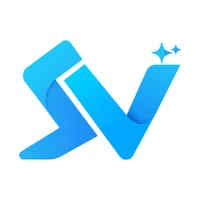
SparkVerseAI
AI & ML interests
SparkVerse AI is a leading enterprise AI company headquartered in Bradford, United Kingdom, dedicated to unlocking business potential through intelligent, data-driven solutions. Founded in 2021, we began our journey as a machine learning service provider, delivering custom AI models and insights to clients across diverse industries. By 2024, SparkVerse AI had evolved into a specialized provider of enterprise knowledge management systems, enabling enterprises to fully utilize their data through scalable, AI-enhanced, and customized knowledge platforms. Our mission is simple but effective: to empower businesses move faster, scale smarter, and serve better by transforming complex data into actionable intelligence. From cloud-native deployments to secure on-site solutions, SparkVerse AI combines cutting-edge machine learning with pragmatic corporate strategy to drive digital transformation on a large scale.
Recent Activity
SparkVerseAI's activity
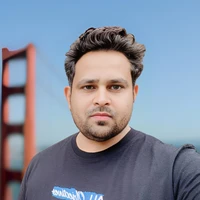
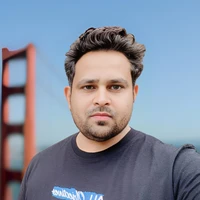
Improving Arabic Multi-Label Emotion Classification using Stacked Embeddings and Hybrid Loss Function (2410.03979)
In this work, we tackle some major challenges in Arabic multi-label emotion classification especially the issues of class imbalance and label correlation that often hurt model performance, particularly for minority emotions.
Our approach:
Stacked contextual embeddings from fine-tuned ArabicBERT, MarBERT, and AraBERT models.
A meta-learning strategy that builds richer representations.
A hybrid loss function combining class weighting, label correlation matrices, and contrastive learning to better handle class imbalances.
๐ง Model pipeline: stacked embeddings โ meta-learner โ Bi-LSTM โ fully connected network โ multi-label classification.
๐ Extensive experiments show significant improvements across Precision, Recall, F1-Score, Jaccard Accuracy, and Hamming Loss.
๐ The hybrid loss function in particular helped close the gap between majority and minority classes!
We also performed ablation studies to break down each componentโs contribution and the results consistently validated our design choices.
This framework isn't just for Arabic it offers a generalizable path for improving multi-label emotion classification in other low-resource languages and domains.
Big thanks to my co-authors: Muhammad Azeem Aslam, Wang Jun, Nisar Ahmed, Li Yanan, Hu Hongfei, Wang Shiyu, and Xin Liu!
Would love to hear your thoughts on this work! ๐
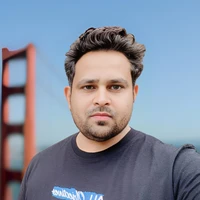
https://huggingface.co/blog/ImranzamanML/llama-4-fine-tuning-with-mental-health-counseling
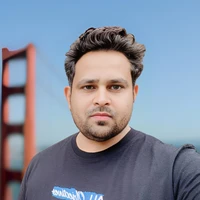
Llama 4 is here and it's making serious waves!
After diving into the latest benchmark results, itโs clear that Metaโs new Llama 4 lineup (Maverick, Scout, and Behemoth) is no joke.
Here are a few standout highlights๐:
Llama 4 Maverick hits the sweet spot between cost and performance
- Outperforms GPT-4o in image tasks like ChartQA (90.0 vs 85.7) and DocVQA (94.4 vs 92.8)
- Beats others in MathVista and MMLU Pro too and at a fraction of the cost ($0.19โ$0.49 vs $4.38 ๐คฏ)
Llama 4 Scout is lean, cost-efficient, and surprisingly capable
- Strong performance across image and language tasks (e.g. ChartQA: 88.8, DocVQA: 94.4)
- More affordable than most competitors and still beats out larger models like Gemini 2.0 Flash-Lite
Llama 4 Behemoth is the heavy hitter.
- Tops the charts in LiveCodeBench (49.4), MATH-500 (95.0), and MMLU Pro (82.2)
- Even edges out Claude 3 Sonnet and Gemini 2 Pro in multiple areas
Meta didnโt just show up, they delivered across multimodal, coding, reasoning, and multilingual benchmarks.
And honestly? Seeing this level of performance, especially at lower inference costs, is a big deal for anyone building on LLMs.
Curious to see how these models do in real-world apps next.
#AI #Meta #Llama4 #LLMs #Benchmarking #MachineLearning #OpenSourceAI #GenerativeAI
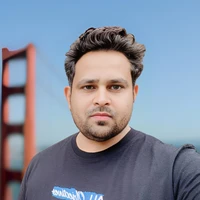
- Learn AI Agent fundamentals, use cases and frameworks
- Use top libraries like LangChain & LlamaIndex
- Compete in challenges & earn a certificate
- Hands-on projects & real-world applications
https://huggingface.co/learn/agents-course/unit0/introduction
You can join for a live Q&A on Feb 12 at 5PM CET to learn more about the course here
https://www.youtube.com/live/PopqUt3MGyQ
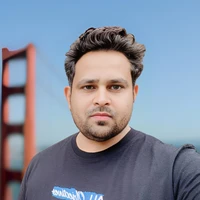
Lets start with three patients groups:
Group A
Group B
Group C
For each patient, we will predict risk score (higher score means higher risk of early event).
