modelId
stringlengths 4
122
| author
stringlengths 2
42
| last_modified
unknown | downloads
int64 0
392M
| likes
int64 0
6.56k
| library_name
stringclasses 368
values | tags
sequencelengths 1
4.05k
| pipeline_tag
stringclasses 51
values | createdAt
unknown | card
stringlengths 1
1M
|
---|---|---|---|---|---|---|---|---|---|
nvidia/speakerverification_en_titanet_large | nvidia | "2023-11-14T16:58:18Z" | 2,818,031 | 70 | nemo | [
"nemo",
"speaker",
"speech",
"audio",
"speaker-verification",
"speaker-recognition",
"speaker-diarization",
"titanet",
"NeMo",
"pytorch",
"en",
"dataset:VOXCELEB-1",
"dataset:VOXCELEB-2",
"dataset:FISHER",
"dataset:switchboard",
"dataset:librispeech_asr",
"dataset:SRE",
"license:cc-by-4.0",
"model-index",
"region:us"
] | null | "2022-07-15T00:26:00Z" | ---
language:
- en
library_name: nemo
datasets:
- VOXCELEB-1
- VOXCELEB-2
- FISHER
- switchboard
- librispeech_asr
- SRE
thumbnail: null
tags:
- speaker
- speech
- audio
- speaker-verification
- speaker-recognition
- speaker-diarization
- titanet
- NeMo
- pytorch
license: cc-by-4.0
widget:
- src: https://huggingface.co/nvidia/speakerverification_en_titanet_large/resolve/main/an255-fash-b.wav
example_title: Speech sample 1
- src: https://huggingface.co/nvidia/speakerverification_en_titanet_large/resolve/main/cen7-fash-b.wav
example_title: Speech sample 2
model-index:
- name: speakerverification_en_titanet_large
results:
- task:
name: Speaker Verification
type: speaker-verification
dataset:
name: voxceleb1
type: voxceleb1-O
config: clean
split: test
args:
language: en
metrics:
- name: Test EER
type: eer
value: 0.66
- task:
type: Speaker Diarization
name: speaker-diarization
dataset:
name: ami-mixheadset
type: ami_diarization
config: oracle-vad-known-number-of-speakers
split: test
args:
language: en
metrics:
- name: Test DER
type: der
value: 1.73
- task:
type: Speaker Diarization
name: speaker-diarization
dataset:
name: ami-lapel
type: ami_diarization
config: oracle-vad-known-number-of-speakers
split: test
args:
language: en
metrics:
- name: Test DER
type: der
value: 2.03
- task:
type: Speaker Diarization
name: speaker-diarization
dataset:
name: ch109
type: callhome_diarization
config: oracle-vad-known-number-of-speakers
split: test
args:
language: en
metrics:
- name: Test DER
type: der
value: 1.19
- task:
type: Speaker Diarization
name: speaker-diarization
dataset:
name: nist-sre-2000
type: nist-sre_diarization
config: oracle-vad-known-number-of-speakers
split: test
args:
language: en
metrics:
- name: Test DER
type: der
value: 6.73
---
# NVIDIA TitaNet-Large (en-US)
<style>
img {
display: inline;
}
</style>
| [](#model-architecture)
| [](#model-architecture)
| [](#datasets)
This model extracts speaker embeddings from given speech, which is the backbone for speaker verification and diarization tasks.
It is a "large" version of TitaNet (around 23M parameters) models.
See the [model architecture](#model-architecture) section and [NeMo documentation](https://docs.nvidia.com/deeplearning/nemo/user-guide/docs/en/stable/asr/speaker_recognition/models.html#titanet) for complete architecture details.
## NVIDIA NeMo: Training
To train, fine-tune or play with the model you will need to install [NVIDIA NeMo](https://github.com/NVIDIA/NeMo). We recommend you install it after you've installed the latest Pytorch version.
```
pip install nemo_toolkit['all']
```
## How to Use this Model
The model is available for use in the NeMo toolkit [3] and can be used as a pre-trained checkpoint for inference or for fine-tuning on another dataset.
### Automatically instantiate the model
```python
import nemo.collections.asr as nemo_asr
speaker_model = nemo_asr.models.EncDecSpeakerLabelModel.from_pretrained("nvidia/speakerverification_en_titanet_large")
```
### Embedding Extraction
Using
```python
emb = speaker_model.get_embedding("an255-fash-b.wav")
```
### Verifying two utterances (Speaker Verification)
Now to check if two audio files are from the same speaker or not, simply do:
```python
speaker_model.verify_speakers("an255-fash-b.wav","cen7-fash-b.wav")
```
### Extracting Embeddings for more audio files
To extract embeddings from a bunch of audio files:
Write audio files to a `manifest.json` file with lines as in format:
```json
{"audio_filepath": "<absolute path to dataset>/audio_file.wav", "duration": "duration of file in sec", "label": "speaker_id"}
```
Then running following script will extract embeddings and writes to current working directory:
```shell
python <NeMo_root>/examples/speaker_tasks/recognition/extract_speaker_embeddings.py --manifest=manifest.json
```
### Input
This model accepts 16000 KHz Mono-channel Audio (wav files) as input.
### Output
This model provides speaker embeddings for an audio file.
## Model Architecture
TitaNet model is a depth-wise separable conv1D model [1] for Speaker Verification and diarization tasks. You may find more info on the detail of this model here: [TitaNet-Model](https://docs.nvidia.com/deeplearning/nemo/user-guide/docs/en/main/asr/speaker_recognition/models.html).
## Training
The NeMo toolkit [3] was used for training the models for over several hundred epochs. These model are trained with this [example script](https://github.com/NVIDIA/NeMo/blob/main/examples/speaker_tasks/recognition/speaker_reco.py) and this [base config](https://github.com/NVIDIA/NeMo/blob/main/examples/speaker_tasks/recognition/conf/titanet-large.yaml).
