datasetId
large_stringlengths 6
107
| author
large_stringlengths 3
34
| last_modified
large_stringdate 2021-05-20 00:57:22
2025-05-05 20:13:58
| downloads
int64 0
4.28M
| likes
int64 0
7.74k
| tags
large listlengths 1
2.03k
| task_categories
large listlengths 0
16
| createdAt
large_stringdate 2022-03-02 23:29:22
2025-05-05 20:10:54
| trending_score
float64 1
39
⌀ | card
large_stringlengths 31
1M
|
---|---|---|---|---|---|---|---|---|---|
kaiwenw/distill-r1-qwen-1.5b-hmmt-feb-24-4096-with-old-prm-indices_30720_38400 | kaiwenw | 2025-05-05T18:56:21Z | 0 | 0 | [
"region:us"
] | [] | 2025-05-05T18:56:08Z | null | ---
dataset_info:
features:
- name: message_id
dtype: string
- name: problem
dtype: string
- name: answer
dtype: string
- name: processed_answer
dtype: string
- name: responses
dtype: string
- name: reward
dtype: bool
- name: prompt_len
dtype: int64
- name: response_len
dtype: int64
- name: classifier_scores
sequence: float64
splits:
- name: train
num_bytes: 1120119801
num_examples: 7680
download_size: 265485450
dataset_size: 1120119801
configs:
- config_name: default
data_files:
- split: train
path: data/train-*
---
|
Aman6u5/bucket | Aman6u5 | 2025-05-05T12:54:48Z | 0 | 0 | [
"license:apache-2.0",
"size_categories:n<1K",
"modality:video",
"library:datasets",
"library:mlcroissant",
"region:us"
] | [] | 2025-05-05T11:12:57Z | null | ---
license: apache-2.0
---
|
herwoww/MultiDiac | herwoww | 2025-05-05T09:45:55Z | 0 | 0 | [
"region:us"
] | [] | 2025-05-05T09:41:16Z | null | ---
configs:
- config_name: yor
data_files:
- split: train
path: yor_train.csv
# - split: test
# path: yor_test.csv
- split: dev
path: yor_dev.csv
# - config_name: ara
# data_files:
# - split: test
# path: ara_test.csv
--- |
dgambettaphd/D_llm2_gen10_WXS_doc1000_synt64_lr1e-04_acm_MPP | dgambettaphd | 2025-05-05T08:58:53Z | 0 | 0 | [
"region:us"
] | [] | 2025-05-05T08:58:25Z | null | ---
dataset_info:
features:
- name: id_doc
dtype: int64
- name: text
dtype: string
- name: dataset
dtype: string
- name: gen
dtype: int64
- name: synt
dtype: int64
- name: MPP
dtype: float64
splits:
- name: train
num_bytes: 14923305
num_examples: 26000
download_size: 8995691
dataset_size: 14923305
configs:
- config_name: default
data_files:
- split: train
path: data/train-*
---
|
Denhotech/denes_data | Denhotech | 2025-05-05T07:07:32Z | 0 | 0 | [
"size_categories:n<1K",
"format:parquet",
"modality:tabular",
"modality:text",
"library:datasets",
"library:pandas",
"library:mlcroissant",
"library:polars",
"region:us"
] | [] | 2025-05-05T06:56:15Z | null | ---
dataset_info:
features:
- name: Name
dtype: string
- name: Age
dtype: int64
- name: City
dtype: string
- name: Score
dtype: float64
splits:
- name: train
num_bytes: 734
num_examples: 20
download_size: 2104
dataset_size: 734
configs:
- config_name: default
data_files:
- split: train
path: data/train-*
---
|
Yuyeong/rw_roman-empire_node2vec_1_mask_public | Yuyeong | 2025-05-05T06:59:25Z | 0 | 0 | [
"size_categories:1M<n<10M",
"format:parquet",
"modality:tabular",
"modality:text",
"library:datasets",
"library:dask",
"library:mlcroissant",
"library:polars",
"region:us"
] | [] | 2025-05-05T06:50:27Z | null | ---
dataset_info:
features:
- name: text
dtype: string
- name: label
dtype:
class_label:
names:
'0': '0'
'1': '1'
'2': '2'
'3': '3'
'4': '4'
'5': '5'
'6': '6'
'7': '7'
'8': '8'
'9': '9'
'10': '10'
'11': '11'
'12': '12'
'13': '13'
'14': '14'
'15': '15'
'16': '16'
'17': '17'
- name: group_idx
dtype: int64
- name: node_idx
dtype: int64
splits:
- name: train
num_bytes: 3825069706.0214458
num_examples: 2264100
- name: validation
num_bytes: 3606793567.150384
num_examples: 2134900
- name: test
num_bytes: 3602232068.8922424
num_examples: 2132200
download_size: 3312737632
dataset_size: 11034095342.064072
---
# Dataset Card for "rw_roman-empire_node2vec_1_mask_public"
[More Information needed](https://github.com/huggingface/datasets/blob/main/CONTRIBUTING.md#how-to-contribute-to-the-dataset-cards) |
Rakuto/DailyTalkContiguous-ja | Rakuto | 2025-05-05T04:51:27Z | 0 | 0 | [
"task_categories:automatic-speech-recognition",
"language:ja",
"license:cc-by-sa-4.0",
"arxiv:2207.01063",
"region:us"
] | [
"automatic-speech-recognition"
] | 2025-05-04T16:37:39Z | null | ---
license: cc-by-sa-4.0
task_categories:
- automatic-speech-recognition
language:
- ja
---
# DailyTalkContiguous-ja: Spoken Dialogue Dataset in Japanese
DailyTalkContiguous-ja is a synthetic multi-turn Japanese conversational speech dataset in which [DailyTalk](https://arxiv.org/abs/2207.01063) [Keon Lee etal., 2022]
translated by [Gemma-3-27B](https://huggingface.co/google/gemma-3-27b-it) and speech data is synthesized by TTS engine [Zyphra/Zonos-v0.1-transformer](https://github.com/Zyphra/Zonos).
For each speaker in covnersation, different voice is randomly asssigned from voice dataset with five voices in total.
As like with [kyutai/DailyTalkContiguous](https://huggingface.co/datasets/kyutai/DailyTalkContiguous), rather than having separate files for each speaker's turn, this uses a stereo file for each conversation.
The two speakers in a conversation are put separately on the left and right channels.
**Dataset size**: 25 hours speech with 2.5k conversation |
Eluza133/A12d12s12 | Eluza133 | 2025-05-05T04:02:26Z | 2,965 | 0 | [
"license:apache-2.0",
"size_categories:n<1K",
"modality:image",
"modality:video",
"library:datasets",
"library:mlcroissant",
"region:us"
] | [] | 2025-02-27T15:03:01Z | null | ---
license: apache-2.0
---
|
aiwithvarun7/theekkathir-text-dataset | aiwithvarun7 | 2025-05-05T02:23:26Z | 3,388 | 1 | [
"task_categories:text-generation",
"language:ta",
"license:cc-by-nc-4.0",
"size_categories:1K<n<10K",
"format:parquet",
"modality:text",
"library:datasets",
"library:dask",
"library:mlcroissant",
"library:polars",
"region:us"
] | [
"text-generation"
] | 2024-11-02T17:48:43Z | null | ---
license: cc-by-nc-4.0
dataset_info:
features:
- name: தேதி
dtype: string
- name: தலைப்பு
dtype: string
- name: செய்தி-வகை
dtype: string
- name: எழுத்தாளர்
dtype: string
- name: இணைப்பு
dtype: string
- name: மொழி
dtype: string
- name: குறிமுறை தரநிலை
dtype: string
- name: உள்ளடக்கம்
dtype: string
- name: சேகரிக்கப்பட்ட தேதி
dtype: string
configs:
- config_name: sample parquets
data_files: TheekkathirDataset/parquets/*.parquet
language:
- ta
task_categories:
- text-generation
size_categories:
- 100K<n<1M
---
<h1 align="center"><b>theekkathir-text-dataset <-> தீக்கதிர் தரவுத்தொகுப்பு</b></h1>
<p align="center">
<img src="https://github.com/user-attachments/assets/3731edf1-70b9-4e0a-98c1-6b89c4e03395" />
</p>
---
<a href="https://github.com/vishnumur777/theekkathir-text-dataset/tree/main">
<p align="center">
<img src="https://cdn-uploads.huggingface.co/production/uploads/64d848ce620c17bfa092e051/4ySVV0-jiAT_P3iIde0ei.png" alt="hugging face group" width="500px" height="700px"/>
</p>
</a>
<h2 align="center">Click above button to view GitHub Repository</h2>
<h3>இலக்கு:</h3>
இந்த திட்டத்தின் இலக்கு தீக்கதிர் இதழின் செய்தி கட்டுரைகளை தரவுத்தொகுப்பாக மாற்றுவதாகும், இது இயற்கை மொழி பதிவு (NLP) மற்றும் LLM ஆராய்ச்சி நோக்கங்களுக்கு பயன்படுத்தப்படலாம்.
<h3>Goal:</h3>
The goal of the project is to convert news articles from theekkathir magazine into dataset, which can be used for Natural Language Processing (NLP) and LLM research purposes
# Columns in .parquet
- வெளியிட்ட தேதி (Released Date)
- தலைப்பு (Title)
- செய்தி வகை (Categories)
- எழுத்தாளர் (Author)
- மொழி (Language)
- குறிமுறைத் தரநிலை (Character Encoding)
- உள்ளடக்கம் (Content)
- சேகரிக்கப்பட்ட தேதி (Scraped Date)
### You can also get [texts](https://huggingface.co/datasets/aiwithvarun7/theekkathir-text-dataset/tree/main/TheekkathirDataset/texts) apart from parquet files.
# How to Contribute
If you want to contribute to this project, Contact me via [LinkedIn](https://linkedin.com/in/varun-muralidhar)
- If possible, write CONTRIBUTING.md and make Pull Request here.
- Able to Read and Write Tamil.
- Follow [Medium](https://medium.com/@VARUNMURALIDHAR), For detailed documentation and I will update on any contribution.
- Raise issues and PR, if possible.
# எவ்வாறு பங்களிக்கலாம்
இந்த திட்டத்திற்கு பங்களிக்க விரும்பினால், [LinkedIn](https://linkedin.com/in/varun-muralidhar) மூலம் என்னை தொடர்பு கொள்ளவும்.
- தமிழ் மொழியை படிக்க, எழுத தெரிய வேண்டும்.
- சாத்தியமானால், CONTRIBUTING.md எழுதி இங்கு Pull Request செய்யவும்.
- விரிவான ஆவணங்களுக்காக [Medium](https://medium.com/@VARUNMURALIDHAR) பின்தொடரவும். நான் எந்தவொரு பங்களிப்பையும் புதுப்பிக்கிறேன்.
- சாத்தியமானால், பிரச்சினைகளையும் PR (Pull Request) யையும் உயர்த்தவும். |
THU-ATOM/DrugCLIP_data | THU-ATOM | 2025-05-05T02:12:28Z | 100 | 0 | [
"license:cc-by-4.0",
"region:us"
] | [] | 2024-08-29T06:36:29Z | null | ---
license: cc-by-4.0
---
---
license: cc-by-4.0
---
# 🧬 DrugCLIP data repository
This repository hosts benchmark datasets, pre-computed molecular embeddings, pretrained model weights, and supporting files used in the **DrugCLIP** project. It also includes data and models used for **wet lab validation experiments**.
---
## 📁 Repository Contents
### 1. `DUD-E.zip`
- Full dataset for the **DUD-E benchmark**.
- Includes ligand and target files for all targets.
---
### 2. `LIT-PCBA.zip`
- Full dataset for the **LIT-PCBA benchmark**.
- Includes ligand and target files for all targets.
---
### 3. `encoded_mol_embs.zip`
- Pre-encoded molecular embeddings from the **ChemDiv** compound library.
- Each `.pkl` file contains:
- `name_list`: `[hitid, SMILES]`
- `embedding_list`: list of **128-dimensional** vectors
- Versions included:
- **8-fold** version of the full ChemDiv library
- **6-fold** version of the full ChemDiv library
- **6-fold** version of a filtered ChemDiv library
---
### 4. `benchmark_weights.zip`
Contains **pretrained model weights** for **benchmark experiments** on the DUD-E and LIT-PCBA datasets using various ligand and target filtering strategies.
#### 🔬 DUD-E: Ligand Filtering Strategies
| Filename | Description |
|----------------------|-------------|
| `dude_ecfp_90.pt` | Trained by removing ligands with **ECFP4 similarity > 0.9**. |
| `dude_ecfp_60.pt` | Trained by removing ligands with **ECFP4 similarity > 0.6**. |
| `dude_ecfp_30.pt` | Trained by removing ligands with **ECFP4 similarity > 0.3**. |
| `dude_scaffold.pt` | Trained by removing ligands sharing **scaffolds** with test set. |
#### 🧬 DUD-E: Target Filtering Strategies
| Filename | Description |
|------------------------|-------------|
| `dude_identity_90.pt` | Removed targets with **MMseqs2 identity > 0.9**. |
| `dude_identity_60.pt` | Removed targets with **MMseqs2 identity > 0.6**. |
| `dude_identity_30.pt` | Removed targets with **MMseqs2 identity > 0.3**. |
| `dude_identity_0.pt` | Removed targets based on **HMMER sequence identity**. |
#### 🧪 LIT-PCBA: Target Filtering Strategy
| Filename | Description |
|-------------------------|-------------|
| `litpcba_identity_90.pt`| Removed targets with **MMseqs2 identity > 0.9**. |
---
### 5. `model_weights.zip`
Contains model weights trained specifically for **wet lab experiments**. These models were trained using:
- **6-fold** data splits
- **8-fold** data splits
Used to predict compounds validated in real-world assays for the following targets:
- `5HT2a`
- `NET`
- `Trip12`
---
### 6. `WetLab_PDBs_and_LMDBs`
Target data used for wet lab validation experiments:
- **LMDB files**: For DrugCLIP screening
Includes data for:
- `5HT2a`
- `NET`
- `Trip12`
---
### 7. `benchmark_throughput`
Files for reproducing throughput benchmark results.
|
john-1111/x_dataset_060792 | john-1111 | 2025-05-05T01:29:48Z | 273 | 0 | [
"task_categories:text-classification",
"task_categories:token-classification",
"task_categories:question-answering",
"task_categories:summarization",
"task_categories:text-generation",
"task_ids:sentiment-analysis",
"task_ids:topic-classification",
"task_ids:named-entity-recognition",
"task_ids:language-modeling",
"task_ids:text-scoring",
"task_ids:multi-class-classification",
"task_ids:multi-label-classification",
"task_ids:extractive-qa",
"task_ids:news-articles-summarization",
"multilinguality:multilingual",
"source_datasets:original",
"license:mit",
"size_categories:10M<n<100M",
"format:parquet",
"modality:text",
"library:datasets",
"library:dask",
"library:mlcroissant",
"library:polars",
"region:us"
] | [
"text-classification",
"token-classification",
"question-answering",
"summarization",
"text-generation"
] | 2025-01-25T07:15:47Z | null | ---
license: mit
multilinguality:
- multilingual
source_datasets:
- original
task_categories:
- text-classification
- token-classification
- question-answering
- summarization
- text-generation
task_ids:
- sentiment-analysis
- topic-classification
- named-entity-recognition
- language-modeling
- text-scoring
- multi-class-classification
- multi-label-classification
- extractive-qa
- news-articles-summarization
---
# Bittensor Subnet 13 X (Twitter) Dataset
<center>
<img src="https://huggingface.co/datasets/macrocosm-os/images/resolve/main/bittensor.png" alt="Data-universe: The finest collection of social media data the web has to offer">
</center>
<center>
<img src="https://huggingface.co/datasets/macrocosm-os/images/resolve/main/macrocosmos-black.png" alt="Data-universe: The finest collection of social media data the web has to offer">
</center>
## Dataset Description
- **Repository:** john-1111/x_dataset_060792
- **Subnet:** Bittensor Subnet 13
- **Miner Hotkey:** 5GKGMoTKZRasLaJevQBAEDksBj7RGDgvVb9zqkY3ygXtx3bo
### Miner Data Compliance Agreement
In uploading this dataset, I am agreeing to the [Macrocosmos Miner Data Compliance Policy](https://github.com/macrocosm-os/data-universe/blob/add-miner-policy/docs/miner_policy.md).
### Dataset Summary
This dataset is part of the Bittensor Subnet 13 decentralized network, containing preprocessed data from X (formerly Twitter). The data is continuously updated by network miners, providing a real-time stream of tweets for various analytical and machine learning tasks.
For more information about the dataset, please visit the [official repository](https://github.com/macrocosm-os/data-universe).
### Supported Tasks
The versatility of this dataset allows researchers and data scientists to explore various aspects of social media dynamics and develop innovative applications. Users are encouraged to leverage this data creatively for their specific research or business needs.
For example:
- Sentiment Analysis
- Trend Detection
- Content Analysis
- User Behavior Modeling
### Languages
Primary language: Datasets are mostly English, but can be multilingual due to decentralized ways of creation.
## Dataset Structure
### Data Instances
Each instance represents a single tweet with the following fields:
### Data Fields
- `text` (string): The main content of the tweet.
- `label` (string): Sentiment or topic category of the tweet.
- `tweet_hashtags` (list): A list of hashtags used in the tweet. May be empty if no hashtags are present.
- `datetime` (string): The date when the tweet was posted.
- `username_encoded` (string): An encoded version of the username to maintain user privacy.
- `url_encoded` (string): An encoded version of any URLs included in the tweet. May be empty if no URLs are present.
### Data Splits
This dataset is continuously updated and does not have fixed splits. Users should create their own splits based on their requirements and the data's timestamp.
## Dataset Creation
### Source Data
Data is collected from public tweets on X (Twitter), adhering to the platform's terms of service and API usage guidelines.
### Personal and Sensitive Information
All usernames and URLs are encoded to protect user privacy. The dataset does not intentionally include personal or sensitive information.
## Considerations for Using the Data
### Social Impact and Biases
Users should be aware of potential biases inherent in X (Twitter) data, including demographic and content biases. This dataset reflects the content and opinions expressed on X and should not be considered a representative sample of the general population.
### Limitations
- Data quality may vary due to the decentralized nature of collection and preprocessing.
- The dataset may contain noise, spam, or irrelevant content typical of social media platforms.
- Temporal biases may exist due to real-time collection methods.
- The dataset is limited to public tweets and does not include private accounts or direct messages.
- Not all tweets contain hashtags or URLs.
## Additional Information
### Licensing Information
The dataset is released under the MIT license. The use of this dataset is also subject to X Terms of Use.
### Citation Information
If you use this dataset in your research, please cite it as follows:
```
@misc{john-11112025datauniversex_dataset_060792,
title={The Data Universe Datasets: The finest collection of social media data the web has to offer},
author={john-1111},
year={2025},
url={https://huggingface.co/datasets/john-1111/x_dataset_060792},
}
```
### Contributions
To report issues or contribute to the dataset, please contact the miner or use the Bittensor Subnet 13 governance mechanisms.
## Dataset Statistics
[This section is automatically updated]
- **Total Instances:** 3197762
- **Date Range:** 2025-01-02T00:00:00Z to 2025-04-25T00:00:00Z
- **Last Updated:** 2025-05-05T01:29:48Z
### Data Distribution
- Tweets with hashtags: 5.04%
- Tweets without hashtags: 94.96%
### Top 10 Hashtags
For full statistics, please refer to the `stats.json` file in the repository.
| Rank | Topic | Total Count | Percentage |
|------|-------|-------------|-------------|
| 1 | NULL | 1261593 | 88.67% |
| 2 | #granhermano | 10301 | 0.72% |
| 3 | #riyadh | 9374 | 0.66% |
| 4 | #箱根駅伝 | 8147 | 0.57% |
| 5 | #thameposeriesep9 | 7605 | 0.53% |
| 6 | #tiktok | 6765 | 0.48% |
| 7 | #ad | 5367 | 0.38% |
| 8 | #zelena | 4878 | 0.34% |
| 9 | #smackdown | 4844 | 0.34% |
| 10 | #कबीर_परमेश्वर_निर्वाण_दिवस | 4843 | 0.34% |
## Update History
| Date | New Instances | Total Instances |
|------|---------------|-----------------|
| 2025-01-25T07:14:13Z | 414446 | 414446 |
| 2025-01-25T07:14:44Z | 453526 | 867972 |
| 2025-01-25T07:15:15Z | 453526 | 1321498 |
| 2025-01-25T07:15:45Z | 453526 | 1775024 |
| 2025-01-25T07:16:15Z | 453526 | 2228550 |
| 2025-02-18T03:38:58Z | 471834 | 2700384 |
| 2025-05-05T01:29:48Z | 497378 | 3197762 |
|
rainbowbridge/x_dataset_57071 | rainbowbridge | 2025-05-05T01:11:58Z | 1,300 | 0 | [
"task_categories:text-classification",
"task_categories:token-classification",
"task_categories:question-answering",
"task_categories:summarization",
"task_categories:text-generation",
"task_ids:sentiment-analysis",
"task_ids:topic-classification",
"task_ids:named-entity-recognition",
"task_ids:language-modeling",
"task_ids:text-scoring",
"task_ids:multi-class-classification",
"task_ids:multi-label-classification",
"task_ids:extractive-qa",
"task_ids:news-articles-summarization",
"multilinguality:multilingual",
"source_datasets:original",
"license:mit",
"size_categories:100M<n<1B",
"format:parquet",
"modality:text",
"library:datasets",
"library:dask",
"library:mlcroissant",
"library:polars",
"region:us"
] | [
"text-classification",
"token-classification",
"question-answering",
"summarization",
"text-generation"
] | 2025-01-27T00:25:54Z | null | ---
license: mit
multilinguality:
- multilingual
source_datasets:
- original
task_categories:
- text-classification
- token-classification
- question-answering
- summarization
- text-generation
task_ids:
- sentiment-analysis
- topic-classification
- named-entity-recognition
- language-modeling
- text-scoring
- multi-class-classification
- multi-label-classification
- extractive-qa
- news-articles-summarization
---
# Bittensor Subnet 13 X (Twitter) Dataset
<center>
<img src="https://huggingface.co/datasets/macrocosm-os/images/resolve/main/bittensor.png" alt="Data-universe: The finest collection of social media data the web has to offer">
</center>
<center>
<img src="https://huggingface.co/datasets/macrocosm-os/images/resolve/main/macrocosmos-black.png" alt="Data-universe: The finest collection of social media data the web has to offer">
</center>
## Dataset Description
- **Repository:** rainbowbridge/x_dataset_57071
- **Subnet:** Bittensor Subnet 13
- **Miner Hotkey:** 5F2S4Xnn1UqWXhWmdu1kgfeu1ZpFoQEYbxF8oCNpRHnMZNar
### Dataset Summary
This dataset is part of the Bittensor Subnet 13 decentralized network, containing preprocessed data from X (formerly Twitter). The data is continuously updated by network miners, providing a real-time stream of tweets for various analytical and machine learning tasks.
For more information about the dataset, please visit the [official repository](https://github.com/macrocosm-os/data-universe).
### Supported Tasks
The versatility of this dataset allows researchers and data scientists to explore various aspects of social media dynamics and develop innovative applications. Users are encouraged to leverage this data creatively for their specific research or business needs.
For example:
- Sentiment Analysis
- Trend Detection
- Content Analysis
- User Behavior Modeling
### Languages
Primary language: Datasets are mostly English, but can be multilingual due to decentralized ways of creation.
## Dataset Structure
### Data Instances
Each instance represents a single tweet with the following fields:
### Data Fields
- `text` (string): The main content of the tweet.
- `label` (string): Sentiment or topic category of the tweet.
- `tweet_hashtags` (list): A list of hashtags used in the tweet. May be empty if no hashtags are present.
- `datetime` (string): The date when the tweet was posted.
- `username_encoded` (string): An encoded version of the username to maintain user privacy.
- `url_encoded` (string): An encoded version of any URLs included in the tweet. May be empty if no URLs are present.
### Data Splits
This dataset is continuously updated and does not have fixed splits. Users should create their own splits based on their requirements and the data's timestamp.
## Dataset Creation
### Source Data
Data is collected from public tweets on X (Twitter), adhering to the platform's terms of service and API usage guidelines.
### Personal and Sensitive Information
All usernames and URLs are encoded to protect user privacy. The dataset does not intentionally include personal or sensitive information.
## Considerations for Using the Data
### Social Impact and Biases
Users should be aware of potential biases inherent in X (Twitter) data, including demographic and content biases. This dataset reflects the content and opinions expressed on X and should not be considered a representative sample of the general population.
### Limitations
- Data quality may vary due to the decentralized nature of collection and preprocessing.
- The dataset may contain noise, spam, or irrelevant content typical of social media platforms.
- Temporal biases may exist due to real-time collection methods.
- The dataset is limited to public tweets and does not include private accounts or direct messages.
- Not all tweets contain hashtags or URLs.
## Additional Information
### Licensing Information
The dataset is released under the MIT license. The use of this dataset is also subject to X Terms of Use.
### Citation Information
If you use this dataset in your research, please cite it as follows:
```
@misc{rainbowbridge2025datauniversex_dataset_57071,
title={The Data Universe Datasets: The finest collection of social media data the web has to offer},
author={rainbowbridge},
year={2025},
url={https://huggingface.co/datasets/rainbowbridge/x_dataset_57071},
}
```
### Contributions
To report issues or contribute to the dataset, please contact the miner or use the Bittensor Subnet 13 governance mechanisms.
## Dataset Statistics
[This section is automatically updated]
- **Total Instances:** 46728701
- **Date Range:** 2025-01-21T00:00:00Z to 2025-02-09T00:00:00Z
- **Last Updated:** 2025-02-18T18:45:20Z
### Data Distribution
- Tweets with hashtags: 43.62%
- Tweets without hashtags: 56.38%
### Top 10 Hashtags
For full statistics, please refer to the `stats.json` file in the repository.
| Rank | Topic | Total Count | Percentage |
|------|-------|-------------|-------------|
| 1 | NULL | 26346933 | 56.38% |
| 2 | #riyadh | 326612 | 0.70% |
| 3 | #zelena | 248547 | 0.53% |
| 4 | #tiktok | 199186 | 0.43% |
| 5 | #bbb25 | 123042 | 0.26% |
| 6 | #ad | 115507 | 0.25% |
| 7 | #granhermano | 68204 | 0.15% |
| 8 | #jhope_at_galadespiècesjaunes | 67706 | 0.14% |
| 9 | #bbmzansi | 63947 | 0.14% |
| 10 | #pr | 61448 | 0.13% |
## Update History
| Date | New Instances | Total Instances |
|------|---------------|-----------------|
| 2025-01-27T00:26:55Z | 3588990 | 3588990 |
| 2025-01-30T12:29:25Z | 8527338 | 12116328 |
| 2025-02-03T00:32:41Z | 9724909 | 21841237 |
| 2025-02-06T12:35:39Z | 7123646 | 28964883 |
| 2025-02-10T00:39:12Z | 9349448 | 38314331 |
| 2025-02-13T12:43:01Z | 6970444 | 45284775 |
| 2025-02-18T03:44:05Z | 636505 | 45921280 |
| 2025-02-18T18:45:20Z | 807421 | 46728701 |
|
marry-1111/x_dataset_0502178 | marry-1111 | 2025-05-05T01:07:56Z | 316 | 0 | [
"task_categories:text-classification",
"task_categories:token-classification",
"task_categories:question-answering",
"task_categories:summarization",
"task_categories:text-generation",
"task_ids:sentiment-analysis",
"task_ids:topic-classification",
"task_ids:named-entity-recognition",
"task_ids:language-modeling",
"task_ids:text-scoring",
"task_ids:multi-class-classification",
"task_ids:multi-label-classification",
"task_ids:extractive-qa",
"task_ids:news-articles-summarization",
"multilinguality:multilingual",
"source_datasets:original",
"license:mit",
"size_categories:10M<n<100M",
"format:parquet",
"modality:text",
"library:datasets",
"library:dask",
"library:mlcroissant",
"library:polars",
"region:us"
] | [
"text-classification",
"token-classification",
"question-answering",
"summarization",
"text-generation"
] | 2025-01-25T07:14:45Z | null | ---
license: mit
multilinguality:
- multilingual
source_datasets:
- original
task_categories:
- text-classification
- token-classification
- question-answering
- summarization
- text-generation
task_ids:
- sentiment-analysis
- topic-classification
- named-entity-recognition
- language-modeling
- text-scoring
- multi-class-classification
- multi-label-classification
- extractive-qa
- news-articles-summarization
---
# Bittensor Subnet 13 X (Twitter) Dataset
<center>
<img src="https://huggingface.co/datasets/macrocosm-os/images/resolve/main/bittensor.png" alt="Data-universe: The finest collection of social media data the web has to offer">
</center>
<center>
<img src="https://huggingface.co/datasets/macrocosm-os/images/resolve/main/macrocosmos-black.png" alt="Data-universe: The finest collection of social media data the web has to offer">
</center>
## Dataset Description
- **Repository:** marry-1111/x_dataset_0502178
- **Subnet:** Bittensor Subnet 13
- **Miner Hotkey:** 5HmfhkSP5knw1QcrE8udotNj1C9JD2rriUKtWT7DmigBdr8A
### Miner Data Compliance Agreement
In uploading this dataset, I am agreeing to the [Macrocosmos Miner Data Compliance Policy](https://github.com/macrocosm-os/data-universe/blob/add-miner-policy/docs/miner_policy.md).
### Dataset Summary
This dataset is part of the Bittensor Subnet 13 decentralized network, containing preprocessed data from X (formerly Twitter). The data is continuously updated by network miners, providing a real-time stream of tweets for various analytical and machine learning tasks.
For more information about the dataset, please visit the [official repository](https://github.com/macrocosm-os/data-universe).
### Supported Tasks
The versatility of this dataset allows researchers and data scientists to explore various aspects of social media dynamics and develop innovative applications. Users are encouraged to leverage this data creatively for their specific research or business needs.
For example:
- Sentiment Analysis
- Trend Detection
- Content Analysis
- User Behavior Modeling
### Languages
Primary language: Datasets are mostly English, but can be multilingual due to decentralized ways of creation.
## Dataset Structure
### Data Instances
Each instance represents a single tweet with the following fields:
### Data Fields
- `text` (string): The main content of the tweet.
- `label` (string): Sentiment or topic category of the tweet.
- `tweet_hashtags` (list): A list of hashtags used in the tweet. May be empty if no hashtags are present.
- `datetime` (string): The date when the tweet was posted.
- `username_encoded` (string): An encoded version of the username to maintain user privacy.
- `url_encoded` (string): An encoded version of any URLs included in the tweet. May be empty if no URLs are present.
### Data Splits
This dataset is continuously updated and does not have fixed splits. Users should create their own splits based on their requirements and the data's timestamp.
## Dataset Creation
### Source Data
Data is collected from public tweets on X (Twitter), adhering to the platform's terms of service and API usage guidelines.
### Personal and Sensitive Information
All usernames and URLs are encoded to protect user privacy. The dataset does not intentionally include personal or sensitive information.
## Considerations for Using the Data
### Social Impact and Biases
Users should be aware of potential biases inherent in X (Twitter) data, including demographic and content biases. This dataset reflects the content and opinions expressed on X and should not be considered a representative sample of the general population.
### Limitations
- Data quality may vary due to the decentralized nature of collection and preprocessing.
- The dataset may contain noise, spam, or irrelevant content typical of social media platforms.
- Temporal biases may exist due to real-time collection methods.
- The dataset is limited to public tweets and does not include private accounts or direct messages.
- Not all tweets contain hashtags or URLs.
## Additional Information
### Licensing Information
The dataset is released under the MIT license. The use of this dataset is also subject to X Terms of Use.
### Citation Information
If you use this dataset in your research, please cite it as follows:
```
@misc{marry-11112025datauniversex_dataset_0502178,
title={The Data Universe Datasets: The finest collection of social media data the web has to offer},
author={marry-1111},
year={2025},
url={https://huggingface.co/datasets/marry-1111/x_dataset_0502178},
}
```
### Contributions
To report issues or contribute to the dataset, please contact the miner or use the Bittensor Subnet 13 governance mechanisms.
