Shuttle 3 Diffusion
Join our Discord to get the latest updates, news, and more.
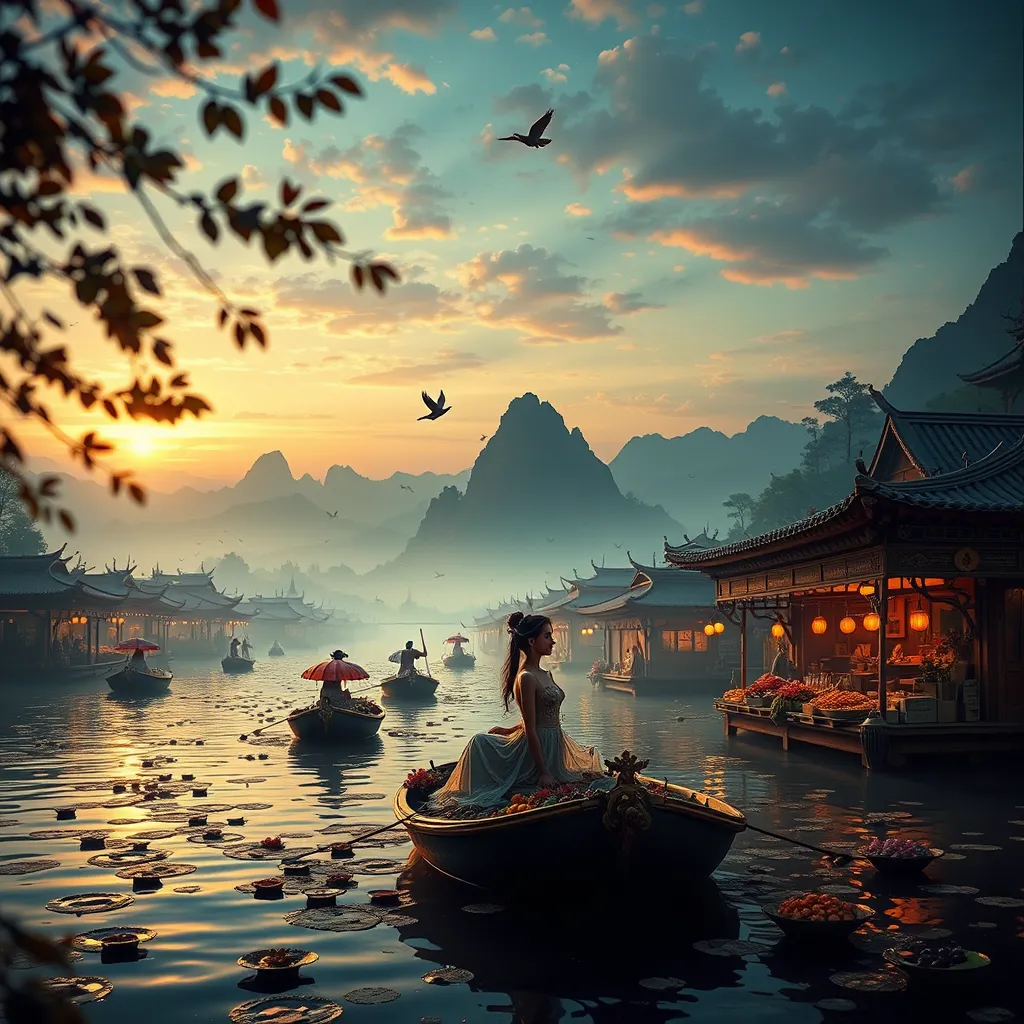
- Prompt
- Venus floating market at dawn, fantasy digital art, highly detailed, atmospheric lighting with film-like light leaks, impressive background, studio photo style, cinematic, intricate details.

- Prompt
- Silent forest, sun barely piercing treetops, mysterious lake turns dark red at dawn, reflecting colorful sky. Lone tree on shore with diamond-like dewdrops, photorealistic.

- Prompt
- A beautiful photo showcases a night waterfall in the jungle, illuminated with a subtle blue tint that adds an ethereal touch. Fireflies float delicately around, their gentle glow enhancing the magical ambiance of the scene.
Model Variants
These model variants provide different precision levels and formats optimized for diverse hardware capabilities and use cases
Shuttle 3 Diffusion is a text-to-image AI model designed to create detailed and diverse images from textual prompts in just 4 steps. It offers enhanced performance in image quality, typography, understanding complex prompts, and resource efficiency.
You can try out the model through a website at https://chat.shuttleai.com/images
Using the model via API
You can use Shuttle 3 Diffusion via API through ShuttleAI
Using the model with ๐งจ Diffusers
Install or upgrade diffusers
pip install -U diffusers
Then you can use DiffusionPipeline
to run the model
import torch
from diffusers import DiffusionPipeline
# Load the diffusion pipeline from a pretrained model, using bfloat16 for tensor types.
pipe = DiffusionPipeline.from_pretrained(
"shuttleai/shuttle-3-diffusion", torch_dtype=torch.bfloat16
).to("cuda")
# Uncomment the following line to save VRAM by offloading the model to CPU if needed.
# pipe.enable_model_cpu_offload()
# Uncomment the lines below to enable torch.compile for potential performance boosts on compatible GPUs.
# Note that this can increase loading times considerably.
# pipe.transformer.to(memory_format=torch.channels_last)
# pipe.transformer = torch.compile(
# pipe.transformer, mode="max-autotune", fullgraph=True
# )
# Set your prompt for image generation.
prompt = "A cat holding a sign that says hello world"
# Generate the image using the diffusion pipeline.
image = pipe(
prompt,
height=1024,
width=1024,
guidance_scale=3.5,
num_inference_steps=4,
max_sequence_length=256,
# Uncomment the line below to use a manual seed for reproducible results.
# generator=torch.Generator("cpu").manual_seed(0)
).images[0]
# Save the generated image.
image.save("shuttle.png")
To learn more check out the diffusers documentation
Using the model with ComfyUI
To run local inference with Shuttle 3 Diffusion using ComfyUI, you can use this safetensors file.
Comparison to other models
Shuttle 3 Diffusion can produce images better images than Flux Dev in just four steps, while being licensed under Apache 2.
More examples
Training Details
Shuttle 3 Diffusion uses Flux.1 Schnell as its base. It can produce images similar to Flux Dev or Pro in just 4 steps, and it is licensed under Apache 2. The model was partially de-distilled during training. When used beyond 10 steps, it enters "refiner mode," enhancing image details without altering the composition. We overcame the limitations of the Schnell-series models by employing a special training method, resulting in improved details and colors.
- Downloads last month
- 28,504