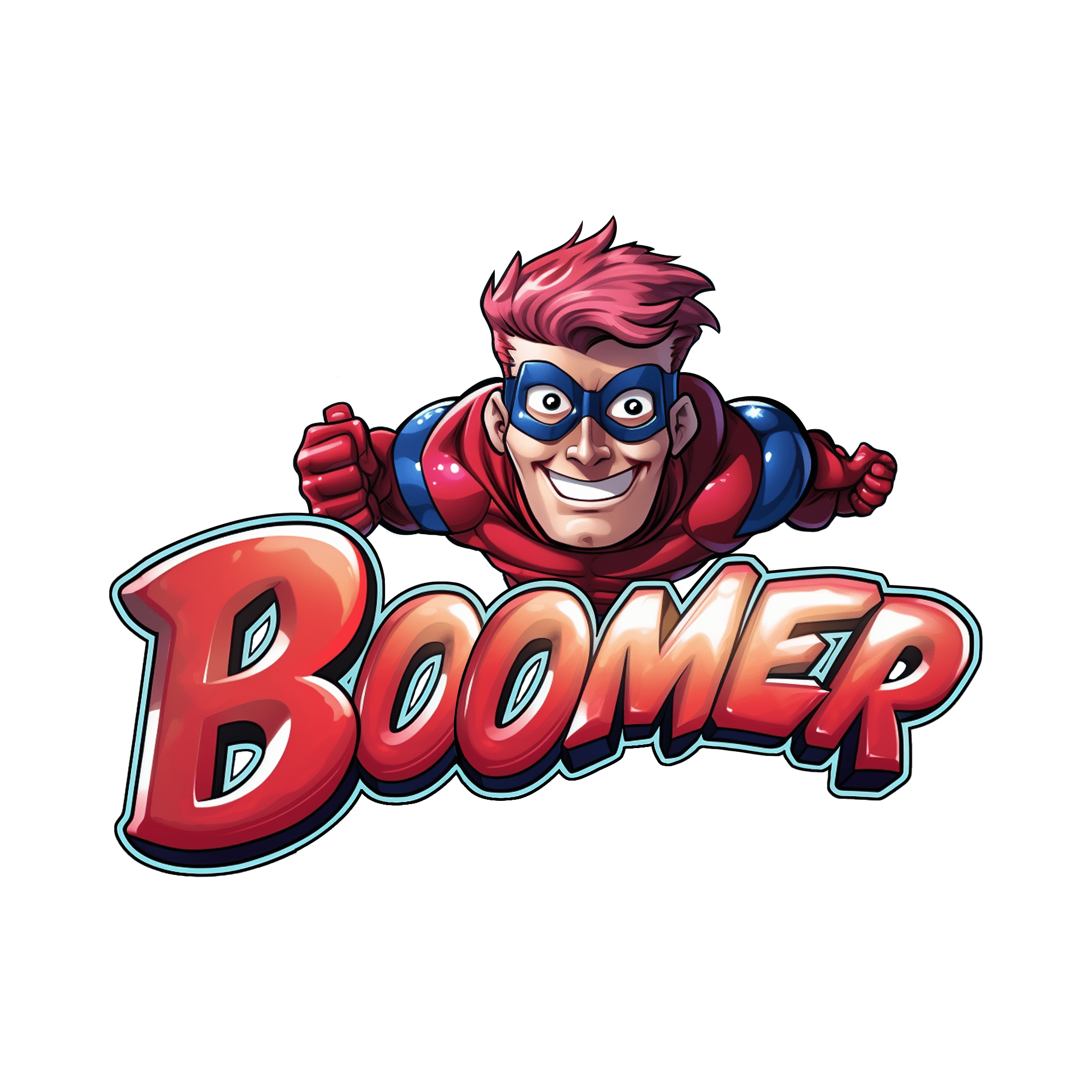
Democratizing access to LLMs for the open-source community.
Let's advance AI, together.
Introduction π
We are open-sourcing one of our early experiments of BitNet b1.58 paper. This 634m parameter model is pre-trained from scratch using a custom synthetic dataset of 5B tokens. The model's architecture experiments contain the modification of using higher depth and shallow configuration
Run the model
Please note that, at the moment, trust_remote_code=True
is required for running the model.
from transformers import AutoModelForCausalLM, AutoTokenizer
model = AutoModelForCausalLM.from_pretrained("budecosystem/boomer-bitnet-634m",
trust_remote_code=True)
tokenizer = AutoTokenizer.from_pretrained("budecosystem/boomer-bitnet-634m")
input_ids = tokenizer("In the recent Super Bowl LVIII,", return_tensors='pt').to(model.device)["input_ids"]
outputs = model.generate(input_ids, max_new_tokens=216)
print(tokenizer.batch_decode(outputs))
Evaluations
We have evaluated the pre-trained model on few of the benchmarks
Model Name | ARC | MMLU | Winogrande | Hellaswag | MathQA | GSM8K |
---|---|---|---|---|---|---|
boomer-bitnet-634m | 26.19 | 25.23 | 51.07 | 34.08 | 23.38 | 0.91 |
Final thought on Boomer!
This isn't the end. It's just the beginning of a journey towards creating more advanced, more efficient, and more accessible language models. We invite you to join us on this exciting journey.
Aknowledgements
We'd like to thank the open-source community and the researchers whose foundational work laid the path for BOOMER. Special shoutout to team who published BitNet b1.58 paper.
- Downloads last month
- 2