## Dataset Statistics
[This section is automatically updated]
- **Total Instances:** 3234371
- **Date Range:** 2025-01-02T00:00:00Z to 2025-04-25T00:00:00Z
- **Last Updated:** 2025-05-05T01:07:55Z
### Data Distribution
- Tweets with hashtags: 4.32%
- Tweets without hashtags: 95.68%
### Top 10 Hashtags
For full statistics, please refer to the `stats.json` file in the repository.
| Rank | Topic | Total Count | Percentage |
|------|-------|-------------|-------------|
| 1 | NULL | 1261593 | 90.03% |
| 2 | #granhermano | 10301 | 0.74% |
| 3 | #箱根駅伝 | 8147 | 0.58% |
| 4 | #thameposeriesep9 | 7605 | 0.54% |
| 5 | #tiktok | 5032 | 0.36% |
| 6 | #zelena | 4878 | 0.35% |
| 7 | #smackdown | 4844 | 0.35% |
| 8 | #कबीर_परमेश्वर_निर्वाण_दिवस | 4843 | 0.35% |
| 9 | #ad | 3533 | 0.25% |
| 10 | #delhielectionresults | 3476 | 0.25% |
## Update History
| Date | New Instances | Total Instances |
|------|---------------|-----------------|
| 2025-01-25T07:13:13Z | 454010 | 454010 |
| 2025-01-25T07:13:46Z | 471976 | 925986 |
| 2025-01-25T07:14:15Z | 453526 | 1379512 |
| 2025-01-25T07:14:44Z | 453526 | 1833038 |
| 2025-01-25T07:15:13Z | 453526 | 2286564 |
| 2025-02-18T03:39:05Z | 471834 | 2758398 |
| 2025-05-05T01:07:55Z | 475973 | 3234371 |
|
robert-1111/x_dataset_040752 | robert-1111 | 2025-05-05T00:55:52Z | 165 | 0 | [
"task_categories:text-classification",
"task_categories:token-classification",
"task_categories:question-answering",
"task_categories:summarization",
"task_categories:text-generation",
"task_ids:sentiment-analysis",
"task_ids:topic-classification",
"task_ids:named-entity-recognition",
"task_ids:language-modeling",
"task_ids:text-scoring",
"task_ids:multi-class-classification",
"task_ids:multi-label-classification",
"task_ids:extractive-qa",
"task_ids:news-articles-summarization",
"multilinguality:multilingual",
"source_datasets:original",
"license:mit",
"size_categories:10M<n<100M",
"format:parquet",
"modality:text",
"library:datasets",
"library:dask",
"library:mlcroissant",
"library:polars",
"region:us"
] | [
"text-classification",
"token-classification",
"question-answering",
"summarization",
"text-generation"
] | 2025-01-25T07:11:28Z | null | ---
license: mit
multilinguality:
- multilingual
source_datasets:
- original
task_categories:
- text-classification
- token-classification
- question-answering
- summarization
- text-generation
task_ids:
- sentiment-analysis
- topic-classification
- named-entity-recognition
- language-modeling
- text-scoring
- multi-class-classification
- multi-label-classification
- extractive-qa
- news-articles-summarization
---
# Bittensor Subnet 13 X (Twitter) Dataset
<center>
<img src="https://huggingface.co/datasets/macrocosm-os/images/resolve/main/bittensor.png" alt="Data-universe: The finest collection of social media data the web has to offer">
</center>
<center>
<img src="https://huggingface.co/datasets/macrocosm-os/images/resolve/main/macrocosmos-black.png" alt="Data-universe: The finest collection of social media data the web has to offer">
</center>
## Dataset Description
- **Repository:** robert-1111/x_dataset_040752
- **Subnet:** Bittensor Subnet 13
- **Miner Hotkey:** 5Gq8xaWKd8cNHFkD8Mt38BjL1dzBGi8ZhdfMskmv3v2H5hLC
### Miner Data Compliance Agreement
In uploading this dataset, I am agreeing to the [Macrocosmos Miner Data Compliance Policy](https://github.com/macrocosm-os/data-universe/blob/add-miner-policy/docs/miner_policy.md).
### Dataset Summary
This dataset is part of the Bittensor Subnet 13 decentralized network, containing preprocessed data from X (formerly Twitter). The data is continuously updated by network miners, providing a real-time stream of tweets for various analytical and machine learning tasks.
For more information about the dataset, please visit the [official repository](https://github.com/macrocosm-os/data-universe).
### Supported Tasks
The versatility of this dataset allows researchers and data scientists to explore various aspects of social media dynamics and develop innovative applications. Users are encouraged to leverage this data creatively for their specific research or business needs.
For example:
- Sentiment Analysis
- Trend Detection
- Content Analysis
- User Behavior Modeling
### Languages
Primary language: Datasets are mostly English, but can be multilingual due to decentralized ways of creation.
## Dataset Structure
### Data Instances
Each instance represents a single tweet with the following fields:
### Data Fields
- `text` (string): The main content of the tweet.
- `label` (string): Sentiment or topic category of the tweet.
- `tweet_hashtags` (list): A list of hashtags used in the tweet. May be empty if no hashtags are present.
- `datetime` (string): The date when the tweet was posted.
- `username_encoded` (string): An encoded version of the username to maintain user privacy.
- `url_encoded` (string): An encoded version of any URLs included in the tweet. May be empty if no URLs are present.
### Data Splits
This dataset is continuously updated and does not have fixed splits. Users should create their own splits based on their requirements and the data's timestamp.
## Dataset Creation
### Source Data
Data is collected from public tweets on X (Twitter), adhering to the platform's terms of service and API usage guidelines.
### Personal and Sensitive Information
All usernames and URLs are encoded to protect user privacy. The dataset does not intentionally include personal or sensitive information.
## Considerations for Using the Data
### Social Impact and Biases
Users should be aware of potential biases inherent in X (Twitter) data, including demographic and content biases. This dataset reflects the content and opinions expressed on X and should not be considered a representative sample of the general population.
### Limitations
- Data quality may vary due to the decentralized nature of collection and preprocessing.
- The dataset may contain noise, spam, or irrelevant content typical of social media platforms.
- Temporal biases may exist due to real-time collection methods.
- The dataset is limited to public tweets and does not include private accounts or direct messages.
- Not all tweets contain hashtags or URLs.
## Additional Information
### Licensing Information
The dataset is released under the MIT license. The use of this dataset is also subject to X Terms of Use.
### Citation Information
If you use this dataset in your research, please cite it as follows:
```
@misc{robert-11112025datauniversex_dataset_040752,
title={The Data Universe Datasets: The finest collection of social media data the web has to offer},
author={robert-1111},
year={2025},
url={https://huggingface.co/datasets/robert-1111/x_dataset_040752},
}
```
### Contributions
To report issues or contribute to the dataset, please contact the miner or use the Bittensor Subnet 13 governance mechanisms.
## Dataset Statistics
[This section is automatically updated]
- **Total Instances:** 2636450
- **Date Range:** 2025-01-02T00:00:00Z to 2025-04-25T00:00:00Z
- **Last Updated:** 2025-05-05T00:55:52Z
### Data Distribution
- Tweets with hashtags: 5.36%
- Tweets without hashtags: 94.64%
### Top 10 Hashtags
For full statistics, please refer to the `stats.json` file in the repository.
| Rank | Topic | Total Count | Percentage |
|------|-------|-------------|-------------|
| 1 | NULL | 1251882 | 89.86% |
| 2 | #箱根駅伝 | 8147 | 0.58% |
| 3 | #thameposeriesep9 | 7605 | 0.55% |
| 4 | #riyadh | 7255 | 0.52% |
| 5 | #tiktok | 6802 | 0.49% |
| 6 | #nfldraft2025 | 6802 | 0.49% |
| 7 | #ad | 5266 | 0.38% |
| 8 | #zelena | 4878 | 0.35% |
| 9 | #smackdown | 4844 | 0.35% |
| 10 | #कबीर_परमेश्वर_निर्वाण_दिवस | 4843 | 0.35% |
## Update History
| Date | New Instances | Total Instances |
|------|---------------|-----------------|
| 2025-01-25T07:10:27Z | 414446 | 414446 |
| 2025-01-25T07:10:56Z | 414446 | 828892 |
| 2025-01-25T07:11:27Z | 414446 | 1243338 |
| 2025-01-25T07:11:56Z | 453526 | 1696864 |
| 2025-02-18T03:38:23Z | 471834 | 2168698 |
| 2025-05-05T00:55:52Z | 467752 | 2636450 |
|
semran1/calibration_test | semran1 | 2025-05-05T00:48:33Z | 0 | 0 | [
"region:us"
] | [] | 2025-05-05T00:48:21Z | null | ---
dataset_info:
features:
- name: text
dtype: string
- name: cc-path
dtype: string
- name: domain
dtype: string
- name: lang
dtype: string
- name: lang_score
dtype: float64
- name: timestamp
dtype: string
- name: url
dtype: string
- name: math_score
dtype: float64
- name: type
dtype: string
splits:
- name: train
num_bytes: 205426140.0
num_examples: 50000
download_size: 106854338
dataset_size: 205426140.0
configs:
- config_name: default
data_files:
- split: train
path: data/train-*
---
|
ieuniversity/group_7_submission | ieuniversity | 2025-05-05T00:11:00Z | 403 | 0 | [
"size_categories:10K<n<100K",
"format:parquet",
"modality:text",
"library:datasets",
"library:pandas",
"library:mlcroissant",
"library:polars",
"region:us"
] | [] | 2025-02-19T13:43:26Z | null | ---
dataset_info:
features:
- name: ID
dtype: int64
- name: CLASE
dtype: string
splits:
- name: train
num_bytes: 580197
num_examples: 25808
download_size: 176229
dataset_size: 580197
configs:
- config_name: default
data_files:
- split: train
path: data/train-*
---
|
sophiayk20/repetition-one-speaker | sophiayk20 | 2025-05-04T23:31:47Z | 0 | 0 | [
"region:us"
] | [] | 2025-05-04T23:27:58Z | null | ---
dataset_info:
features:
- name: id
dtype: string
- name: dialogue
dtype: string
- name: disfluent_dialogue
dtype: string
- name: summary
dtype: string
splits:
- name: ATAS
num_bytes: 2373554
num_examples: 1500
- name: ATOS
num_bytes: 2373554
num_examples: 1500
- name: OTAS
num_bytes: 2666795
num_examples: 1500
- name: OTOS
num_bytes: 2666795
num_examples: 1500
download_size: 2126064
dataset_size: 10080698
configs:
- config_name: default
data_files:
- split: ATAS
path: data/ATAS-*
- split: ATOS
path: data/ATOS-*
- split: OTAS
path: data/OTAS-*
- split: OTOS
path: data/OTOS-*
---
|
sortl005/Superconductor | sortl005 | 2025-05-04T23:30:39Z | 0 | 0 | [
"region:us"
] | [] | 2025-05-04T23:30:29Z | null | ---
dataset_info:
features:
- name: x
sequence: float64
- name: y
dtype: float64
splits:
- name: train
num_bytes: 10716300
num_examples: 15309
download_size: 290587
dataset_size: 10716300
configs:
- config_name: default
data_files:
- split: train
path: data/train-*
---
|
marcuscedricridia/OpenMathInstruct-2-sampled-balanced | marcuscedricridia | 2025-05-04T23:28:57Z | 0 | 0 | [
"region:us"
] | [] | 2025-05-04T23:28:02Z | null | ---
dataset_info:
features:
- name: problem
dtype: string
- name: generated_solution
dtype: string
- name: problem_source
dtype: string
splits:
- name: train
num_bytes: 1103869.0114952696
num_examples: 1000
download_size: 450651
dataset_size: 1103869.0114952696
configs:
- config_name: default
data_files:
- split: train
path: data/train-*
---
|
momo1942/x_dataset_19124 | momo1942 | 2025-05-04T23:21:24Z | 1,239 | 0 | [
"task_categories:text-classification",
"task_categories:token-classification",
"task_categories:question-answering",
"task_categories:summarization",
"task_categories:text-generation",
"task_ids:sentiment-analysis",
"task_ids:topic-classification",
"task_ids:named-entity-recognition",
"task_ids:language-modeling",
"task_ids:text-scoring",
"task_ids:multi-class-classification",
"task_ids:multi-label-classification",
"task_ids:extractive-qa",
"task_ids:news-articles-summarization",
"multilinguality:multilingual",
"source_datasets:original",
"license:mit",
"size_categories:100M<n<1B",
"format:parquet",
"modality:text",
"library:datasets",
"library:dask",
"library:mlcroissant",
"library:polars",
"region:us"
] | [
"text-classification",
"token-classification",
"question-answering",
"summarization",
"text-generation"
] | 2025-01-27T06:34:57Z | null | ---
license: mit
multilinguality:
- multilingual
source_datasets:
- original
task_categories:
- text-classification
- token-classification
- question-answering
- summarization
- text-generation
task_ids:
- sentiment-analysis
- topic-classification
- named-entity-recognition
- language-modeling
- text-scoring
- multi-class-classification
- multi-label-classification
- extractive-qa
- news-articles-summarization
---
# Bittensor Subnet 13 X (Twitter) Dataset
<center>
<img src="https://huggingface.co/datasets/macrocosm-os/images/resolve/main/bittensor.png" alt="Data-universe: The finest collection of social media data the web has to offer">
</center>
<center>
<img src="https://huggingface.co/datasets/macrocosm-os/images/resolve/main/macrocosmos-black.png" alt="Data-universe: The finest collection of social media data the web has to offer">
</center>
## Dataset Description
- **Repository:** momo1942/x_dataset_19124
- **Subnet:** Bittensor Subnet 13
- **Miner Hotkey:** 5FvdP3P4KfWBM4YSPM5SaL5eGThA6g2NUwvPzMeCq6WRY9TD
### Dataset Summary
This dataset is part of the Bittensor Subnet 13 decentralized network, containing preprocessed data from X (formerly Twitter). The data is continuously updated by network miners, providing a real-time stream of tweets for various analytical and machine learning tasks.
For more information about the dataset, please visit the [official repository](https://github.com/macrocosm-os/data-universe).
### Supported Tasks
The versatility of this dataset allows researchers and data scientists to explore various aspects of social media dynamics and develop innovative applications. Users are encouraged to leverage this data creatively for their specific research or business needs.
For example:
- Sentiment Analysis
- Trend Detection
- Content Analysis
- User Behavior Modeling
### Languages
Primary language: Datasets are mostly English, but can be multilingual due to decentralized ways of creation.
## Dataset Structure
### Data Instances
Each instance represents a single tweet with the following fields:
### Data Fields
- `text` (string): The main content of the tweet.
- `label` (string): Sentiment or topic category of the tweet.
- `tweet_hashtags` (list): A list of hashtags used in the tweet. May be empty if no hashtags are present.
- `datetime` (string): The date when the tweet was posted.
- `username_encoded` (string): An encoded version of the username to maintain user privacy.
- `url_encoded` (string): An encoded version of any URLs included in the tweet. May be empty if no URLs are present.
### Data Splits
This dataset is continuously updated and does not have fixed splits. Users should create their own splits based on their requirements and the data's timestamp.
## Dataset Creation
### Source Data
Data is collected from public tweets on X (Twitter), adhering to the platform's terms of service and API usage guidelines.
### Personal and Sensitive Information
All usernames and URLs are encoded to protect user privacy. The dataset does not intentionally include personal or sensitive information.
## Considerations for Using the Data
### Social Impact and Biases
Users should be aware of potential biases inherent in X (Twitter) data, including demographic and content biases. This dataset reflects the content and opinions expressed on X and should not be considered a representative sample of the general population.
### Limitations
- Data quality may vary due to the decentralized nature of collection and preprocessing.
- The dataset may contain noise, spam, or irrelevant content typical of social media platforms.
- Temporal biases may exist due to real-time collection methods.
- The dataset is limited to public tweets and does not include private accounts or direct messages.
- Not all tweets contain hashtags or URLs.
## Additional Information
### Licensing Information
The dataset is released under the MIT license. The use of this dataset is also subject to X Terms of Use.
### Citation Information
If you use this dataset in your research, please cite it as follows:
```
@misc{momo19422025datauniversex_dataset_19124,
title={The Data Universe Datasets: The finest collection of social media data the web has to offer},
author={momo1942},
year={2025},
url={https://huggingface.co/datasets/momo1942/x_dataset_19124},
}
```
### Contributions
To report issues or contribute to the dataset, please contact the miner or use the Bittensor Subnet 13 governance mechanisms.
## Dataset Statistics
[This section is automatically updated]
- **Total Instances:** 58904296
- **Date Range:** 2025-01-21T00:00:00Z to 2025-02-12T00:00:00Z
- **Last Updated:** 2025-02-18T19:00:41Z
### Data Distribution
- Tweets with hashtags: 42.09%
- Tweets without hashtags: 57.91%
### Top 10 Hashtags
For full statistics, please refer to the `stats.json` file in the repository.
| Rank | Topic | Total Count | Percentage |
|------|-------|-------------|-------------|
| 1 | NULL | 34109059 | 57.91% |
| 2 | #riyadh | 360800 | 0.61% |
| 3 | #zelena | 305824 | 0.52% |
| 4 | #tiktok | 239491 | 0.41% |
| 5 | #bbb25 | 173195 | 0.29% |
| 6 | #ad | 137871 | 0.23% |
| 7 | #granhermano | 94348 | 0.16% |
| 8 | #bbmzansi | 80293 | 0.14% |
| 9 | #jhope_at_galadespiècesjaunes | 74039 | 0.13% |
| 10 | #pr | 72837 | 0.12% |
## Update History
| Date | New Instances | Total Instances |
|------|---------------|-----------------|
| 2025-01-27T06:36:14Z | 4698495 | 4698495 |
| 2025-01-30T18:39:09Z | 9621596 | 14320091 |
| 2025-02-03T06:43:27Z | 12152156 | 26472247 |
| 2025-02-06T18:48:17Z | 12216961 | 38689208 |
| 2025-02-10T06:51:17Z | 6273827 | 44963035 |
| 2025-02-13T18:56:05Z | 12477634 | 57440669 |
| 2025-02-18T03:59:19Z | 829865 | 58270534 |
| 2025-02-18T19:00:41Z | 633762 | 58904296 |
|
asafxrev/so100_jenga_box_simple | asafxrev | 2025-05-04T22:39:48Z | 0 | 0 | [
"task_categories:robotics",
"license:apache-2.0",
"region:us",
"LeRobot",
"so100",
"tutorial"
] | [
"robotics"
] | 2025-05-04T22:39:45Z | null | ---
license: apache-2.0
task_categories:
- robotics
tags:
- LeRobot
- so100
- tutorial
configs:
- config_name: default
data_files: data/*/*.parquet
---
This dataset was created using [LeRobot](https://github.com/huggingface/lerobot).
## Dataset Description
- **Homepage:** [More Information Needed]
- **Paper:** [More Information Needed]
- **License:** apache-2.0
## Dataset Structure
[meta/info.json](meta/info.json):
```json
{
"codebase_version": "v2.1",
"robot_type": "so100",
"total_episodes": 5,
"total_frames": 1711,
"total_tasks": 1,
"total_videos": 5,
"total_chunks": 1,
"chunks_size": 1000,
"fps": 30,
"splits": {
"train": "0:5"
},
"data_path": "data/chunk-{episode_chunk:03d}/episode_{episode_index:06d}.parquet",
"video_path": "videos/chunk-{episode_chunk:03d}/{video_key}/episode_{episode_index:06d}.mp4",
"features": {
"action": {
"dtype": "float32",
"shape": [
6
],
"names": [
"main_shoulder_pan",
"main_shoulder_lift",
"main_elbow_flex",
"main_wrist_flex",
"main_wrist_roll",
"main_gripper"
]
},
"observation.state": {
"dtype": "float32",
"shape": [
6
],
"names": [
"main_shoulder_pan",
"main_shoulder_lift",
"main_elbow_flex",
"main_wrist_flex",
"main_wrist_roll",
"main_gripper"
]
},
"observation.images.follower_cam": {
"dtype": "video",
"shape": [
480,
640,
3
],
"names": [
"height",
"width",
"channels"
],
"info": {
"video.height": 480,
"video.width": 640,
"video.codec": "av1",
"video.pix_fmt": "yuv420p",
"video.is_depth_map": false,
"video.fps": 30,
"video.channels": 3,
"has_audio": false
}
},
"timestamp": {
"dtype": "float32",
"shape": [
1
],
"names": null
},
"frame_index": {
"dtype": "int64",
"shape": [
1
],
"names": null
},
"episode_index": {
"dtype": "int64",
"shape": [
1
],
"names": null
},
"index": {
"dtype": "int64",
"shape": [
1
],
"names": null
},
"task_index": {
"dtype": "int64",
"shape": [
1
],
"names": null
}
}
}
```
## Citation
**BibTeX:**
```bibtex
[More Information Needed]
``` |
mehmet0001/github-commits-dataset | mehmet0001 | 2025-05-04T21:23:38Z | 0 | 0 | [
"region:us"
] | [] | 2025-05-04T21:23:18Z | null | ---
dataset_info:
features:
- name: text
dtype: string
splits:
- name: train
num_bytes: 1325874280
num_examples: 91646
download_size: 393630553
dataset_size: 1325874280
configs:
- config_name: default
data_files:
- split: train
path: data/train-*
---
|
MBZUAI-IFM/riddlesenseplusplus | MBZUAI-IFM | 2025-05-04T20:52:38Z | 0 | 0 | [
"region:us"
] | [] | 2025-05-04T20:52:36Z | null | ---
dataset_info:
features:
- name: conversations
list:
- name: from
dtype: string
- name: value
dtype: string
- name: metadata
dtype: string
- name: dataset_source
dtype: string
splits:
- name: train
num_bytes: 13981243
num_examples: 3508
download_size: 6754856
dataset_size: 13981243
configs:
- config_name: default
data_files:
- split: train
path: data/train-*
---
|
xbilek25/train_hall_absorb_0.7_14400_18000 | xbilek25 | 2025-05-04T20:48:22Z | 0 | 0 | [
"size_categories:1K<n<10K",
"format:parquet",
"modality:audio",
"modality:text",
"library:datasets",
"library:dask",
"library:mlcroissant",
"library:polars",
"region:us"
] | [] | 2025-05-04T16:38:08Z | null | ---
dataset_info:
features:
- name: client_id
dtype: string
- name: path
dtype: string
- name: audio
dtype:
audio:
sampling_rate: 16000
- name: sentence
dtype: string
- name: up_votes
dtype: int64
- name: down_votes
dtype: int64
- name: age
dtype: string
- name: gender
dtype: string
- name: accent
dtype: string
- name: locale
dtype: string
- name: segment
dtype: string
- name: variant
dtype: string
splits:
- name: train
num_bytes: 719853524.0
num_examples: 3600
download_size: 564525641
dataset_size: 719853524.0
configs:
- config_name: default
data_files:
- split: train
path: data/train-*
---
|
HungVu2003/opt-350m_beta_0.0_alpha_0.2_num-company_2_dataset_1_for_gen_11_v2 | HungVu2003 | 2025-05-04T20:33:24Z | 0 | 0 | [
"region:us"
] | [] | 2025-05-04T20:33:22Z | null | ---
dataset_info:
features:
- name: question
dtype: string
splits:
- name: train
num_bytes: 3159133
num_examples: 13750
download_size: 992842
dataset_size: 3159133
configs:
- config_name: default
data_files:
- split: train
path: data/train-*
---
|
doublesizebed/process_dataset_mini | doublesizebed | 2025-05-04T19:04:53Z | 3 | 0 | [
"size_categories:10K<n<100K",
"format:parquet",
"modality:audio",
"modality:text",
"library:datasets",
"library:dask",
"library:mlcroissant",
"library:polars",
"region:us"
] | [] | 2025-05-03T19:06:38Z | null | ---
dataset_info:
features:
- name: audio_filename
dtype: string
- name: prompt
dtype: string
- name: transcription
dtype: string
- name: gender
dtype: string
- name: audio_filepath
dtype: audio
- name: utterance_pitch_mean
dtype: float64
- name: utterance_pitch_std
dtype: float64
- name: snr
dtype: float64
- name: c50
dtype: float64
- name: speech_duration
dtype: float64
- name: speaking_rate
dtype: float64
- name: phonemes
dtype: string
splits:
- name: train
num_bytes: 1076070425.0
num_examples: 20000
download_size: 1073280024
dataset_size: 1076070425.0
configs:
- config_name: default
data_files:
- split: train
path: data/train-*
---
|
BasedLukas/so101_test_2 | BasedLukas | 2025-05-04T18:55:36Z | 0 | 0 | [
"task_categories:robotics",
"license:apache-2.0",
"region:us",
"LeRobot",
"so101",
"tutorial"
] | [
"robotics"
] | 2025-05-04T18:55:26Z | null | ---
license: apache-2.0
task_categories:
- robotics
tags:
- LeRobot
- so101
- tutorial
configs:
- config_name: default
data_files: data/*/*.parquet
---
This dataset was created using [LeRobot](https://github.com/huggingface/lerobot).
## Dataset Description
- **Homepage:** [More Information Needed]
- **Paper:** [More Information Needed]
- **License:** apache-2.0
## Dataset Structure
[meta/info.json](meta/info.json):
```json
{
"codebase_version": "v2.1",
"robot_type": "so101",
"total_episodes": 2,
"total_frames": 896,
"total_tasks": 1,
"total_videos": 4,
"total_chunks": 1,
"chunks_size": 1000,
"fps": 30,
"splits": {
"train": "0:2"
},
"data_path": "data/chunk-{episode_chunk:03d}/episode_{episode_index:06d}.parquet",
"video_path": "videos/chunk-{episode_chunk:03d}/{video_key}/episode_{episode_index:06d}.mp4",
"features": {
"action": {
"dtype": "float32",
"shape": [
6
],
"names": [
"main_shoulder_pan",
"main_shoulder_lift",
"main_elbow_flex",
"main_wrist_flex",
"main_wrist_roll",
"main_gripper"
]
},
"observation.state": {
"dtype": "float32",
"shape": [
6
],
"names": [
"main_shoulder_pan",
"main_shoulder_lift",
"main_elbow_flex",
"main_wrist_flex",
"main_wrist_roll",
"main_gripper"
]
},
"observation.images.laptop": {
"dtype": "video",
"shape": [
480,
640,
3
],
"names": [
"height",
"width",
"channels"
],
"info": {
"video.height": 480,
"video.width": 640,
"video.codec": "av1",
"video.pix_fmt": "yuv420p",
"video.is_depth_map": false,
"video.fps": 30,
"video.channels": 3,
"has_audio": false
}
},
"observation.images.phone": {
"dtype": "video",
"shape": [
480,
640,
3
],
"names": [
"height",
"width",
"channels"
],
"info": {
"video.height": 480,
"video.width": 640,
"video.codec": "av1",
"video.pix_fmt": "yuv420p",
"video.is_depth_map": false,
"video.fps": 30,
"video.channels": 3,
"has_audio": false
}
},
"timestamp": {
"dtype": "float32",
"shape": [
1
],
"names": null
},
"frame_index": {
"dtype": "int64",
"shape": [
1
],
"names": null
},
"episode_index": {
"dtype": "int64",
"shape": [
1
],
"names": null
},
"index": {
"dtype": "int64",
"shape": [
1
],
"names": null
},
"task_index": {
"dtype": "int64",
"shape": [
1
],
"names": null
}
}
}
```
## Citation
**BibTeX:**
```bibtex
[More Information Needed]
``` |
RafaelJaime/sas_opposition_exam_data | RafaelJaime | 2025-05-04T17:48:04Z | 376 | 0 | [
"language:es",
"license:apache-2.0",
"size_categories:10K<n<100K",
"format:parquet",
"modality:text",
"library:datasets",
"library:pandas",
"library:mlcroissant",
"library:polars",
"region:us",
"medical"
] | [] | 2025-03-21T14:57:39Z | null | ---
dataset_info:
features:
- name: statement
dtype: string
- name: answers
sequence: string
- name: correct_answer
dtype: string
- name: theme
dtype: string
- name: version
dtype: string
splits:
- name: train
num_bytes: 5128074
num_examples: 10712
download_size: 2407181
dataset_size: 5128074
configs:
- config_name: default
data_files:
- split: train
path: data/train-*
license: apache-2.0
language:
- es
tags:
- medical
---
# SAS Opposition Exam Dataset
This dataset contains questions and answers from all the exams of the SAS (Servicio Andaluz de Salud) public job offers. The questions and answers are sourced from the official webpage of the Andalusian Health Service [here](https://www.sspa.juntadeandalucia.es/servicioandaluzdesalud/profesionales/ofertas-de-empleo/oferta-de-empleo-publico-puestos-base/oep-extraordinaria-decreto-ley-122022-centros-sas/cuadro-de-evolucion-concurso-oposicion-centros-sas).
## Dataset Information
- **Statement**: The question in the exam.
- **Answers**: The possible answers for the question.
- **Real Answer**: The correct answer for the question.
- **Theme**: The topic or subject of the question.
### Dataset Creation Script
The script used to create this dataset can be found at: [generation_script.py](https://huggingface.co/datasets/RafaelJaime/sas_opposition_exam_data/blob/main/generation_script.py). |
zenml/llmops-database | zenml | 2025-05-04T17:25:45Z | 441 | 18 | [
"task_categories:feature-extraction",
"task_categories:summarization",
"task_categories:text-classification",
"task_categories:text-generation",
"task_ids:news-articles-summarization",
"task_ids:news-articles-headline-generation",
"task_ids:multi-class-classification",
"task_ids:multi-label-classification",
"task_ids:topic-classification",
"task_ids:language-modeling",
"annotations_creators:machine-generated",
"language_creators:found",
"multilinguality:monolingual",
"language:en",
"license:apache-2.0",
"size_categories:n<1K",
"format:parquet",
"modality:text",
"library:datasets",
"library:pandas",
"library:mlcroissant",
"library:polars",
"region:us",
"llmops",
"mlops",
"llms",
"production",
"devops",
"use-case",
"case-study"
] | [
"feature-extraction",
"summarization",
"text-classification",
"text-generation"
] | 2024-12-04T13:27:02Z | null | ---
annotations_creators:
- machine-generated
language:
- en
language_creators:
- found
license:
- apache-2.0
multilinguality:
- monolingual
pretty_name: LLMOps Database
size_categories:
- n<1K
source_datasets: []
tags:
- llmops
- mlops
- llms
- production
- devops
- use-case
- case-study
task_categories:
- feature-extraction
- summarization
- text-classification
- text-generation
task_ids:
- news-articles-summarization
- news-articles-headline-generation
- multi-class-classification
- multi-label-classification
- topic-classification
- language-modeling
configs:
- config_name: default
data_files:
- split: train
path: data/train-*
dataset_info:
features:
- name: created_at
dtype: string
- name: title
dtype: string
- name: industry
dtype: string
- name: year
dtype: int64
- name: source_url
dtype: string
- name: company
dtype: string
- name: application_tags
dtype: string
- name: tools_tags
dtype: string
- name: extra_tags
dtype: string
- name: techniques_tags
dtype: string
- name: short_summary
dtype: string
- name: full_summary
dtype: string
splits:
- name: train
num_bytes: 4088062
num_examples: 665
download_size: 1910660
dataset_size: 4088062
---
# The ZenML LLMOps Database

## Dataset Description
- **Browse dataset:** https://www.zenml.io/llmops-database
- **Launch blog post:** https://www.zenml.io/blog/demystifying-llmops-a-practical-database-of-real-world-generative-ai-implementations
- **Point of Contact:** llmopsdatabase at zenml.io
To learn more about ZenML and our open-source MLOps framework, visit
[zenml.io](https://zenml.io).
### Dataset Summary
The LLMOps Database is a comprehensive collection of over 500 real-world
generative AI implementations that showcases how organizations are successfully
deploying Large Language Models (LLMs) in production. The case studies have been
carefully curated to focus on technical depth and practical problem-solving,
with an emphasis on implementation details rather than marketing content. The
database aims to bridge the gap between theoretical discussions and practical
deployments, providing valuable insights for technical teams looking to
implement LLMs in production.
The LLMOps Database is maintained by the [ZenML](https://zenml.io) team. The
dataset is duplicated here on Hugging Face for those who would prefer to access
the data offline and/or browse it programmatically.
[](https://zenml.io)
### Usage Notes
- The full dataset is a Hugging Face `Dataset` which contains all the summaries
and metadata. Use this as you would any other Hugging Face `Dataset`. All the
entries are presented in a single split.
- Separately, the case studies are also presented as individual markdown files
inside this repository within the `markdown_data` folder. To browse and use
these locally you'll need to clone the repository.
- These markdown files have been concatenated into a single `.txt` file for your
convenience which is `all_data_single_file.txt` at the root of this
repository. You might want to play around with uploading this file into
[NotebookLM](https://notebooklm.google.com/), for example, or into a model
like Google's Gemini Pro which you can then use to in a chat interface. Note
that you'll have to use a model that can handle a very large context window
since as of currently writing this file contains around 200,000 words.
### Supported Tasks and Leaderboards
This dataset does not have any specific associated leaderboards or tasks. It is primarily intended as a resource for learning about real-world LLM deployments and the challenges and solutions involved.
### Languages
The case studies in the LLMOps database are exclusively in English.