### Datasets
All the models in this collection are trained on a composite dataset comprising several thousand hours of English speech:
- Voxceleb-1
- Voxceleb-2
- Fisher
- Switchboard
- Librispeech
- SRE (2004-2010)
## Performance
Performances of the these models are reported in terms of Equal Error Rate (EER%) on speaker verification evaluation trial files and as Diarization Error Rate (DER%) on diarization test sessions.
* Speaker Verification (EER%)
| Version | Model | Model Size | VoxCeleb1 (Cleaned trial file) |
|---------|--------------|-----|---------------|
| 1.10.0 | TitaNet-Large | 23M | 0.66 |
* Speaker Diarization (DER%)
| Version | Model | Model Size | Evaluation Condition | NIST SRE 2000 | AMI (Lapel) | AMI (MixHeadset) | CH109 |
|---------|--------------|-----|----------------------|---------------|-------------|------------------|-------|
| 1.10.0 | TitaNet-Large | 23M | Oracle VAD KNOWN # of Speakers | 6.73 | 2.03 | 1.73 | 1.19 |
| 1.10.0 | TitaNet-Large | 23M | Oracle VAD UNKNOWN # of Speakers | 5.38 | 2.03 | 1.89 | 1.63 |
## Limitations
This model is trained on both telephonic and non-telephonic speech from voxceleb datasets, Fisher and switch board. If your domain of data differs from trained data or doesnot show relatively good performance consider finetuning for that speech domain.
## NVIDIA Riva: Deployment
[NVIDIA Riva](https://developer.nvidia.com/riva), is an accelerated speech AI SDK deployable on-prem, in all clouds, multi-cloud, hybrid, on edge, and embedded.
Additionally, Riva provides:
* World-class out-of-the-box accuracy for the most common languages with model checkpoints trained on proprietary data with hundreds of thousands of GPU-compute hours
* Best in class accuracy with run-time word boosting (e.g., brand and product names) and customization of acoustic model, language model, and inverse text normalization
* Streaming speech recognition, Kubernetes compatible scaling, and enterprise-grade support
Although this model isn’t supported yet by Riva, the [list of supported models is here](https://huggingface.co/models?other=Riva).
Check out [Riva live demo](https://developer.nvidia.com/riva#demos).
## References
[1] [TitaNet: Neural Model for Speaker Representation with 1D Depth-wise Separable convolutions and global context](https://ieeexplore.ieee.org/stamp/stamp.jsp?arnumber=9746806)
[2] [NVIDIA NeMo Toolkit](https://github.com/NVIDIA/NeMo)
## Licence
License to use this model is covered by the [CC-BY-4.0](https://creativecommons.org/licenses/by/4.0/). By downloading the public and release version of the model, you accept the terms and conditions of the [CC-BY-4.0](https://creativecommons.org/licenses/by/4.0/) license. |
distilbert/distilroberta-base | distilbert | "2024-02-19T11:09:58Z" | 2,744,900 | 136 | transformers | [
"transformers",
"pytorch",
"tf",
"jax",
"rust",
"safetensors",
"roberta",
"fill-mask",
"exbert",
"en",
"dataset:openwebtext",
"arxiv:1910.01108",
"arxiv:1910.09700",
"license:apache-2.0",
"autotrain_compatible",
"endpoints_compatible",
"region:us"
] | fill-mask | "2022-03-02T23:29:04Z" | ---
language: en
tags:
- exbert
license: apache-2.0
datasets:
- openwebtext
---
# Model Card for DistilRoBERTa base
# Table of Contents
1. [Model Details](#model-details)
2. [Uses](#uses)
3. [Bias, Risks, and Limitations](#bias-risks-and-limitations)
4. [Training Details](#training-details)
5. [Evaluation](#evaluation)
6. [Environmental Impact](#environmental-impact)
7. [Citation](#citation)
8. [How To Get Started With the Model](#how-to-get-started-with-the-model)
# Model Details
## Model Description
This model is a distilled version of the [RoBERTa-base model](https://huggingface.co/roberta-base). It follows the same training procedure as [DistilBERT](https://huggingface.co/distilbert-base-uncased).
The code for the distillation process can be found [here](https://github.com/huggingface/transformers/tree/master/examples/distillation).
This model is case-sensitive: it makes a difference between english and English.
The model has 6 layers, 768 dimension and 12 heads, totalizing 82M parameters (compared to 125M parameters for RoBERTa-base).
On average DistilRoBERTa is twice as fast as Roberta-base.
We encourage users of this model card to check out the [RoBERTa-base model card](https://huggingface.co/roberta-base) to learn more about usage, limitations and potential biases.
- **Developed by:** Victor Sanh, Lysandre Debut, Julien Chaumond, Thomas Wolf (Hugging Face)
- **Model type:** Transformer-based language model
- **Language(s) (NLP):** English
- **License:** Apache 2.0
- **Related Models:** [RoBERTa-base model card](https://huggingface.co/roberta-base)
- **Resources for more information:**
- [GitHub Repository](https://github.com/huggingface/transformers/blob/main/examples/research_projects/distillation/README.md)
- [Associated Paper](https://arxiv.org/abs/1910.01108)
# Uses
## Direct Use and Downstream Use
You can use the raw model for masked language modeling, but it's mostly intended to be fine-tuned on a downstream task. See the [model hub](https://huggingface.co/models?filter=roberta) to look for fine-tuned versions on a task that interests you.
Note that this model is primarily aimed at being fine-tuned on tasks that use the whole sentence (potentially masked) to make decisions, such as sequence classification, token classification or question answering. For tasks such as text generation you should look at model like GPT2.
## Out of Scope Use
The model should not be used to intentionally create hostile or alienating environments for people. The model was not trained to be factual or true representations of people or events, and therefore using the models to generate such content is out-of-scope for the abilities of this model.
# Bias, Risks, and Limitations
Significant research has explored bias and fairness issues with language models (see, e.g., [Sheng et al. (2021)](https://aclanthology.org/2021.acl-long.330.pdf) and [Bender et al. (2021)](https://dl.acm.org/doi/pdf/10.1145/3442188.3445922)). Predictions generated by the model may include disturbing and harmful stereotypes across protected classes; identity characteristics; and sensitive, social, and occupational groups. For example:
```python
>>> from transformers import pipeline
>>> unmasker = pipeline('fill-mask', model='distilroberta-base')
>>> unmasker("The man worked as a <mask>.")
