|
--- |
|
license: mit |
|
datasets: |
|
- hpprc/emb |
|
- hotchpotch/hpprc_emb-scores |
|
- microsoft/ms_marco |
|
- hotchpotch/japanese-splade-v1-hard-negatives |
|
- hpprc/msmarco-ja |
|
language: |
|
- ja |
|
base_model: |
|
- cl-nagoya/ruri-v3-pt-30m |
|
library_name: sentence-transformers |
|
pipeline_tag: text-ranking |
|
--- |
|
|
|
# hotchpotch/japanese-reranker-xsmall-v2 |
|
|
|
とても小さく速い日本語リランカーモデルシリーズ(v2)です。 |
|
|
|
| モデル名 | レイヤー数 | 隠れ層サイズ | スコア(avg) | 速度(GPU) | |
|
| --- | --- | --- | --- | --- | |
|
| [hotchpotch/japanese-reranker-tiny-v2](https://huggingface.co/hotchpotch/japanese-reranker-tiny-v2) | 3 | 256 | 0.8138 | 2.1s | |
|
| [hotchpotch/japanese-reranker-xsmall-v2](https://huggingface.co/hotchpotch/japanese-reranker-xsmall-v2) | 10 | 256 | 0.8699 | 6.5s | |
|
| [hotchpotch/japanese-reranker-cross-encoder-xsmall-v1](https://huggingface.co/hotchpotch/japanese-reranker-cross-encoder-xsmall-v1) | 6 | 384 | 0.8131 | 20.5s | |
|
| [hotchpotch/japanese-reranker-cross-encoder-small-v1](https://huggingface.co/hotchpotch/japanese-reranker-cross-encoder-small-v1) | 12 | 384 | 0.8254 | 40.3s | |
|
| [hotchpotch/japanese-reranker-cross-encoder-base-v1](https://huggingface.co/hotchpotch/japanese-reranker-cross-encoder-base-v1) | 12 | 768 | 0.8484 | 96.8s | |
|
| [hotchpotch/japanese-reranker-cross-encoder-large-v1](https://huggingface.co/hotchpotch/japanese-reranker-cross-encoder-large-v1) | 24 | 1024 | 0.8661 | 312.2s | |
|
| [hotchpotch/japanese-bge-reranker-v2-m3-v1](https://huggingface.co/hotchpotch/japanese-bge-reranker-v2-m3-v1) | 24 | 1024 | 0.8584 | 310.6s | |
|
|
|
|
|
リランカーについてや、技術レポート・評価等は以下を参考ください。 |
|
|
|
- [とても小さく速く実用的な日本語リランカー japanese-reranker-tiny,xsmall v2 を公開](https://secon.dev/entry/2025/05/08/100000-japanese-reranker-v2/) |
|
- [日本語最高性能のRerankerをリリース / そもそも Reranker とは?](https://secon.dev/entry/2024/04/02/070000-japanese-reranker-release/) |
|
- [日本語 Reranker 作成のテクニカルレポート](https://secon.dev/entry/2024/04/02/080000-japanese-reranker-tech-report/) |
|
|
|
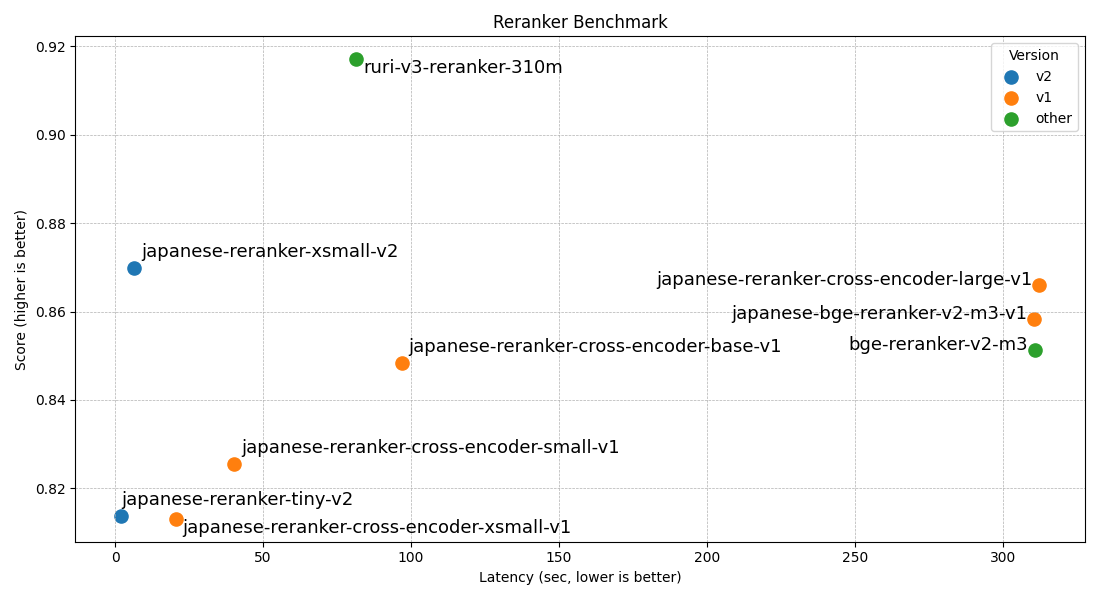 |
|
|
|
|
|
## 使い方 |
|
|
|
動作には transformers ライブラリの v4.48 以上が必要です。 |
|
|
|
``` |
|
pip install -U "transformers>=4.48.0" sentence-transformers |
|
``` |
|
|
|
GPU が Flash Attention 2 をサポートしている場合、flash-attn ライブラリを入れることで、高速な推論が可能です。 |
|
|
|
``` |
|
pip install flash-attn --no-build-isolation |
|
``` |
|
|
|
|
|
### SentenceTransformers |
|
|
|
```python |
|
from sentence_transformers import CrossEncoder |
|
import torch |
|
|
|
MODEL_NAME = "hotchpotch/japanese-reranker-xsmall-v2" |
|
|
|
model = CrossEncoder(MODEL_NAME) |
|