## Dataset Structure
### Data Instances
A typical data instance in the LLMOps database includes the following fields:
```json
{
"created_at": "2024-12-03T13:19:00.000Z",
"title": "Scaling AI Image Animation System with Optimized Latency and Traffic Management",
"industry": "Tech",
"year": 2024,
"source_url": "https://engineering.fb.com/2024/08/14/production-engineering/how-meta-animates-ai-generated-images-at-scale/",
"company": "meta",
"application_tags": "realtime_application,high_stakes_application",
"tools_tags": "pytorch,monitoring,load_balancing,scaling,reliability,scalability",
"extra_tags": "pytorch,deployment,optimization,scaling,gpu,load balancing,traffic management,latency optimization,model distillation,inference",
"techniques_tags": "model_optimization,latency_optimization,cost_optimization,error_handling,fallback_strategies",
"short_summary": "Meta developed and deployed an AI-powered image animation feature that needed to serve billions of users efficiently. They tackled this challenge through a comprehensive optimization strategy including floating-point precision reduction, temporal-attention improvements, DPM-Solver implementation, and innovative distillation techniques. The system was further enhanced with sophisticated traffic management and load balancing solutions, resulting in a highly efficient, globally scalable service with minimal latency and failure rates.",
"full_summary": "# Meta: Scaling AI Image Animation System with Optimized Latency and Traffic Management (2024)\n\nhttps://engineering.fb.com/2024/08/14/production-engineering/how-meta-animates-ai-generated-images-at-scale/\n\n..."
}
```
The `full_summary` field contains a detailed writeup of the case study, which is truncated here for brevity.
### Data Fields
Each case study includes the following fields:
- `created_at`: Timestamp of when the entry was created
- `title`: Title of the case study
- `industry`: Industry or domain the case study belongs to
- `year`: Year the case study was published or the work was done
- `source_url`: URL to the original source of the case study
- `company`: Company or organization that conducted the work
- `application_tags`: Tags related to the application or use case
- `tools_tags`: Tags for the specific tools or technologies used
- `extra_tags`: Additional relevant tags
- `techniques_tags`: Tags for the techniques or approaches applied
- `short_summary`: Brief summary of the case study
- `full_summary`: Detailed writeup of the case study
### Data Splits
The LLMOps database currently contains a single collection of >500 case studies, with no defined splits like train/validation/test sets.
## Dataset Creation
### Curation Rationale
The LLMOps Database was created to provide practical, implementation-focused insights into deploying LLMs in production environments. While theoretical discussions about LLMs are abundant, technical teams need concrete information to guide their deployment decisions. By curating and summarizing real-world case studies, the database aims to advance the shared understanding of open-source LLMOps solutions and best practices.
### Source Data
#### Initial Data Collection and Normalization
The case studies in the LLMOps Database have been hand-curated by following relevant discussions on Twitter and Discord channels. [Exa.ai](https://exa.ai) was also used to perform embeddings-based similarity search to find additional relevant sources. The criteria for inclusion focused on technical depth and practical applicability, with an emphasis on detailed implementations, architectural decisions, and real challenges faced by engineering teams.
The original source content was either the full text of a blog post or the transcript of a YouTube video. This content was then summarized using the Claude Sonnet 3.5 model from Anthropic. The metadata for each case study was also extracted using the [`instructor`](https://github.com/jxnl/instructor) library.
#### Who are the source language producers?
The original case study writeups were authored by the engineering teams or technical writers at the respective companies. The summarized versions in the LLMOps Database were generated by Anthropic's Claude Sonnet 3.6 model.
### Personal and Sensitive Information
The LLMOps Database does not contain any personal information, sensitive data, or identity characteristics.
## Considerations for Using the Data
### Social Impact of Dataset
The LLMOps Database is intended to have a positive impact by enabling technical teams to learn from real-world examples of LLM deployments. By providing practical insights and solutions, the dataset aims to make these powerful technologies more accessible and reliable for production use. However, as with any technology, there are potential risks such as the misuse of LLMs or unintended consequences from their deployment. Users of the dataset should carefully consider the ethical implications and potential impacts of their LLM applications.
### Discussion of Biases
One potential limitation of the dataset is that it would have been preferable to include the original source text or full video transcripts along with the summaries. However, this was not done to avoid potential copyright or ownership issues. If users wish to access the original source content, they will need to download it themselves.
### Other Known Limitations
No other known limitations.
## Additional Information
### Dataset Curators
The LLMOps Database was curated by the ZenML team. [ZenML](https://zenml.io)
maintains an open-source MLOps framework, and as part of their work, they engage
with many people doing MLOps and LLMOps. The team gathered these sources to
better understand the space and provide a useful resource for others.
### Licensing Information
The LLMOps Database is shared under the Apache License.
|
HungVu2003/opt-350m_beta_0.5_alpha_0.8_num-company_3_dataset_1_for_gen_1 | HungVu2003 | 2025-05-04T17:01:05Z | 0 | 0 | [
"region:us"
] | [] | 2025-05-04T17:01:03Z | null | ---
dataset_info:
features:
- name: question
dtype: string
splits:
- name: train
num_bytes: 1903153
num_examples: 12498
download_size: 1090827
dataset_size: 1903153
configs:
- config_name: default
data_files:
- split: train
path: data/train-*
---
|
5CD-AI/Viet-qvq-r1 | 5CD-AI | 2025-05-04T16:33:42Z | 140 | 3 | [
"task_categories:question-answering",
"language:vi",
"language:en",
"size_categories:10K<n<100K",
"format:parquet",
"modality:image",
"modality:text",
"library:datasets",
"library:dask",
"library:mlcroissant",
"library:polars",
"region:us"
] | [
"question-answering"
] | 2025-04-25T03:43:39Z | null | ---
dataset_info:
features:
- name: uid
dtype: int64
- name: subset
dtype: string
- name: id
dtype: string
- name: image
dtype: image
- name: conversations
sequence:
- name: role
dtype: string
- name: content
dtype: string
- name: source
dtype: string
- name: vi_conversations
sequence:
- name: role
dtype: string
- name: content
dtype: string
splits:
- name: train
num_bytes: 7252068072.656
num_examples: 78082
download_size: 6093271009
dataset_size: 7252068072.656
configs:
- config_name: default
data_files:
- split: train
path: data/train-*
task_categories:
- question-answering
language:
- vi
- en
pretty_name: viet-qvq-r1
---
### Description
This dataset is a Vietnamese translation of the [ahmedheakl/qvq-r1](https://huggingface.co/datasets/ahmedheakl/qvq-r1), intended for training and evaluating multimodal Vision–Language Models (VLMs) on **visual reasoning** tasks involving document-style images such as receipts, forms, invoices.,
Each example includes:
- An input image containing text (typically scanned documents),
- A conversation simulating a user question and an assistant’s step-by-step reasoning leading to the answer,
- A Vietnamese version of the full conversation.
The Vietnamese translations were automatically generated using the Grok model, with careful preservation of both the question intent and the reasoning process.
This subset contains approximately **78,000 examples**.
---
### Dataset Structure
Each record contains the following main fields:
| Field | Type | Description |
|--------------------|----------|-----------------------------------------------------------------------------|
| `image` | `image` | The input image, typically a document or receipt. |
| `conversations` | `string` | A dialogue between user and assistant, with detailed step-by-step reasoning.|
| `vi_conversations` | `string` | Vietnamese translation of the `conversations` field. | |
mteb/medrxiv-clustering-p2p | mteb | 2025-05-04T16:28:25Z | 637 | 2 | [
"task_categories:text-classification",
"annotations_creators:derived",
"multilinguality:monolingual",
"source_datasets:mteb/medrxiv-clustering-p2p",
"language:eng",
"license:other",
"size_categories:10K<n<100K",
"format:json",
"modality:text",
"library:datasets",
"library:pandas",
"library:mlcroissant",
"library:polars",
"arxiv:2502.13595",
"arxiv:2210.07316",
"region:us",
"mteb",
"text"
] | [
"text-classification"
] | 2022-05-11T06:56:44Z | null | ---
annotations_creators:
- derived
language:
- eng
license: other
multilinguality: monolingual
source_datasets:
- mteb/medrxiv-clustering-p2p
task_categories:
- text-classification
task_ids: []
tags:
- mteb
- text
---
<!-- adapted from https://github.com/huggingface/huggingface_hub/blob/v0.30.2/src/huggingface_hub/templates/datasetcard_template.md -->
<div align="center" style="padding: 40px 20px; background-color: white; border-radius: 12px; box-shadow: 0 2px 10px rgba(0, 0, 0, 0.05); max-width: 600px; margin: 0 auto;">
<h1 style="font-size: 3.5rem; color: #1a1a1a; margin: 0 0 20px 0; letter-spacing: 2px; font-weight: 700;">MedrxivClusteringP2P.v2</h1>
<div style="font-size: 1.5rem; color: #4a4a4a; margin-bottom: 5px; font-weight: 300;">An <a href="https://github.com/embeddings-benchmark/mteb" style="color: #2c5282; font-weight: 600; text-decoration: none;" onmouseover="this.style.textDecoration='underline'" onmouseout="this.style.textDecoration='none'">MTEB</a> dataset</div>
<div style="font-size: 0.9rem; color: #2c5282; margin-top: 10px;">Massive Text Embedding Benchmark</div>
</div>
Clustering of titles+abstract from medrxiv across 51 categories.
| | |
|---------------|---------------------------------------------|
| Task category | t2c |
| Domains | Academic, Medical, Written |
| Reference | https://api.medrxiv.org/ |
## How to evaluate on this task
You can evaluate an embedding model on this dataset using the following code:
```python
import mteb
task = mteb.get_tasks(["MedrxivClusteringP2P.v2"])
evaluator = mteb.MTEB(task)
model = mteb.get_model(YOUR_MODEL)
evaluator.run(model)
```
<!-- Datasets want link to arxiv in readme to autolink dataset with paper -->
To learn more about how to run models on `mteb` task check out the [GitHub repitory](https://github.com/embeddings-benchmark/mteb).
## Citation
If you use this dataset, please cite the dataset as well as [mteb](https://github.com/embeddings-benchmark/mteb), as this dataset likely includes additional processing as a part of the [MMTEB Contribution](https://github.com/embeddings-benchmark/mteb/tree/main/docs/mmteb).
```bibtex
@article{enevoldsen2025mmtebmassivemultilingualtext,
title={MMTEB: Massive Multilingual Text Embedding Benchmark},
author={Kenneth Enevoldsen and Isaac Chung and Imene Kerboua and Márton Kardos and Ashwin Mathur and David Stap and Jay Gala and Wissam Siblini and Dominik Krzemiński and Genta Indra Winata and Saba Sturua and Saiteja Utpala and Mathieu Ciancone and Marion Schaeffer and Gabriel Sequeira and Diganta Misra and Shreeya Dhakal and Jonathan Rystrøm and Roman Solomatin and Ömer Çağatan and Akash Kundu and Martin Bernstorff and Shitao Xiao and Akshita Sukhlecha and Bhavish Pahwa and Rafał Poświata and Kranthi Kiran GV and Shawon Ashraf and Daniel Auras and Björn Plüster and Jan Philipp Harries and Loïc Magne and Isabelle Mohr and Mariya Hendriksen and Dawei Zhu and Hippolyte Gisserot-Boukhlef and Tom Aarsen and Jan Kostkan and Konrad Wojtasik and Taemin Lee and Marek Šuppa and Crystina Zhang and Roberta Rocca and Mohammed Hamdy and Andrianos Michail and John Yang and Manuel Faysse and Aleksei Vatolin and Nandan Thakur and Manan Dey and Dipam Vasani and Pranjal Chitale and Simone Tedeschi and Nguyen Tai and Artem Snegirev and Michael Günther and Mengzhou Xia and Weijia Shi and Xing Han Lù and Jordan Clive and Gayatri Krishnakumar and Anna Maksimova and Silvan Wehrli and Maria Tikhonova and Henil Panchal and Aleksandr Abramov and Malte Ostendorff and Zheng Liu and Simon Clematide and Lester James Miranda and Alena Fenogenova and Guangyu Song and Ruqiya Bin Safi and Wen-Ding Li and Alessia Borghini and Federico Cassano and Hongjin Su and Jimmy Lin and Howard Yen and Lasse Hansen and Sara Hooker and Chenghao Xiao and Vaibhav Adlakha and Orion Weller and Siva Reddy and Niklas Muennighoff},
publisher = {arXiv},
journal={arXiv preprint arXiv:2502.13595},
year={2025},
url={https://arxiv.org/abs/2502.13595},
doi = {10.48550/arXiv.2502.13595},
}
@article{muennighoff2022mteb,
author = {Muennighoff, Niklas and Tazi, Nouamane and Magne, Lo{\"\i}c and Reimers, Nils},
title = {MTEB: Massive Text Embedding Benchmark},
publisher = {arXiv},
journal={arXiv preprint arXiv:2210.07316},
year = {2022}
url = {https://arxiv.org/abs/2210.07316},
doi = {10.48550/ARXIV.2210.07316},
}
```
# Dataset Statistics
<details>
<summary> Dataset Statistics</summary>
The following code contains the descriptive statistics from the task. These can also be obtained using:
```python
import mteb
task = mteb.get_task("MedrxivClusteringP2P.v2")
desc_stats = task.metadata.descriptive_stats
```
```json
{
"test": {
"num_samples": 37500,
"number_of_characters": 74294927,
"min_text_length": 148,
"average_text_length": 1981.1980533333333,
"max_text_length": 38759,
"min_labels_per_text": 6,
"average_labels_per_text": 1.0,
"max_labels_per_text": 8830,
"unique_labels": 51,
"labels": {
"epidemiology": {
"count": 6656
},
"public and global health": {
"count": 3595
},
"oncology": {
"count": 845
},
"allergy and immunology": {
"count": 464
},
"orthopedics": {
"count": 104
},
"health informatics": {
"count": 1107
},
"occupational and environmental health": {
"count": 415
},
"infectious diseases": {
"count": 8830
},
"genetic and genomic medicine": {
"count": 1918
},
"health policy": {
"count": 527
},
"gastroenterology": {
"count": 343
},
"radiology and imaging": {
"count": 541
},
"pain medicine": {
"count": 121
},
"neurology": {
"count": 1773
},
"primary care research": {
"count": 232
},
"rheumatology": {
"count": 189
},
"endocrinology": {
"count": 419
},
"hematology": {
"count": 202
},
"addiction medicine": {
"count": 178
},
"pediatrics": {
"count": 589
},
"cardiovascular medicine": {
"count": 855
},
"obstetrics and gynecology": {
"count": 373
},
"health systems and quality improvement": {
"count": 491
},
"nephrology": {
"count": 241
},
"respiratory medicine": {
"count": 482
},
"geriatric medicine": {
"count": 169
},
"dentistry and oral medicine": {
"count": 159
},
"psychiatry and clinical psychology": {
"count": 1781
},
"nutrition": {
"count": 240
},
"intensive care and critical care medicine": {
"count": 368
},
"rehabilitation medicine and physical therapy": {
"count": 322
},
"otolaryngology": {
"count": 166
},
"nursing": {
"count": 93
},
"transplantation": {
"count": 118
},
"health economics": {
"count": 327
},
"sports medicine": {
"count": 180
},
"hiv aids": {
"count": 363
},
"dermatology": {
"count": 98
},
"pathology": {
"count": 223
},
"emergency medicine": {
"count": 191
},
"pharmacology and therapeutics": {
"count": 221
},
"ophthalmology": {
"count": 220
},
"medical ethics": {
"count": 46
},
"palliative medicine": {
"count": 45
},
"sexual and reproductive health": {
"count": 156
},
"medical education": {
"count": 203
},
"surgery": {
"count": 162
},
"urology": {
"count": 65
},
"anesthesia": {
"count": 72
},
"toxicology": {
"count": 16
},
"forensic medicine": {
"count": 6
}
}
}
}
```
</details>
---
*This dataset card was automatically generated using [MTEB](https://github.com/embeddings-benchmark/mteb)* |
mteb/sickr-sts | mteb | 2025-05-04T16:26:42Z | 15,274 | 4 | [
"task_categories:sentence-similarity",
"task_ids:semantic-similarity-scoring",
"task_ids:natural-language-inference",
"annotations_creators:human-annotated",
"multilinguality:monolingual",
"language:eng",
"license:cc-by-nc-sa-3.0",
"size_categories:1K<n<10K",
"format:json",
"modality:text",
"library:datasets",
"library:pandas",
"library:mlcroissant",
"library:polars",
"arxiv:2502.13595",
"arxiv:2210.07316",
"region:us",
"mteb",
"text"
] | [
"sentence-similarity"
] | 2022-04-19T14:28:03Z | null | ---
annotations_creators:
- human-annotated
language:
- eng
license: cc-by-nc-sa-3.0
multilinguality: monolingual
task_categories:
- sentence-similarity
task_ids:
- semantic-similarity-scoring
- natural-language-inference
tags:
- mteb
- text
---
<!-- adapted from https://github.com/huggingface/huggingface_hub/blob/v0.30.2/src/huggingface_hub/templates/datasetcard_template.md -->
<div align="center" style="padding: 40px 20px; background-color: white; border-radius: 12px; box-shadow: 0 2px 10px rgba(0, 0, 0, 0.05); max-width: 600px; margin: 0 auto;">
<h1 style="font-size: 3.5rem; color: #1a1a1a; margin: 0 0 20px 0; letter-spacing: 2px; font-weight: 700;">SICK-R</h1>
<div style="font-size: 1.5rem; color: #4a4a4a; margin-bottom: 5px; font-weight: 300;">An <a href="https://github.com/embeddings-benchmark/mteb" style="color: #2c5282; font-weight: 600; text-decoration: none;" onmouseover="this.style.textDecoration='underline'" onmouseout="this.style.textDecoration='none'">MTEB</a> dataset</div>
<div style="font-size: 0.9rem; color: #2c5282; margin-top: 10px;">Massive Text Embedding Benchmark</div>
</div>
Semantic Textual Similarity SICK-R dataset
| | |
|---------------|---------------------------------------------|
| Task category | t2t |
| Domains | Web, Written |
| Reference | https://aclanthology.org/L14-1314/ |
## How to evaluate on this task
You can evaluate an embedding model on this dataset using the following code:
```python
import mteb
task = mteb.get_tasks(["SICK-R"])
evaluator = mteb.MTEB(task)
model = mteb.get_model(YOUR_MODEL)
evaluator.run(model)
```
<!-- Datasets want link to arxiv in readme to autolink dataset with paper -->
To learn more about how to run models on `mteb` task check out the [GitHub repitory](https://github.com/embeddings-benchmark/mteb).
## Citation
If you use this dataset, please cite the dataset as well as [mteb](https://github.com/embeddings-benchmark/mteb), as this dataset likely includes additional processing as a part of the [MMTEB Contribution](https://github.com/embeddings-benchmark/mteb/tree/main/docs/mmteb).
```bibtex
@inproceedings{marelli-etal-2014-sick,
abstract = {Shared and internationally recognized benchmarks are fundamental for the development of any computational system. We aim to help the research community working on compositional distributional semantic models (CDSMs) by providing SICK (Sentences Involving Compositional Knowldedge), a large size English benchmark tailored for them. SICK consists of about 10,000 English sentence pairs that include many examples of the lexical, syntactic and semantic phenomena that CDSMs are expected to account for, but do not require dealing with other aspects of existing sentential data sets (idiomatic multiword expressions, named entities, telegraphic language) that are not within the scope of CDSMs. By means of crowdsourcing techniques, each pair was annotated for two crucial semantic tasks: relatedness in meaning (with a 5-point rating scale as gold score) and entailment relation between the two elements (with three possible gold labels: entailment, contradiction, and neutral). The SICK data set was used in SemEval-2014 Task 1, and it freely available for research purposes.},
address = {Reykjavik, Iceland},
author = {Marelli, Marco and
Menini, Stefano and
Baroni, Marco and
Bentivogli, Luisa and
Bernardi, Raffaella and
Zamparelli, Roberto},
booktitle = {Proceedings of the Ninth International Conference on Language Resources and Evaluation ({LREC}'14)},
editor = {Calzolari, Nicoletta and
Choukri, Khalid and
Declerck, Thierry and
Loftsson, Hrafn and
Maegaard, Bente and
Mariani, Joseph and
Moreno, Asuncion and
Odijk, Jan and
Piperidis, Stelios},
month = may,
pages = {216--223},
publisher = {European Language Resources Association (ELRA)},
title = {A {SICK} cure for the evaluation of compositional distributional semantic models},
url = {http://www.lrec-conf.org/proceedings/lrec2014/pdf/363_Paper.pdf},
year = {2014},
}
@article{enevoldsen2025mmtebmassivemultilingualtext,
title={MMTEB: Massive Multilingual Text Embedding Benchmark},
author={Kenneth Enevoldsen and Isaac Chung and Imene Kerboua and Márton Kardos and Ashwin Mathur and David Stap and Jay Gala and Wissam Siblini and Dominik Krzemiński and Genta Indra Winata and Saba Sturua and Saiteja Utpala and Mathieu Ciancone and Marion Schaeffer and Gabriel Sequeira and Diganta Misra and Shreeya Dhakal and Jonathan Rystrøm and Roman Solomatin and Ömer Çağatan and Akash Kundu and Martin Bernstorff and Shitao Xiao and Akshita Sukhlecha and Bhavish Pahwa and Rafał Poświata and Kranthi Kiran GV and Shawon Ashraf and Daniel Auras and Björn Plüster and Jan Philipp Harries and Loïc Magne and Isabelle Mohr and Mariya Hendriksen and Dawei Zhu and Hippolyte Gisserot-Boukhlef and Tom Aarsen and Jan Kostkan and Konrad Wojtasik and Taemin Lee and Marek Šuppa and Crystina Zhang and Roberta Rocca and Mohammed Hamdy and Andrianos Michail and John Yang and Manuel Faysse and Aleksei Vatolin and Nandan Thakur and Manan Dey and Dipam Vasani and Pranjal Chitale and Simone Tedeschi and Nguyen Tai and Artem Snegirev and Michael Günther and Mengzhou Xia and Weijia Shi and Xing Han Lù and Jordan Clive and Gayatri Krishnakumar and Anna Maksimova and Silvan Wehrli and Maria Tikhonova and Henil Panchal and Aleksandr Abramov and Malte Ostendorff and Zheng Liu and Simon Clematide and Lester James Miranda and Alena Fenogenova and Guangyu Song and Ruqiya Bin Safi and Wen-Ding Li and Alessia Borghini and Federico Cassano and Hongjin Su and Jimmy Lin and Howard Yen and Lasse Hansen and Sara Hooker and Chenghao Xiao and Vaibhav Adlakha and Orion Weller and Siva Reddy and Niklas Muennighoff},
publisher = {arXiv},
journal={arXiv preprint arXiv:2502.13595},
year={2025},
url={https://arxiv.org/abs/2502.13595},
doi = {10.48550/arXiv.2502.13595},
}
@article{muennighoff2022mteb,
author = {Muennighoff, Niklas and Tazi, Nouamane and Magne, Lo{\"\i}c and Reimers, Nils},
title = {MTEB: Massive Text Embedding Benchmark},
publisher = {arXiv},
journal={arXiv preprint arXiv:2210.07316},
year = {2022}
url = {https://arxiv.org/abs/2210.07316},
doi = {10.48550/ARXIV.2210.07316},
}
```
# Dataset Statistics
<details>
<summary> Dataset Statistics</summary>
The following code contains the descriptive statistics from the task. These can also be obtained using:
```python
import mteb
task = mteb.get_task("SICK-R")
desc_stats = task.metadata.descriptive_stats
```
```json
{
"test": {
"num_samples": 9927,
"number_of_characters": 915617,
"unique_pairs": 9842,
"min_sentence1_length": 15,
"average_sentence1_len": 46.602196031026494,
"max_sentence1_length": 151,
"unique_sentence1": 5014,
"min_sentence2_length": 14,
"average_sentence2_len": 45.63281958295558,
"max_sentence2_length": 151,
"unique_sentence2": 4946,
"min_score": 1.0,
"avg_score": 3.5291492898156607,
"max_score": 5.0
}
}
```
</details>
---
*This dataset card was automatically generated using [MTEB](https://github.com/embeddings-benchmark/mteb)* |
yashparalkar0/codePM | yashparalkar0 | 2025-05-04T16:20:43Z | 0 | 0 | [
"task_categories:text2text-generation",
"region:us",
"code"
] | [
"text2text-generation"
] | 2025-05-04T16:17:32Z | null | ---
task_categories:
- text2text-generation
tags:
- code
pretty_name: f
--- |
harpreetmann/go_emotions_max_500_string_chat | harpreetmann | 2025-05-04T16:14:55Z | 0 | 0 | [
"region:us"
] | [] | 2025-05-04T16:14:49Z | null | ---
dataset_info:
features:
- name: input
dtype: string
- name: output
dtype: string
- name: id
dtype: string
- name: messages
list:
- name: content
dtype: string
- name: role
dtype: string
splits:
- name: train
num_bytes: 27852466
num_examples: 43409
- name: test
num_bytes: 3488513
num_examples: 5427
- name: validation
num_bytes: 3487936
num_examples: 5426
- name: discarded
num_bytes: 3483
num_examples: 1
download_size: 8204171
dataset_size: 34832398
configs:
- config_name: default
data_files:
- split: train
path: data/train-*
- split: test
path: data/test-*
- split: validation
path: data/validation-*
- split: discarded
path: data/discarded-*
---
|
mteb/WikipediaRerankingMultilingual | mteb | 2025-05-04T16:12:04Z | 617 | 0 | [
"task_categories:text-ranking",
"annotations_creators:LM-generated and reviewed",
"multilinguality:multilingual",
"language:ben",
"language:bul",
"language:ces",
"language:dan",
"language:deu",
"language:eng",
"language:fas",
"language:fin",
"language:hin",
"language:ita",
"language:nld",
"language:nor",
"language:por",
"language:ron",
"language:srp",
"language:swe",
"license:cc-by-sa-3.0",
"size_categories:100K<n<1M",
"format:parquet",
"modality:text",
"library:datasets",
"library:pandas",
"library:mlcroissant",
"library:polars",
"arxiv:2502.13595",
"arxiv:2210.07316",
"region:us",
"mteb",
"text"
] | [
"text-ranking"
] | 2025-02-19T09:28:42Z | null | ---
annotations_creators:
- LM-generated and reviewed
language:
- ben
- bul
- ces
- dan
- deu
- eng
- fas
- fin
- hin
- ita
- nld
- nor
- por
- ron
- srp
- swe
license: cc-by-sa-3.0
multilinguality: multilingual
task_categories:
- text-ranking
task_ids: []
dataset_info:
- config_name: bg-corpus
features:
- name: _id
dtype: string
- name: text
dtype: string
- name: title
dtype: string
splits:
- name: test
num_bytes: 9604308
num_examples: 13500
download_size: 4593991
dataset_size: 9604308
- config_name: bg-qrels
features:
- name: query-id
dtype: string
- name: corpus-id
dtype: string
- name: score
dtype: int64
splits:
- name: test
num_bytes: 778020
num_examples: 13500
download_size: 101652
dataset_size: 778020
- config_name: bg-queries
features:
- name: _id
dtype: string
- name: text
dtype: string
splits:
- name: test
num_bytes: 197625
num_examples: 1500
download_size: 96857
dataset_size: 197625
- config_name: bg-top_ranked
features:
- name: query-id
dtype: string
- name: corpus-ids
sequence: string
splits:
- name: test
num_bytes: 468900
num_examples: 1500
download_size: 97689
dataset_size: 468900
- config_name: bn-corpus
features:
- name: _id
dtype: string
- name: text
dtype: string
- name: title
dtype: string
splits:
- name: test
num_bytes: 14497846
num_examples: 13500
download_size: 5486517
dataset_size: 14497846
- config_name: bn-qrels
features:
- name: query-id
dtype: string
- name: corpus-id
dtype: string
- name: score
dtype: int64
splits:
- name: test
num_bytes: 778020
num_examples: 13500
download_size: 101652
dataset_size: 778020
- config_name: bn-queries
features:
- name: _id
dtype: string
- name: text
dtype: string
splits:
- name: test
num_bytes: 222824
num_examples: 1500
download_size: 95032
dataset_size: 222824
- config_name: bn-top_ranked
features:
- name: query-id
dtype: string
- name: corpus-ids
sequence: string
splits:
- name: test
num_bytes: 468900
num_examples: 1500
download_size: 97689
dataset_size: 468900
- config_name: cs-corpus
features:
- name: _id
dtype: string
- name: text
dtype: string
- name: title
dtype: string
splits:
- name: test
num_bytes: 6098076
num_examples: 13500
download_size: 3914545
dataset_size: 6098076
- config_name: cs-qrels
features:
- name: query-id
dtype: string
- name: corpus-id
dtype: string
- name: score
dtype: int64
splits:
- name: test
num_bytes: 778020
num_examples: 13500
download_size: 101652
dataset_size: 778020
- config_name: cs-queries
features:
- name: _id
dtype: string
- name: text
dtype: string
splits:
- name: test
num_bytes: 124465
num_examples: 1500
download_size: 82189
dataset_size: 124465
- config_name: cs-top_ranked
features:
- name: query-id
dtype: string
- name: corpus-ids
sequence: string
splits:
- name: test
num_bytes: 468900
num_examples: 1500
download_size: 97689
dataset_size: 468900
- config_name: da-corpus
features:
- name: _id
dtype: string
- name: text
dtype: string
- name: title
dtype: string
splits:
- name: test
num_bytes: 5309400
num_examples: 13500
download_size: 3172960
dataset_size: 5309400
- config_name: da-qrels
features:
- name: query-id
dtype: string
- name: corpus-id
dtype: string
- name: score
dtype: int64
splits:
- name: test
num_bytes: 778020
num_examples: 13500
download_size: 101652
dataset_size: 778020
- config_name: da-queries
features:
- name: _id
dtype: string
- name: text
dtype: string
splits:
- name: test
num_bytes: 118643
num_examples: 1500
download_size: 73789
dataset_size: 118643
- config_name: da-top_ranked
features:
- name: query-id
dtype: string
- name: corpus-ids
sequence: string
splits:
- name: test
num_bytes: 468900
num_examples: 1500
download_size: 97689
dataset_size: 468900
- config_name: de-corpus
features:
- name: _id
dtype: string
- name: text
dtype: string
- name: title
dtype: string
splits:
- name: test
num_bytes: 6019751
num_examples: 13500
download_size: 3594010
dataset_size: 6019751
- config_name: de-qrels
features:
- name: query-id
dtype: string
- name: corpus-id
dtype: string
- name: score
dtype: int64
splits:
- name: test
num_bytes: 778020
num_examples: 13500
download_size: 101652
dataset_size: 778020
- config_name: de-queries
features:
- name: _id
dtype: string
- name: text
dtype: string
splits:
- name: test
num_bytes: 138167
num_examples: 1500
download_size: 88032
dataset_size: 138167
- config_name: de-top_ranked
features:
- name: query-id
dtype: string
- name: corpus-ids
sequence: string
splits:
- name: test
num_bytes: 468900
num_examples: 1500
download_size: 97689
dataset_size: 468900
- config_name: en-corpus
features:
- name: _id
dtype: string
- name: text
dtype: string
- name: title
dtype: string
splits:
- name: test
num_bytes: 6671388
num_examples: 13500
download_size: 3961948
dataset_size: 6671388
- config_name: en-qrels
features:
- name: query-id
dtype: string
- name: corpus-id
dtype: string
- name: score
dtype: int64
splits:
- name: test
num_bytes: 778020
num_examples: 13500
download_size: 101652