[{'score': 0.1237526461482048,
'sequence': 'The man worked as a waiter.',
'token': 38233,
'token_str': ' waiter'},
{'score': 0.08968018740415573,
'sequence': 'The man worked as a waitress.',
'token': 35698,
'token_str': ' waitress'},
{'score': 0.08387645334005356,
'sequence': 'The man worked as a bartender.',
'token': 33080,
'token_str': ' bartender'},
{'score': 0.061059024184942245,
'sequence': 'The man worked as a mechanic.',
'token': 25682,
'token_str': ' mechanic'},
{'score': 0.03804653510451317,
'sequence': 'The man worked as a courier.',
'token': 37171,
'token_str': ' courier'}]
>>> unmasker("The woman worked as a <mask>.")
[{'score': 0.23149248957633972,
'sequence': 'The woman worked as a waitress.',
'token': 35698,
'token_str': ' waitress'},
{'score': 0.07563332468271255,
'sequence': 'The woman worked as a waiter.',
'token': 38233,
'token_str': ' waiter'},
{'score': 0.06983394920825958,
'sequence': 'The woman worked as a bartender.',
'token': 33080,
'token_str': ' bartender'},
{'score': 0.05411609262228012,
'sequence': 'The woman worked as a nurse.',
'token': 9008,
'token_str': ' nurse'},
{'score': 0.04995106905698776,
'sequence': 'The woman worked as a maid.',
'token': 29754,
'token_str': ' maid'}]
```
## Recommendations
Users (both direct and downstream) should be made aware of the risks, biases and limitations of the model.
# Training Details
DistilRoBERTa was pre-trained on [OpenWebTextCorpus](https://skylion007.github.io/OpenWebTextCorpus/), a reproduction of OpenAI's WebText dataset (it is ~4 times less training data than the teacher RoBERTa). See the [roberta-base model card](https://huggingface.co/roberta-base/blob/main/README.md) for further details on training.
# Evaluation
When fine-tuned on downstream tasks, this model achieves the following results (see [GitHub Repo](https://github.com/huggingface/transformers/blob/main/examples/research_projects/distillation/README.md)):
Glue test results:
| Task | MNLI | QQP | QNLI | SST-2 | CoLA | STS-B | MRPC | RTE |
|:----:|:----:|:----:|:----:|:-----:|:----:|:-----:|:----:|:----:|
| | 84.0 | 89.4 | 90.8 | 92.5 | 59.3 | 88.3 | 86.6 | 67.9 |
# Environmental Impact
Carbon emissions can be estimated using the [Machine Learning Impact calculator](https://mlco2.github.io/impact#compute) presented in [Lacoste et al. (2019)](https://arxiv.org/abs/1910.09700).
- **Hardware Type:** More information needed
- **Hours used:** More information needed
- **Cloud Provider:** More information needed
- **Compute Region:** More information needed
- **Carbon Emitted:** More information needed
# Citation
```bibtex
@article{Sanh2019DistilBERTAD,
title={DistilBERT, a distilled version of BERT: smaller, faster, cheaper and lighter},
author={Victor Sanh and Lysandre Debut and Julien Chaumond and Thomas Wolf},
journal={ArXiv},
year={2019},
volume={abs/1910.01108}
}
```
APA
- Sanh, V., Debut, L., Chaumond, J., & Wolf, T. (2019). DistilBERT, a distilled version of BERT: smaller, faster, cheaper and lighter. arXiv preprint arXiv:1910.01108.
# How to Get Started With the Model
You can use the model directly with a pipeline for masked language modeling:
```python
>>> from transformers import pipeline
>>> unmasker = pipeline('fill-mask', model='distilroberta-base')
>>> unmasker("Hello I'm a <mask> model.")
[{'score': 0.04673689603805542,
'sequence': "Hello I'm a business model.",
'token': 265,
'token_str': ' business'},
{'score': 0.03846118599176407,
'sequence': "Hello I'm a freelance model.",
'token': 18150,
'token_str': ' freelance'},
{'score': 0.03308931365609169,
'sequence': "Hello I'm a fashion model.",
'token': 2734,
'token_str': ' fashion'},
{'score': 0.03018997237086296,
'sequence': "Hello I'm a role model.",
'token': 774,
'token_str': ' role'},
{'score': 0.02111748233437538,
'sequence': "Hello I'm a Playboy model.",
'token': 24526,
'token_str': ' Playboy'}]
```
<a href="https://huggingface.co/exbert/?model=distilroberta-base">
<img width="300px" src="https://cdn-media.huggingface.co/exbert/button.png">
</a>
|
Alibaba-NLP/gte-large-en-v1.5 | Alibaba-NLP | "2024-08-09T03:32:05Z" | 2,732,953 | 172 | transformers | [
"transformers",
"onnx",
"safetensors",
"new",
"feature-extraction",
"sentence-transformers",
"gte",
"mteb",
"transformers.js",
"sentence-similarity",
"custom_code",
"en",
"dataset:allenai/c4",
"arxiv:2407.19669",
"arxiv:2308.03281",
"license:apache-2.0",
"model-index",
"text-embeddings-inference",
"endpoints_compatible",
"region:us"
] | sentence-similarity | "2024-04-20T02:54:30Z" | ---
datasets:
- allenai/c4
library_name: transformers
tags:
- sentence-transformers
- gte
- mteb
- transformers.js
- sentence-similarity
license: apache-2.0
language:
- en
model-index:
- name: gte-large-en-v1.5
results:
- task:
type: Classification
dataset:
type: mteb/amazon_counterfactual
name: MTEB AmazonCounterfactualClassification (en)
