xmin': 180, 'ymin': 71, 'xmax': 271, 'ymax': 178}},
# ...
# ]
Useful Resources
This page was made possible thanks to the efforts of Victor Guichard
Compatible libraries
No example widget is defined for this task.
Note Contribute by proposing a widget for this task !
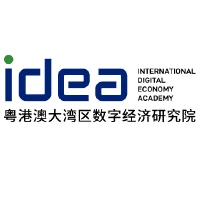
Note Solid zero-shot object detection model.
Note Cutting-edge zero-shot object detection model.
No example dataset is defined for this task.
Note Contribute by proposing a dataset for this task !
Note A demo to try the state-of-the-art zero-shot object detection model, OWLv2.
Note A demo that combines a zero-shot object detection and mask generation model for zero-shot segmentation.
- Average Precision
- The Average Precision (AP) metric is the Area Under the PR Curve (AUC-PR). It is calculated for each class separately
- Mean Average Precision
- The Mean Average Precision (mAP) metric is the overall average of the AP values
- APα
- The APα metric is the Average Precision at the IoU threshold of a α value, for example, AP50 and AP75