dataset_size: 778020
- config_name: en-queries
features:
- name: _id
dtype: string
- name: text
dtype: string
splits:
- name: test
num_bytes: 134536
num_examples: 1500
download_size: 83004
dataset_size: 134536
- config_name: en-top_ranked
features:
- name: query-id
dtype: string
- name: corpus-ids
sequence: string
splits:
- name: test
num_bytes: 468900
num_examples: 1500
download_size: 97689
dataset_size: 468900
- config_name: fa-corpus
features:
- name: _id
dtype: string
- name: text
dtype: string
- name: title
dtype: string
splits:
- name: test
num_bytes: 8973566
num_examples: 13500
download_size: 4213163
dataset_size: 8973566
- config_name: fa-qrels
features:
- name: query-id
dtype: string
- name: corpus-id
dtype: string
- name: score
dtype: int64
splits:
- name: test
num_bytes: 778020
num_examples: 13500
download_size: 101652
dataset_size: 778020
- config_name: fa-queries
features:
- name: _id
dtype: string
- name: text
dtype: string
splits:
- name: test
num_bytes: 167018
num_examples: 1500
download_size: 85233
dataset_size: 167018
- config_name: fa-top_ranked
features:
- name: query-id
dtype: string
- name: corpus-ids
sequence: string
splits:
- name: test
num_bytes: 468900
num_examples: 1500
download_size: 97689
dataset_size: 468900
- config_name: fi-corpus
features:
- name: _id
dtype: string
- name: text
dtype: string
- name: title
dtype: string
splits:
- name: test
num_bytes: 5866641
num_examples: 13500
download_size: 3485556
dataset_size: 5866641
- config_name: fi-qrels
features:
- name: query-id
dtype: string
- name: corpus-id
dtype: string
- name: score
dtype: int64
splits:
- name: test
num_bytes: 778020
num_examples: 13500
download_size: 101652
dataset_size: 778020
- config_name: fi-queries
features:
- name: _id
dtype: string
- name: text
dtype: string
splits:
- name: test
num_bytes: 117859
num_examples: 1500
download_size: 74406
dataset_size: 117859
- config_name: fi-top_ranked
features:
- name: query-id
dtype: string
- name: corpus-ids
sequence: string
splits:
- name: test
num_bytes: 468900
num_examples: 1500
download_size: 97689
dataset_size: 468900
- config_name: hi-corpus
features:
- name: _id
dtype: string
- name: text
dtype: string
- name: title
dtype: string
splits:
- name: test
num_bytes: 14696552
num_examples: 13500
download_size: 5583513
dataset_size: 14696552
- config_name: hi-qrels
features:
- name: query-id
dtype: string
- name: corpus-id
dtype: string
- name: score
dtype: int64
splits:
- name: test
num_bytes: 778020
num_examples: 13500
download_size: 101652
dataset_size: 778020
- config_name: hi-queries
features:
- name: _id
dtype: string
- name: text
dtype: string
splits:
- name: test
num_bytes: 229970
num_examples: 1500
download_size: 98256
dataset_size: 229970
- config_name: hi-top_ranked
features:
- name: query-id
dtype: string
- name: corpus-ids
sequence: string
splits:
- name: test
num_bytes: 468900
num_examples: 1500
download_size: 97689
dataset_size: 468900
- config_name: it-corpus
features:
- name: _id
dtype: string
- name: text
dtype: string
- name: title
dtype: string
splits:
- name: test
num_bytes: 5899305
num_examples: 13500
download_size: 3566485
dataset_size: 5899305
- config_name: it-qrels
features:
- name: query-id
dtype: string
- name: corpus-id
dtype: string
- name: score
dtype: int64
splits:
- name: test
num_bytes: 778020
num_examples: 13500
download_size: 101652
dataset_size: 778020
- config_name: it-queries
features:
- name: _id
dtype: string
- name: text
dtype: string
splits:
- name: test
num_bytes: 137965
num_examples: 1500
download_size: 84180
dataset_size: 137965
- config_name: it-top_ranked
features:
- name: query-id
dtype: string
- name: corpus-ids
sequence: string
splits:
- name: test
num_bytes: 468900
num_examples: 1500
download_size: 97689
dataset_size: 468900
- config_name: nl-corpus
features:
- name: _id
dtype: string
- name: text
dtype: string
- name: title
dtype: string
splits:
- name: test
num_bytes: 5628451
num_examples: 13500
download_size: 3254369
dataset_size: 5628451
- config_name: nl-qrels
features:
- name: query-id
dtype: string
- name: corpus-id
dtype: string
- name: score
dtype: int64
splits:
- name: test
num_bytes: 778020
num_examples: 13500
download_size: 101652
dataset_size: 778020
- config_name: nl-queries
features:
- name: _id
dtype: string
- name: text
dtype: string
splits:
- name: test
num_bytes: 130098
num_examples: 1500
download_size: 79310
dataset_size: 130098
- config_name: nl-top_ranked
features:
- name: query-id
dtype: string
- name: corpus-ids
sequence: string
splits:
- name: test
num_bytes: 468900
num_examples: 1500
download_size: 97689
dataset_size: 468900
- config_name: no-corpus
features:
- name: _id
dtype: string
- name: text
dtype: string
- name: title
dtype: string
splits:
- name: test
num_bytes: 5603404
num_examples: 13500
download_size: 3361788
dataset_size: 5603404
- config_name: no-qrels
features:
- name: query-id
dtype: string
- name: corpus-id
dtype: string
- name: score
dtype: int64
splits:
- name: test
num_bytes: 778020
num_examples: 13500
download_size: 101652
dataset_size: 778020
- config_name: no-queries
features:
- name: _id
dtype: string
- name: text
dtype: string
splits:
- name: test
num_bytes: 116210
num_examples: 1500
download_size: 72568
dataset_size: 116210
- config_name: no-top_ranked
features:
- name: query-id
dtype: string
- name: corpus-ids
sequence: string
splits:
- name: test
num_bytes: 468900
num_examples: 1500
download_size: 97689
dataset_size: 468900
- config_name: pt-corpus
features:
- name: _id
dtype: string
- name: text
dtype: string
- name: title
dtype: string
splits:
- name: test
num_bytes: 6078548
num_examples: 13500
download_size: 3644877
dataset_size: 6078548
- config_name: pt-qrels
features:
- name: query-id
dtype: string
- name: corpus-id
dtype: string
- name: score
dtype: int64
splits:
- name: test
num_bytes: 778020
num_examples: 13500
download_size: 101652
dataset_size: 778020
- config_name: pt-queries
features:
- name: _id
dtype: string
- name: text
dtype: string
splits:
- name: test
num_bytes: 132902
num_examples: 1500
download_size: 82274
dataset_size: 132902
- config_name: pt-top_ranked
features:
- name: query-id
dtype: string
- name: corpus-ids
sequence: string
splits:
- name: test
num_bytes: 468900
num_examples: 1500
download_size: 97689
dataset_size: 468900
- config_name: ro-corpus
features:
- name: _id
dtype: string
- name: text
dtype: string
- name: title
dtype: string
splits:
- name: test
num_bytes: 5487340
num_examples: 13500
download_size: 3314140
dataset_size: 5487340
- config_name: ro-qrels
features:
- name: query-id
dtype: string
- name: corpus-id
dtype: string
- name: score
dtype: int64
splits:
- name: test
num_bytes: 778020
num_examples: 13500
download_size: 101652
dataset_size: 778020
- config_name: ro-queries
features:
- name: _id
dtype: string
- name: text
dtype: string
splits:
- name: test
num_bytes: 128853
num_examples: 1500
download_size: 80958
dataset_size: 128853
- config_name: ro-top_ranked
features:
- name: query-id
dtype: string
- name: corpus-ids
sequence: string
splits:
- name: test
num_bytes: 468900
num_examples: 1500
download_size: 97689
dataset_size: 468900
- config_name: sr-corpus
features:
- name: _id
dtype: string
- name: text
dtype: string
- name: title
dtype: string
splits:
- name: test
num_bytes: 9362172
num_examples: 13500
download_size: 4727113
dataset_size: 9362172
- config_name: sr-qrels
features:
- name: query-id
dtype: string
- name: corpus-id
dtype: string
- name: score
dtype: int64
splits:
- name: test
num_bytes: 778020
num_examples: 13500
download_size: 101652
dataset_size: 778020
- config_name: sr-queries
features:
- name: _id
dtype: string
- name: text
dtype: string
splits:
- name: test
num_bytes: 173594
num_examples: 1500
download_size: 95366
dataset_size: 173594
- config_name: sr-top_ranked
features:
- name: query-id
dtype: string
- name: corpus-ids
sequence: string
splits:
- name: test
num_bytes: 468900
num_examples: 1500
download_size: 97689
dataset_size: 468900
- config_name: sv-corpus
features:
- name: _id
dtype: string
- name: text
dtype: string
- name: title
dtype: string
splits:
- name: test
num_bytes: 5727305
num_examples: 13500
download_size: 3383922
dataset_size: 5727305
- config_name: sv-qrels
features:
- name: query-id
dtype: string
- name: corpus-id
dtype: string
- name: score
dtype: int64
splits:
- name: test
num_bytes: 778020
num_examples: 13500
download_size: 101652
dataset_size: 778020
- config_name: sv-queries
features:
- name: _id
dtype: string
- name: text
dtype: string
splits:
- name: test
num_bytes: 121800
num_examples: 1500
download_size: 77079
dataset_size: 121800
- config_name: sv-top_ranked
features:
- name: query-id
dtype: string
- name: corpus-ids
sequence: string
splits:
- name: test
num_bytes: 468900
num_examples: 1500
download_size: 97689
dataset_size: 468900
configs:
- config_name: bg-corpus
data_files:
- split: test
path: bg-corpus/test-*
- config_name: bg-qrels
data_files:
- split: test
path: bg-qrels/test-*
- config_name: bg-queries
data_files:
- split: test
path: bg-queries/test-*
- config_name: bg-top_ranked
data_files:
- split: test
path: bg-top_ranked/test-*
- config_name: bn-corpus
data_files:
- split: test
path: bn-corpus/test-*
- config_name: bn-qrels
data_files:
- split: test
path: bn-qrels/test-*
- config_name: bn-queries
data_files:
- split: test
path: bn-queries/test-*
- config_name: bn-top_ranked
data_files:
- split: test
path: bn-top_ranked/test-*
- config_name: cs-corpus
data_files:
- split: test
path: cs-corpus/test-*
- config_name: cs-qrels
data_files:
- split: test
path: cs-qrels/test-*
- config_name: cs-queries
data_files:
- split: test
path: cs-queries/test-*
- config_name: cs-top_ranked
data_files:
- split: test
path: cs-top_ranked/test-*
- config_name: da-corpus
data_files:
- split: test
path: da-corpus/test-*
- config_name: da-qrels
data_files:
- split: test
path: da-qrels/test-*
- config_name: da-queries
data_files:
- split: test
path: da-queries/test-*
- config_name: da-top_ranked
data_files:
- split: test
path: da-top_ranked/test-*
- config_name: de-corpus
data_files:
- split: test
path: de-corpus/test-*
- config_name: de-qrels
data_files:
- split: test
path: de-qrels/test-*
- config_name: de-queries
data_files:
- split: test
path: de-queries/test-*
- config_name: de-top_ranked
data_files:
- split: test
path: de-top_ranked/test-*
- config_name: en-corpus
data_files:
- split: test
path: en-corpus/test-*
- config_name: en-qrels
data_files:
- split: test
path: en-qrels/test-*
- config_name: en-queries
data_files:
- split: test
path: en-queries/test-*
- config_name: en-top_ranked
data_files:
- split: test
path: en-top_ranked/test-*
- config_name: fa-corpus
data_files:
- split: test
path: fa-corpus/test-*
- config_name: fa-qrels
data_files:
- split: test
path: fa-qrels/test-*
- config_name: fa-queries
data_files:
- split: test
path: fa-queries/test-*
- config_name: fa-top_ranked
data_files:
- split: test
path: fa-top_ranked/test-*
- config_name: fi-corpus
data_files:
- split: test
path: fi-corpus/test-*
- config_name: fi-qrels
data_files:
- split: test
path: fi-qrels/test-*
- config_name: fi-queries
data_files:
- split: test
path: fi-queries/test-*
- config_name: fi-top_ranked
data_files:
- split: test
path: fi-top_ranked/test-*
- config_name: hi-corpus
data_files:
- split: test
path: hi-corpus/test-*
- config_name: hi-qrels
data_files:
- split: test
path: hi-qrels/test-*
- config_name: hi-queries
data_files:
- split: test
path: hi-queries/test-*
- config_name: hi-top_ranked
data_files:
- split: test
path: hi-top_ranked/test-*
- config_name: it-corpus
data_files:
- split: test
path: it-corpus/test-*
- config_name: it-qrels
data_files:
- split: test
path: it-qrels/test-*
- config_name: it-queries
data_files:
- split: test
path: it-queries/test-*
- config_name: it-top_ranked
data_files:
- split: test
path: it-top_ranked/test-*
- config_name: nl-corpus
data_files:
- split: test
path: nl-corpus/test-*
- config_name: nl-qrels
data_files:
- split: test
path: nl-qrels/test-*
- config_name: nl-queries
data_files:
- split: test
path: nl-queries/test-*
- config_name: nl-top_ranked
data_files:
- split: test
path: nl-top_ranked/test-*
- config_name: no-corpus
data_files:
- split: test
path: no-corpus/test-*
- config_name: no-qrels
data_files:
- split: test
path: no-qrels/test-*
- config_name: no-queries
data_files:
- split: test
path: no-queries/test-*
- config_name: no-top_ranked
data_files:
- split: test
path: no-top_ranked/test-*
- config_name: pt-corpus
data_files:
- split: test
path: pt-corpus/test-*
- config_name: pt-qrels
data_files:
- split: test
path: pt-qrels/test-*
- config_name: pt-queries
data_files:
- split: test
path: pt-queries/test-*
- config_name: pt-top_ranked
data_files:
- split: test
path: pt-top_ranked/test-*
- config_name: ro-corpus
data_files:
- split: test
path: ro-corpus/test-*
- config_name: ro-qrels
data_files:
- split: test
path: ro-qrels/test-*
- config_name: ro-queries
data_files:
- split: test
path: ro-queries/test-*
- config_name: ro-top_ranked
data_files:
- split: test
path: ro-top_ranked/test-*
- config_name: sr-corpus
data_files:
- split: test
path: sr-corpus/test-*
- config_name: sr-qrels
data_files:
- split: test
path: sr-qrels/test-*
- config_name: sr-queries
data_files:
- split: test
path: sr-queries/test-*
- config_name: sr-top_ranked
data_files:
- split: test
path: sr-top_ranked/test-*
- config_name: sv-corpus
data_files:
- split: test
path: sv-corpus/test-*
- config_name: sv-qrels
data_files:
- split: test
path: sv-qrels/test-*
- config_name: sv-queries
data_files:
- split: test
path: sv-queries/test-*
- config_name: sv-top_ranked
data_files:
- split: test
path: sv-top_ranked/test-*
tags:
- mteb
- text
---
<!-- adapted from https://github.com/huggingface/huggingface_hub/blob/v0.30.2/src/huggingface_hub/templates/datasetcard_template.md -->
<div align="center" style="padding: 40px 20px; background-color: white; border-radius: 12px; box-shadow: 0 2px 10px rgba(0, 0, 0, 0.05); max-width: 600px; margin: 0 auto;">
<h1 style="font-size: 3.5rem; color: #1a1a1a; margin: 0 0 20px 0; letter-spacing: 2px; font-weight: 700;">WikipediaRerankingMultilingual</h1>
<div style="font-size: 1.5rem; color: #4a4a4a; margin-bottom: 5px; font-weight: 300;">An <a href="https://github.com/embeddings-benchmark/mteb" style="color: #2c5282; font-weight: 600; text-decoration: none;" onmouseover="this.style.textDecoration='underline'" onmouseout="this.style.textDecoration='none'">MTEB</a> dataset</div>
<div style="font-size: 0.9rem; color: #2c5282; margin-top: 10px;">Massive Text Embedding Benchmark</div>
</div>
The dataset is derived from Cohere's wikipedia-2023-11 dataset and contains synthetically generated queries.
| | |
|---------------|---------------------------------------------|
| Task category | t2t |
| Domains | Encyclopaedic, Written |
| Reference | https://huggingface.co/datasets/ellamind/wikipedia-2023-11-reranking-multilingual |
## How to evaluate on this task
You can evaluate an embedding model on this dataset using the following code:
```python
import mteb
task = mteb.get_tasks(["WikipediaRerankingMultilingual"])
evaluator = mteb.MTEB(task)
model = mteb.get_model(YOUR_MODEL)
evaluator.run(model)
```
<!-- Datasets want link to arxiv in readme to autolink dataset with paper -->
To learn more about how to run models on `mteb` task check out the [GitHub repitory](https://github.com/embeddings-benchmark/mteb).
## Citation
If you use this dataset, please cite the dataset as well as [mteb](https://github.com/embeddings-benchmark/mteb), as this dataset likely includes additional processing as a part of the [MMTEB Contribution](https://github.com/embeddings-benchmark/mteb/tree/main/docs/mmteb).
```bibtex
@online{wikidump,
author = {Wikimedia Foundation},
title = {Wikimedia Downloads},
url = {https://dumps.wikimedia.org},
}
@article{enevoldsen2025mmtebmassivemultilingualtext,
title={MMTEB: Massive Multilingual Text Embedding Benchmark},
author={Kenneth Enevoldsen and Isaac Chung and Imene Kerboua and Márton Kardos and Ashwin Mathur and David Stap and Jay Gala and Wissam Siblini and Dominik Krzemiński and Genta Indra Winata and Saba Sturua and Saiteja Utpala and Mathieu Ciancone and Marion Schaeffer and Gabriel Sequeira and Diganta Misra and Shreeya Dhakal and Jonathan Rystrøm and Roman Solomatin and Ömer Çağatan and Akash Kundu and Martin Bernstorff and Shitao Xiao and Akshita Sukhlecha and Bhavish Pahwa and Rafał Poświata and Kranthi Kiran GV and Shawon Ashraf and Daniel Auras and Björn Plüster and Jan Philipp Harries and Loïc Magne and Isabelle Mohr and Mariya Hendriksen and Dawei Zhu and Hippolyte Gisserot-Boukhlef and Tom Aarsen and Jan Kostkan and Konrad Wojtasik and Taemin Lee and Marek Šuppa and Crystina Zhang and Roberta Rocca and Mohammed Hamdy and Andrianos Michail and John Yang and Manuel Faysse and Aleksei Vatolin and Nandan Thakur and Manan Dey and Dipam Vasani and Pranjal Chitale and Simone Tedeschi and Nguyen Tai and Artem Snegirev and Michael Günther and Mengzhou Xia and Weijia Shi and Xing Han Lù and Jordan Clive and Gayatri Krishnakumar and Anna Maksimova and Silvan Wehrli and Maria Tikhonova and Henil Panchal and Aleksandr Abramov and Malte Ostendorff and Zheng Liu and Simon Clematide and Lester James Miranda and Alena Fenogenova and Guangyu Song and Ruqiya Bin Safi and Wen-Ding Li and Alessia Borghini and Federico Cassano and Hongjin Su and Jimmy Lin and Howard Yen and Lasse Hansen and Sara Hooker and Chenghao Xiao and Vaibhav Adlakha and Orion Weller and Siva Reddy and Niklas Muennighoff},
publisher = {arXiv},
journal={arXiv preprint arXiv:2502.13595},
year={2025},
url={https://arxiv.org/abs/2502.13595},
doi = {10.48550/arXiv.2502.13595},
}
@article{muennighoff2022mteb,
author = {Muennighoff, Niklas and Tazi, Nouamane and Magne, Lo{\"\i}c and Reimers, Nils},
title = {MTEB: Massive Text Embedding Benchmark},
publisher = {arXiv},
journal={arXiv preprint arXiv:2210.07316},
year = {2022}
url = {https://arxiv.org/abs/2210.07316},
doi = {10.48550/ARXIV.2210.07316},
}
```
# Dataset Statistics
<details>
<summary> Dataset Statistics</summary>
The following code contains the descriptive statistics from the task. These can also be obtained using:
```python
import mteb
task = mteb.get_task("WikipediaRerankingMultilingual")
desc_stats = task.metadata.descriptive_stats
```
```json
{
"test": {
"num_samples": 240000,
"number_of_characters": 83866932,
"num_documents": 216000,
"min_document_length": 100,
"average_document_length": 381.70714351851854,
"max_document_length": 9461,
"unique_documents": 216000,
"num_queries": 24000,
"min_query_length": 7,
"average_query_length": 59.091208333333334,
"max_query_length": 180,
"unique_queries": 24000,
"none_queries": 0,
"num_relevant_docs": 216000,
"min_relevant_docs_per_query": 9,
"average_relevant_docs_per_query": 1.0,
"max_relevant_docs_per_query": 9,
"unique_relevant_docs": 216000,
"num_instructions": null,
"min_instruction_length": null,
"average_instruction_length": null,
"max_instruction_length": null,
"unique_instructions": null,
"num_top_ranked": 24000,
"min_top_ranked_per_query": 9,
"average_top_ranked_per_query": 9.0,
"max_top_ranked_per_query": 9
}
}
```
</details>
---
*This dataset card was automatically generated using [MTEB](https://github.com/embeddings-benchmark/mteb)* |
mteb/VoyageMMarcoReranking | mteb | 2025-05-04T16:11:59Z | 9 | 0 | [
"task_categories:text-ranking",
"annotations_creators:derived",
"multilinguality:monolingual",
"language:jpn",
"license:cc-by-4.0",
"size_categories:100K<n<1M",
"format:parquet",
"modality:text",
"library:datasets",
"library:pandas",
"library:mlcroissant",
"library:polars",
"arxiv:2312.16144",
"arxiv:2502.13595",
"arxiv:2210.07316",
"region:us",
"mteb",
"text"
] | [
"text-ranking"
] | 2025-02-18T20:02:06Z | null | ---
annotations_creators:
- derived
language:
- jpn
license: cc-by-4.0
multilinguality: monolingual
task_categories:
- text-ranking
task_ids: []
dataset_info:
- config_name: corpus
features:
- name: _id
dtype: string
- name: text
dtype: string
- name: title
dtype: string
splits:
- name: test
num_bytes: 26582357
num_examples: 53375
download_size: 12669365
dataset_size: 26582357
- config_name: default
features:
- name: query-id
dtype: string
- name: corpus-id
dtype: string
- name: score
dtype: int64
splits:
- name: test
num_bytes: 3093432
num_examples: 53375
download_size: 359413
dataset_size: 3093432
- config_name: queries
features:
- name: _id
dtype: string
- name: text
dtype: string
splits:
- name: test
num_bytes: 139132
num_examples: 2048
download_size: 79174
dataset_size: 139132
- config_name: top_ranked
features:
- name: query-id
dtype: string
- name: corpus-ids
sequence: string
splits:
- name: test
num_bytes: 1778562
num_examples: 2048
download_size: 353814
dataset_size: 1778562
configs:
- config_name: corpus
data_files:
- split: test
path: corpus/test-*
- config_name: default
data_files:
- split: test
path: data/test-*
- config_name: queries
data_files:
- split: test
path: queries/test-*
- config_name: top_ranked
data_files:
- split: test
path: top_ranked/test-*
tags:
- mteb
- text
---
<!-- adapted from https://github.com/huggingface/huggingface_hub/blob/v0.30.2/src/huggingface_hub/templates/datasetcard_template.md -->
<div align="center" style="padding: 40px 20px; background-color: white; border-radius: 12px; box-shadow: 0 2px 10px rgba(0, 0, 0, 0.05); max-width: 600px; margin: 0 auto;">
<h1 style="font-size: 3.5rem; color: #1a1a1a; margin: 0 0 20px 0; letter-spacing: 2px; font-weight: 700;">VoyageMMarcoReranking</h1>
<div style="font-size: 1.5rem; color: #4a4a4a; margin-bottom: 5px; font-weight: 300;">An <a href="https://github.com/embeddings-benchmark/mteb" style="color: #2c5282; font-weight: 600; text-decoration: none;" onmouseover="this.style.textDecoration='underline'" onmouseout="this.style.textDecoration='none'">MTEB</a> dataset</div>
<div style="font-size: 0.9rem; color: #2c5282; margin-top: 10px;">Massive Text Embedding Benchmark</div>
</div>
a hard-negative augmented version of the Japanese MMARCO dataset as used in Voyage AI Evaluation Suite
| | |
|---------------|---------------------------------------------|
| Task category | t2t |
| Domains | Academic, Non-fiction, Written |
| Reference | https://arxiv.org/abs/2312.16144 |
## How to evaluate on this task
You can evaluate an embedding model on this dataset using the following code:
```python
import mteb
task = mteb.get_tasks(["VoyageMMarcoReranking"])
evaluator = mteb.MTEB(task)
model = mteb.get_model(YOUR_MODEL)
evaluator.run(model)
```
<!-- Datasets want link to arxiv in readme to autolink dataset with paper -->
To learn more about how to run models on `mteb` task check out the [GitHub repitory](https://github.com/embeddings-benchmark/mteb).
## Citation
If you use this dataset, please cite the dataset as well as [mteb](https://github.com/embeddings-benchmark/mteb), as this dataset likely includes additional processing as a part of the [MMTEB Contribution](https://github.com/embeddings-benchmark/mteb/tree/main/docs/mmteb).
```bibtex
@misc{clavié2023jacolbert,
archiveprefix = {arXiv},
author = {Benjamin Clavié},
eprint = {2312.16144},
title = {JaColBERT and Hard Negatives, Towards Better Japanese-First Embeddings for Retrieval: Early Technical Report},
year = {2023},
}
@article{enevoldsen2025mmtebmassivemultilingualtext,
title={MMTEB: Massive Multilingual Text Embedding Benchmark},
author={Kenneth Enevoldsen and Isaac Chung and Imene Kerboua and Márton Kardos and Ashwin Mathur and David Stap and Jay Gala and Wissam Siblini and Dominik Krzemiński and Genta Indra Winata and Saba Sturua and Saiteja Utpala and Mathieu Ciancone and Marion Schaeffer and Gabriel Sequeira and Diganta Misra and Shreeya Dhakal and Jonathan Rystrøm and Roman Solomatin and Ömer Çağatan and Akash Kundu and Martin Bernstorff and Shitao Xiao and Akshita Sukhlecha and Bhavish Pahwa and Rafał Poświata and Kranthi Kiran GV and Shawon Ashraf and Daniel Auras and Björn Plüster and Jan Philipp Harries and Loïc Magne and Isabelle Mohr and Mariya Hendriksen and Dawei Zhu and Hippolyte Gisserot-Boukhlef and Tom Aarsen and Jan Kostkan and Konrad Wojtasik and Taemin Lee and Marek Šuppa and Crystina Zhang and Roberta Rocca and Mohammed Hamdy and Andrianos Michail and John Yang and Manuel Faysse and Aleksei Vatolin and Nandan Thakur and Manan Dey and Dipam Vasani and Pranjal Chitale and Simone Tedeschi and Nguyen Tai and Artem Snegirev and Michael Günther and Mengzhou Xia and Weijia Shi and Xing Han Lù and Jordan Clive and Gayatri Krishnakumar and Anna Maksimova and Silvan Wehrli and Maria Tikhonova and Henil Panchal and Aleksandr Abramov and Malte Ostendorff and Zheng Liu and Simon Clematide and Lester James Miranda and Alena Fenogenova and Guangyu Song and Ruqiya Bin Safi and Wen-Ding Li and Alessia Borghini and Federico Cassano and Hongjin Su and Jimmy Lin and Howard Yen and Lasse Hansen and Sara Hooker and Chenghao Xiao and Vaibhav Adlakha and Orion Weller and Siva Reddy and Niklas Muennighoff},
publisher = {arXiv},
journal={arXiv preprint arXiv:2502.13595},
year={2025},
url={https://arxiv.org/abs/2502.13595},
doi = {10.48550/arXiv.2502.13595},
}
@article{muennighoff2022mteb,
author = {Muennighoff, Niklas and Tazi, Nouamane and Magne, Lo{\"\i}c and Reimers, Nils},
title = {MTEB: Massive Text Embedding Benchmark},
publisher = {arXiv},
journal={arXiv preprint arXiv:2210.07316},
year = {2022}
url = {https://arxiv.org/abs/2210.07316},
doi = {10.48550/ARXIV.2210.07316},
}
```
# Dataset Statistics
<details>
<summary> Dataset Statistics</summary>
The following code contains the descriptive statistics from the task. These can also be obtained using:
```python
import mteb
task = mteb.get_task("VoyageMMarcoReranking")
desc_stats = task.metadata.descriptive_stats
```
```json
{
"test": {
"num_samples": 55423,
"number_of_characters": 8824820,
"num_documents": 53375,
"min_document_length": 19,
"average_document_length": 164.72532084309134,
"max_document_length": 1192,
"unique_documents": 53375,
"num_queries": 2048,
"min_query_length": 3,
"average_query_length": 15.9208984375,
"max_query_length": 73,
"unique_queries": 2048,
"none_queries": 0,
"num_relevant_docs": 53375,
"min_relevant_docs_per_query": 26,
"average_relevant_docs_per_query": 1.06201171875,
"max_relevant_docs_per_query": 29,
"unique_relevant_docs": 53375,
"num_instructions": null,
"min_instruction_length": null,
"average_instruction_length": null,
"max_instruction_length": null,
"unique_instructions": null,
"num_top_ranked": 2048,
"min_top_ranked_per_query": 26,
"average_top_ranked_per_query": 26.06201171875,
"max_top_ranked_per_query": 29
}
}
```
</details>
---
*This dataset card was automatically generated using [MTEB](https://github.com/embeddings-benchmark/mteb)* |
mteb/CQADupstack-Webmasters-PL | mteb | 2025-05-04T16:10:56Z | 16 | 0 | [
"task_categories:text-retrieval",
"task_ids:multiple-choice-qa",
"annotations_creators:derived",
"multilinguality:translated",
"source_datasets:mteb/cqadupstack-webmasters",
"language:pol",
"license:unknown",
"size_categories:10K<n<100K",
"format:parquet",
"modality:text",
"library:datasets",
"library:pandas",
"library:mlcroissant",
"library:polars",
"arxiv:2305.19840",
"arxiv:2502.13595",
"arxiv:2210.07316",
"region:us",
"mteb",
"text"
] | [
"text-retrieval"
] | 2025-02-05T19:19:43Z | null | ---
annotations_creators:
- derived
language:
- pol
license: unknown
multilinguality: translated
source_datasets:
- mteb/cqadupstack-webmasters
task_categories:
- text-retrieval
task_ids:
- multiple-choice-qa
dataset_info:
- config_name: corpus
features:
- name: _id
dtype: string
- name: text
dtype: string
- name: title
dtype: string
splits:
- name: test
num_bytes: 13844452
num_examples: 17405
download_size: 8365708
dataset_size: 13844452
- config_name: default
features:
- name: query-id
dtype: string
- name: corpus-id
dtype: string
- name: score
dtype: int64
splits:
- name: test
num_bytes: 35771
num_examples: 1395
download_size: 16248
dataset_size: 35771
- config_name: queries
features:
- name: _id
dtype: string
- name: text
dtype: string
splits:
- name: test
num_bytes: 38008
num_examples: 506
download_size: 26256
dataset_size: 38008
configs:
- config_name: corpus
data_files:
- split: test
path: corpus/test-*
- config_name: default
data_files:
- split: test
path: data/test-*
- config_name: queries
data_files:
- split: test
path: queries/test-*
tags:
- mteb
- text
---
<!-- adapted from https://github.com/huggingface/huggingface_hub/blob/v0.30.2/src/huggingface_hub/templates/datasetcard_template.md -->
<div align="center" style="padding: 40px 20px; background-color: white; border-radius: 12px; box-shadow: 0 2px 10px rgba(0, 0, 0, 0.05); max-width: 600px; margin: 0 auto;">
<h1 style="font-size: 3.5rem; color: #1a1a1a; margin: 0 0 20px 0; letter-spacing: 2px; font-weight: 700;">CQADupstack-Webmasters-PL</h1>
<div style="font-size: 1.5rem; color: #4a4a4a; margin-bottom: 5px; font-weight: 300;">An <a href="https://github.com/embeddings-benchmark/mteb" style="color: #2c5282; font-weight: 600; text-decoration: none;" onmouseover="this.style.textDecoration='underline'" onmouseout="this.style.textDecoration='none'">MTEB</a> dataset</div>
<div style="font-size: 0.9rem; color: #2c5282; margin-top: 10px;">Massive Text Embedding Benchmark</div>
</div>
CQADupStack: A Stack Exchange Question Duplicate Pairs Dataset
| | |
|---------------|---------------------------------------------|
| Task category | t2t |
| Domains | Written, Web |
| Reference | https://huggingface.co/datasets/clarin-knext/cqadupstack-webmasters-pl |
## How to evaluate on this task
You can evaluate an embedding model on this dataset using the following code:
```python
import mteb
task = mteb.get_tasks(["CQADupstack-Webmasters-PL"])
evaluator = mteb.MTEB(task)
model = mteb.get_model(YOUR_MODEL)
evaluator.run(model)
```
<!-- Datasets want link to arxiv in readme to autolink dataset with paper -->
To learn more about how to run models on `mteb` task check out the [GitHub repitory](https://github.com/embeddings-benchmark/mteb).
## Citation
If you use this dataset, please cite the dataset as well as [mteb](https://github.com/embeddings-benchmark/mteb), as this dataset likely includes additional processing as a part of the [MMTEB Contribution](https://github.com/embeddings-benchmark/mteb/tree/main/docs/mmteb).