config: en
split: test
revision: e8379541af4e31359cca9fbcf4b00f2671dba205
metrics:
- type: accuracy
value: 73.01492537313432
- type: ap
value: 35.05341696659522
- type: f1
value: 66.71270310883853
- task:
type: Classification
dataset:
type: mteb/amazon_polarity
name: MTEB AmazonPolarityClassification
config: default
split: test
revision: e2d317d38cd51312af73b3d32a06d1a08b442046
metrics:
- type: accuracy
value: 93.97189999999999
- type: ap
value: 90.5952493948908
- type: f1
value: 93.95848137716877
- task:
type: Classification
dataset:
type: mteb/amazon_reviews_multi
name: MTEB AmazonReviewsClassification (en)
config: en
split: test
revision: 1399c76144fd37290681b995c656ef9b2e06e26d
metrics:
- type: accuracy
value: 54.196
- type: f1
value: 53.80122334012787
- task:
type: Retrieval
dataset:
type: mteb/arguana
name: MTEB ArguAna
config: default
split: test
revision: c22ab2a51041ffd869aaddef7af8d8215647e41a
metrics:
- type: map_at_1
value: 47.297
- type: map_at_10
value: 64.303
- type: map_at_100
value: 64.541
- type: map_at_1000
value: 64.541
- type: map_at_3
value: 60.728
- type: map_at_5
value: 63.114000000000004
- type: mrr_at_1
value: 48.435
- type: mrr_at_10
value: 64.657
- type: mrr_at_100
value: 64.901
- type: mrr_at_1000
value: 64.901
- type: mrr_at_3
value: 61.06
- type: mrr_at_5
value: 63.514
- type: ndcg_at_1
value: 47.297
- type: ndcg_at_10
value: 72.107
- type: ndcg_at_100
value: 72.963
- type: ndcg_at_1000
value: 72.963
- type: ndcg_at_3
value: 65.063
- type: ndcg_at_5
value: 69.352
- type: precision_at_1
value: 47.297
- type: precision_at_10
value: 9.623
- type: precision_at_100
value: 0.996
- type: precision_at_1000
value: 0.1
- type: precision_at_3
value: 25.865
- type: precision_at_5
value: 17.596
- type: recall_at_1
value: 47.297
- type: recall_at_10
value: 96.23
- type: recall_at_100
value: 99.644
- type: recall_at_1000
value: 99.644
- type: recall_at_3
value: 77.596
- type: recall_at_5
value: 87.98
- task:
type: Clustering
dataset:
type: mteb/arxiv-clustering-p2p
name: MTEB ArxivClusteringP2P
config: default
split: test
revision: a122ad7f3f0291bf49cc6f4d32aa80929df69d5d
metrics:
- type: v_measure
value: 48.467787861077475
- task:
type: Clustering
dataset:
type: mteb/arxiv-clustering-s2s
name: MTEB ArxivClusteringS2S
config: default
split: test
revision: f910caf1a6075f7329cdf8c1a6135696f37dbd53
metrics:
- type: v_measure
value: 43.39198391914257
- task:
type: Reranking
dataset:
type: mteb/askubuntudupquestions-reranking
name: MTEB AskUbuntuDupQuestions
config: default
split: test
revision: 2000358ca161889fa9c082cb41daa8dcfb161a54
metrics:
- type: map
value: 63.12794820591384
- type: mrr
value: 75.9331442641692
- task:
type: STS
dataset:
type: mteb/biosses-sts
name: MTEB BIOSSES
config: default
split: test
revision: d3fb88f8f02e40887cd149695127462bbcf29b4a
metrics:
- type: cos_sim_pearson
value: 87.85062993863319
- type: cos_sim_spearman
value: 85.39049989733459
- type: euclidean_pearson
value: 86.00222680278333
- type: euclidean_spearman
value: 85.45556162077396
- type: manhattan_pearson
value: 85.88769871785621
- type: manhattan_spearman
value: 85.11760211290839
- task:
type: Classification
dataset:
type: mteb/banking77
name: MTEB Banking77Classification
config: default
split: test
revision: 0fd18e25b25c072e09e0d92ab615fda904d66300
metrics:
- type: accuracy
value: 87.32792207792208
- type: f1
value: 87.29132945999555
- task:
type: Clustering
dataset:
type: mteb/biorxiv-clustering-p2p
name: MTEB BiorxivClusteringP2P
config: default
split: test
revision: 65b79d1d13f80053f67aca9498d9402c2d9f1f40
metrics:
- type: v_measure
value: 40.5779328301945
- task:
type: Clustering
dataset:
type: mteb/biorxiv-clustering-s2s
name: MTEB BiorxivClusteringS2S
config: default
split: test
revision: 258694dd0231531bc1fd9de6ceb52a0853c6d908
metrics:
- type: v_measure
value: 37.94425623865118
- task:
type: Retrieval
dataset:
type: mteb/cqadupstack-android
name: MTEB CQADupstackAndroidRetrieval
config: default
split: test
revision: f46a197baaae43b4f621051089b82a364682dfeb
metrics:
- type: map_at_1
value: 32.978
- type: map_at_10
value: 44.45
- type: map_at_100
value: 46.19
- type: map_at_1000
value: 46.303
- type: map_at_3
value: 40.849000000000004
- type: map_at_5
value: 42.55
- type: mrr_at_1
value: 40.629
- type: mrr_at_10
value: 50.848000000000006
- type: mrr_at_100
value: 51.669
- type: mrr_at_1000
value: 51.705
- type: mrr_at_3
value: 47.997
- type: mrr_at_5
value: 49.506
- type: ndcg_at_1
value: 40.629
- type: ndcg_at_10
value: 51.102000000000004
- type: ndcg_at_100
value: 57.159000000000006
- type: ndcg_at_1000
value: 58.669000000000004
- type: ndcg_at_3
value: 45.738
- type: ndcg_at_5
value: 47.632999999999996
- type: precision_at_1
value: 40.629
- type: precision_at_10
value: 9.700000000000001
- type: precision_at_100
value: 1.5970000000000002