if model.device == "cuda" or model.device == "mps": |
|
model.model.half() |
|
query = "感動的な映画について" |
|
passages = [ |
|
"深いテーマを持ちながらも、観る人の心を揺さぶる名作。登場人物の心情描写が秀逸で、ラストは涙なしでは見られない。", |
|
"重要なメッセージ性は評価できるが、暗い話が続くので気分が落ち込んでしまった。もう少し明るい要素があればよかった。", |
|
"どうにもリアリティに欠ける展開が気になった。もっと深みのある人間ドラマが見たかった。", |
|
"アクションシーンが楽しすぎる。見ていて飽きない。ストーリーはシンプルだが、それが逆に良い。", |
|
] |
|
scores = model.predict( |
|
[(query, passage) for passage in passages], |
|
show_progress_bar=True, |
|
) |
|
print("Scores:", scores) |
|
``` |
|
|
|
## HuggingFace transformers |
|
|
|
```python |
|
from transformers import AutoTokenizer, AutoModelForSequenceClassification |
|
from torch.nn import Sigmoid |
|
|
|
|
|
def detect_device(): |
|
if torch.cuda.is_available(): |
|
return "cuda" |
|
elif hasattr(torch, "mps") and torch.mps.is_available(): |
|
return "mps" |
|
return "cpu" |
|
|
|
|
|
device = detect_device() |
|
tokenizer = AutoTokenizer.from_pretrained(MODEL_NAME) |
|
model = AutoModelForSequenceClassification.from_pretrained(MODEL_NAME) |
|
model.to(device) |
|
model.eval() |
|
|
|
if device == "cuda": |
|
model.half() |
|
|
|
query = "感動的な映画について" |
|
passages = [ |
|
"深いテーマを持ちながらも、観る人の心を揺さぶる名作。登場人物の心情描写が秀逸で、ラストは涙なしでは見られない。", |
|
"重要なメッセージ性は評価できるが、暗い話が続くので気分が落ち込んでしまった。もう少し明るい要素があればよかった。", |
|
"どうにもリアリティに欠ける展開が気になった。もっと深みのある人間ドラマが見たかった。", |
|
"アクションシーンが楽しすぎる。見ていて飽きない。ストーリーはシンプルだが、それが逆に良い。", |
|
] |
|
inputs = tokenizer( |
|
[(query, passage) for passage in passages], |
|
padding=True, |
|
truncation=True, |
|
max_length=512, |
|
return_tensors="pt", |
|
) |
|
inputs = {k: v.to(device) for k, v in inputs.items()} |
|
logits = model(**inputs).logits |
|
activation = Sigmoid() |
|
scores = activation(logits).squeeze().tolist() |
|
|
|
print("Scores:", scores) |
|
``` |
|
|
|
## 小型リランカーの特徴 |
|
|
|
japanese-reranker-tiny-v2とjapanese-reranker-xsmall-v2は、以下の特徴を持つ小型リランカーモデルです: |
|
|
|
1. CPUやAppleシリコン環境でも実用的な速度で動作 |
|
2. 高価なGPUリソースなしでもRAGシステムの精度向上が可能 |
|
3. エッジデバイスでの展開や低レイテンシが要求される本番環境で活用可能 |
|
4. ModernBertベースの[ruri-v3-pt-30m](https://huggingface.co/cl-nagoya/ruri-v3-pt-30m)を利用 |
|
|
|
|
|
## 評価結果 |
|
|
|
| モデル名 | avg | JQaRA | JaCWIR | MIRACL | JSQuAD | |
|
|---------|-----|-------|--------|--------|--------| |
|
| [japanese-reranker-tiny-v2](https://huggingface.co/hotchpotch/japanese-reranker-tiny-v2) | 0.8138 | 0.6455 | 0.9287 | 0.7201 | 0.9608 | |
|
| [japanese-reranker-xsmall-v2](https://huggingface.co/hotchpotch/japanese-reranker-xsmall-v2) | 0.8699 | 0.7403 | 0.9409 | 0.8206 | 0.9776 | |
|
| [japanese-reranker-cross-encoder-xsmall-v1](https://huggingface.co/hotchpotch/japanese-reranker-cross-encoder-xsmall-v1) | 0.8131 | 0.6136 | 0.9376 | 0.7411 | 0.9602 | |