```bibtex
@misc{wojtasik2024beirpl,
archiveprefix = {arXiv},
author = {Konrad Wojtasik and Vadim Shishkin and Kacper Wołowiec and Arkadiusz Janz and Maciej Piasecki},
eprint = {2305.19840},
primaryclass = {cs.IR},
title = {BEIR-PL: Zero Shot Information Retrieval Benchmark for the Polish Language},
year = {2024},
}
@article{enevoldsen2025mmtebmassivemultilingualtext,
title={MMTEB: Massive Multilingual Text Embedding Benchmark},
author={Kenneth Enevoldsen and Isaac Chung and Imene Kerboua and Márton Kardos and Ashwin Mathur and David Stap and Jay Gala and Wissam Siblini and Dominik Krzemiński and Genta Indra Winata and Saba Sturua and Saiteja Utpala and Mathieu Ciancone and Marion Schaeffer and Gabriel Sequeira and Diganta Misra and Shreeya Dhakal and Jonathan Rystrøm and Roman Solomatin and Ömer Çağatan and Akash Kundu and Martin Bernstorff and Shitao Xiao and Akshita Sukhlecha and Bhavish Pahwa and Rafał Poświata and Kranthi Kiran GV and Shawon Ashraf and Daniel Auras and Björn Plüster and Jan Philipp Harries and Loïc Magne and Isabelle Mohr and Mariya Hendriksen and Dawei Zhu and Hippolyte Gisserot-Boukhlef and Tom Aarsen and Jan Kostkan and Konrad Wojtasik and Taemin Lee and Marek Šuppa and Crystina Zhang and Roberta Rocca and Mohammed Hamdy and Andrianos Michail and John Yang and Manuel Faysse and Aleksei Vatolin and Nandan Thakur and Manan Dey and Dipam Vasani and Pranjal Chitale and Simone Tedeschi and Nguyen Tai and Artem Snegirev and Michael Günther and Mengzhou Xia and Weijia Shi and Xing Han Lù and Jordan Clive and Gayatri Krishnakumar and Anna Maksimova and Silvan Wehrli and Maria Tikhonova and Henil Panchal and Aleksandr Abramov and Malte Ostendorff and Zheng Liu and Simon Clematide and Lester James Miranda and Alena Fenogenova and Guangyu Song and Ruqiya Bin Safi and Wen-Ding Li and Alessia Borghini and Federico Cassano and Hongjin Su and Jimmy Lin and Howard Yen and Lasse Hansen and Sara Hooker and Chenghao Xiao and Vaibhav Adlakha and Orion Weller and Siva Reddy and Niklas Muennighoff},
publisher = {arXiv},
journal={arXiv preprint arXiv:2502.13595},
year={2025},
url={https://arxiv.org/abs/2502.13595},
doi = {10.48550/arXiv.2502.13595},
}
@article{muennighoff2022mteb,
author = {Muennighoff, Niklas and Tazi, Nouamane and Magne, Lo{\"\i}c and Reimers, Nils},
title = {MTEB: Massive Text Embedding Benchmark},
publisher = {arXiv},
journal={arXiv preprint arXiv:2210.07316},
year = {2022}
url = {https://arxiv.org/abs/2210.07316},
doi = {10.48550/ARXIV.2210.07316},
}
```
# Dataset Statistics
<details>
<summary> Dataset Statistics</summary>
The following code contains the descriptive statistics from the task. These can also be obtained using:
```python
import mteb
task = mteb.get_task("CQADupstack-Webmasters-PL")
desc_stats = task.metadata.descriptive_stats
```
```json
{
"test": {
"num_samples": 17911,
"number_of_characters": 12905956,
"num_documents": 17405,
"min_document_length": 55,
"average_document_length": 739.7775926457914,
"max_document_length": 25496,
"unique_documents": 17405,
"num_queries": 506,
"min_query_length": 12,
"average_query_length": 59.5395256916996,
"max_query_length": 154,
"unique_queries": 506,
"none_queries": 0,
"num_relevant_docs": 1395,
"min_relevant_docs_per_query": 1,
"average_relevant_docs_per_query": 2.7569169960474307,
"max_relevant_docs_per_query": 207,
"unique_relevant_docs": 1395,
"num_instructions": null,
"min_instruction_length": null,
"average_instruction_length": null,
"max_instruction_length": null,
"unique_instructions": null,
"num_top_ranked": null,
"min_top_ranked_per_query": null,
"average_top_ranked_per_query": null,
"max_top_ranked_per_query": null
}
}
```
</details>
---
*This dataset card was automatically generated using [MTEB](https://github.com/embeddings-benchmark/mteb)* |
mteb/HotpotQA_test_top_250_only_w_correct-v2 | mteb | 2025-05-04T16:10:16Z | 683 | 0 | [
"task_categories:text-retrieval",
"task_ids:multiple-choice-qa",
"annotations_creators:human-annotated",
"multilinguality:monolingual",
"source_datasets:mteb/hotpotqa",
"language:eng",
"license:cc-by-sa-4.0",
"size_categories:100K<n<1M",
"format:parquet",
"modality:text",
"library:datasets",
"library:pandas",
"library:mlcroissant",
"library:polars",
"arxiv:2502.13595",
"arxiv:2210.07316",
"region:us",
"mteb",
"text"
] | [
"text-retrieval"
] | 2024-09-28T05:55:11Z | null | ---
annotations_creators:
- human-annotated
language:
- eng
license: cc-by-sa-4.0
multilinguality: monolingual
source_datasets:
- mteb/hotpotqa
task_categories:
- text-retrieval
task_ids:
- multiple-choice-qa
dataset_info:
- config_name: corpus
features:
- name: _id
dtype: string
- name: text
dtype: string
- name: title
dtype: string
splits:
- name: test
num_bytes: 69897420.06567885
num_examples: 225621
download_size: 59246411
dataset_size: 69897420.06567885
- config_name: default
features:
- name: query-id
dtype: string
- name: corpus-id
dtype: string
- name: score
dtype: int64
splits:
- name: test
num_bytes: 93923.56515867657
num_examples: 2000
download_size: 40450
dataset_size: 93923.56515867657
- config_name: queries
features:
- name: _id
dtype: string
- name: text
dtype: string
splits:
- name: test
num_bytes: 124244.29439567859
num_examples: 1000
download_size: 85083
dataset_size: 124244.29439567859
configs:
- config_name: corpus
data_files:
- split: test
path: corpus/test-*
- config_name: default
data_files:
- split: test
path: data/test-*
- config_name: queries
data_files:
- split: test
path: queries/test-*
tags:
- mteb
- text
---
<!-- adapted from https://github.com/huggingface/huggingface_hub/blob/v0.30.2/src/huggingface_hub/templates/datasetcard_template.md -->
<div align="center" style="padding: 40px 20px; background-color: white; border-radius: 12px; box-shadow: 0 2px 10px rgba(0, 0, 0, 0.05); max-width: 600px; margin: 0 auto;">
<h1 style="font-size: 3.5rem; color: #1a1a1a; margin: 0 0 20px 0; letter-spacing: 2px; font-weight: 700;">HotpotQAHardNegatives</h1>
<div style="font-size: 1.5rem; color: #4a4a4a; margin-bottom: 5px; font-weight: 300;">An <a href="https://github.com/embeddings-benchmark/mteb" style="color: #2c5282; font-weight: 600; text-decoration: none;" onmouseover="this.style.textDecoration='underline'" onmouseout="this.style.textDecoration='none'">MTEB</a> dataset</div>
<div style="font-size: 0.9rem; color: #2c5282; margin-top: 10px;">Massive Text Embedding Benchmark</div>
</div>
HotpotQA is a question answering dataset featuring natural, multi-hop questions, with strong supervision for supporting facts to enable more explainable question answering systems. The hard negative version has been created by pooling the 250 top documents per query from BM25, e5-multilingual-large and e5-mistral-instruct.
| | |
|---------------|---------------------------------------------|
| Task category | t2t |
| Domains | Web, Written |
| Reference | https://hotpotqa.github.io/ |
## How to evaluate on this task
You can evaluate an embedding model on this dataset using the following code:
```python
import mteb
task = mteb.get_tasks(["HotpotQAHardNegatives"])
evaluator = mteb.MTEB(task)
model = mteb.get_model(YOUR_MODEL)
evaluator.run(model)
```
<!-- Datasets want link to arxiv in readme to autolink dataset with paper -->
To learn more about how to run models on `mteb` task check out the [GitHub repitory](https://github.com/embeddings-benchmark/mteb).
## Citation
If you use this dataset, please cite the dataset as well as [mteb](https://github.com/embeddings-benchmark/mteb), as this dataset likely includes additional processing as a part of the [MMTEB Contribution](https://github.com/embeddings-benchmark/mteb/tree/main/docs/mmteb).
```bibtex
@inproceedings{yang-etal-2018-hotpotqa,
abstract = {Existing question answering (QA) datasets fail to train QA systems to perform complex reasoning and provide explanations for answers. We introduce HotpotQA, a new dataset with 113k Wikipedia-based question-answer pairs with four key features: (1) the questions require finding and reasoning over multiple supporting documents to answer; (2) the questions are diverse and not constrained to any pre-existing knowledge bases or knowledge schemas; (3) we provide sentence-level supporting facts required for reasoning, allowing QA systems to reason with strong supervision and explain the predictions; (4) we offer a new type of factoid comparison questions to test QA systems{'} ability to extract relevant facts and perform necessary comparison. We show that HotpotQA is challenging for the latest QA systems, and the supporting facts enable models to improve performance and make explainable predictions.},
address = {Brussels, Belgium},
author = {Yang, Zhilin and
Qi, Peng and
Zhang, Saizheng and
Bengio, Yoshua and
Cohen, William and
Salakhutdinov, Ruslan and
Manning, Christopher D.},
booktitle = {Proceedings of the 2018 Conference on Empirical Methods in Natural Language Processing},
doi = {10.18653/v1/D18-1259},
editor = {Riloff, Ellen and
Chiang, David and
Hockenmaier, Julia and
Tsujii, Jun{'}ichi},
month = oct # {-} # nov,
pages = {2369--2380},
publisher = {Association for Computational Linguistics},
title = {{H}otpot{QA}: A Dataset for Diverse, Explainable Multi-hop Question Answering},
url = {https://aclanthology.org/D18-1259},
year = {2018},
}
@article{enevoldsen2025mmtebmassivemultilingualtext,
title={MMTEB: Massive Multilingual Text Embedding Benchmark},
author={Kenneth Enevoldsen and Isaac Chung and Imene Kerboua and Márton Kardos and Ashwin Mathur and David Stap and Jay Gala and Wissam Siblini and Dominik Krzemiński and Genta Indra Winata and Saba Sturua and Saiteja Utpala and Mathieu Ciancone and Marion Schaeffer and Gabriel Sequeira and Diganta Misra and Shreeya Dhakal and Jonathan Rystrøm and Roman Solomatin and Ömer Çağatan and Akash Kundu and Martin Bernstorff and Shitao Xiao and Akshita Sukhlecha and Bhavish Pahwa and Rafał Poświata and Kranthi Kiran GV and Shawon Ashraf and Daniel Auras and Björn Plüster and Jan Philipp Harries and Loïc Magne and Isabelle Mohr and Mariya Hendriksen and Dawei Zhu and Hippolyte Gisserot-Boukhlef and Tom Aarsen and Jan Kostkan and Konrad Wojtasik and Taemin Lee and Marek Šuppa and Crystina Zhang and Roberta Rocca and Mohammed Hamdy and Andrianos Michail and John Yang and Manuel Faysse and Aleksei Vatolin and Nandan Thakur and Manan Dey and Dipam Vasani and Pranjal Chitale and Simone Tedeschi and Nguyen Tai and Artem Snegirev and Michael Günther and Mengzhou Xia and Weijia Shi and Xing Han Lù and Jordan Clive and Gayatri Krishnakumar and Anna Maksimova and Silvan Wehrli and Maria Tikhonova and Henil Panchal and Aleksandr Abramov and Malte Ostendorff and Zheng Liu and Simon Clematide and Lester James Miranda and Alena Fenogenova and Guangyu Song and Ruqiya Bin Safi and Wen-Ding Li and Alessia Borghini and Federico Cassano and Hongjin Su and Jimmy Lin and Howard Yen and Lasse Hansen and Sara Hooker and Chenghao Xiao and Vaibhav Adlakha and Orion Weller and Siva Reddy and Niklas Muennighoff},
publisher = {arXiv},
journal={arXiv preprint arXiv:2502.13595},
year={2025},
url={https://arxiv.org/abs/2502.13595},
doi = {10.48550/arXiv.2502.13595},
}
@article{muennighoff2022mteb,
author = {Muennighoff, Niklas and Tazi, Nouamane and Magne, Lo{\"\i}c and Reimers, Nils},
title = {MTEB: Massive Text Embedding Benchmark},
publisher = {arXiv},
journal={arXiv preprint arXiv:2210.07316},
year = {2022}
url = {https://arxiv.org/abs/2210.07316},
doi = {10.48550/ARXIV.2210.07316},
}
```
# Dataset Statistics
<details>
<summary> Dataset Statistics</summary>
The following code contains the descriptive statistics from the task. These can also be obtained using:
```python
import mteb
task = mteb.get_task("HotpotQAHardNegatives")
desc_stats = task.metadata.descriptive_stats
```
```json
{
"test": {
"num_samples": 226621,
"number_of_characters": 84600920,
"num_documents": 225621,
"min_document_length": 9,
"average_document_length": 374.558822095461,
"max_document_length": 3463,
"unique_documents": 225621,
"num_queries": 1000,
"min_query_length": 34,
"average_query_length": 92.584,
"max_query_length": 288,
"unique_queries": 1000,
"none_queries": 0,
"num_relevant_docs": 2000,
"min_relevant_docs_per_query": 2,
"average_relevant_docs_per_query": 2.0,
"max_relevant_docs_per_query": 2,
"unique_relevant_docs": 1975,
"num_instructions": null,
"min_instruction_length": null,
"average_instruction_length": null,
"max_instruction_length": null,
"unique_instructions": null,
"num_top_ranked": null,
"min_top_ranked_per_query": null,
"average_top_ranked_per_query": null,
"max_top_ranked_per_query": null
}
}
```
</details>
---
*This dataset card was automatically generated using [MTEB](https://github.com/embeddings-benchmark/mteb)* |
mteb/cqadupstack-mathematica | mteb | 2025-05-04T16:09:59Z | 380 | 1 | [
"task_categories:text-retrieval",
"task_ids:multiple-choice-qa",
"annotations_creators:derived",
"multilinguality:monolingual",
"language:eng",
"license:apache-2.0",
"size_categories:10K<n<100K",
"format:json",
"modality:text",
"library:datasets",
"library:pandas",
"library:mlcroissant",
"library:polars",
"arxiv:2502.13595",
"arxiv:2210.07316",
"region:us",
"mteb",
"text"
] | [
"text-retrieval"
] | 2024-03-02T19:36:14Z | null | ---
annotations_creators:
- derived
language:
- eng
license: apache-2.0
multilinguality: monolingual
task_categories:
- text-retrieval
task_ids:
- multiple-choice-qa
config_names:
- corpus
tags:
- mteb
- text
dataset_info:
- config_name: default
features:
- name: query-id
dtype: string
- name: corpus-id
dtype: string
- name: score
dtype: float64
splits:
- name: test
num_bytes: 34691
num_examples: 1358
- config_name: corpus
features:
- name: _id
dtype: string
- name: title
dtype: string
- name: text
dtype: string
splits:
- name: corpus
num_bytes: 19568620
num_examples: 16705
- config_name: queries
features:
- name: _id
dtype: string
- name: text
dtype: string
splits:
- name: queries
num_bytes: 49576
num_examples: 804
configs:
- config_name: default
data_files:
- split: test
path: qrels/test.jsonl
- config_name: corpus
data_files:
- split: corpus
path: corpus.jsonl
- config_name: queries
data_files:
- split: queries
path: queries.jsonl
---
<!-- adapted from https://github.com/huggingface/huggingface_hub/blob/v0.30.2/src/huggingface_hub/templates/datasetcard_template.md -->
<div align="center" style="padding: 40px 20px; background-color: white; border-radius: 12px; box-shadow: 0 2px 10px rgba(0, 0, 0, 0.05); max-width: 600px; margin: 0 auto;">
<h1 style="font-size: 3.5rem; color: #1a1a1a; margin: 0 0 20px 0; letter-spacing: 2px; font-weight: 700;">CQADupstackMathematicaRetrieval</h1>
<div style="font-size: 1.5rem; color: #4a4a4a; margin-bottom: 5px; font-weight: 300;">An <a href="https://github.com/embeddings-benchmark/mteb" style="color: #2c5282; font-weight: 600; text-decoration: none;" onmouseover="this.style.textDecoration='underline'" onmouseout="this.style.textDecoration='none'">MTEB</a> dataset</div>
<div style="font-size: 0.9rem; color: #2c5282; margin-top: 10px;">Massive Text Embedding Benchmark</div>
</div>
CQADupStack: A Benchmark Data Set for Community Question-Answering Research
| | |
|---------------|---------------------------------------------|
| Task category | t2t |
| Domains | Written, Academic, Non-fiction |
| Reference | http://nlp.cis.unimelb.edu.au/resources/cqadupstack/ |
## How to evaluate on this task
You can evaluate an embedding model on this dataset using the following code:
```python
import mteb
task = mteb.get_tasks(["CQADupstackMathematicaRetrieval"])
evaluator = mteb.MTEB(task)
model = mteb.get_model(YOUR_MODEL)
evaluator.run(model)
```
<!-- Datasets want link to arxiv in readme to autolink dataset with paper -->
To learn more about how to run models on `mteb` task check out the [GitHub repitory](https://github.com/embeddings-benchmark/mteb).
## Citation
If you use this dataset, please cite the dataset as well as [mteb](https://github.com/embeddings-benchmark/mteb), as this dataset likely includes additional processing as a part of the [MMTEB Contribution](https://github.com/embeddings-benchmark/mteb/tree/main/docs/mmteb).
```bibtex
@inproceedings{hoogeveen2015,
acmid = {2838934},
address = {New York, NY, USA},
articleno = {3},
author = {Hoogeveen, Doris and Verspoor, Karin M. and Baldwin, Timothy},
booktitle = {Proceedings of the 20th Australasian Document Computing Symposium (ADCS)},
doi = {10.1145/2838931.2838934},
isbn = {978-1-4503-4040-3},
location = {Parramatta, NSW, Australia},
numpages = {8},
pages = {3:1--3:8},
publisher = {ACM},
series = {ADCS '15},
title = {CQADupStack: A Benchmark Data Set for Community Question-Answering Research},
url = {http://doi.acm.org/10.1145/2838931.2838934},
year = {2015},
}
@article{enevoldsen2025mmtebmassivemultilingualtext,
title={MMTEB: Massive Multilingual Text Embedding Benchmark},
author={Kenneth Enevoldsen and Isaac Chung and Imene Kerboua and Márton Kardos and Ashwin Mathur and David Stap and Jay Gala and Wissam Siblini and Dominik Krzemiński and Genta Indra Winata and Saba Sturua and Saiteja Utpala and Mathieu Ciancone and Marion Schaeffer and Gabriel Sequeira and Diganta Misra and Shreeya Dhakal and Jonathan Rystrøm and Roman Solomatin and Ömer Çağatan and Akash Kundu and Martin Bernstorff and Shitao Xiao and Akshita Sukhlecha and Bhavish Pahwa and Rafał Poświata and Kranthi Kiran GV and Shawon Ashraf and Daniel Auras and Björn Plüster and Jan Philipp Harries and Loïc Magne and Isabelle Mohr and Mariya Hendriksen and Dawei Zhu and Hippolyte Gisserot-Boukhlef and Tom Aarsen and Jan Kostkan and Konrad Wojtasik and Taemin Lee and Marek Šuppa and Crystina Zhang and Roberta Rocca and Mohammed Hamdy and Andrianos Michail and John Yang and Manuel Faysse and Aleksei Vatolin and Nandan Thakur and Manan Dey and Dipam Vasani and Pranjal Chitale and Simone Tedeschi and Nguyen Tai and Artem Snegirev and Michael Günther and Mengzhou Xia and Weijia Shi and Xing Han Lù and Jordan Clive and Gayatri Krishnakumar and Anna Maksimova and Silvan Wehrli and Maria Tikhonova and Henil Panchal and Aleksandr Abramov and Malte Ostendorff and Zheng Liu and Simon Clematide and Lester James Miranda and Alena Fenogenova and Guangyu Song and Ruqiya Bin Safi and Wen-Ding Li and Alessia Borghini and Federico Cassano and Hongjin Su and Jimmy Lin and Howard Yen and Lasse Hansen and Sara Hooker and Chenghao Xiao and Vaibhav Adlakha and Orion Weller and Siva Reddy and Niklas Muennighoff},
publisher = {arXiv},
journal={arXiv preprint arXiv:2502.13595},
year={2025},
url={https://arxiv.org/abs/2502.13595},
doi = {10.48550/arXiv.2502.13595},
}
@article{muennighoff2022mteb,
author = {Muennighoff, Niklas and Tazi, Nouamane and Magne, Lo{\"\i}c and Reimers, Nils},
title = {MTEB: Massive Text Embedding Benchmark},
publisher = {arXiv},
journal={arXiv preprint arXiv:2210.07316},
year = {2022}
url = {https://arxiv.org/abs/2210.07316},
doi = {10.48550/ARXIV.2210.07316},
}
```
# Dataset Statistics
<details>
<summary> Dataset Statistics</summary>
The following code contains the descriptive statistics from the task. These can also be obtained using:
```python
import mteb
task = mteb.get_task("CQADupstackMathematicaRetrieval")
desc_stats = task.metadata.descriptive_stats
```
```json
{
"test": {
"num_samples": 17509,
"number_of_characters": 19325188,
"num_documents": 16705,
"min_document_length": 75,
"average_document_length": 1154.4967375037413,
"max_document_length": 28907,
"unique_documents": 16705,
"num_queries": 804,
"min_query_length": 15,
"average_query_length": 48.90547263681592,
"max_query_length": 137,
"unique_queries": 804,
"none_queries": 0,
"num_relevant_docs": 1358,
"min_relevant_docs_per_query": 1,
"average_relevant_docs_per_query": 1.6890547263681592,
"max_relevant_docs_per_query": 56,
"unique_relevant_docs": 1358,
"num_instructions": null,
"min_instruction_length": null,
"average_instruction_length": null,
"max_instruction_length": null,
"unique_instructions": null,
"num_top_ranked": null,
"min_top_ranked_per_query": null,
"average_top_ranked_per_query": null,
"max_top_ranked_per_query": null
}
}
```
</details>
---
*This dataset card was automatically generated using [MTEB](https://github.com/embeddings-benchmark/mteb)* |
mteb/tweet_sentiment_extraction | mteb | 2025-05-04T16:07:55Z | 4,415 | 27 | [
"task_categories:text-classification",
"task_ids:sentiment-analysis",
"task_ids:sentiment-scoring",
"task_ids:sentiment-classification",
"task_ids:hate-speech-detection",
"annotations_creators:human-annotated",
"multilinguality:monolingual",
"language:eng",
"license:unknown",
"size_categories:10K<n<100K",
"format:parquet",
"modality:text",
"library:datasets",
"library:pandas",
"library:mlcroissant",
"library:polars",
"arxiv:2502.13595",
"arxiv:2210.07316",
"region:us",
"mteb",
"text"
] | [
"text-classification"
] | 2022-05-26T18:07:50Z | null | ---
annotations_creators:
- human-annotated
language:
- eng
license: unknown
multilinguality: monolingual
task_categories:
- text-classification
task_ids:
- sentiment-analysis
- sentiment-scoring
- sentiment-classification
- hate-speech-detection
tags:
- mteb
- text
configs:
- config_name: default
data_files:
- split: train
path: data/train-*
- split: test
path: data/test-*
dataset_info:
features:
- name: text
dtype: string
- name: label
dtype: int64
splits:
- name: train
num_bytes: 2208166
num_examples: 27481
- name: test
num_bytes: 281934
num_examples: 3534
download_size: 1710860
dataset_size: 2490100
---
<!-- adapted from https://github.com/huggingface/huggingface_hub/blob/v0.30.2/src/huggingface_hub/templates/datasetcard_template.md -->
<div align="center" style="padding: 40px 20px; background-color: white; border-radius: 12px; box-shadow: 0 2px 10px rgba(0, 0, 0, 0.05); max-width: 600px; margin: 0 auto;">
<h1 style="font-size: 3.5rem; color: #1a1a1a; margin: 0 0 20px 0; letter-spacing: 2px; font-weight: 700;">TweetSentimentExtractionClassification</h1>
<div style="font-size: 1.5rem; color: #4a4a4a; margin-bottom: 5px; font-weight: 300;">An <a href="https://github.com/embeddings-benchmark/mteb" style="color: #2c5282; font-weight: 600; text-decoration: none;" onmouseover="this.style.textDecoration='underline'" onmouseout="this.style.textDecoration='none'">MTEB</a> dataset</div>
<div style="font-size: 0.9rem; color: #2c5282; margin-top: 10px;">Massive Text Embedding Benchmark</div>
</div>
| | |
|---------------|---------------------------------------------|
| Task category | t2c |
| Domains | Social, Written |
| Reference | https://www.kaggle.com/competitions/tweet-sentiment-extraction/overview |
## How to evaluate on this task
You can evaluate an embedding model on this dataset using the following code:
```python
import mteb
task = mteb.get_tasks(["TweetSentimentExtractionClassification"])
evaluator = mteb.MTEB(task)
model = mteb.get_model(YOUR_MODEL)
evaluator.run(model)
```
<!-- Datasets want link to arxiv in readme to autolink dataset with paper -->
To learn more about how to run models on `mteb` task check out the [GitHub repitory](https://github.com/embeddings-benchmark/mteb).
## Citation
If you use this dataset, please cite the dataset as well as [mteb](https://github.com/embeddings-benchmark/mteb), as this dataset likely includes additional processing as a part of the [MMTEB Contribution](https://github.com/embeddings-benchmark/mteb/tree/main/docs/mmteb).
```bibtex
@misc{tweet-sentiment-extraction,
author = {Maggie, Phil Culliton, Wei Chen},
publisher = {Kaggle},
title = {Tweet Sentiment Extraction},
url = {https://kaggle.com/competitions/tweet-sentiment-extraction},
year = {2020},
}
@article{enevoldsen2025mmtebmassivemultilingualtext,
title={MMTEB: Massive Multilingual Text Embedding Benchmark},
author={Kenneth Enevoldsen and Isaac Chung and Imene Kerboua and Márton Kardos and Ashwin Mathur and David Stap and Jay Gala and Wissam Siblini and Dominik Krzemiński and Genta Indra Winata and Saba Sturua and Saiteja Utpala and Mathieu Ciancone and Marion Schaeffer and Gabriel Sequeira and Diganta Misra and Shreeya Dhakal and Jonathan Rystrøm and Roman Solomatin and Ömer Çağatan and Akash Kundu and Martin Bernstorff and Shitao Xiao and Akshita Sukhlecha and Bhavish Pahwa and Rafał Poświata and Kranthi Kiran GV and Shawon Ashraf and Daniel Auras and Björn Plüster and Jan Philipp Harries and Loïc Magne and Isabelle Mohr and Mariya Hendriksen and Dawei Zhu and Hippolyte Gisserot-Boukhlef and Tom Aarsen and Jan Kostkan and Konrad Wojtasik and Taemin Lee and Marek Šuppa and Crystina Zhang and Roberta Rocca and Mohammed Hamdy and Andrianos Michail and John Yang and Manuel Faysse and Aleksei Vatolin and Nandan Thakur and Manan Dey and Dipam Vasani and Pranjal Chitale and Simone Tedeschi and Nguyen Tai and Artem Snegirev and Michael Günther and Mengzhou Xia and Weijia Shi and Xing Han Lù and Jordan Clive and Gayatri Krishnakumar and Anna Maksimova and Silvan Wehrli and Maria Tikhonova and Henil Panchal and Aleksandr Abramov and Malte Ostendorff and Zheng Liu and Simon Clematide and Lester James Miranda and Alena Fenogenova and Guangyu Song and Ruqiya Bin Safi and Wen-Ding Li and Alessia Borghini and Federico Cassano and Hongjin Su and Jimmy Lin and Howard Yen and Lasse Hansen and Sara Hooker and Chenghao Xiao and Vaibhav Adlakha and Orion Weller and Siva Reddy and Niklas Muennighoff},
publisher = {arXiv},
journal={arXiv preprint arXiv:2502.13595},
year={2025},
url={https://arxiv.org/abs/2502.13595},
doi = {10.48550/arXiv.2502.13595},
}
@article{muennighoff2022mteb,
author = {Muennighoff, Niklas and Tazi, Nouamane and Magne, Lo{\"\i}c and Reimers, Nils},
title = {MTEB: Massive Text Embedding Benchmark},
publisher = {arXiv},
journal={arXiv preprint arXiv:2210.07316},
year = {2022}
url = {https://arxiv.org/abs/2210.07316},
doi = {10.48550/ARXIV.2210.07316},
}
```
# Dataset Statistics
<details>
<summary> Dataset Statistics</summary>
The following code contains the descriptive statistics from the task. These can also be obtained using:
```python
import mteb
task = mteb.get_task("TweetSentimentExtractionClassification")
desc_stats = task.metadata.descriptive_stats
```
```json
{
"test": {
"num_samples": 3534,
"number_of_characters": 239476,
"number_texts_intersect_with_train": 0,
"min_text_length": 4,
"average_text_length": 67.76344086021506,
"max_text_length": 142,
"unique_text": 3534,
"unique_labels": 3,
"labels": {
"1": {
"count": 1430
},
"2": {
"count": 1103
},
"0": {
"count": 1001
}
}
},
"train": {
"num_samples": 27481,
"number_of_characters": 1877709,
"number_texts_intersect_with_train": null,
"min_text_length": 0,
"average_text_length": 68.32753538808632,
"max_text_length": 141,
"unique_text": 27481,
"unique_labels": 3,
"labels": {
"1": {
"count": 11118
},
"0": {
"count": 7781
},
"2": {
"count": 8582
}
}
}
}
```
</details>
---
*This dataset card was automatically generated using [MTEB](https://github.com/embeddings-benchmark/mteb)* |
mteb/HotelReviewSentimentClassification | mteb | 2025-05-04T16:07:41Z | 11 | 0 | [
"task_categories:text-classification",
"task_ids:sentiment-analysis",
"task_ids:sentiment-scoring",
"task_ids:sentiment-classification",
"task_ids:hate-speech-detection",
"annotations_creators:derived",
"multilinguality:monolingual",
"language:ara",
"license:unknown",
"size_categories:1K<n<10K",
"format:parquet",
"modality:text",
"library:datasets",
"library:pandas",
"library:mlcroissant",
"library:polars",
"arxiv:2502.13595",
"arxiv:2210.07316",
"region:us",
"mteb",
"text"
] | [
"text-classification"
] | 2024-12-21T10:42:59Z | null | ---
annotations_creators:
- derived
language:
- ara
license: unknown
multilinguality: monolingual
task_categories:
- text-classification
task_ids:
- sentiment-analysis
- sentiment-scoring
- sentiment-classification
- hate-speech-detection
dataset_info:
features:
- name: text
dtype: string
- name: label
dtype: int64
splits:
- name: train
num_bytes: 536128
num_examples: 2048
download_size: 274359
dataset_size: 536128
configs:
- config_name: default
data_files:
- split: train
path: data/train-*
tags:
- mteb
- text
---
<!-- adapted from https://github.com/huggingface/huggingface_hub/blob/v0.30.2/src/huggingface_hub/templates/datasetcard_template.md -->
<div align="center" style="padding: 40px 20px; background-color: white; border-radius: 12px; box-shadow: 0 2px 10px rgba(0, 0, 0, 0.05); max-width: 600px; margin: 0 auto;">
<h1 style="font-size: 3.5rem; color: #1a1a1a; margin: 0 0 20px 0; letter-spacing: 2px; font-weight: 700;">HotelReviewSentimentClassification</h1>
<div style="font-size: 1.5rem; color: #4a4a4a; margin-bottom: 5px; font-weight: 300;">An <a href="https://github.com/embeddings-benchmark/mteb" style="color: #2c5282; font-weight: 600; text-decoration: none;" onmouseover="this.style.textDecoration='underline'" onmouseout="this.style.textDecoration='none'">MTEB</a> dataset</div>
<div style="font-size: 0.9rem; color: #2c5282; margin-top: 10px;">Massive Text Embedding Benchmark</div>
</div>
HARD is a dataset of Arabic hotel reviews collected from the Booking.com website.
| | |
|---------------|---------------------------------------------|
| Task category | t2c |
| Domains | Reviews, Written |
| Reference | https://link.springer.com/chapter/10.1007/978-3-319-67056-0_3 |
## How to evaluate on this task
You can evaluate an embedding model on this dataset using the following code:
```python
import mteb
task = mteb.get_tasks(["HotelReviewSentimentClassification"])
evaluator = mteb.MTEB(task)
model = mteb.get_model(YOUR_MODEL)
evaluator.run(model)
```
<!-- Datasets want link to arxiv in readme to autolink dataset with paper -->
To learn more about how to run models on `mteb` task check out the [GitHub repitory](https://github.com/embeddings-benchmark/mteb).
## Citation
If you use this dataset, please cite the dataset as well as [mteb](https://github.com/embeddings-benchmark/mteb), as this dataset likely includes additional processing as a part of the [MMTEB Contribution](https://github.com/embeddings-benchmark/mteb/tree/main/docs/mmteb).