- type: precision_at_1000
value: 0.202
- type: precision_at_3
value: 21.698
- type: precision_at_5
value: 15.393
- type: recall_at_1
value: 32.978
- type: recall_at_10
value: 63.711
- type: recall_at_100
value: 88.39399999999999
- type: recall_at_1000
value: 97.513
- type: recall_at_3
value: 48.025
- type: recall_at_5
value: 53.52
- task:
type: Retrieval
dataset:
type: mteb/cqadupstack-english
name: MTEB CQADupstackEnglishRetrieval
config: default
split: test
revision: ad9991cb51e31e31e430383c75ffb2885547b5f0
metrics:
- type: map_at_1
value: 30.767
- type: map_at_10
value: 42.195
- type: map_at_100
value: 43.541999999999994
- type: map_at_1000
value: 43.673
- type: map_at_3
value: 38.561
- type: map_at_5
value: 40.532000000000004
- type: mrr_at_1
value: 38.79
- type: mrr_at_10
value: 48.021
- type: mrr_at_100
value: 48.735
- type: mrr_at_1000
value: 48.776
- type: mrr_at_3
value: 45.594
- type: mrr_at_5
value: 46.986
- type: ndcg_at_1
value: 38.79
- type: ndcg_at_10
value: 48.468
- type: ndcg_at_100
value: 53.037
- type: ndcg_at_1000
value: 55.001999999999995
- type: ndcg_at_3
value: 43.409
- type: ndcg_at_5
value: 45.654
- type: precision_at_1
value: 38.79
- type: precision_at_10
value: 9.452
- type: precision_at_100
value: 1.518
- type: precision_at_1000
value: 0.201
- type: precision_at_3
value: 21.21
- type: precision_at_5
value: 15.171999999999999
- type: recall_at_1
value: 30.767
- type: recall_at_10
value: 60.118
- type: recall_at_100
value: 79.271
- type: recall_at_1000
value: 91.43299999999999
- type: recall_at_3
value: 45.36
- type: recall_at_5
value: 51.705
- task:
type: Retrieval
dataset:
type: mteb/cqadupstack-gaming
name: MTEB CQADupstackGamingRetrieval
config: default
split: test
revision: 4885aa143210c98657558c04aaf3dc47cfb54340
metrics:
- type: map_at_1
value: 40.007
- type: map_at_10
value: 53.529
- type: map_at_100
value: 54.602
- type: map_at_1000
value: 54.647
- type: map_at_3
value: 49.951
- type: map_at_5
value: 52.066
- type: mrr_at_1
value: 45.705
- type: mrr_at_10
value: 56.745000000000005
- type: mrr_at_100
value: 57.43899999999999
- type: mrr_at_1000
value: 57.462999999999994
- type: mrr_at_3
value: 54.25299999999999
- type: mrr_at_5
value: 55.842000000000006
- type: ndcg_at_1
value: 45.705
- type: ndcg_at_10
value: 59.809
- type: ndcg_at_100
value: 63.837999999999994
- type: ndcg_at_1000
value: 64.729
- type: ndcg_at_3
value: 53.994
- type: ndcg_at_5
value: 57.028
- type: precision_at_1
value: 45.705
- type: precision_at_10
value: 9.762
- type: precision_at_100
value: 1.275
- type: precision_at_1000
value: 0.13899999999999998
- type: precision_at_3
value: 24.368000000000002
- type: precision_at_5
value: 16.84
- type: recall_at_1
value: 40.007
- type: recall_at_10
value: 75.017
- type: recall_at_100
value: 91.99000000000001
- type: recall_at_1000
value: 98.265
- type: recall_at_3
value: 59.704
- type: recall_at_5
value: 67.109
- task:
type: Retrieval
dataset:
type: mteb/cqadupstack-gis
name: MTEB CQADupstackGisRetrieval
config: default
split: test
revision: 5003b3064772da1887988e05400cf3806fe491f2
metrics:
- type: map_at_1
value: 26.639000000000003
- type: map_at_10
value: 35.926
- type: map_at_100
value: 37.126999999999995
- type: map_at_1000
value: 37.202
- type: map_at_3
value: 32.989000000000004
- type: map_at_5
value: 34.465
- type: mrr_at_1
value: 28.475
- type: mrr_at_10
value: 37.7
- type: mrr_at_100
value: 38.753
- type: mrr_at_1000
value: 38.807
- type: mrr_at_3
value: 35.066
- type: mrr_at_5
value: 36.512
- type: ndcg_at_1
value: 28.475
- type: ndcg_at_10
value: 41.245
- type: ndcg_at_100
value: 46.814
- type: ndcg_at_1000
value: 48.571
- type: ndcg_at_3
value: 35.528999999999996
- type: ndcg_at_5
value: 38.066
- type: precision_at_1
value: 28.475
- type: precision_at_10
value: 6.497
- type: precision_at_100
value: 0.9650000000000001
- type: precision_at_1000
value: 0.11499999999999999
- type: precision_at_3
value: 15.065999999999999
- type: precision_at_5
value: 10.599
- type: recall_at_1
value: 26.639000000000003
- type: recall_at_10
value: 55.759
- type: recall_at_100
value: 80.913
- type: recall_at_1000
value: 93.929
- type: recall_at_3
value: 40.454
- type: recall_at_5
value: 46.439
- task:
type: Retrieval
dataset:
type: mteb/cqadupstack-mathematica
name: MTEB CQADupstackMathematicaRetrieval
config: default
split: test
revision: 90fceea13679c63fe563ded68f3b6f06e50061de
metrics:
- type: map_at_1
value: 15.767999999999999
- type: map_at_10
value: 24.811
- type: map_at_100
value: 26.064999999999998
- type: map_at_1000
value: 26.186999999999998
- type: map_at_3
value: 21.736
- type: map_at_5
value: 23.283
- type: mrr_at_1
value: 19.527
- type: mrr_at_10
value: 29.179
- type: mrr_at_100
value: 30.153999999999996
- type: mrr_at_1000
value: 30.215999999999998
- type: mrr_at_3
value: 26.223000000000003
- type: mrr_at_5
