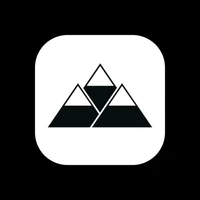
Curated by: AtlasIA members: Abdelaziz Bounhar, Abdeljalil Elmajoudi, Imane Momayiz.
Overview: This is a compilation of text written in MSA and multiple Arabic dialects from various sources. The dialects include Moroccan, Algerian, Egyptian, Levantine, Middle-Eastern and others. This dataset was used to train the Moroccan language identifier.
The dataset is designed to enable research and development in tasks such as language identification, dialect classification, and natural language processing (NLP) for Arabic text. It was specifically used to train and evaluate a Moroccan dialect identifier, achieving significant performance improvements in distinguishing Moroccan Arabic from other dialects.
Key Features
- Diversity of Dialects: Text samples span a wide range of Arabic dialects, ensuring coverage of both regional and cultural variations.
- Inclusion of MSA: Provides a strong baseline for comparing dialectal variation with Modern Standard Arabic.
- High-Quality Sources: Data is curated from various reliable sources such as social media, news articles, and informal conversations to reflect real-world language usage.
Dataset Format
The dataset is provided in a tabular format, with the following fields:
text
: The text content in Arabic.dialect
: The dialect label (e.g., "Moroccan," "Egyptian," "MSA", etc).dataset_source
: The original dataset of the text.metadata
: Some metadata from the original dataset.
Usage
This dataset is versatile and supports a variety of NLP applications, including but not limited to:
- Dialect Classification: Differentiating between various Arabic dialects.
- Words embeddings: Training an embedding for Arabic dialects.
Curation Process
- Source Collection: Text samples were gathered from a mix of online sources, ensuring diverse representation of dialects.
- Cleaning and Preprocessing: Text data underwent rigorous preprocessing to remove noise, normalize content, and ensure quality.
Limitations
- Dialect Overlap: Some dialects share linguistic similarities, which may introduce classification challenges.
- Bias: The dataset may reflect biases inherent in its sources, such as regional or topical bias.
Ethical Considerations
- Privacy: No personally identifiable information (PII) is included in the dataset.
- Fair Use: The dataset is intended for research and educational purposes only.
- Cultural Sensitivity: Efforts were made to ensure cultural and linguistic respect in curating the data.
Acknowledgments
Special thanks to contributors, annotators, and the Arabic NLP community for their support in developing this resource.
Future works
- Balanced Distribution: We are seeking better proportions of data across dialects are balanced to support fair model training.
- Downloads last month
- 37
Models trained or fine-tuned on atlasia/No-Arabic-Dialect-Left-Behind
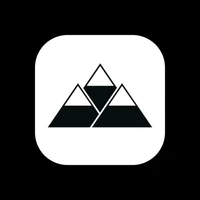