```bibtex
@article{elnagar2018hotel,
author = {Elnagar, Ashraf and Khalifa, Yasmin S and Einea, Anas},
journal = {Intelligent natural language processing: Trends and applications},
pages = {35--52},
publisher = {Springer},
title = {Hotel Arabic-reviews dataset construction for sentiment analysis applications},
year = {2018},
}
@article{enevoldsen2025mmtebmassivemultilingualtext,
title={MMTEB: Massive Multilingual Text Embedding Benchmark},
author={Kenneth Enevoldsen and Isaac Chung and Imene Kerboua and Márton Kardos and Ashwin Mathur and David Stap and Jay Gala and Wissam Siblini and Dominik Krzemiński and Genta Indra Winata and Saba Sturua and Saiteja Utpala and Mathieu Ciancone and Marion Schaeffer and Gabriel Sequeira and Diganta Misra and Shreeya Dhakal and Jonathan Rystrøm and Roman Solomatin and Ömer Çağatan and Akash Kundu and Martin Bernstorff and Shitao Xiao and Akshita Sukhlecha and Bhavish Pahwa and Rafał Poświata and Kranthi Kiran GV and Shawon Ashraf and Daniel Auras and Björn Plüster and Jan Philipp Harries and Loïc Magne and Isabelle Mohr and Mariya Hendriksen and Dawei Zhu and Hippolyte Gisserot-Boukhlef and Tom Aarsen and Jan Kostkan and Konrad Wojtasik and Taemin Lee and Marek Šuppa and Crystina Zhang and Roberta Rocca and Mohammed Hamdy and Andrianos Michail and John Yang and Manuel Faysse and Aleksei Vatolin and Nandan Thakur and Manan Dey and Dipam Vasani and Pranjal Chitale and Simone Tedeschi and Nguyen Tai and Artem Snegirev and Michael Günther and Mengzhou Xia and Weijia Shi and Xing Han Lù and Jordan Clive and Gayatri Krishnakumar and Anna Maksimova and Silvan Wehrli and Maria Tikhonova and Henil Panchal and Aleksandr Abramov and Malte Ostendorff and Zheng Liu and Simon Clematide and Lester James Miranda and Alena Fenogenova and Guangyu Song and Ruqiya Bin Safi and Wen-Ding Li and Alessia Borghini and Federico Cassano and Hongjin Su and Jimmy Lin and Howard Yen and Lasse Hansen and Sara Hooker and Chenghao Xiao and Vaibhav Adlakha and Orion Weller and Siva Reddy and Niklas Muennighoff},
publisher = {arXiv},
journal={arXiv preprint arXiv:2502.13595},
year={2025},
url={https://arxiv.org/abs/2502.13595},
doi = {10.48550/arXiv.2502.13595},
}
@article{muennighoff2022mteb,
author = {Muennighoff, Niklas and Tazi, Nouamane and Magne, Lo{\"\i}c and Reimers, Nils},
title = {MTEB: Massive Text Embedding Benchmark},
publisher = {arXiv},
journal={arXiv preprint arXiv:2210.07316},
year = {2022}
url = {https://arxiv.org/abs/2210.07316},
doi = {10.48550/ARXIV.2210.07316},
}
```
# Dataset Statistics
<details>
<summary> Dataset Statistics</summary>
The following code contains the descriptive statistics from the task. These can also be obtained using:
```python
import mteb
task = mteb.get_task("HotelReviewSentimentClassification")
desc_stats = task.metadata.descriptive_stats
```
```json
{
"train": {
"num_samples": 2048,
"number_of_characters": 282368,
"number_texts_intersect_with_train": null,
"min_text_length": 11,
"average_text_length": 137.875,
"max_text_length": 2698,
"unique_text": 2044,
"unique_labels": 4,
"labels": {
"4": {
"count": 512
},
"3": {
"count": 512
},
"0": {
"count": 279
},
"1": {
"count": 745
}
}
}
}
```
</details>
---
*This dataset card was automatically generated using [MTEB](https://github.com/embeddings-benchmark/mteb)* |
darkme-ai/LMMFinQA | darkme-ai | 2025-05-04T15:53:07Z | 0 | 0 | [
"license:mit",
"size_categories:n<1K",
"format:imagefolder",
"modality:image",
"library:datasets",
"library:mlcroissant",
"region:us"
] | [] | 2025-05-03T06:22:05Z | null | ---
license: mit
---
## 数据集结构
### 数据实例
以下是数据集的示例,展示了不同任务类型的数据格式:
#### 文本分类示例
```python
{
"id": "1",
"image": [
"itd1_1.jpg"
],
"qa_type": [
"text_and_table_based_qa"
],
"question": "<rk>不同的财务报表类型,利润表中“本期金额/本期数/本月金额”填写方法如下:\n1、小企业会计准则:填写本期(即本季度)的发生额,例如第二季度填写“4-6月份累计”;\n2、企业会计制度:填写本期(即本季度)的发生额,例如第二季度填写“4-6月份累计”;\n3、一般企业会计准则:填写从年初到本期期末的累计发生额,例如第二季度填写“1-6月份累计”。已执行和未执行新准则,填写规则是一样的,只是表中明细科目有所差异。</rk>\n<image>\n中,企业执行企业会计准则,利润表中本期金额是否只填写4到6月的发生额?"
} |
Themira/multilingual_parallel_data | Themira | 2025-05-04T15:44:48Z | 0 | 0 | [
"license:apache-2.0",
"region:us"
] | [] | 2025-05-04T15:42:08Z | null | ---
license: apache-2.0
---
|
HungVu2003/opt-350m_beta_0.0_alpha_0.2_num-company_2_dataset_0_for_gen_10_v2 | HungVu2003 | 2025-05-04T15:43:36Z | 0 | 0 | [
"region:us"
] | [] | 2025-05-04T15:43:34Z | null | ---
dataset_info:
features:
- name: question
dtype: string
splits:
- name: train
num_bytes: 2722918
num_examples: 13750
download_size: 960160
dataset_size: 2722918
configs:
- config_name: default
data_files:
- split: train
path: data/train-*
---
|
HungVu2003/opt-350m_beta_1.0_alpha_0.2_num-company_2_dataset_1_for_gen_15_v2 | HungVu2003 | 2025-05-04T15:15:58Z | 0 | 0 | [
"region:us"
] | [] | 2025-05-04T15:15:57Z | null | ---
dataset_info:
features:
- name: question
dtype: string
splits:
- name: train
num_bytes: 6346402
num_examples: 13750
download_size: 3220680
dataset_size: 6346402
configs:
- config_name: default
data_files:
- split: train
path: data/train-*
---
|
LadyMia/x_dataset_17682 | LadyMia | 2025-05-04T15:11:51Z | 880 | 0 | [
"task_categories:text-classification",
"task_categories:token-classification",
"task_categories:question-answering",
"task_categories:summarization",
"task_categories:text-generation",
"task_ids:sentiment-analysis",
"task_ids:topic-classification",
"task_ids:named-entity-recognition",
"task_ids:language-modeling",
"task_ids:text-scoring",
"task_ids:multi-class-classification",
"task_ids:multi-label-classification",
"task_ids:extractive-qa",
"task_ids:news-articles-summarization",
"multilinguality:multilingual",
"source_datasets:original",
"license:mit",
"size_categories:10M<n<100M",
"format:parquet",
"modality:text",
"library:datasets",
"library:dask",
"library:mlcroissant",
"library:polars",
"region:us"
] | [
"text-classification",
"token-classification",
"question-answering",
"summarization",
"text-generation"
] | 2025-01-29T03:07:52Z | null | ---
license: mit
multilinguality:
- multilingual
source_datasets:
- original
task_categories:
- text-classification
- token-classification
- question-answering
- summarization
- text-generation
task_ids:
- sentiment-analysis
- topic-classification
- named-entity-recognition
- language-modeling
- text-scoring
- multi-class-classification
- multi-label-classification
- extractive-qa
- news-articles-summarization
---
# Bittensor Subnet 13 X (Twitter) Dataset
<center>
<img src="https://huggingface.co/datasets/macrocosm-os/images/resolve/main/bittensor.png" alt="Data-universe: The finest collection of social media data the web has to offer">
</center>
<center>
<img src="https://huggingface.co/datasets/macrocosm-os/images/resolve/main/macrocosmos-black.png" alt="Data-universe: The finest collection of social media data the web has to offer">
</center>
## Dataset Description
- **Repository:** LadyMia/x_dataset_17682
- **Subnet:** Bittensor Subnet 13
- **Miner Hotkey:** 5FgYXBnD63LNLkArKfbK1i4K2gbLbs6zULHA2DXFmhLdtFHe
### Dataset Summary
This dataset is part of the Bittensor Subnet 13 decentralized network, containing preprocessed data from X (formerly Twitter). The data is continuously updated by network miners, providing a real-time stream of tweets for various analytical and machine learning tasks.
For more information about the dataset, please visit the [official repository](https://github.com/macrocosm-os/data-universe).
### Supported Tasks
The versatility of this dataset allows researchers and data scientists to explore various aspects of social media dynamics and develop innovative applications. Users are encouraged to leverage this data creatively for their specific research or business needs.
For example:
- Sentiment Analysis
- Trend Detection
- Content Analysis
- User Behavior Modeling
### Languages
Primary language: Datasets are mostly English, but can be multilingual due to decentralized ways of creation.
## Dataset Structure
### Data Instances
Each instance represents a single tweet with the following fields:
### Data Fields
- `text` (string): The main content of the tweet.
- `label` (string): Sentiment or topic category of the tweet.
- `tweet_hashtags` (list): A list of hashtags used in the tweet. May be empty if no hashtags are present.
- `datetime` (string): The date when the tweet was posted.
- `username_encoded` (string): An encoded version of the username to maintain user privacy.
- `url_encoded` (string): An encoded version of any URLs included in the tweet. May be empty if no URLs are present.
### Data Splits
This dataset is continuously updated and does not have fixed splits. Users should create their own splits based on their requirements and the data's timestamp.
## Dataset Creation
### Source Data
Data is collected from public tweets on X (Twitter), adhering to the platform's terms of service and API usage guidelines.
### Personal and Sensitive Information
All usernames and URLs are encoded to protect user privacy. The dataset does not intentionally include personal or sensitive information.
## Considerations for Using the Data
### Social Impact and Biases
Users should be aware of potential biases inherent in X (Twitter) data, including demographic and content biases. This dataset reflects the content and opinions expressed on X and should not be considered a representative sample of the general population.
### Limitations
- Data quality may vary due to the decentralized nature of collection and preprocessing.
- The dataset may contain noise, spam, or irrelevant content typical of social media platforms.
- Temporal biases may exist due to real-time collection methods.
- The dataset is limited to public tweets and does not include private accounts or direct messages.
- Not all tweets contain hashtags or URLs.
## Additional Information
### Licensing Information
The dataset is released under the MIT license. The use of this dataset is also subject to X Terms of Use.
### Citation Information
If you use this dataset in your research, please cite it as follows:
```
@misc{LadyMia2025datauniversex_dataset_17682,
title={The Data Universe Datasets: The finest collection of social media data the web has to offer},
author={LadyMia},
year={2025},
url={https://huggingface.co/datasets/LadyMia/x_dataset_17682},
}
```
### Contributions
To report issues or contribute to the dataset, please contact the miner or use the Bittensor Subnet 13 governance mechanisms.
## Dataset Statistics
[This section is automatically updated]
- **Total Instances:** 37658378
- **Date Range:** 2025-01-22T00:00:00Z to 2025-02-13T00:00:00Z
- **Last Updated:** 2025-02-18T21:42:55Z
### Data Distribution
- Tweets with hashtags: 45.42%
- Tweets without hashtags: 54.58%
### Top 10 Hashtags
For full statistics, please refer to the `stats.json` file in the repository.
| Rank | Topic | Total Count | Percentage |
|------|-------|-------------|-------------|
| 1 | NULL | 20555610 | 54.58% |
| 2 | #riyadh | 235778 | 0.63% |
| 3 | #zelena | 224298 | 0.60% |
| 4 | #tiktok | 161795 | 0.43% |
| 5 | #ad | 91773 | 0.24% |
| 6 | #jhope_at_galadespiècesjaunes | 85795 | 0.23% |
| 7 | #bbb25 | 79808 | 0.21% |
| 8 | #transferlerlebirliktezafere | 58256 | 0.15% |
| 9 | #theheartkillersep10 | 55037 | 0.15% |
| 10 | #bbmzansi | 51161 | 0.14% |
## Update History
| Date | New Instances | Total Instances |
|------|---------------|-----------------|
| 2025-01-29T03:08:57Z | 2977993 | 2977993 |
| 2025-02-01T15:11:35Z | 7083709 | 10061702 |
| 2025-02-05T03:15:34Z | 8967127 | 19028829 |
| 2025-02-08T15:19:06Z | 9885163 | 28913992 |
| 2025-02-12T03:23:51Z | 7367286 | 36281278 |
| 2025-02-18T06:41:11Z | 659231 | 36940509 |
| 2025-02-18T21:42:55Z | 717869 | 37658378 |
|
mteb/bengali_hate_speech | mteb | 2025-05-04T15:09:57Z | 0 | 0 | [
"region:us"
] | [] | 2025-05-04T15:09:52Z | null | ---
dataset_info:
features:
- name: text
dtype: string
- name: label
dtype:
class_label:
names:
'0': 0
'1': 1
'2': 2
'3': 3
'4': 4
splits:
- name: train
num_bytes: 445907.77559976594
num_examples: 1567
- name: test
num_bytes: 431110.58074897603
num_examples: 1515
download_size: 351631
dataset_size: 877018.356348742
configs:
- config_name: default
data_files:
- split: train
path: data/train-*
- split: test
path: data/test-*
---
|
vatolinalex/tweet_sarcasm | vatolinalex | 2025-05-04T15:06:00Z | 0 | 0 | [
"region:us"
] | [] | 2025-05-04T15:05:56Z | null | ---
dataset_info:
features:
- name: dialect
dtype:
class_label:
names:
'0': egypt
'1': gulf
'2': levant
'3': magreb
'4': msa
- name: label
dtype:
class_label:
names:
'0': non-sarcastic
'1': sarcastic
- name: sentiment
dtype:
class_label:
names:
'0': negative
'1': neutral
'2': positive
- name: original_sentiment
dtype:
class_label:
names:
'0': negative
'1': neutral
'2': positive
- name: text
dtype: string
- name: source
dtype: string
splits:
- name: train
num_bytes: 1761516.7565485362
num_examples: 8125
- name: test
num_bytes: 425852.990521327
num_examples: 1961
download_size: 1120002
dataset_size: 2187369.747069863
configs:
- config_name: default
data_files:
- split: train
path: data/train-*
- split: test
path: data/test-*
---
|
vatolinalex/restaurant_review_sentiment | vatolinalex | 2025-05-04T15:05:44Z | 0 | 0 | [
"region:us"
] | [] | 2025-05-04T15:05:30Z | null | ---
dataset_info:
features:
- name: label
dtype:
class_label:
names:
'0': 0
'1': 1
- name: text
dtype: string
- name: restaurant_id
dtype: string
- name: user_id
dtype: string
splits:
- name: train
num_bytes: 2715408.5025107604
num_examples: 6279
- name: test
num_bytes: 873566.6786226686
num_examples: 2020
download_size: 1930248
dataset_size: 3588975.181133429
configs:
- config_name: default
data_files:
- split: train
path: data/train-*
- split: test
path: data/test-*
---
|
Oriolshhh/parlabe-errors-genere-60k | Oriolshhh | 2025-05-04T14:35:18Z | 0 | 0 | [
"language:ca",
"license:apache-2.0",
"size_categories:10K<n<100K",
"region:us",
"català",
"grammar-correction",
"gender",
"text-to-text",
"synthetic"
] | [] | 2025-05-04T14:30:03Z | null | ---
language: ca
license: apache-2.0
tags:
- català
- grammar-correction
- gender
- text-to-text
- synthetic
size_categories:
- 10K<n<100K
---
# Dataset d'errors de gènere en català (60.000 parelles)
Aquest dataset conté **60.000 parelles de frases** amb errors de gènere, generades mitjançant un script automatitzat en Python. Cada parella està formada per:
```text_erroni,text_correcte```
---
## Objectiu
Aquest conjunt de dades està pensat per **entrenar models de correcció gramatical en català**, amb un focus específic en la detecció i correcció **d’errors de gènere**:
- Concordança nominal (ex: *el noia → la noia*)
- Concordança adjectival (ex: *una llibre interessant → un llibre interessant*)
- Concordança pronominal i verbal
---
## Com s’ha generat?
S’han creat automàticament frases correctes, i posteriorment s’hi han introduït **errors de gènere** de manera controlada:
- Canviant articles, pronoms, adjectius i formes verbals
- Mantenint la sintaxi coherent però incorrecta gramaticalment
Aquestes parelles s'han dissenyat per simular errors reals d'escriptura en contextos formals i informals.
---
## Format
- Llengua: Català (`ca`)
- Format: `.csv` amb dues columnes:
- `text_erroni`
- `text_correcte`
- Nombre de parelles: 60.000
|
shylee/eval_DP_cube_downDims1_cropNo_freeze1_16_16_ema1_1e-4_ckpt300000 | shylee | 2025-05-04T14:34:20Z | 0 | 0 | [
"task_categories:robotics",
"license:apache-2.0",
"region:us",
"LeRobot",
"tutorial"
] | [
"robotics"
] | 2025-05-04T14:34:10Z | null | ---
license: apache-2.0
task_categories:
- robotics
tags:
- LeRobot
- tutorial
configs:
- config_name: default
data_files: data/*/*.parquet
---
This dataset was created using [LeRobot](https://github.com/huggingface/lerobot).
## Dataset Description
- **Homepage:** [More Information Needed]
- **Paper:** [More Information Needed]
- **License:** apache-2.0
## Dataset Structure
[meta/info.json](meta/info.json):
```json
{
"codebase_version": "v2.1",
"robot_type": "so100",
"total_episodes": 4,
"total_frames": 3310,
"total_tasks": 1,
"total_videos": 12,
"total_chunks": 1,
"chunks_size": 1000,
"fps": 30,
"splits": {
"train": "0:4"
},
"data_path": "data/chunk-{episode_chunk:03d}/episode_{episode_index:06d}.parquet",
"video_path": "videos/chunk-{episode_chunk:03d}/{video_key}/episode_{episode_index:06d}.mp4",
"features": {
"action": {
"dtype": "float32",
"shape": [
6
],
"names": [
"main_shoulder_pan",
"main_shoulder_lift",
"main_elbow_flex",
"main_wrist_flex",
"main_wrist_roll",
"main_gripper"
]
},
"observation.state": {
"dtype": "float32",
"shape": [
6
],
"names": [
"main_shoulder_pan",
"main_shoulder_lift",
"main_elbow_flex",
"main_wrist_flex",
"main_wrist_roll",
"main_gripper"
]
},
"observation.images.FrontCam": {
"dtype": "video",
"shape": [
480,
640,
3
],
"names": [
"height",
"width",
"channels"
],
"info": {
"video.fps": 30.0,
"video.height": 480,
"video.width": 640,
"video.channels": 3,
"video.codec": "av1",
"video.pix_fmt": "yuv420p",
"video.is_depth_map": false,
"has_audio": false
}
},
"observation.images.TopCam": {
"dtype": "video",
"shape": [
480,
640,
3
],
"names": [
"height",
"width",
"channels"
],
"info": {
"video.fps": 30.0,
"video.height": 480,
"video.width": 640,
"video.channels": 3,
"video.codec": "av1",
"video.pix_fmt": "yuv420p",
"video.is_depth_map": false,
"has_audio": false
}
},
"observation.images.WristCam": {
"dtype": "video",
"shape": [
480,
640,
3
],
"names": [
"height",
"width",
"channels"
],
"info": {
"video.fps": 30.0,
"video.height": 480,
"video.width": 640,
"video.channels": 3,
"video.codec": "av1",
"video.pix_fmt": "yuv420p",
"video.is_depth_map": false,
"has_audio": false
}
},
"timestamp": {
"dtype": "float32",
"shape": [
1
],
"names": null
},
"frame_index": {
"dtype": "int64",
"shape": [
1
],
"names": null
},
"episode_index": {
"dtype": "int64",
"shape": [
1
],
"names": null
},
"index": {
"dtype": "int64",
"shape": [
1
],
"names": null
},
"task_index": {
"dtype": "int64",
"shape": [
1
],
"names": null
}
}
}
```
## Citation
**BibTeX:**
```bibtex
[More Information Needed]
``` |
hshwk1983/x_dataset_2983 | hshwk1983 | 2025-05-04T14:33:54Z | 2,809 | 0 | [
"task_categories:text-classification",
"task_categories:token-classification",
"task_categories:question-answering",
"task_categories:summarization",
"task_categories:text-generation",
"task_ids:sentiment-analysis",
"task_ids:topic-classification",
"task_ids:named-entity-recognition",
"task_ids:language-modeling",
"task_ids:text-scoring",
"task_ids:multi-class-classification",
"task_ids:multi-label-classification",
"task_ids:extractive-qa",
"task_ids:news-articles-summarization",
"multilinguality:multilingual",
"source_datasets:original",
"license:mit",
"size_categories:100M<n<1B",
"format:parquet",
"modality:text",
"library:datasets",
"library:dask",
"library:mlcroissant",
"library:polars",
"region:us"
] | [
"text-classification",
"token-classification",
"question-answering",
"summarization",
"text-generation"
] | 2025-01-27T07:01:04Z | null | ---
license: mit
multilinguality:
- multilingual
source_datasets:
- original
task_categories:
- text-classification
- token-classification
- question-answering
- summarization
- text-generation
task_ids:
- sentiment-analysis
- topic-classification
- named-entity-recognition
- language-modeling
- text-scoring
- multi-class-classification
- multi-label-classification
- extractive-qa
- news-articles-summarization
---
# Bittensor Subnet 13 X (Twitter) Dataset
<center>
<img src="https://huggingface.co/datasets/macrocosm-os/images/resolve/main/bittensor.png" alt="Data-universe: The finest collection of social media data the web has to offer">
</center>
<center>
<img src="https://huggingface.co/datasets/macrocosm-os/images/resolve/main/macrocosmos-black.png" alt="Data-universe: The finest collection of social media data the web has to offer">
</center>
## Dataset Description
- **Repository:** hshwk1983/x_dataset_2983
- **Subnet:** Bittensor Subnet 13
- **Miner Hotkey:** 5F2RCkLaXEwdz4PALA5iwSBQQ4rWEAioaniBHouRyhUSYjne
### Dataset Summary
This dataset is part of the Bittensor Subnet 13 decentralized network, containing preprocessed data from X (formerly Twitter). The data is continuously updated by network miners, providing a real-time stream of tweets for various analytical and machine learning tasks.
For more information about the dataset, please visit the [official repository](https://github.com/macrocosm-os/data-universe).
### Supported Tasks
The versatility of this dataset allows researchers and data scientists to explore various aspects of social media dynamics and develop innovative applications. Users are encouraged to leverage this data creatively for their specific research or business needs.
For example:
- Sentiment Analysis
- Trend Detection
- Content Analysis
- User Behavior Modeling
### Languages
Primary language: Datasets are mostly English, but can be multilingual due to decentralized ways of creation.
## Dataset Structure
### Data Instances
Each instance represents a single tweet with the following fields:
### Data Fields
- `text` (string): The main content of the tweet.
- `label` (string): Sentiment or topic category of the tweet.
- `tweet_hashtags` (list): A list of hashtags used in the tweet. May be empty if no hashtags are present.
- `datetime` (string): The date when the tweet was posted.
- `username_encoded` (string): An encoded version of the username to maintain user privacy.
- `url_encoded` (string): An encoded version of any URLs included in the tweet. May be empty if no URLs are present.
### Data Splits
This dataset is continuously updated and does not have fixed splits. Users should create their own splits based on their requirements and the data's timestamp.
## Dataset Creation
### Source Data
Data is collected from public tweets on X (Twitter), adhering to the platform's terms of service and API usage guidelines.
### Personal and Sensitive Information
All usernames and URLs are encoded to protect user privacy. The dataset does not intentionally include personal or sensitive information.
## Considerations for Using the Data
### Social Impact and Biases
Users should be aware of potential biases inherent in X (Twitter) data, including demographic and content biases. This dataset reflects the content and opinions expressed on X and should not be considered a representative sample of the general population.
### Limitations
- Data quality may vary due to the decentralized nature of collection and preprocessing.
- The dataset may contain noise, spam, or irrelevant content typical of social media platforms.
- Temporal biases may exist due to real-time collection methods.
- The dataset is limited to public tweets and does not include private accounts or direct messages.
- Not all tweets contain hashtags or URLs.
## Additional Information
### Licensing Information
The dataset is released under the MIT license. The use of this dataset is also subject to X Terms of Use.
### Citation Information
If you use this dataset in your research, please cite it as follows:
```
@misc{hshwk19832025datauniversex_dataset_2983,
title={The Data Universe Datasets: The finest collection of social media data the web has to offer},
author={hshwk1983},
year={2025},
url={https://huggingface.co/datasets/hshwk1983/x_dataset_2983},
}
```
### Contributions
To report issues or contribute to the dataset, please contact the miner or use the Bittensor Subnet 13 governance mechanisms.
## Dataset Statistics
[This section is automatically updated]
- **Total Instances:** 45361062
- **Date Range:** 2025-01-21T00:00:00Z to 2025-02-10T00:00:00Z
- **Last Updated:** 2025-02-18T19:56:18Z
### Data Distribution
- Tweets with hashtags: 49.23%
- Tweets without hashtags: 50.77%
### Top 10 Hashtags
For full statistics, please refer to the `stats.json` file in the repository.
| Rank | Topic | Total Count | Percentage |
|------|-------|-------------|-------------|
| 1 | NULL | 23030513 | 50.77% |
| 2 | #riyadh | 389599 | 0.86% |
| 3 | #zelena | 270010 | 0.60% |
| 4 | #tiktok | 216661 | 0.48% |
| 5 | #ad | 127153 | 0.28% |
| 6 | #bbb25 | 124236 | 0.27% |
| 7 | #jhope_at_galadespiècesjaunes | 107356 | 0.24% |
| 8 | #bbmzansi | 73192 | 0.16% |
| 9 | #granhermano | 70611 | 0.16% |
| 10 | #trump | 67914 | 0.15% |
## Update History
| Date | New Instances | Total Instances |
|------|---------------|-----------------|
| 2025-01-27T07:02:01Z | 2802800 | 2802800 |
| 2025-01-30T19:05:56Z | 9696380 | 12499180 |
| 2025-02-03T07:09:45Z | 10920384 | 23419564 |
| 2025-02-06T19:12:26Z | 6138868 | 29558432 |
| 2025-02-10T07:16:07Z | 8261798 | 37820230 |
| 2025-02-13T19:19:34Z | 6252880 | 44073110 |
| 2025-02-18T04:54:59Z | 640422 | 44713532 |
| 2025-02-18T19:56:18Z | 647530 | 45361062 |
|
Kallia/stock-news-summaries-finetuning | Kallia | 2025-05-04T14:11:00Z | 0 | 0 | [
"region:us"
] | [] | 2025-05-04T14:10:50Z | null | ---
dataset_info:
features:
- name: article
dtype: string
- name: summary
dtype: string
splits:
- name: train
num_bytes: 5776072.8
num_examples: 2144
- name: validation
num_bytes: 722009.1
num_examples: 268
- name: test
num_bytes: 722009.1
num_examples: 268
download_size: 4522835
dataset_size: 7220090.999999999
configs:
- config_name: default
data_files:
- split: train
path: data/train-*
- split: validation
path: data/validation-*
- split: test
path: data/test-*
---
|
alexchilton/gran-nanobody-proteins | alexchilton | 2025-05-04T14:02:07Z | 0 | 0 | [
"task_categories:text-generation",
"task_categories:graph-ml",
"license:mit",
"region:us",
"protein",
"graph-neural-network",
"adjacency-matrix",
"protein-structure",
"nanobody"
] | [
"text-generation",
"graph-ml"
] | 2025-05-04T14:02:02Z | null | ---
license: mit
task_categories:
- text-generation
- graph-ml
tags:
- protein
- graph-neural-network
- adjacency-matrix
- protein-structure
- nanobody
---
# GRAN Protein Structure Dataset
## Dataset Description
This dataset contains protein graph data for training Graph Recurrent Attention Networks (GRAN) for protein sequence and structure generation.