value: 27.733999999999998
- type: ndcg_at_1
value: 19.527
- type: ndcg_at_10
value: 30.786
- type: ndcg_at_100
value: 36.644
- type: ndcg_at_1000
value: 39.440999999999995
- type: ndcg_at_3
value: 24.958
- type: ndcg_at_5
value: 27.392
- type: precision_at_1
value: 19.527
- type: precision_at_10
value: 5.995
- type: precision_at_100
value: 1.03
- type: precision_at_1000
value: 0.14100000000000001
- type: precision_at_3
value: 12.520999999999999
- type: precision_at_5
value: 9.129
- type: recall_at_1
value: 15.767999999999999
- type: recall_at_10
value: 44.824000000000005
- type: recall_at_100
value: 70.186
- type: recall_at_1000
value: 89.934
- type: recall_at_3
value: 28.607
- type: recall_at_5
value: 34.836
- task:
type: Retrieval
dataset:
type: mteb/cqadupstack-physics
name: MTEB CQADupstackPhysicsRetrieval
config: default
split: test
revision: 79531abbd1fb92d06c6d6315a0cbbbf5bb247ea4
metrics:
- type: map_at_1
value: 31.952
- type: map_at_10
value: 44.438
- type: map_at_100
value: 45.778
- type: map_at_1000
value: 45.883
- type: map_at_3
value: 41.044000000000004
- type: map_at_5
value: 42.986000000000004
- type: mrr_at_1
value: 39.172000000000004
- type: mrr_at_10
value: 49.76
- type: mrr_at_100
value: 50.583999999999996
- type: mrr_at_1000
value: 50.621
- type: mrr_at_3
value: 47.353
- type: mrr_at_5
value: 48.739
- type: ndcg_at_1
value: 39.172000000000004
- type: ndcg_at_10
value: 50.760000000000005
- type: ndcg_at_100
value: 56.084
- type: ndcg_at_1000
value: 57.865
- type: ndcg_at_3
value: 45.663
- type: ndcg_at_5
value: 48.178
- type: precision_at_1
value: 39.172000000000004
- type: precision_at_10
value: 9.22
- type: precision_at_100
value: 1.387
- type: precision_at_1000
value: 0.17099999999999999
- type: precision_at_3
value: 21.976000000000003
- type: precision_at_5
value: 15.457
- type: recall_at_1
value: 31.952
- type: recall_at_10
value: 63.900999999999996
- type: recall_at_100
value: 85.676
- type: recall_at_1000
value: 97.03699999999999
- type: recall_at_3
value: 49.781
- type: recall_at_5
value: 56.330000000000005
- task:
type: Retrieval
dataset:
type: mteb/cqadupstack-programmers
name: MTEB CQADupstackProgrammersRetrieval
config: default
split: test
revision: 6184bc1440d2dbc7612be22b50686b8826d22b32
metrics:
- type: map_at_1
value: 25.332
- type: map_at_10
value: 36.874
- type: map_at_100
value: 38.340999999999994
- type: map_at_1000
value: 38.452
- type: map_at_3
value: 33.068
- type: map_at_5
value: 35.324
- type: mrr_at_1
value: 30.822
- type: mrr_at_10
value: 41.641
- type: mrr_at_100
value: 42.519
- type: mrr_at_1000
value: 42.573
- type: mrr_at_3
value: 38.413000000000004
- type: mrr_at_5
value: 40.542
- type: ndcg_at_1
value: 30.822
- type: ndcg_at_10
value: 43.414
- type: ndcg_at_100
value: 49.196
- type: ndcg_at_1000
value: 51.237
- type: ndcg_at_3
value: 37.230000000000004
- type: ndcg_at_5
value: 40.405
- type: precision_at_1
value: 30.822
- type: precision_at_10
value: 8.379
- type: precision_at_100
value: 1.315
- type: precision_at_1000
value: 0.168
- type: precision_at_3
value: 18.417
- type: precision_at_5
value: 13.744
- type: recall_at_1
value: 25.332
- type: recall_at_10
value: 57.774
- type: recall_at_100
value: 82.071
- type: recall_at_1000
value: 95.60600000000001
- type: recall_at_3
value: 40.722
- type: recall_at_5
value: 48.754999999999995
- task:
type: Retrieval
dataset:
type: mteb/cqadupstack
name: MTEB CQADupstackRetrieval
config: default
split: test
revision: 4ffe81d471b1924886b33c7567bfb200e9eec5c4
metrics:
- type: map_at_1
value: 25.91033333333334
- type: map_at_10
value: 36.23225000000001
- type: map_at_100
value: 37.55766666666667
- type: map_at_1000
value: 37.672583333333336
- type: map_at_3
value: 32.95666666666667
- type: map_at_5
value: 34.73375
- type: mrr_at_1
value: 30.634
- type: mrr_at_10
value: 40.19449999999999
- type: mrr_at_100
value: 41.099250000000005
- type: mrr_at_1000
value: 41.15091666666667
- type: mrr_at_3
value: 37.4615
- type: mrr_at_5
value: 39.00216666666667
- type: ndcg_at_1
value: 30.634
- type: ndcg_at_10
value: 42.162166666666664
- type: ndcg_at_100
value: 47.60708333333333
- type: ndcg_at_1000
value: 49.68616666666666
- type: ndcg_at_3
value: 36.60316666666666
- type: ndcg_at_5
value: 39.15616666666668
- type: precision_at_1
value: 30.634
- type: precision_at_10
value: 7.6193333333333335
- type: precision_at_100
value: 1.2198333333333333
- type: precision_at_1000
value: 0.15975000000000003
- type: precision_at_3
value: 17.087
- type: precision_at_5
value: 12.298333333333334
- type: recall_at_1
value: 25.91033333333334
- type: recall_at_10
value: 55.67300000000001
- type: recall_at_100
value: 79.20608333333334
- type: recall_at_1000
value: 93.34866666666667
- type: recall_at_3
value: 40.34858333333333
- type: recall_at_5
value: 46.834083333333325
- task:
type: Retrieval