Step 1: Understanding Concordance Index
The Concordance Index (C-index) evaluate that how well the model ranks survival times.
Understand with sample data:
Group A has 3 patients with actual survival times and predicted risk scores:
Patient Actual Survival Time Predicted Risk Score
P1 5 months 0.8
P2 3 months 0.9
P3 10 months 0.2
Comparable pairs:
(P1, P2): P2 has a shorter survival time and a higher risk score โ Concordant โ
(P1, P3): P3 has a longer survival time and a lower risk score โ Concordant โ
(P2, P3): P3 has a longer survival time and a lower risk score โ Concordant โ
Total pairs = 3
Total concordant pairs = 3
C-index for Group A = Concordant pairs/Total pairs= 3/3 = 1.0
Step 2: Calculate C-index for All Groups
Repeat the process for all groups. For now we can assume:
Group A: C-index = 1.0
Group B: C-index = 0.8
Group C: C-index = 0.6
Step 3: Stratified Concordance Index
The Stratified Concordance Index combines the C-index scores of all groups and focusing on the following:
Average performance across groups (mean of C-indices).
Consistency across groups (low standard deviation of C-indices).
Formula:
Stratified C-index = Mean(C-index scores) - Standard Deviation(C-index scores)
Calculate the mean:
Mean=1.0 + 0.8 + 0.6/3 = 0.8
Calculate the standard deviation:
Standard Deviation= sqrt((1.0-0.8)^2 + (0.8-0.8)^2 + (0.6-0.8)^/3) = 0.16
Stratified C-index:
Stratified C-index = 0.8 - 0.16 = 0.64
Step 4: Interpret the Results
A high Stratified C-index means:
The model predicts well overall (high mean C-index).
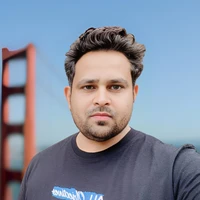
1. Document Embedding & Indexing
We can start with the use of embedding models to vectorize documents, store them in vector databases (Elasticsearch, Pinecone, Weaviate) for efficient retrieval.
2. Smart Querying
Then we can generate query embeddings, retrieve top-K relevant chunks and can apply hybrid search if needed for better precision.
3. Context Management
We can concatenate retrieved chunks, optimize chunk order and keep within token limits to preserve response coherence.
4. Prompt Engineering
Then we can instruct the LLM to leverage retrieved context, using clear instructions to prioritize the provided information.
5. Post-Processing
Finally we can implement response verification, fact-checking and integrate feedback loops to refine the responses.
Happy to connect :)
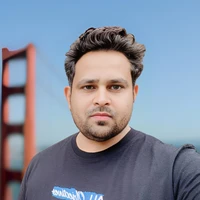
Logging
Logging is very important part of any project you start. It help you to track the execution of a program, debug issues, monitor system performance and keep an audit trail of events.
Basic Logging Setup
The basic way to add logging to a Python code is by using the logging.basicConfig() function. This function set up basic configuration for logging messages to either console or to a file.
Here is how we can use basic console logging
#Call built in library
import logging
# lets call library and start logging
logging.basicConfig(level=logging.DEBUG) #you can add more format specifier
# It will show on the console since we did not added filename to save logs
logging.debug('Here we go for debug message')
logging.info('Here we go for info message')
logging.warning('Here we go for warning message')
logging.error('Here we go for error message')
logging.critical('Here we go for critical message')
#Note:
# If you want to add anything in the log then do like this way
records=100
logging.debug('There are total %s number of records.', records)
# same like string format
lost=20
logging.debug('There are total %s number of records from which %s are lost', records, lost)
Logging to a File
We can also save the log to a file instead of console. For this, we can add the filename parameter to logging.basicConfig().
import logging
# Saving the log to a file. The logs will be written to app.log
logging.basicConfig(filename='app.log', level=logging.DEBUG)
logging.debug('Here we go for debug message')
logging.info('Here we go for info message')
logging.warning('Here we go for warning message')
logging.error('Here we go for error message')
logging.critical('Here we go for critical message')
You can read more on my medium blog https://medium.com/@imranzaman-5202/are-you-a-professional-python-developer-8596e2b2edaa
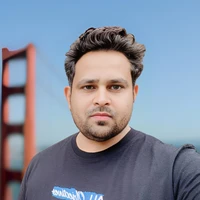
LoRA (Low-Rank Adaptation)
LoRA adds low-rank matrices to specific layers and reduce the number of trainable parameters for efficient fine-tuning.