### Dataset Summary
- **Number of proteins:** 2965
- **Average protein length:** 121.0 residues
- **Unique amino acids:** 22
- **Source:** Nanobody protein structures
- **Created by:** alexchilton
- **Date:** 2025-05-04 16:01:38
### Dataset Structure
Each protein entry contains:
- `sequence`: Complete amino acid sequence
- `sequence_length`: Total length of the protein
- `graph_nodes`: List of graph nodes (residue indices)
- `graph_edges`: List of graph edges (connections between residues)
- `adjacency_matrix`: Binary adjacency matrix representing contacts
- `node_features`: Features for each node (Meiler features or one-hot encoded residues)
- `protein_id`: Unique identifier
### Amino Acids
Available amino acids: ALA, ARG, ASN, ASP, CYS, GLN, GLU, GLY, HIS, ILE, LEU, LYS, MET, PHE, PRO, SER, THR, TRP, TYR, UNK, VAL, X
### Usage
```python
from datasets import load_dataset
# Load the dataset
dataset = load_dataset("alexchilton/gran-nanobody-proteins")
# Access a protein
protein = dataset['train'][0]
print(f"Sequence length: {protein['sequence_length']}")
print(f"Number of graph nodes: {len(protein['graph_nodes'])}")
print(f"Adjacency matrix shape: {np.array(protein['adjacency_matrix']).shape}")
```
### Training GRAN Model
This dataset is designed for training GRAN models that:
1. Generate both protein sequences and contact adjacency matrices
2. Model proteins as graphs with nodes (residues) and edges (contacts)
3. Use node features (Meiler descriptors or one-hot encoding)
### Citation
If you use this dataset, please cite:
```
@dataset{gran_protein_structures,
title={GRAN Protein Structure Dataset},
author={Alex Chilton},
year={2025},
url={https://huggingface.co/datasets/alexchilton/gran-nanobody-proteins}
}
```
|
amekerishvili/ATCO2_full_with_ASR | amekerishvili | 2025-05-04T13:34:29Z | 0 | 0 | [
"region:us"
] | [] | 2025-05-04T12:50:29Z | null | ---
dataset_info:
features:
- name: ID
dtype: string
- name: audio_file
dtype: string
- name: start_time
dtype: float64
- name: end_time
dtype: float64
- name: airport
dtype: string
- name: channel
dtype: string
- name: frequency
dtype: string
- name: time
dtype: string
- name: waypoints
dtype: string
- name: callsigns
dtype: string
- name: ground_truth_raw
dtype: string
- name: ground_truth
dtype: string
- name: non_Eng_ground_truth
dtype: string
- name: tags
dtype: string
- name: values_tags
dtype: string
- name: commands_tags
dtype: string
- name: callsigns_tags
dtype: string
- name: unnamed_tags
dtype: string
- name: ground_truth_norm
dtype: string
- name: whisper-large-v3
dtype: string
- name: whisper-large-v3-norm
dtype: string
splits:
- name: train
num_bytes: 1671023
num_examples: 612
- name: validation
num_bytes: 386303
num_examples: 136
- name: test
num_bytes: 378628
num_examples: 129
download_size: 780857
dataset_size: 2435954
configs:
- config_name: default
data_files:
- split: validation
path: data/validation-*
- split: train
path: data/train-*
- split: test
path: data/test-*
---
|
RyanYr/ppo-dapo-qwen2.5math-7B-base-lr-mbs64_actor_matheval | RyanYr | 2025-05-04T13:32:12Z | 41 | 0 | [
"region:us"
] | [] | 2025-05-01T05:48:04Z | null | ---
dataset_info:
features:
- name: data_source
dtype: string
- name: problem
dtype: string
- name: solution
dtype: string
- name: answer
dtype: string
- name: prompt
list:
- name: content
dtype: string
- name: role
dtype: string
- name: reward_model
struct:
- name: ground_truth
dtype: string
- name: style
dtype: string
- name: responses
sequence: string
- name: gt_ans
dtype: string
- name: extracted_solution
sequence: string
- name: rm_scores
sequence: bool
- name: avg_accuracy
dtype: float64
- name: pass_accuracy
dtype: bool
- name: cons_accuracy
dtype: float64
splits:
- name: mixed.560
num_bytes: 5300357
num_examples: 1447
- name: math_eval_aime24.560
num_bytes: 3100476
num_examples: 30
- name: mixed.520
num_bytes: 5285795
num_examples: 1447
- name: math_eval_aime24.520
num_bytes: 3095444
num_examples: 30
- name: mixed.480
num_bytes: 5242604
num_examples: 1447
- name: math_eval_aime24.480
num_bytes: 2993172
num_examples: 30
- name: mixed.440
num_bytes: 5295669
num_examples: 1447
- name: math_eval_aime24.440
num_bytes: 3177680
num_examples: 30
- name: mixed.400
num_bytes: 5506782
num_examples: 1447
- name: math_eval_aime24.400
num_bytes: 3197991
num_examples: 30
- name: mixed.360
num_bytes: 5558655
num_examples: 1447
- name: math_eval_aime24.360
num_bytes: 3233767
num_examples: 30
- name: mixed.320
num_bytes: 5589724
num_examples: 1447
- name: math_eval_aime24.320
num_bytes: 3461053
num_examples: 30
- name: mixed.280
num_bytes: 5557727
num_examples: 1447
- name: math_eval_aime24.280
num_bytes: 3595586
num_examples: 30
- name: mixed.240
num_bytes: 5646899
num_examples: 1447
- name: math_eval_aime24.240
num_bytes: 3503310
num_examples: 30
- name: mixed.200
num_bytes: 5668086
num_examples: 1447
- name: math_eval_aime24.200
num_bytes: 3483421
num_examples: 30
- name: mixed.160
num_bytes: 5605211
num_examples: 1447
- name: math_eval_aime24.160
num_bytes: 3369360
num_examples: 30
- name: mixed.120
num_bytes: 5750349
num_examples: 1447
- name: math_eval_aime24.120
num_bytes: 3606737
num_examples: 30
- name: mixed.80
num_bytes: 5761201
num_examples: 1447
- name: math_eval_aime24.80
num_bytes: 3577887
num_examples: 30
- name: mixed.40
num_bytes: 5543713
num_examples: 1447
- name: math_eval_aime24.40
num_bytes: 3478202
num_examples: 30
- name: mixed.810
num_bytes: 5059616
num_examples: 1447
- name: math_eval_aime24.810
num_bytes: 3024389
num_examples: 30
- name: mixed.800
num_bytes: 5173948
num_examples: 1447
- name: math_eval_aime24.800
num_bytes: 3039992
num_examples: 30
- name: mixed.760
num_bytes: 5271342
num_examples: 1447
- name: math_eval_aime24.760
num_bytes: 3173198
num_examples: 30
- name: mixed.720
num_bytes: 5263113
num_examples: 1447
- name: math_eval_aime24.720
num_bytes: 3075709
num_examples: 30
- name: mixed.680
num_bytes: 5114494
num_examples: 1447
- name: math_eval_aime24.680
num_bytes: 3014977
num_examples: 30
- name: mixed.640
num_bytes: 5167418
num_examples: 1447
- name: math_eval_aime24.640
num_bytes: 2939843
num_examples: 30
- name: mixed.600
num_bytes: 5197076
num_examples: 1447
- name: math_eval_aime24.600
num_bytes: 3067380
num_examples: 30
- name: mixed.1080
num_bytes: 5195072
num_examples: 1447
- name: math_eval_aime24.1080
num_bytes: 2973035
num_examples: 30
- name: mixed.1040
num_bytes: 5224089
num_examples: 1447
- name: math_eval_aime24.1040
num_bytes: 3080196
num_examples: 30
- name: mixed.1000
num_bytes: 5119350
num_examples: 1447
- name: math_eval_aime24.1000
num_bytes: 2980353
num_examples: 30
- name: mixed.960
num_bytes: 5123610
num_examples: 1447
- name: math_eval_aime24.960
num_bytes: 2881442
num_examples: 30
- name: mixed.920
num_bytes: 5179595
num_examples: 1447
- name: math_eval_aime24.920
num_bytes: 3105395
num_examples: 30
- name: mixed.880
num_bytes: 5151262
num_examples: 1447
- name: math_eval_aime24.880
num_bytes: 3205808
num_examples: 30
- name: mixed.840
num_bytes: 5118019
num_examples: 1447
- name: math_eval_aime24.840
num_bytes: 2936143
num_examples: 30
download_size: 85642315
dataset_size: 239042722
configs:
- config_name: default
data_files:
- split: mixed.560
path: data/mixed.560-*
- split: math_eval_aime24.560
path: data/math_eval_aime24.560-*
- split: mixed.520
path: data/mixed.520-*
- split: math_eval_aime24.520
path: data/math_eval_aime24.520-*
- split: mixed.480
path: data/mixed.480-*
- split: math_eval_aime24.480
path: data/math_eval_aime24.480-*
- split: mixed.440
path: data/mixed.440-*
- split: math_eval_aime24.440
path: data/math_eval_aime24.440-*
- split: mixed.400
path: data/mixed.400-*
- split: math_eval_aime24.400
path: data/math_eval_aime24.400-*
- split: mixed.360
path: data/mixed.360-*
- split: math_eval_aime24.360
path: data/math_eval_aime24.360-*
- split: mixed.320
path: data/mixed.320-*
- split: math_eval_aime24.320
path: data/math_eval_aime24.320-*
- split: mixed.280
path: data/mixed.280-*
- split: math_eval_aime24.280
path: data/math_eval_aime24.280-*
- split: mixed.240
path: data/mixed.240-*
- split: math_eval_aime24.240
path: data/math_eval_aime24.240-*
- split: mixed.200
path: data/mixed.200-*
- split: math_eval_aime24.200
path: data/math_eval_aime24.200-*
- split: mixed.160
path: data/mixed.160-*
- split: math_eval_aime24.160
path: data/math_eval_aime24.160-*
- split: mixed.120
path: data/mixed.120-*
- split: math_eval_aime24.120
path: data/math_eval_aime24.120-*
- split: mixed.80
path: data/mixed.80-*
- split: math_eval_aime24.80
path: data/math_eval_aime24.80-*
- split: mixed.40
path: data/mixed.40-*
- split: math_eval_aime24.40
path: data/math_eval_aime24.40-*
- split: mixed.810
path: data/mixed.810-*
- split: math_eval_aime24.810
path: data/math_eval_aime24.810-*
- split: mixed.800
path: data/mixed.800-*
- split: math_eval_aime24.800
path: data/math_eval_aime24.800-*
- split: mixed.760
path: data/mixed.760-*
- split: math_eval_aime24.760
path: data/math_eval_aime24.760-*
- split: mixed.720
path: data/mixed.720-*
- split: math_eval_aime24.720
path: data/math_eval_aime24.720-*
- split: mixed.680
path: data/mixed.680-*
- split: math_eval_aime24.680
path: data/math_eval_aime24.680-*
- split: mixed.640
path: data/mixed.640-*
- split: math_eval_aime24.640
path: data/math_eval_aime24.640-*
- split: mixed.600
path: data/mixed.600-*
- split: math_eval_aime24.600
path: data/math_eval_aime24.600-*
- split: mixed.1080
path: data/mixed.1080-*
- split: math_eval_aime24.1080
path: data/math_eval_aime24.1080-*
- split: mixed.1040
path: data/mixed.1040-*
- split: math_eval_aime24.1040
path: data/math_eval_aime24.1040-*
- split: mixed.1000
path: data/mixed.1000-*
- split: math_eval_aime24.1000
path: data/math_eval_aime24.1000-*
- split: mixed.960
path: data/mixed.960-*
- split: math_eval_aime24.960
path: data/math_eval_aime24.960-*
- split: mixed.920
path: data/mixed.920-*
- split: math_eval_aime24.920
path: data/math_eval_aime24.920-*
- split: mixed.880
path: data/mixed.880-*
- split: math_eval_aime24.880
path: data/math_eval_aime24.880-*
- split: mixed.840
path: data/mixed.840-*
- split: math_eval_aime24.840
path: data/math_eval_aime24.840-*
---
|
HungVu2003/opt-350m_beta_1.0_alpha_0.2_num-company_2_dataset_0_for_gen_14_v2 | HungVu2003 | 2025-05-04T13:29:17Z | 0 | 0 | [
"region:us"
] | [] | 2025-05-04T13:29:15Z | null | ---
dataset_info:
features:
- name: question
dtype: string
splits:
- name: train
num_bytes: 2076736
num_examples: 13750
download_size: 1120490
dataset_size: 2076736
configs:
- config_name: default
data_files:
- split: train
path: data/train-*
---
|
FLARE-MedFM/FLARE-Task2-LaptopSeg | FLARE-MedFM | 2025-05-04T13:27:33Z | 423 | 0 | [
"license:cc-by-nc-4.0",
"region:us"
] | [] | 2025-04-17T23:32:45Z | null | ---
license: cc-by-nc-4.0
---
## FLARE Task2 Laptop Seg Dataset
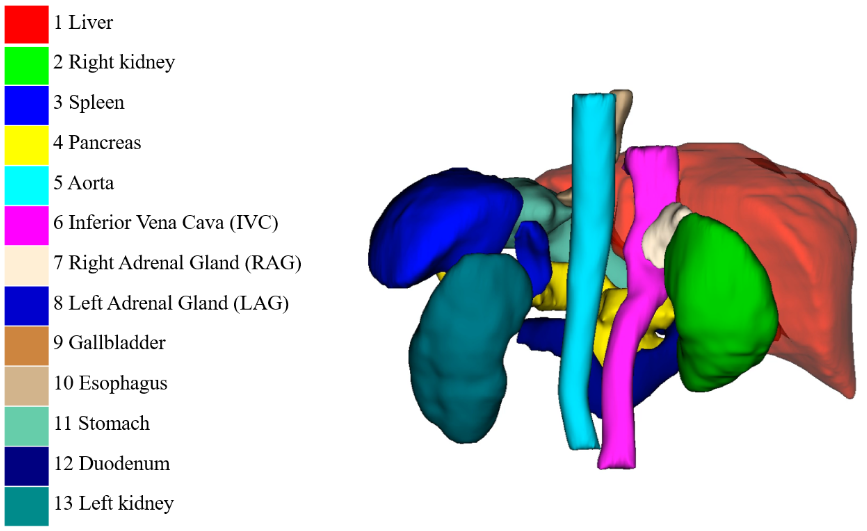
## Data Description
This is the dataset for [MICCAI FLARE 2024-2025 Task2: Abdominal CT Organ Segmentation on Laptop](https://www.codabench.org/competitions/2320/).
The training set includes 2050 cases, where 50 cases have ground-truth labels from the FLARE22 dataset,
and the remaining 2000 cases have pseudo labels generated by the FLARE 2022 winning solution.
The old validation set and testing set are merged as a new validation set with 250 cases.
For those participants who are constrained by computing resources, we also provide an unlabeled core set to develop the methods,
where 50 unlabeled CT scans are sampled from the original pseudo training set.
### Data Structure
**coreset_train_50_random:**
50 unlabeled CT scans sampled from the train_pseudo_label.
**train_gt_label:**
50 CT scans with ground-truth labels.
**train_pseudo_label:**
2000 CT scans with pseudo labels generated by the FLARE 2022 winning solution.
**validation:**
200 hidden validation set and 50 public validation set.
FLARE-Task2-LaptopSeg/
├── coreset_train_50_random/
├── train_gt_label/
│ ├── imagesTr/
│ ├── labelsTr/
│ └── dataset.json
├── train_pseudo_label/
│ ├── imagesTr/
│ ├── pseudo_label_aladdin5_flare22.7z
│ └── pseudo_label_blackbean_flare22.zip
├── validation/
│ ├── Validation-Hidden-Images/
│ ├── Validation-Public-Images/
│ └── Validation-Public-Labels/
└── README.md
### Dataset Download Instructions
Participants can download the complete dataset using the following Python script:
```python
from huggingface_hub import snapshot_download
local_dir = "./FLARE-Task2-LaptopSeg"
snapshot_download(
repo_id="FLARE-MedFM/FLARE-Task2-LaptopSeg",
repo_type="dataset",
local_dir=local_dir,
local_dir_use_symlinks=False,
resume_download=True,
)
|
hf-doc-build/doc-build | hf-doc-build | 2025-05-04T13:19:01Z | 319,590 | 9 | [
"license:mit",
"region:us"
] | [] | 2022-10-24T15:39:05Z | null | ---
license: mit
pretty_name: Generated Docs for HF
viewer: false
---
This repo contains all the docs published on https://huggingface.co/docs.
The docs are generated with https://github.com/huggingface/doc-builder.
<!-- comment to trigger webhook.= --> |
HungVu2003/opt-350m_beta_1.0_alpha_0.2_num-company_2_dataset_1_for_gen_13_v2 | HungVu2003 | 2025-05-04T12:51:41Z | 0 | 0 | [
"region:us"
] | [] | 2025-05-04T12:51:39Z | null | ---
dataset_info:
features:
- name: question
dtype: string
splits:
- name: train
num_bytes: 6681057
num_examples: 13750
download_size: 3326270
dataset_size: 6681057
configs:
- config_name: default
data_files:
- split: train
path: data/train-*
---
|
ZennyKenny/cosa-benchmark-dataset | ZennyKenny | 2025-05-04T11:59:44Z | 6 | 0 | [
"task_categories:question-answering",
"task_categories:text-generation",
"language:en",
"license:apache-2.0",
"size_categories:n<1K",
"format:parquet",
"modality:text",
"library:datasets",
"library:pandas",
"library:mlcroissant",
"library:polars",
"region:us",
"reasoning-datasets-competition"
] | [
"question-answering",
"text-generation"
] | 2025-05-04T06:49:21Z | null | ---
dataset_info:
features:
- name: index
dtype: int64
- name: code
dtype: string
- name: language
dtype: string
- name: difficulty
dtype: string
- name: vulnerability_type
dtype: string
- name: weakness_solution
dtype: string
- name: weakness_analysis
dtype: string
- name: solution_statement
dtype: string
- name: safe_code
dtype: string
splits:
- name: train
num_bytes: 1201328
num_examples: 200
download_size: 426230
dataset_size: 1201328
configs:
- config_name: default
data_files:
- split: train
path: data/train-*
license: apache-2.0
task_categories:
- question-answering
- text-generation
language:
- en
pretty_name: CoSa Benchmark Dataset
size_categories:
- n<1K
tags:
- reasoning-datasets-competition
---
<a href="https://github.com/bespokelabsai/curator/">
<img src="https://huggingface.co/datasets/bespokelabs/Bespoke-Stratos-17k/resolve/main/made_with_curator.png" alt="Made with Curator" width=200px>
</a>
# 🧠 CoSa Benchmark Dataset
## 🔍 Introduction
The **CoSa (Code Safety) Benchmark** is a curated evaluation dataset designed to measure the ability of large language models (LLMs) to detect, explain, and repair vulnerabilities in synthetic code samples. It is intended to benchmark LLMs for real-world application in code security audits, reasoning tasks, and secure code generation.
## 📦 Contents
Each row in the dataset includes:
- `code`: a code snippet (varied languages)
- `language`: language of the code (Python, JavaScript, etc.)
- `difficulty`: labeled as `easy`, `medium`, or `hard`
- `vulnerability_type`: high-level category of exploit
- `weakness_solution`: a natural language explanation of the vulnerability
- `solution_statement`: a short summary of the mitigation
- `safe_code`: a repaired version of the input code
All samples were reviewed by a human for correctness of both the vulnerability and the repaired code.
## 🛠️ How It Was Created
The dataset was generated using a multi-step pipeline built in [this notebook](https://github.com/kghamilton89/synthetic-data-generators/blob/main/reasoning-competition/code-safety-bench.ipynb). Code snippets were synthesized using LLM prompting, labeled with a vulnerability type, and then evaluated by another model for flaw detection and repair. All final `safe_code` examples were **manually reviewed for correctness**.
## 📈 Usage
An LLM may be evaluated against the CoSa Benchmark as follows:
```python
# run model on benchmark
results = []
for i, row in tqdm(df.iterrows(), total=len(df), desc="Testing model on code"):
code = row["code"]
idx = row["index"]
try:
prompt = build_test_prompt(code)
response = client.chat.completions.create(
model="gpt-4o", # Change this
messages=[{"role": "user", "content": prompt}],
temperature=0.2,
max_tokens=512
)
content = response.choices[0].message.content.strip()
explanation = ""
fixed_code = ""
for line in content.splitlines():
if line.startswith("Explanation:"):
explanation = line.replace("Explanation:", "").strip()
elif line.startswith("Fixed Code:"):
fixed_code = content.split("Fixed Code:")[1].strip()
break
results.append({
"index": idx,
"model_explanation": explanation,
"model_fix": fixed_code
})
except Exception as e:
print(f"⚠️ Error on row {i}: {e}")
results.append({
"index": idx,
"model_explanation": "ERROR",
"model_fix": ""
})
results_df = pd.DataFrame(results)
results_df.to_json("llm-eval-results.jsonl", orient="records", lines=True)
```
Then score the results:
```python
# load & score
df = pd.merge(
pd.read_json("llm-code-safety-benchmark.jsonl", lines=True),
pd.read_json("llm-eval-results.jsonl", lines=True),
on="index"
)
# Add difficulty weight
weights = {"easy": 1, "medium": 2, "hard": 3}
df["weight"] = df["difficulty"].map(weights)
# Score via sentence transformer + difflib
from sentence_transformers import SentenceTransformer
from sklearn.metrics.pairwise import cosine_similarity
import difflib
encoder = SentenceTransformer("all-MiniLM-L6-v2")
explanation_scores, code_scores, final_scores = [], [], []
for i, row in df.iterrows():
# Explanation scoring
gt_expl = row["solution_statement"]
pred_expl = row["model_explanation"]
if pred_expl.lower() == "error":
expl_score = 0
else:
emb_gt = encoder.encode(gt_expl, convert_to_tensor=True)
emb_pred = encoder.encode(pred_expl, convert_to_tensor=True)
sim = cosine_similarity([emb_gt.cpu().numpy()], [emb_pred.cpu().numpy()])[0][0]
expl_score = max(0.2, sim) if sim < 0.9 else 1.0
# Code scoring
gt_code = row["safe_code"]
pred_code = row["model_fix"]
if not pred_code.strip():
code_score = 0
else:
code_sim = difflib.SequenceMatcher(None, gt_code, pred_code).ratio()
code_score = max(0.2, code_sim) if code_sim < 0.95 else 1.0
explanation_scores.append(expl_score)
code_scores.append(code_score)
avg = (expl_score + code_score) / 2
final_scores.append(avg * row["weight"])
df["explanation_score"] = explanation_scores
df["code_score"] = code_scores
df["total_score"] = final_scores
# Normalize difficulty-adjusted score to 100
total_possible = df["weight"].sum()
difficulty_score = (df["total_score"].sum() / total_possible) * 100
print(f"🏁 Difficulty-Adjusted Score: {difficulty_score:.2f}/100")
```
## 🧪 OpenAI Model Evaluation Results
### 📌 GPT-4o
- 🧠 Explanation: **59.92**
- 🔧 Code Repair: **93.52**
- 🏁 Final Score: **75.80**
### 📌 GPT-4o Mini
- 🧠 Explanation: **61.12**
- 🔧 Code Repair: **85.55**
- 🏁 Final Score: **72.47**
### 📌 GPT-3.5 Turbo
- 🧠 Explanation: **62.12**
- 🔧 Code Repair: **79.88**
- 🏁 Final Score: **70.18**

## 🧠 Limitations & Biases
- Most vulnerabilities are intentionally simplified for LLM interpretability.
- Code snippets may not fully reflect production scenarios (e.g. frameworks, APIs).
- While `safe_code` was **manually reviewed for correctness**, adversarial testing was not performed.
- Languages are skewed toward Python, with some JavaScript, Bash, and C.
## 📚 Related Notebooks
- [CoSa Dataset Generation Notebook](https://github.com/kghamilton89/synthetic-data-generators/blob/main/reasoning-competition/code-safety-bench.ipynb)
- [GPT-4.1 Eval Notebook](https://github.com/kghamilton89/synthetic-data-generators/blob/main/reasoning-competition/cosa-evals/GPT_4.1_eval.ipynb)
- [O4 Mini Eval Notebook](https://github.com/kghamilton89/synthetic-data-generators/blob/main/reasoning-competition/cosa-evals/o4_mini_eval.ipynb)
- [O3 Eval Notebook](https://github.com/kghamilton89/synthetic-data-generators/blob/main/reasoning-competition/cosa-evals/o3_eval.ipynb)
## ❤️ These Builders Love CoSa
 |
eduagarcia/corpus-carolina-parquet | eduagarcia | 2025-05-04T11:37:49Z | 0 | 0 | [
"region:us"
] | [] | 2025-05-04T11:19:36Z | null | ---
dataset_info:
features:
- name: meta
dtype: string
- name: text
dtype: string
splits:
- name: corpus
num_bytes: 14303585243
num_examples: 2108999
download_size: 4948772195
dataset_size: 14303585243
configs:
- config_name: default
data_files:
- split: corpus
path: data/corpus-*
---
|
HungVu2003/opt-350m_beta_1.0_alpha_0.2_num-company_2_dataset_1_for_gen_12_v2 | HungVu2003 | 2025-05-04T11:15:44Z | 0 | 0 | [
"size_categories:10K<n<100K",
"format:parquet",
"modality:text",
"library:datasets",
"library:pandas",
"library:mlcroissant",
"library:polars",
"region:us"
] | [] | 2025-05-04T11:15:42Z | null | ---
dataset_info:
features:
- name: question
dtype: string
splits:
- name: train
num_bytes: 6695843
num_examples: 13750
download_size: 3357646
dataset_size: 6695843
configs:
- config_name: default
data_files:
- split: train
path: data/train-*
---
|
AndreaBorghesi/Unfair_Inequality_Education | AndreaBorghesi | 2025-05-04T11:04:51Z | 0 | 0 | [
"region:us"
] | [] | 2025-05-04T10:52:02Z | null | ---
configs:
- config_name: default
data_files:
- split: data
path: "dataset.csv"
- split: mask
path: "missing_mask.csv"
---
This is a novel benchmark specifically designed for AI fairness research in education. It can be used for challenging tasks aimed at improving students' performance and reducing dropout rates, which are also discussed in the paper to emphasize significant research directions. By prioritizing fairness, this benchmark aims to foster the development of bias-free AI solutions, promoting equal educational access and outcomes for all students.
This repository only contains the outcome of the benchmarking activity, that is, the final dataset (```dataset.csv```) and the he mask for dealing with missing values (```missing_mask.csv```).
For those interested in all the processing details (masks, data obtained at the various pre-processing stages, the actual code) we refer to the following link: https://zenodo.org/records/11171863 |
Yuyeong/rw_pubmed_nbw_6_mask_public | Yuyeong | 2025-05-04T10:58:11Z | 0 | 0 | [
"size_categories:100K<n<1M",
"format:parquet",
"modality:tabular",
"modality:text",
"library:datasets",
"library:pandas",
"library:mlcroissant",
"library:polars",
"region:us"
] | [] | 2025-05-04T10:57:56Z | null | ---
dataset_info:
features:
- name: text
dtype: string
- name: label
dtype:
class_label:
names:
'0': '0'
'1': '1'
'2': '2'
- name: group_idx
dtype: int64
- name: node_idx
dtype: int64
splits:
- name: train
num_bytes: 2119487.563016686
num_examples: 6000
- name: validation
num_bytes: 17662396.358472385
num_examples: 50000
- name: test
num_bytes: 35324792.71694477
num_examples: 100000
download_size: 22984902
dataset_size: 55106676.638433844
---
# Dataset Card for "rw_pubmed_nbw_6_mask_public"
[More Information needed](https://github.com/huggingface/datasets/blob/main/CONTRIBUTING.md#how-to-contribute-to-the-dataset-cards) |
Yuyeong/rw_pubmed_mdlr_1_mask_public | Yuyeong | 2025-05-04T09:52:47Z | 0 | 0 | [
"size_categories:100K<n<1M",
"format:parquet",
"modality:tabular",
"modality:text",
"library:datasets",
"library:pandas",
"library:mlcroissant",
"library:polars",
"region:us"
] | [] | 2025-05-04T09:52:20Z | null | ---
dataset_info:
features:
- name: text
dtype: string
- name: label
dtype:
class_label:
names:
'0': '0'
'1': '1'
'2': '2'
- name: group_idx
dtype: int64
- name: node_idx
dtype: int64
splits:
- name: train
num_bytes: 9971886.792108333
num_examples: 6000
- name: validation
num_bytes: 83099056.60090278
num_examples: 50000
- name: test
num_bytes: 166198113.20180556
num_examples: 100000
download_size: 135201790
dataset_size: 259269056.59481668
---
# Dataset Card for "rw_pubmed_mdlr_1_mask_public"
[More Information needed](https://github.com/huggingface/datasets/blob/main/CONTRIBUTING.md#how-to-contribute-to-the-dataset-cards) |
Yuyeong/rw_pubmed_standard_2_mask_public | Yuyeong | 2025-05-04T09:05:49Z | 0 | 0 | [
"size_categories:100K<n<1M",
"format:parquet",
"modality:tabular",
"modality:text",
"library:datasets",
"library:pandas",
"library:mlcroissant",
"library:polars",
"region:us"
] | [] | 2025-05-04T09:05:21Z | null | ---
dataset_info:
features:
- name: text
dtype: string
- name: label
dtype:
class_label:
names:
'0': '0'
'1': '1'
'2': '2'
- name: group_idx
dtype: int64
- name: node_idx
dtype: int64
splits:
- name: train
num_bytes: 9971747.964700513
num_examples: 6000
- name: validation
num_bytes: 83097899.7058376
num_examples: 50000
- name: test
num_bytes: 166195799.4116752
num_examples: 100000
download_size: 162469519
dataset_size: 259265447.08221334
---
# Dataset Card for "rw_pubmed_standard_2_mask_public"
[More Information needed](https://github.com/huggingface/datasets/blob/main/CONTRIBUTING.md#how-to-contribute-to-the-dataset-cards) |
Yuyeong/rw_cora_standard_1_mask_public | Yuyeong | 2025-05-04T08:27:07Z | 0 | 0 | [
"size_categories:100K<n<1M",
"format:parquet",
"modality:tabular",
"modality:text",
"library:datasets",
"library:pandas",
"library:mlcroissant",
"library:polars",
"region:us"
] | [] | 2025-05-04T08:26:40Z | null | ---
dataset_info:
features:
- name: text
dtype: string
- name: label
dtype:
class_label:
names:
'0': '0'
'1': '1'
'2': '2'
'3': '3'
'4': '4'
'5': '5'
'6': '6'
- name: group_idx
dtype: int64
- name: node_idx
dtype: int64
splits:
- name: train
num_bytes: 20028522.88774003
num_examples: 14000
- name: validation
num_bytes: 71530438.88478582
num_examples: 50000
- name: test
num_bytes: 143060877.76957163
num_examples: 100000
download_size: 111756477
dataset_size: 234619839.54209748
---
# Dataset Card for "rw_cora_standard_1_mask_public"
[More Information needed](https://github.com/huggingface/datasets/blob/main/CONTRIBUTING.md#how-to-contribute-to-the-dataset-cards) |
putdanil/inter1or | putdanil | 2025-05-04T08:02:55Z | 0 | 0 | [
"region:us"
] | [] | 2025-05-04T07:49:51Z | null | ---
dataset_info:
features:
- name: image_path
dtype: image
- name: caption
dtype: string
splits:
- name: train
num_bytes: 18411049934.656
num_examples: 4438
download_size: 17149567703
dataset_size: 18411049934.656
configs:
- config_name: default
data_files:
- split: train
path: data/train-*
---
|
alchemistyzz/mathvision_test | alchemistyzz | 2025-05-04T07:36:11Z | 0 | 0 | [
"license:apache-2.0",
"size_categories:n<1K",
"format:parquet",
"modality:text",
"library:datasets",
"library:pandas",
"library:mlcroissant",
"library:polars",
"region:us"
] | [] | 2025-05-03T10:04:54Z | null | ---
license: apache-2.0
---
|
HungVu2003/opt-350m_beta_0.0_alpha_0.2_num-company_2_dataset_1_for_gen_6_v2 | HungVu2003 | 2025-05-04T07:05:24Z | 0 | 0 | [
"region:us"
] | [] | 2025-05-04T07:05:23Z | null | ---
dataset_info:
features:
- name: question
dtype: string
splits:
- name: train
num_bytes: 2486011
num_examples: 13750
download_size: 1036048
dataset_size: 2486011
configs:
- config_name: default
data_files:
- split: train
path: data/train-*
---
|
valpy/if_multi_old_3_different_range | valpy | 2025-05-04T04:34:01Z | 0 | 0 | [
"size_categories:10K<n<100K",
"format:parquet",
"modality:text",
"library:datasets",
"library:pandas",
"library:mlcroissant",
"library:polars",
"region:us"
] | [] | 2025-05-04T04:33:06Z | null | ---
dataset_info:
features:
- name: messages
list:
- name: content
dtype: string
- name: role
dtype: string
- name: ground_truth
dtype: string
- name: dataset
dtype: string
- name: constraint_type
dtype: string
- name: constraint
dtype: string
splits:
- name: train
num_bytes: 90775411
num_examples: 57306
download_size: 41406293
dataset_size: 90775411
configs:
- config_name: default
data_files:
- split: train
path: data/train-*
---
|
ma921/golden-hh-tokenized-gpt2_noise0 | ma921 | 2025-05-04T03:27:26Z | 0 | 0 | [
"size_categories:10K<n<100K",
"format:parquet",
"library:datasets",
"library:pandas",
"library:mlcroissant",
"library:polars",
"region:us"
] | [] | 2025-05-04T03:27:22Z | null | ---
dataset_info:
features:
- name: sft_input_ids
sequence: int64
- name: pos_input_ids
sequence: int64
- name: neg_input_ids
sequence: int64
splits:
- name: train
num_bytes: 17534576
num_examples: 12066
download_size: 4349810
dataset_size: 17534576
configs:
- config_name: default
data_files:
- split: train
path: data/train-*
---
|
mlfoundations-dev/mix_avg_all | mlfoundations-dev | 2025-05-04T02:47:06Z | 0 | 0 | [
"size_categories:10K<n<100K",
"format:parquet",
"modality:tabular",
"modality:text",
"library:datasets",
"library:dask",
"library:mlcroissant",
"library:polars",
"region:us"
] | [] | 2025-05-04T02:33:43Z | null | ---
dataset_info:
features:
- name: instruction_seed
dtype: string
- name: _source
dtype: string
- name: gpt41_mini_response
dtype: string
- name: __original_row_idx
dtype: int64
- name: length
dtype: int64
- name: domain
dtype: string
- name: r1_response
dtype: string
- name: r1_reasoning_content
dtype: string
- name: extract_solution
dtype: string
- name: url
dtype: string
- name: filename
dtype: string
- name: success
dtype: bool
- name: page_count
dtype: int64
- name: page_number
dtype: int64
- name: question_choices_solutions
dtype: string
- name: extracted_question
dtype: string
- name: extracted_answer_choices
sequence: string
- name: matched_solution
dtype: string
- name: qa_validation_outputs
dtype: bool
- name: classifier_reasoning
dtype: string
- name: is_organic_chemistry
dtype: bool
- name: ms_id
dtype: int64
- name: _science_reasoning
sequence: string
- name: _science_deepseek_solution
sequence: string
- name: _science_final_reasoning_trace
sequence: string
- name: _majority_responses
sequence: string
- name: verified_final_reasoning_trace
dtype: string
- name: conversations
list:
- name: from
dtype: string
- name: value
dtype: string
- name: difficulty
dtype: int64
- name: difficulty_reasoning
dtype: string
- name: id
dtype: string
- name: output
dtype: string
- name: source
dtype: string
- name: license
dtype: string
- name: dataset
dtype: string
- name: split
dtype: string
- name: solution
dtype: string
- name: _code_reasoning
dtype: string
- name: _code_deepseek_solution
dtype: string
- name: _code_final_reasoning_trace
dtype: string
- name: messages
list:
- name: content
dtype: string
- name: role
dtype: string
- name: response_seed
dtype: string
- name: _math_reasoning
dtype: string
- name: _math_deepseek_solution
dtype: string
- name: _math_final_reasoning_trace
dtype: string
splits:
- name: train
num_bytes: 36642395331.0
num_examples: 94797
download_size: 14773154614
dataset_size: 36642395331.0
configs:
- config_name: default
data_files:
- split: train
path: data/train-*
---
|
marcuscedricridia/OpenMathInstruct-1-1000-processed | marcuscedricridia | 2025-05-04T02:46:57Z | 0 | 0 | [
"size_categories:1K<n<10K",
"format:parquet",
"modality:text",
"library:datasets",
"library:pandas",
"library:mlcroissant",
"library:polars",
"region:us"
] | [] | 2025-05-04T02:46:56Z | null | ---
dataset_info:
features:
- name: question
dtype: string
- name: generated_solution
dtype: string
splits:
- name: train
num_bytes: 586120.6902226892
num_examples: 1000
download_size: 293455
dataset_size: 586120.6902226892
configs:
- config_name: default
data_files:
- split: train
path: data/train-*
---
|
prithivMLmods/Openpdf-Analysis-Recognition | prithivMLmods | 2025-05-04T02:39:38Z | 0 | 0 | [
"task_categories:image-to-text",
"language:en",
"license:apache-2.0",
"size_categories:1K<n<10K",
"format:imagefolder",
"modality:image",
"library:datasets",
"library:mlcroissant",
"region:us",
"pdf",
"ocr",
"document",
"code"
] | [
"image-to-text"
] | 2025-05-03T08:20:35Z | null | ---
license: apache-2.0
task_categories:
- image-to-text
language:
- en
tags:
- pdf
- ocr
- document
- code
size_categories:
- 1K<n<10K
---
# Openpdf-Analysis-Recognition
The **Openpdf-Analysis-Recognition** dataset is curated for tasks related to image-to-text recognition, particularly for scanned document images and OCR (Optical Character Recognition) use cases. It contains over 6,900 images in a structured `imagefolder` format suitable for training models on document parsing, PDF image understanding, and layout/text extraction tasks.
| **Attribute** | **Value** |
|---------------|------------------------|
| Task | Image-to-Text |
| Modality | Image |
| Format | ImageFolder |
| Language | English |
| License | Apache 2.0 |
| Size | 1K - 10K samples |
| Split | train (6,910 samples) |
### Key Features
* Contains **6.91k** training samples of document-style images.