dataset:
type: mteb/cqadupstack-stats
name: MTEB CQADupstackStatsRetrieval
config: default
split: test
revision: 65ac3a16b8e91f9cee4c9828cc7c335575432a2a
metrics:
- type: map_at_1
value: 25.006
- type: map_at_10
value: 32.177
- type: map_at_100
value: 33.324999999999996
- type: map_at_1000
value: 33.419
- type: map_at_3
value: 29.952
- type: map_at_5
value: 31.095
- type: mrr_at_1
value: 28.066999999999997
- type: mrr_at_10
value: 34.995
- type: mrr_at_100
value: 35.978
- type: mrr_at_1000
value: 36.042
- type: mrr_at_3
value: 33.103
- type: mrr_at_5
value: 34.001
- type: ndcg_at_1
value: 28.066999999999997
- type: ndcg_at_10
value: 36.481
- type: ndcg_at_100
value: 42.022999999999996
- type: ndcg_at_1000
value: 44.377
- type: ndcg_at_3
value: 32.394
- type: ndcg_at_5
value: 34.108
- type: precision_at_1
value: 28.066999999999997
- type: precision_at_10
value: 5.736
- type: precision_at_100
value: 0.9259999999999999
- type: precision_at_1000
value: 0.12
- type: precision_at_3
value: 13.804
- type: precision_at_5
value: 9.508999999999999
- type: recall_at_1
value: 25.006
- type: recall_at_10
value: 46.972
- type: recall_at_100
value: 72.138
- type: recall_at_1000
value: 89.479
- type: recall_at_3
value: 35.793
- type: recall_at_5
value: 39.947
- task:
type: Retrieval
dataset:
type: mteb/cqadupstack-tex
name: MTEB CQADupstackTexRetrieval
config: default
split: test
revision: 46989137a86843e03a6195de44b09deda022eec7
metrics:
- type: map_at_1
value: 16.07
- type: map_at_10
value: 24.447
- type: map_at_100
value: 25.685999999999996
- type: map_at_1000
value: 25.813999999999997
- type: map_at_3
value: 21.634
- type: map_at_5
value: 23.133
- type: mrr_at_1
value: 19.580000000000002
- type: mrr_at_10
value: 28.127999999999997
- type: mrr_at_100
value: 29.119
- type: mrr_at_1000
value: 29.192
- type: mrr_at_3
value: 25.509999999999998
- type: mrr_at_5
value: 26.878
- type: ndcg_at_1
value: 19.580000000000002
- type: ndcg_at_10
value: 29.804000000000002
- type: ndcg_at_100
value: 35.555
- type: ndcg_at_1000
value: 38.421
- type: ndcg_at_3
value: 24.654999999999998
- type: ndcg_at_5
value: 26.881
- type: precision_at_1
value: 19.580000000000002
- type: precision_at_10
value: 5.736
- type: precision_at_100
value: 1.005
- type: precision_at_1000
value: 0.145
- type: precision_at_3
value: 12.033000000000001
- type: precision_at_5
value: 8.871
- type: recall_at_1
value: 16.07
- type: recall_at_10
value: 42.364000000000004
- type: recall_at_100
value: 68.01899999999999
- type: recall_at_1000
value: 88.122
- type: recall_at_3
value: 27.846
- type: recall_at_5
value: 33.638
- task:
type: Retrieval
dataset:
type: mteb/cqadupstack-unix
name: MTEB CQADupstackUnixRetrieval
config: default
split: test
revision: 6c6430d3a6d36f8d2a829195bc5dc94d7e063e53
metrics:
- type: map_at_1
value: 26.365
- type: map_at_10
value: 36.591
- type: map_at_100
value: 37.730000000000004
- type: map_at_1000
value: 37.84
- type: map_at_3
value: 33.403
- type: map_at_5
value: 35.272999999999996
- type: mrr_at_1
value: 30.503999999999998
- type: mrr_at_10
value: 39.940999999999995
- type: mrr_at_100
value: 40.818
- type: mrr_at_1000
value: 40.876000000000005
- type: mrr_at_3
value: 37.065
- type: mrr_at_5
value: 38.814
- type: ndcg_at_1
value: 30.503999999999998
- type: ndcg_at_10
value: 42.185
- type: ndcg_at_100
value: 47.416000000000004
- type: ndcg_at_1000
value: 49.705
- type: ndcg_at_3
value: 36.568
- type: ndcg_at_5
value: 39.416000000000004
- type: precision_at_1
value: 30.503999999999998
- type: precision_at_10
value: 7.276000000000001
- type: precision_at_100
value: 1.118
- type: precision_at_1000
value: 0.14300000000000002
- type: precision_at_3
value: 16.729
- type: precision_at_5
value: 12.107999999999999
- type: recall_at_1
value: 26.365
- type: recall_at_10
value: 55.616
- type: recall_at_100
value: 78.129
- type: recall_at_1000
value: 93.95599999999999
- type: recall_at_3
value: 40.686
- type: recall_at_5
value: 47.668
- task:
type: Retrieval
dataset:
type: mteb/cqadupstack-webmasters
name: MTEB CQADupstackWebmastersRetrieval
config: default
split: test
revision: 160c094312a0e1facb97e55eeddb698c0abe3571
metrics:
- type: map_at_1
value: 22.750999999999998
- type: map_at_10
value: 33.446
- type: map_at_100
value: 35.235
- type: map_at_1000
value: 35.478
- type: map_at_3
value: 29.358
- type: map_at_5
value: 31.525
- type: mrr_at_1
value: 27.668
- type: mrr_at_10
value: 37.694
- type: mrr_at_100
value: 38.732
- type: mrr_at_1000
value: 38.779
- type: mrr_at_3
value: 34.223
- type: mrr_at_5
value: 36.08
- type: ndcg_at_1
value: 27.668
- type: ndcg_at_10
value: 40.557
- type: ndcg_at_100
value: 46.605999999999995
- type: ndcg_at_1000
value: 48.917
- type: ndcg_at_3
value: 33.677
- type: ndcg_at_5
value: 36.85
- type: precision_at_1
value: 27.668
- type: precision_at_10
value: 8.3
- type: precision_at_100
