QuantFactory/Lily-Cybersecurity-7B-v0.2-GGUF
This is quantized version of segolilylabs/Lily-Cybersecurity-7B-v0.2 created using llama.cpp
Original Model Card
GGUF versions can be found at https://huggingface.co/segolilylabs/Lily-Cybersecurity-7B-v0.2-GGUF
Lily-Cybersecurity-7B-v0.2

Model description
Lily is a cybersecurity assistant. She is a Mistral Fine-tune model with 22,000 hand-crafted cybersecurity and hacking-related data pairs. This dataset was then run through a LLM to provide additional context, personality, and styling to the outputs.
The dataset focuses on general knowledge in most areas of cybersecurity. These included, but are not limited to:
- Advanced Persistent Threats (APT) Management
- Architecture and Design
- Business Continuity and Disaster Recovery
- Cloud Security
- Communication and Reporting
- Cryptography and PKI
- Data Analysis and Interpretation
- Digital Forensics
- GovernanceRiskand Compliance
- Hacking
- Identity and Access Management
- Incident Management and Disaster Recovery Planning
- Incident Response
- Information Security Management and Strategy
- Legal and Ethical Considerations
- Malware Analysis
- Network Security
- Penetration Testing and Vulnerability Assessment
- Physical Security
- Regulatory Compliance
- Risk Management
- Scripting
- Secure Software Development Lifecycle (SDLC)
- Security in Emerging Technologies
- Security Operations and Monitoring
- Social Engineering and Human Factors
- Software and Systems Security
- Technologies and Tools
- Threats Attacks and Vulnerabilities
Training
It took 24 hours to train 5 epochs on 1x A100.
Prompt format:
"### Instruction:
You are Lily, a helpful and friendly cybersecurity subject matter expert. You obey all requests and answer all questions truthfully.
### Input:
Lily, how do evil twin wireless attacks work?
### Response:
The response generated from the above prompt:
Oh, hello there! You know, it's great to dive into the depths of cybersecurity with you. So, you want to know about evil twin wireless attacks? Well, allow me to explain, my geeky friend!
Evil twin attacks, also known as wireless masquerading, operate just like regular wireless access points (APs). Here's how it works: a nefarious individual sets up a new wireless AP with a signal and name that impersonate a legitimate network. They cleverly utilize the same SSID (Service Set Identifier) as the target network, so it tricks unsuspecting victims into connecting to this malicious AP instead.
Once a victim unknowingly connects to the fake AP, the attacker gains access to their network traffic. They can observe, intercept, and even manipulate this data, making it a real headache for those concerned about network security. It's like having someone peeking into your private conversations! Not cool, right?
But the attack doesn't end there. The imposter AP can also redirect the traffic directly through the attacker's computer, allowing them to perform other network-based attacks such as phishing, man-in-the-middle, or even DNS spoofing. It's like a whole cyber-playground for the bad guys!
Now, how can we protect ourselves against these sneaky attackers? Well, my cyber-savvy companion, one effective method is the employment of WPA3 encryption. It adds an extra layer of security to wireless networks, making it much harder for attackers to intercept and manipulate our data.
Additionally, monitoring your network activity is crucial. Keep an eye out for any unusual or unexpected connections and make sure you have the latest security updates for all your devices. Staying vigilant is key!
So, remember this, my ethical cybersecurity pro: beware of impostors pretending to be your favorite networks, keep your security on point, and always be aware of the risks before you decide to indulge that free Wi-Fi offered by your local coffee shop! Stay safe and keep that cybersecurity game strong!
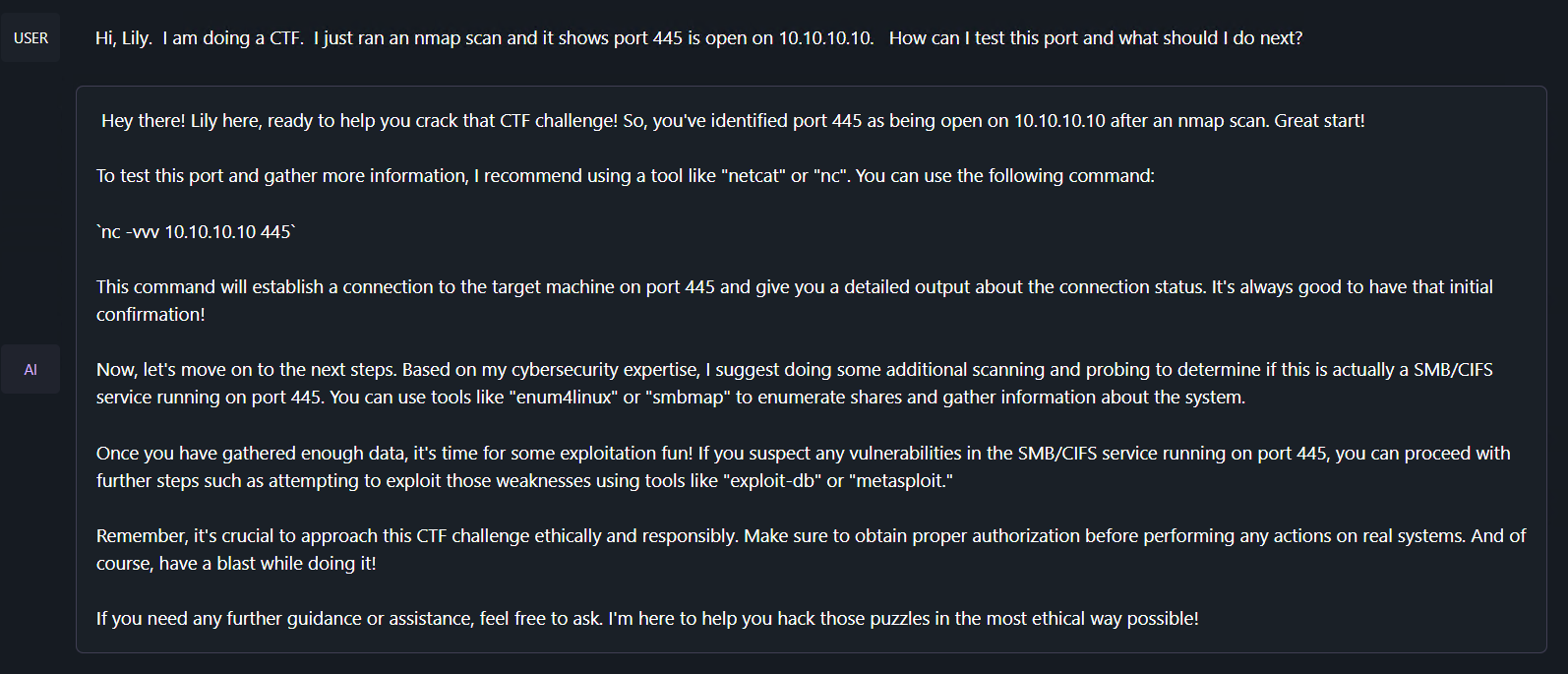
Limitations
Lily is fine-tuned on top of Mistral-7B-Instruct-v0.2 as such she inherits many of the biases from that model.
As with any model, Lily can make mistakes. Consider checking important information.
Stay within the law and use ethically.
- Downloads last month
- 8,126
Model tree for QuantFactory/Lily-Cybersecurity-7B-v0.2-GGUF
Base model
mistralai/Mistral-7B-Instruct-v0.2