Code:
Please install these libraries first:
pip install peft
pip install datasets
pip install transformers
from transformers import AutoModelForSequenceClassification, Trainer, TrainingArguments
from peft import LoraConfig, get_peft_model
from datasets import load_dataset
# Loading the pre-trained BERT model
model = AutoModelForSequenceClassification.from_pretrained('bert-base-uncased', num_labels=2)
# Configuring the LoRA parameters
lora_config = LoraConfig(
r=8,
lora_alpha=16,
lora_dropout=0.1,
bias="none"
)
# Applying LoRA to the model
model = get_peft_model(model, lora_config)
# Loading dataset for classification
dataset = load_dataset("glue", "sst2")
train_dataset = dataset["train"]
# Setting the training arguments
training_args = TrainingArguments(
output_dir="./results",
per_device_train_batch_size=16,
num_train_epochs=3,
logging_dir="./logs",
)
# Creating a Trainer instance for fine-tuning
trainer = Trainer(
model=model,
args=training_args,
train_dataset=train_dataset,
)
# Finally we can fine-tune the model
trainer.train()
LoRA adds low-rank matrices to fine-tune only a small portion of the model and reduces training overhead by training fewer parameters.
We can perform efficient fine-tuning with minimal impact on accuracy and its suitable for large models where full-precision training is still feasible.
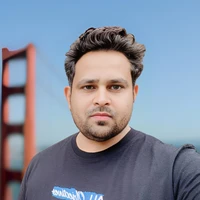
Floating-point numbers are used to represent real numbers (like decimals) and they consist of three parts:
Sign bit:
Indicates whether the number is positive (0) or negative (1).
Exponent:
Determines the scale of the number (i.e., how large or small it is by shifting the decimal point).
Mantissa (or fraction):
Represents the actual digits of the number.
32-bit Floating Point (FP32)
Total bits: 32 bits
Sign bit: 1 bit
Exponent: 8 bits
Mantissa: 23 bits
For example:
A number like -15.375 would be represented as:
Sign bit: 1 (negative number)
Exponent: Stored after being adjusted by a bias (127 in FP32).
Mantissa: The significant digits after converting the number to binary.
16-bit Floating Point (FP16)
Total bits: 16 bits
Sign bit: 1 bit
Exponent: 5 bits
Mantissa: 10 bits
Example:
A number like -15.375 would be stored similarly:
Sign bit: 1 (negative number)
Exponent: Uses 5 bits, limiting the range compared to FP32.
Mantissa: Only 10 bits for precision.
Precision and Range
FP32: Higher precision and larger range, with about 7 decimal places of accuracy.
FP16: Less precision (around 3-4 decimal places), smaller range but faster computations and less memory use.
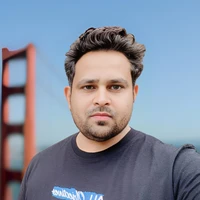
https://drive.google.com/file/d/1p5sT4_DeyBuwCqmYt4dCJKZOgLMpESzR/view
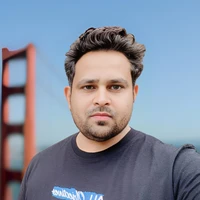
Each parameter in LLM models is typically stored as a floating-point number. The size of each parameter in bytes depends on the precision.
32-bit precision: Each parameter takes 4 bytes.
16-bit precision: Each parameter takes 2 bytes
To calculate the total memory usage of the model:
Memory usage (in bytes) = No. of Parameters ร Size of Each Parameter
For example:
32-bit Precision (FP32)
In 32-bit floating-point precision, each parameter takes 4 bytes.
Memory usage in bytes = 1 billion parameters ร 4 bytes
1,000,000,000 ร 4 = 4,000,000,000 bytes
In gigabytes: โ 3.73 GB
16-bit Precision (FP16)
In 16-bit floating-point precision, each parameter takes 2 bytes.
Memory usage in bytes = 1 billion parameters ร 2 bytes
1,000,000,000 ร 2 = 2,000,000,000 bytes
In gigabytes: โ 1.86 GB
It depends on whether you use 32-bit or 16-bit precision, a model with 1 billion parameters would use approximately 3.73 GB or 1.86 GB of memory, respectively.
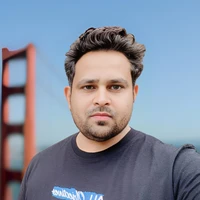
It's easier to assess the quality of a response than to generate one which enables LLM models to evaluate their own performance.
Itโs like trying to figure out how many ingredients you left out while cooking a recipe but without knowing exactly which ones you missed. LLM models like experienced cooks, canโt always tell you what specific step they skipped but they can guess how close they got to the final dish. For example, if your meal tastes 75%, you know something is off, but you are not sure what exactly.
Now instead of focusing on identifying every missed ingredient, think about just estimating how well the dish turned out overall. Itโs easier to guess if the meal tastes good than to pinpoint each small mistake. LLMs do the same, they estimate how well they performed without knowing every single error, allowing them to self-evaluate!
meta-llama/Llama-3.2-1B