|
| [japanese-reranker-cross-encoder-small-v1](https://huggingface.co/hotchpotch/japanese-reranker-cross-encoder-small-v1) | 0.8254 | 0.6247 | 0.9390 | 0.7776 | 0.9604 | |
|
| [japanese-reranker-cross-encoder-base-v1](https://huggingface.co/hotchpotch/japanese-reranker-cross-encoder-base-v1) | 0.8484 | 0.6711 | 0.9337 | 0.8180 | 0.9708 | |
|
| [japanese-reranker-cross-encoder-large-v1](https://huggingface.co/hotchpotch/japanese-reranker-cross-encoder-large-v1) | 0.8661 | 0.7099 | 0.9364 | 0.8406 | 0.9773 | |
|
| [japanese-bge-reranker-v2-m3-v1](https://huggingface.co/hotchpotch/japanese-bge-reranker-v2-m3-v1) | 0.8584 | 0.6918 | 0.9372 | 0.8423 | 0.9624 | |
|
| [bge-reranker-v2-m3](https://huggingface.co/BAAI/bge-reranker-v2-m3) | 0.8512 | 0.6730 | 0.9343 | 0.8374 | 0.9599 | |
|
| [ruri-v3-reranker-310m](https://huggingface.co/cl-nagoya/ruri-v3-reranker-310m) | 0.9171 | 0.8688 | 0.9506 | 0.8670 | 0.9820 | |
|
|
|
## 推論速度 |
|
|
|
以下は約15万ペアをリランキングした際の推論速度結果(トークナイズ時間を除く純粋なモデル推論時間)です。MPS(Appleシリコン)とCPU測定にはM4 Max、GPUにはRTX5090を使用しています。GPU処理では flash-attention2 を使用しています。 |
|
|
|
| モデル名 | レイヤー数 | 隠れ層サイズ | 速度(GPU) | 速度(MPS) | 速度(CPU) | |
|
|---------|------------|-------------|-----------|-----------|-----------| |
|
| [japanese-reranker-tiny-v2](https://huggingface.co/hotchpotch/japanese-reranker-tiny-v2) | 3 | 256 | 2.1s | 82s | 702s | |
|
| [japanese-reranker-xsmall-v2](https://huggingface.co/hotchpotch/japanese-reranker-xsmall-v2) | 10 | 256 | 6.5s | 303s | 2300s | |
|
| [japanese-reranker-cross-encoder-xsmall-v1](https://huggingface.co/hotchpotch/japanese-reranker-cross-encoder-xsmall-v1) | 6 | 384 | 20.5s | | | |
|
| [japanese-reranker-cross-encoder-small-v1](https://huggingface.co/hotchpotch/japanese-reranker-cross-encoder-small-v1) | 12 | 384 | 40.3s | | | |
|
| [japanese-reranker-cross-encoder-base-v1](https://huggingface.co/hotchpotch/japanese-reranker-cross-encoder-base-v1) | 12 | 768 | 96.8s | | | |
|
| [japanese-reranker-cross-encoder-large-v1](https://huggingface.co/hotchpotch/japanese-reranker-cross-encoder-large-v1) | 24 | 1024 | 312.2s | | | |
|
| [japanese-bge-reranker-v2-m3-v1](https://huggingface.co/hotchpotch/japanese-bge-reranker-v2-m3-v1) | 24 | 1024 | 310.6s | | | |
|
| [bge-reranker-v2-m3](https://huggingface.co/BAAI/bge-reranker-v2-m3) | 24 | 1024 | 310.7s | | | |
|
| [ruri-v3-reranker-310m](https://huggingface.co/cl-nagoya/ruri-v3-reranker-310m) | 25 | 768 | 81.4s | | | |
|
|
|
推論速度のベンチマークに用いた[スクリプトはこちら](https://gist.github.com/hotchpotch/bab03c7d4399aa13beb2702600ad9371)です。 |
|
|
|
## ライセンス |
|
|
|
MIT License |