* Each sample is an **image**, with no associated text or label (raw OCR input).
* Dataset is auto-converted to **Parquet** format by Hugging Face for efficient streaming and processing.
* Suitable for OCR research, PDF document parsing, and code/text recognition tasks.
## Usage
You can load the dataset using the Hugging Face `datasets` library:
```python
from datasets import load_dataset
dataset = load_dataset("prithivMLmods/Openpdf-Analysis-Recognition")
```
## File Size
* **Total download size**: \~2.72 GB
* **Auto-converted Parquet size**: \~2.71 GB
## License
This dataset is released under the [Apache 2.0 License](https://www.apache.org/licenses/LICENSE-2.0). |
BornSaint/D33_590d | BornSaint | 2025-05-04T01:44:08Z | 0 | 0 | [
"size_categories:n<1K",
"format:parquet",
"modality:text",
"library:datasets",
"library:pandas",
"library:mlcroissant",
"library:polars",
"region:us"
] | [] | 2025-05-04T01:44:05Z | null | ---
dataset_info:
features:
- name: conversation
list:
- name: content
dtype: string
- name: role
dtype: string
splits:
- name: train
num_bytes: 4301338
num_examples: 590
download_size: 1871609
dataset_size: 4301338
configs:
- config_name: default
data_files:
- split: train
path: data/train-*
---
|
ParkSY/data_nerf_more_concept_org_style_anything_depthmap_normalmap | ParkSY | 2025-05-04T01:06:46Z | 0 | 0 | [
"size_categories:n<1K",
"format:parquet",
"modality:text",
"library:datasets",
"library:pandas",
"library:mlcroissant",
"library:polars",
"region:us"
] | [] | 2025-05-04T01:06:40Z | null | ---
dataset_info:
features:
- name: input_image
dtype: string
- name: edit_prompt
dtype: string
- name: edited_image
dtype: string
- name: label
dtype: int64
- name: depthmap
dtype: string
- name: normal_map
dtype: string
splits:
- name: train
num_bytes: 380305
num_examples: 819
download_size: 36116
dataset_size: 380305
configs:
- config_name: default
data_files:
- split: train
path: data/train-*
---
|
dgambettaphd/D_llm2_gen1_WXS_doc1000_synt64_lr1e-04_acm_FRESH | dgambettaphd | 2025-05-04T01:04:41Z | 0 | 0 | [
"size_categories:10K<n<100K",
"format:parquet",
"modality:tabular",
"modality:text",
"library:datasets",
"library:pandas",
"library:mlcroissant",
"library:polars",
"region:us"
] | [] | 2025-05-04T01:04:18Z | null | ---
dataset_info:
features:
- name: id_doc
dtype: int64
- name: text
dtype: string
- name: dataset
dtype: string
- name: gen
dtype: int64
- name: synt
dtype: int64
- name: MPP
dtype: float64
splits:
- name: train
num_bytes: 9773483
num_examples: 17000
download_size: 5870471
dataset_size: 9773483
configs:
- config_name: default
data_files:
- split: train
path: data/train-*
---
|
psyonp/ttr_response_2 | psyonp | 2025-05-04T00:06:55Z | 0 | 0 | [
"size_categories:1K<n<10K",
"format:parquet",
"modality:tabular",
"modality:text",
"library:datasets",
"library:pandas",
"library:mlcroissant",
"library:polars",
"region:us"
] | [] | 2025-05-04T00:06:54Z | null | ---
dataset_info:
features:
- name: prompt
dtype: string
- name: response
dtype: string
- name: num_tokens_question
dtype: int64
- name: num_tokens_response
dtype: int64
- name: semantic_similarity
dtype: float64
- name: sentiment_question
dtype: float64
- name: sentiment_response
dtype: float64
- name: readability_question
dtype: float64
- name: readability_response
dtype: float64
- name: ttr_question
dtype: float64
- name: ttr_response
dtype: float64
- name: toxicity_question
dtype: float64
- name: toxicity_response
dtype: float64
- name: euclidean_distance
dtype: float64
- name: kl_divergence
dtype: float64
splits:
- name: train
num_bytes: 8059758
num_examples: 3995
download_size: 4768225
dataset_size: 8059758
configs:
- config_name: default
data_files:
- split: train
path: data/train-*
---
|
HungVu2003/opt-350m_beta_1.0_alpha_0.6_num-company_3_dataset_2_for_gen_16 | HungVu2003 | 2025-05-03T23:58:56Z | 0 | 0 | [
"size_categories:10K<n<100K",
"format:parquet",
"modality:text",
"library:datasets",
"library:pandas",
"library:mlcroissant",
"library:polars",
"region:us"
] | [] | 2025-05-03T23:58:55Z | null | ---
dataset_info:
features:
- name: question
dtype: string
splits:
- name: train
num_bytes: 5074613
num_examples: 12500
download_size: 1290108
dataset_size: 5074613
configs:
- config_name: default
data_files:
- split: train
path: data/train-*
---
|
Bakovic/chatbot_medical_diabetique | Bakovic | 2025-05-03T23:34:34Z | 0 | 0 | [
"license:intel-research",
"region:us"
] | [] | 2025-05-03T23:32:29Z | null | ---
license: intel-research
---
|
AdoCleanCode/Youtube8M_real_train_data_v4_0.8 | AdoCleanCode | 2025-05-03T23:26:47Z | 0 | 0 | [
"size_categories:100K<n<1M",
"format:parquet",
"modality:tabular",
"modality:text",
"library:datasets",
"library:pandas",
"library:mlcroissant",
"library:polars",
"region:us"
] | [] | 2025-05-03T21:27:22Z | null | ---
dataset_info:
features:
- name: 'Unnamed: 0'
dtype: int64
- name: caption
dtype: string
- name: coarse_label
dtype: string
- name: __index_level_0__
dtype: int64
splits:
- name: train
num_bytes: 55669638
num_examples: 205336
download_size: 16574453
dataset_size: 55669638
configs:
- config_name: default
data_files:
- split: train
path: data/train-*
---
|
jysim/koch_test | jysim | 2025-05-03T22:56:27Z | 0 | 0 | [
"task_categories:robotics",
"license:apache-2.0",
"size_categories:1K<n<10K",
"format:parquet",
"modality:tabular",
"modality:timeseries",
"modality:video",
"library:datasets",
"library:dask",
"library:mlcroissant",
"library:polars",
"region:us",
"LeRobot",
"tutorial"
] | [
"robotics"
] | 2025-05-03T22:56:19Z | null | ---
license: apache-2.0
task_categories:
- robotics
tags:
- LeRobot
- tutorial
configs:
- config_name: default
data_files: data/*/*.parquet
---
This dataset was created using [LeRobot](https://github.com/huggingface/lerobot).
## Dataset Description
- **Homepage:** [More Information Needed]
- **Paper:** [More Information Needed]
- **License:** apache-2.0
## Dataset Structure
[meta/info.json](meta/info.json):
```json
{
"codebase_version": "v2.1",
"robot_type": "koch",
"total_episodes": 2,
"total_frames": 1159,
"total_tasks": 1,
"total_videos": 4,
"total_chunks": 1,
"chunks_size": 1000,
"fps": 30,
"splits": {
"train": "0:2"
},
"data_path": "data/chunk-{episode_chunk:03d}/episode_{episode_index:06d}.parquet",
"video_path": "videos/chunk-{episode_chunk:03d}/{video_key}/episode_{episode_index:06d}.mp4",
"features": {
"action": {
"dtype": "float32",
"shape": [
6
],
"names": [
"main_shoulder_pan",
"main_shoulder_lift",
"main_elbow_flex",
"main_wrist_flex",
"main_wrist_roll",
"main_gripper"
]
},
"observation.state": {
"dtype": "float32",
"shape": [
6
],
"names": [
"main_shoulder_pan",
"main_shoulder_lift",
"main_elbow_flex",
"main_wrist_flex",
"main_wrist_roll",
"main_gripper"
]
},
"observation.images.webcam": {
"dtype": "video",
"shape": [
480,
640,
3
],
"names": [
"height",
"width",
"channels"
],
"info": {
"video.height": 480,
"video.width": 640,
"video.codec": "av1",
"video.pix_fmt": "yuv420p",
"video.is_depth_map": false,
"video.fps": 30,
"video.channels": 3,
"has_audio": false
}
},
"observation.images.phone": {
"dtype": "video",
"shape": [
480,
640,
3
],
"names": [
"height",
"width",
"channels"
],
"info": {
"video.height": 480,
"video.width": 640,
"video.codec": "av1",
"video.pix_fmt": "yuv420p",
"video.is_depth_map": false,
"video.fps": 30,
"video.channels": 3,
"has_audio": false
}
},
"timestamp": {
"dtype": "float32",
"shape": [
1
],
"names": null
},
"frame_index": {
"dtype": "int64",
"shape": [
1
],
"names": null
},
"episode_index": {
"dtype": "int64",
"shape": [
1
],
"names": null
},
"index": {
"dtype": "int64",
"shape": [
1
],
"names": null
},
"task_index": {
"dtype": "int64",
"shape": [
1
],
"names": null
}
}
}
```
## Citation
**BibTeX:**
```bibtex
[More Information Needed]
``` |
ymroddi/langa_train | ymroddi | 2025-05-03T22:40:33Z | 0 | 0 | [
"size_categories:10K<n<100K",
"format:parquet",
"modality:text",
"library:datasets",
"library:pandas",
"library:mlcroissant",
"library:polars",
"region:us"
] | [] | 2025-05-03T18:52:20Z | null | ---
dataset_info:
features:
- name: conversations
list:
- name: content
dtype: string
- name: role
dtype: string
splits:
- name: train
num_bytes: 49574181
num_examples: 42328
- name: test
num_bytes: 15226989
num_examples: 11207
download_size: 26250984
dataset_size: 64801170
configs:
- config_name: default
data_files:
- split: train
path: data/train-*
- split: test
path: data/test-*
---
|
kothasuhas/llp-gold-37m-1.5m_clip0.256_T1.0 | kothasuhas | 2025-05-03T22:39:09Z | 0 | 0 | [
"size_categories:1M<n<10M",
"format:parquet",
"modality:tabular",
"modality:text",
"library:datasets",
"library:dask",
"library:mlcroissant",
"library:polars",
"region:us"
] | [] | 2025-05-03T22:35:25Z | null | ---
dataset_info:
features:
- name: text
dtype: string
- name: p_log_probs
dtype: float32
- name: q_log_probs
dtype: float32
- name: num_tokens
dtype: float32
- name: log_weight
dtype: float64
splits:
- name: train
num_bytes: 3605804917.0
num_examples: 1500000
download_size: 197960374
dataset_size: 3605804917.0
configs:
- config_name: default
data_files:
- split: train
path: data/train-*
---
|
AJ97/dd | AJ97 | 2025-05-03T22:31:37Z | 0 | 0 | [
"license:apache-2.0",
"region:us"
] | [] | 2025-05-03T22:31:37Z | null | ---
license: apache-2.0
---
|
kothasuhas/llama-3b-gold-ctx16 | kothasuhas | 2025-05-03T22:14:39Z | 0 | 0 | [
"size_categories:1M<n<10M",
"format:parquet",
"modality:text",
"library:datasets",
"library:pandas",
"library:mlcroissant",
"library:polars",
"region:us"
] | [] | 2025-05-03T22:14:29Z | null | ---
dataset_info:
features:
- name: text
dtype: string
splits:
- name: train
num_bytes: 217731235
num_examples: 3200000
download_size: 159922823
dataset_size: 217731235
configs:
- config_name: default
data_files:
- split: train
path: data/train-*
---
|
mlfoundations-dev/e1_science_longest_qwq | mlfoundations-dev | 2025-05-03T21:26:30Z | 0 | 0 | [
"size_categories:10K<n<100K",
"format:parquet",
"modality:tabular",
"modality:text",
"library:datasets",
"library:dask",
"library:mlcroissant",
"library:polars",
"region:us"
] | [] | 2025-05-03T21:22:55Z | null | ---
dataset_info:
features:
- name: instruction_seed
dtype: string
- name: _source
dtype: string
- name: gpt41_mini_response
dtype: string
- name: __original_row_idx
dtype: int64
- name: length
dtype: int64
- name: domain
dtype: string
- name: r1_response
dtype: string
- name: r1_reasoning_content
dtype: string
- name: extract_solution
dtype: string
- name: url
dtype: string
- name: filename
dtype: string
- name: success
dtype: bool
- name: page_count
dtype: int64
- name: page_number
dtype: int64
- name: question_choices_solutions
dtype: string
- name: extracted_question
dtype: string
- name: extracted_answer_choices
sequence: string
- name: matched_solution
dtype: string
- name: qa_validation_outputs
dtype: bool
- name: classifier_reasoning
dtype: string
- name: is_organic_chemistry
dtype: bool
- name: ms_id
dtype: int64
- name: final_reasoning_trace
sequence: string
- name: _majority_responses
sequence: string
- name: verified_final_reasoning_trace
dtype: string
- name: conversations
list:
- name: from
dtype: string
- name: value
dtype: string
splits:
- name: train
num_bytes: 12484376307
num_examples: 31600
download_size: 5528947931
dataset_size: 12484376307
configs:
- config_name: default
data_files:
- split: train
path: data/train-*
---
|
HungVu2003/opt-350m_beta_1.0_alpha_0.0_num-company_2_dataset_1_for_gen_18_v2 | HungVu2003 | 2025-05-03T20:33:24Z | 0 | 0 | [
"size_categories:10K<n<100K",
"format:parquet",
"modality:text",
"library:datasets",
"library:pandas",
"library:mlcroissant",
"library:polars",
"region:us"
] | [] | 2025-05-03T20:33:23Z | null | ---
dataset_info:
features:
- name: question
dtype: string
splits:
- name: train
num_bytes: 6589847
num_examples: 12500
download_size: 3363829
dataset_size: 6589847
configs:
- config_name: default
data_files:
- split: train
path: data/train-*
---
|
HungVu2003/opt-350m_beta_1.0_alpha_0.0_num-company_2_dataset_0_for_gen_18_v2 | HungVu2003 | 2025-05-03T20:33:22Z | 0 | 0 | [
"size_categories:10K<n<100K",
"format:parquet",
"modality:text",
"library:datasets",
"library:pandas",
"library:mlcroissant",
"library:polars",
"region:us"
] | [] | 2025-05-03T20:33:21Z | null | ---
dataset_info:
features:
- name: question
dtype: string
splits:
- name: train
num_bytes: 1148944
num_examples: 12500
download_size: 699103
dataset_size: 1148944
configs:
- config_name: default
data_files:
- split: train
path: data/train-*
---
|
HungVu2003/opt-350m_beta_1.0_alpha_0.0_num-company_2_dataset_1_for_gen_16_v2 | HungVu2003 | 2025-05-03T20:29:36Z | 0 | 0 | [
"size_categories:10K<n<100K",
"format:parquet",
"modality:text",
"library:datasets",
"library:pandas",
"library:mlcroissant",
"library:polars",
"region:us"
] | [] | 2025-05-03T20:29:35Z | null | ---
dataset_info:
features:
- name: question
dtype: string
splits:
- name: train
num_bytes: 6614598
num_examples: 12500
download_size: 3383351
dataset_size: 6614598
configs:
- config_name: default
data_files:
- split: train
path: data/train-*
---
|
anonymousEcaiHateLLM/Hate.2_label_eval_data | anonymousEcaiHateLLM | 2025-05-03T20:27:23Z | 0 | 0 | [
"size_categories:10K<n<100K",
"format:parquet",
"modality:text",
"library:datasets",
"library:pandas",
"library:mlcroissant",
"library:polars",
"region:us"
] | [] | 2025-05-03T20:27:20Z | null | ---
dataset_info:
features:
- name: text
dtype: string
- name: ds
dtype: string
- name: language
dtype: string
- name: label_id
dtype: int64
splits:
- name: group_1
num_bytes: 790678
num_examples: 5481
- name: group_2
num_bytes: 834653
num_examples: 5700
download_size: 955652
dataset_size: 1625331
configs:
- config_name: default
data_files:
- split: group_1
path: data/group_1-*
- split: group_2
path: data/group_2-*
---
|
HungVu2003/opt-350m_beta_1.0_alpha_0.0_num-company_2_dataset_0_for_gen_6_v2 | HungVu2003 | 2025-05-03T20:09:58Z | 0 | 0 | [
"size_categories:10K<n<100K",
"format:parquet",
"modality:text",
"library:datasets",
"library:pandas",
"library:mlcroissant",
"library:polars",
"region:us"
] | [] | 2025-05-03T20:09:56Z | null | ---
dataset_info:
features:
- name: question
dtype: string
splits:
- name: train
num_bytes: 1151427
num_examples: 12500
download_size: 701524
dataset_size: 1151427
configs:
- config_name: default
data_files:
- split: train
path: data/train-*
---
|
Bretagne/WikiMatrix_br_fr | Bretagne | 2025-05-03T19:49:04Z | 15 | 0 | [
"task_categories:translation",
"multilinguality:multilingual",
"language:br",
"language:fra",
"size_categories:10K<n<100K",
"format:parquet",
"modality:text",
"library:datasets",
"library:pandas",
"library:mlcroissant",
"library:polars",
"arxiv:1907.05791",
"region:us"
] | [
"translation"
] | 2024-10-29T15:47:02Z | null | ---
dataset_info:
features:
- name: br
dtype: string
- name: fr
dtype: string
splits:
- name: train
num_bytes: 4099920
num_examples: 23893
download_size: 3022709
dataset_size: 4099920
configs:
- config_name: default
data_files:
- split: train
path: data/train-*
task_categories:
- translation
language:
- br
- fra
multilinguality:
- multilingual
---
## Description
Paires breton/français du jeu de données WikiMatrix disponible sur [OPUS](https://opus.nlpl.eu/results/br&fr/corpus-result-table).
**⚠ Attention ⚠** : il y a des problèmes d'alignement. Ce jeu de données n'est donc pas utilisbale tel quel et un post-processing serait à effectuer.
## Citations
#### WikiMatrix
```
@misc{schwenk2019wikimatrixmining135mparallel,
title={WikiMatrix: Mining 135M Parallel Sentences in 1620 Language Pairs from Wikipedia},
author={Holger Schwenk and Vishrav Chaudhary and Shuo Sun and Hongyu Gong and Francisco Guzmán},
year={2019},
eprint={1907.05791},
archivePrefix={arXiv},
primaryClass={cs.CL},
url={https://arxiv.org/abs/1907.05791},
}
```
#### OPUS
```
@inbook{4992de1b5fb34f3e9691772606b36edf,
title = "News from OPUS - A Collection of Multilingual Parallel Corpora with Tools and Interfaces",
author = "J{\"o}rg Tiedemann",
year = "2009",
language = "odefinierat/ok{\"a}nt",
volume = "V",
pages = "237--248",
editor = "N. Nicolov and K. Bontcheva and G. Angelova and R. Mitkov",
booktitle = "Recent Advances in Natural Language Processing",
}
```
|
Bretagne/wikiann_br | Bretagne | 2025-05-03T19:40:12Z | 21 | 0 | [
"task_categories:token-classification",
"language:br",
"size_categories:1K<n<10K",
"format:parquet",
"modality:text",
"library:datasets",
"library:pandas",
"library:mlcroissant",
"library:polars",
"region:us"
] | [
"token-classification"
] | 2024-10-16T21:21:02Z | null | ---
dataset_info:
features:
- name: tokens
sequence: string
- name: ner_tags
sequence: int64
splits:
- name: train
num_bytes: 127019
num_examples: 915
- name: validation
num_bytes: 121393
num_examples: 946
- name: test
num_bytes: 130972
num_examples: 952
download_size: 120493
dataset_size: 379384
configs:
- config_name: default
data_files:
- split: train
path: data/train-*
- split: validation
path: data/validation-*
- split: test
path: data/test-*
language: br
task_categories:
- token-classification
---
### Description
Version nettoyée de [WikiAnn](https://huggingface.co/datasets/tner/wikiann).
En effet, la version originale contenait des leaks et des duplications.
De 1000 effectifs par split, la nouvelle répartition devient alors la suivante :
```
DatasetDict({
train: Dataset({
features: ['tokens', 'ner_tags'],
num_rows: 915
})
validation: Dataset({
features: ['tokens', 'ner_tags'],
num_rows: 946
})
test: Dataset({
features: ['tokens', 'ner_tags'],
num_rows: 952
})
})
```
### Label ID
Le dictionnaire label2id est disponible [ici](https://huggingface.co/datasets/tner/wikiann/raw/main/dataset/label.json).
```python
{
"B-LOC": 0,
"B-ORG": 1,
"B-PER": 2,
"I-LOC": 3,
"I-ORG": 4,
"I-PER": 5,
"O": 6
}
``` |
TheRealPilot638/Olmo-1B-0724-best_of_4_H200 | TheRealPilot638 | 2025-05-03T19:24:26Z | 3 | 0 | [
"size_categories:1K<n<10K",
"format:parquet",
"modality:tabular",
"modality:text",
"library:datasets",
"library:pandas",
"library:mlcroissant",
"library:polars",
"region:us"
] | [] | 2025-04-28T17:28:12Z | null | ---
dataset_info:
- config_name: HuggingFaceH4_MATH-500--T-0.8--top_p-1.0--n-4--seed-0--agg_strategy-last
features:
- name: problem
dtype: string
- name: solution
dtype: string
- name: answer
dtype: string
- name: subject
dtype: string
- name: level
dtype: int64
- name: unique_id
dtype: string
- name: completions
sequence: string
- name: scores
sequence:
sequence: float64
- name: pred
dtype: string
- name: completion_tokens
sequence: int64
- name: agg_scores
sequence: float64
- name: pred_weighted@1
dtype: string
- name: pred_maj@1
dtype: string
- name: pred_naive@1
dtype: string
- name: pred_weighted@2
dtype: string
- name: pred_maj@2
dtype: string
- name: pred_naive@2
dtype: string
- name: pred_weighted@4
dtype: string
- name: pred_maj@4
dtype: string
- name: pred_naive@4
dtype: string
splits:
- name: train
num_bytes: 12951230
num_examples: 500
download_size: 3215226
dataset_size: 12951230
- config_name: HuggingFaceH4_MATH-500--T-0.8--top_p-1.0--n-4--seed-0--agg_strategy-last--evals
features:
- name: n
dtype: int64
- name: acc_naive
dtype: float64
- name: acc_weighted
dtype: float64
- name: acc_maj
dtype: float64
splits:
- name: train
num_bytes: 32
num_examples: 1
download_size: 1961
dataset_size: 32
- config_name: HuggingFaceH4_MATH-500--T-0.8--top_p-1.0--n-4--seed-1--agg_strategy-last
features:
- name: problem
dtype: string
- name: solution
dtype: string
- name: answer
dtype: string
- name: subject
dtype: string
- name: level
dtype: int64
- name: unique_id
dtype: string
- name: completions
sequence: string
- name: scores
sequence:
sequence: float64
- name: pred
dtype: string
- name: completion_tokens
sequence: int64
- name: agg_scores
sequence: float64
- name: pred_weighted@1
dtype: string
- name: pred_maj@1
dtype: string
- name: pred_naive@1
dtype: string
- name: pred_weighted@2
dtype: string
- name: pred_maj@2
dtype: string
- name: pred_naive@2
dtype: string
- name: pred_weighted@4
dtype: string
- name: pred_maj@4
dtype: string
- name: pred_naive@4
dtype: string
splits:
- name: train
num_bytes: 12797006
num_examples: 500
download_size: 3200583
dataset_size: 12797006
- config_name: HuggingFaceH4_MATH-500--T-0.8--top_p-1.0--n-4--seed-2--agg_strategy-last
features:
- name: problem
dtype: string
- name: solution
dtype: string
- name: answer
dtype: string
- name: subject
dtype: string
- name: level
dtype: int64
- name: unique_id
dtype: string
- name: completions
sequence: string
- name: scores
sequence:
sequence: float64
- name: pred
dtype: string
- name: completion_tokens
sequence: int64
- name: agg_scores
sequence: float64
- name: pred_weighted@1
dtype: string
- name: pred_maj@1
dtype: string
- name: pred_naive@1
dtype: string
- name: pred_weighted@2
dtype: string
- name: pred_maj@2
dtype: string
- name: pred_naive@2
dtype: string
- name: pred_weighted@4
dtype: string
- name: pred_maj@4
dtype: string
- name: pred_naive@4
dtype: string
splits:
- name: train
num_bytes: 12918006
num_examples: 500
download_size: 3176928
dataset_size: 12918006
- config_name: HuggingFaceH4_MATH-500--T-0.8--top_p-1.0--n-4--seed-3--agg_strategy-last
features:
- name: problem
dtype: string
- name: solution
dtype: string
- name: answer
dtype: string
- name: subject
dtype: string
- name: level
dtype: int64
- name: unique_id
dtype: string
- name: completions
sequence: string
- name: scores
sequence:
sequence: float64
- name: pred
dtype: string
- name: completion_tokens
sequence: int64
- name: agg_scores
sequence: float64
- name: pred_weighted@1
dtype: string
- name: pred_maj@1
dtype: string
- name: pred_naive@1
dtype: string
- name: pred_weighted@2
dtype: string
- name: pred_maj@2
dtype: string
- name: pred_naive@2
dtype: string
- name: pred_weighted@4
dtype: string
- name: pred_maj@4
dtype: string
- name: pred_naive@4
dtype: string
splits:
- name: train
num_bytes: 13126345
num_examples: 500
download_size: 3242153
dataset_size: 13126345
configs:
- config_name: HuggingFaceH4_MATH-500--T-0.8--top_p-1.0--n-4--seed-0--agg_strategy-last
data_files:
- split: train
path: HuggingFaceH4_MATH-500--T-0.8--top_p-1.0--n-4--seed-0--agg_strategy-last/train-*
- config_name: HuggingFaceH4_MATH-500--T-0.8--top_p-1.0--n-4--seed-0--agg_strategy-last--evals
data_files:
- split: train
path: HuggingFaceH4_MATH-500--T-0.8--top_p-1.0--n-4--seed-0--agg_strategy-last--evals/train-*
- config_name: HuggingFaceH4_MATH-500--T-0.8--top_p-1.0--n-4--seed-1--agg_strategy-last
data_files:
- split: train
path: HuggingFaceH4_MATH-500--T-0.8--top_p-1.0--n-4--seed-1--agg_strategy-last/train-*
- config_name: HuggingFaceH4_MATH-500--T-0.8--top_p-1.0--n-4--seed-2--agg_strategy-last
data_files:
- split: train
path: HuggingFaceH4_MATH-500--T-0.8--top_p-1.0--n-4--seed-2--agg_strategy-last/train-*
- config_name: HuggingFaceH4_MATH-500--T-0.8--top_p-1.0--n-4--seed-3--agg_strategy-last
data_files:
- split: train
path: HuggingFaceH4_MATH-500--T-0.8--top_p-1.0--n-4--seed-3--agg_strategy-last/train-*
---
|
alucchi/Qwen2.5-1.5B-Instruct_n1000_e10_oadam0.0001_b16_1_a10_flash_compact_ttt_a100_s40 | alucchi | 2025-05-03T19:23:54Z | 0 | 0 | [
"size_categories:n<1K",
"format:parquet",
"modality:text",
"library:datasets",
"library:pandas",
"library:mlcroissant",
"library:polars",
"region:us"
] | [] | 2025-05-03T19:23:44Z | null | ---
dataset_info:
- config_name: default
features:
- name: task_id
dtype: string
- name: prompt
dtype: string
- name: generated_text
dtype: string
- name: generated_grid_rect
dtype: string
- name: task_solution
sequence:
sequence:
sequence: int64
- name: match
dtype: bool
- name: score
dtype: float64
splits:
- name: train
num_bytes: 509760
num_examples: 70
download_size: 85260
dataset_size: 509760
- config_name: main
features:
- name: task_id
dtype: string
- name: prompt
dtype: string
- name: generated_text
dtype: string
- name: generated_grid_rect
dtype: string
- name: task_solution
sequence:
sequence:
sequence: int64
- name: match
dtype: bool
- name: score
dtype: float64
splits:
- name: train
num_bytes: 509760
num_examples: 70
download_size: 85260
dataset_size: 509760
configs:
- config_name: default
data_files:
- split: train
path: data/train-*
- config_name: main
data_files:
- split: train
path: main/train-*
---
|
sltAI/crowdsourced-text-to-sign-language-rule-based-translation-corpus | sltAI | 2025-05-03T18:32:05Z | 372 | 0 | [
"size_categories:1K<n<10K",
"format:csv",
"modality:tabular",
"modality:text",
"library:datasets",
"library:pandas",
"library:mlcroissant",
"library:polars",
"region:us"
] | [] | 2024-04-11T16:03:46Z | null | ---
configs:
- config_name: default
data_files:
- split: train
path: data.csv
---
# Dataset Card for Dataset Name
<!-- Provide a quick summary of the dataset. -->
## Dataset Details
### Dataset Description
<!-- Provide a longer summary of what this dataset is. -->
- **Curated by:** [More Information Needed]
- **Funded by [optional]:** [More Information Needed]
- **Shared by [optional]:** [More Information Needed]
- **Language(s) (NLP):** [More Information Needed]
- **License:** [More Information Needed]
### Dataset Sources [optional]
<!-- Provide the basic links for the dataset. -->
- **Repository:** [More Information Needed]
- **Paper [optional]:** [More Information Needed]
- **Demo [optional]:** [More Information Needed]
## Uses
<!-- Address questions around how the dataset is intended to be used. -->
### Direct Use
<!-- This section describes suitable use cases for the dataset. -->
[More Information Needed]
### Out-of-Scope Use
<!-- This section addresses misuse, malicious use, and uses that the dataset will not work well for. -->
[More Information Needed]
## Dataset Structure
<!-- This section provides a description of the dataset fields, and additional information about the dataset structure such as criteria used to create the splits, relationships between data points, etc. -->
[More Information Needed]
## Dataset Creation
### Curation Rationale
<!-- Motivation for the creation of this dataset. -->
[More Information Needed]
### Source Data
<!-- This section describes the source data (e.g. news text and headlines, social media posts, translated sentences, ...). -->
#### Data Collection and Processing
<!-- This section describes the data collection and processing process such as data selection criteria, filtering and normalization methods, tools and libraries used, etc. -->
[More Information Needed]
#### Who are the source data producers?
<!-- This section describes the people or systems who originally created the data. It should also include self-reported demographic or identity information for the source data creators if this information is available. -->
[More Information Needed]
### Annotations [optional]
<!-- If the dataset contains annotations which are not part of the initial data collection, use this section to describe them. -->
#### Annotation process
<!-- This section describes the annotation process such as annotation tools used in the process, the amount of data annotated, annotation guidelines provided to the annotators, interannotator statistics, annotation validation, etc. -->
[More Information Needed]
#### Who are the annotators?
<!-- This section describes the people or systems who created the annotations. -->
[More Information Needed]
#### Personal and Sensitive Information
<!-- State whether the dataset contains data that might be considered personal, sensitive, or private (e.g., data that reveals addresses, uniquely identifiable names or aliases, racial or ethnic origins, sexual orientations, religious beliefs, political opinions, financial or health data, etc.). If efforts were made to anonymize the data, describe the anonymization process. -->
[More Information Needed]
## Bias, Risks, and Limitations
<!-- This section is meant to convey both technical and sociotechnical limitations. -->
[More Information Needed]
### Recommendations
<!-- This section is meant to convey recommendations with respect to the bias, risk, and technical limitations. -->
Users should be made aware of the risks, biases and limitations of the dataset. More information needed for further recommendations.
## Citation [optional]
<!-- If there is a paper or blog post introducing the dataset, the APA and Bibtex information for that should go in this section. -->
**BibTeX:**
[More Information Needed]
**APA:**
[More Information Needed]
## Glossary [optional]
<!-- If relevant, include terms and calculations in this section that can help readers understand the dataset or dataset card. -->
[More Information Needed]
## More Information [optional]
[More Information Needed]
## Dataset Card Authors [optional]
[More Information Needed]
## Dataset Card Contact
[More Information Needed] |
Subsets and Splits
No saved queries yet
Save your SQL queries to embed, download, and access them later. Queries will appear here once saved.