value: 1.6260000000000001
- type: precision_at_1000
value: 0.253
- type: precision_at_3
value: 16.008
- type: precision_at_5
value: 12.292
- type: recall_at_1
value: 22.750999999999998
- type: recall_at_10
value: 55.643
- type: recall_at_100
value: 82.151
- type: recall_at_1000
value: 95.963
- type: recall_at_3
value: 36.623
- type: recall_at_5
value: 44.708
- task:
type: Retrieval
dataset:
type: mteb/cqadupstack-wordpress
name: MTEB CQADupstackWordpressRetrieval
config: default
split: test
revision: 4ffe81d471b1924886b33c7567bfb200e9eec5c4
metrics:
- type: map_at_1
value: 17.288999999999998
- type: map_at_10
value: 25.903
- type: map_at_100
value: 27.071
- type: map_at_1000
value: 27.173000000000002
- type: map_at_3
value: 22.935
- type: map_at_5
value: 24.573
- type: mrr_at_1
value: 18.669
- type: mrr_at_10
value: 27.682000000000002
- type: mrr_at_100
value: 28.691
- type: mrr_at_1000
value: 28.761
- type: mrr_at_3
value: 24.738
- type: mrr_at_5
value: 26.392
- type: ndcg_at_1
value: 18.669
- type: ndcg_at_10
value: 31.335
- type: ndcg_at_100
value: 36.913000000000004
- type: ndcg_at_1000
value: 39.300000000000004
- type: ndcg_at_3
value: 25.423000000000002
- type: ndcg_at_5
value: 28.262999999999998
- type: precision_at_1
value: 18.669
- type: precision_at_10
value: 5.379
- type: precision_at_100
value: 0.876
- type: precision_at_1000
value: 0.11900000000000001
- type: precision_at_3
value: 11.214
- type: precision_at_5
value: 8.466
- type: recall_at_1
value: 17.288999999999998
- type: recall_at_10
value: 46.377
- type: recall_at_100
value: 71.53500000000001
- type: recall_at_1000
value: 88.947
- type: recall_at_3
value: 30.581999999999997
- type: recall_at_5
value: 37.354
- task:
type: Retrieval
dataset:
type: mteb/climate-fever
name: MTEB ClimateFEVER
config: default
split: test
revision: 47f2ac6acb640fc46020b02a5b59fdda04d39380
metrics:
- type: map_at_1
value: 21.795
- type: map_at_10
value: 37.614999999999995
- type: map_at_100
value: 40.037
- type: map_at_1000
value: 40.184999999999995
- type: map_at_3
value: 32.221
- type: map_at_5
value: 35.154999999999994
- type: mrr_at_1
value: 50.358000000000004
- type: mrr_at_10
value: 62.129
- type: mrr_at_100
value: 62.613
- type: mrr_at_1000
value: 62.62
- type: mrr_at_3
value: 59.272999999999996
- type: mrr_at_5
value: 61.138999999999996
- type: ndcg_at_1
value: 50.358000000000004
- type: ndcg_at_10
value: 48.362
- type: ndcg_at_100
value: 55.932
- type: ndcg_at_1000
value: 58.062999999999995
- type: ndcg_at_3
value: 42.111
- type: ndcg_at_5
value: 44.063
- type: precision_at_1
value: 50.358000000000004
- type: precision_at_10
value: 14.677999999999999
- type: precision_at_100
value: 2.2950000000000004
- type: precision_at_1000
value: 0.271
- type: precision_at_3
value: 31.77
- type: precision_at_5
value: 23.375
- type: recall_at_1
value: 21.795
- type: recall_at_10
value: 53.846000000000004
- type: recall_at_100
value: 78.952
- type: recall_at_1000
value: 90.41900000000001
- type: recall_at_3
value: 37.257
- type: recall_at_5
value: 44.661
- task:
type: Retrieval
dataset:
type: mteb/dbpedia
name: MTEB DBPedia
config: default
split: test
revision: c0f706b76e590d620bd6618b3ca8efdd34e2d659
metrics:
- type: map_at_1
value: 9.728
- type: map_at_10
value: 22.691
- type: map_at_100
value: 31.734
- type: map_at_1000
value: 33.464
- type: map_at_3
value: 16.273
- type: map_at_5
value: 19.016
- type: mrr_at_1
value: 73.25
- type: mrr_at_10
value: 80.782
- type: mrr_at_100
value: 81.01899999999999
- type: mrr_at_1000
value: 81.021
- type: mrr_at_3
value: 79.583
- type: mrr_at_5
value: 80.146
- type: ndcg_at_1
value: 59.62499999999999
- type: ndcg_at_10
value: 46.304
- type: ndcg_at_100
value: 51.23
- type: ndcg_at_1000
value: 58.048
- type: ndcg_at_3
value: 51.541000000000004
- type: ndcg_at_5
value: 48.635
- type: precision_at_1
value: 73.25
- type: precision_at_10
value: 36.375
- type: precision_at_100
value: 11.53
- type: precision_at_1000
value: 2.23
- type: precision_at_3
value: 55.583000000000006
- type: precision_at_5
value: 47.15
- type: recall_at_1
value: 9.728
- type: recall_at_10
value: 28.793999999999997
- type: recall_at_100
value: 57.885
- type: recall_at_1000
value: 78.759
- type: recall_at_3
value: 17.79
- type: recall_at_5
value: 21.733
- task:
type: Classification
dataset:
type: mteb/emotion
name: MTEB EmotionClassification
config: default
split: test
revision: 4f58c6b202a23cf9a4da393831edf4f9183cad37
metrics:
- type: accuracy
value: 46.775
- type: f1
value: 41.89794273264891
- task:
type: Retrieval
dataset:
type: mteb/fever
name: MTEB FEVER
config: default
split: test
revision: bea83ef9e8fb933d90a2f1d5515737465d613e12
metrics:
- type: map_at_1
value: 85.378
- type: map_at_10
value: 91.51
- type: map_at_100
value: 91.666
- type: map_at_1000
value: 91.676
- type: map_at_3
value: 90.757
- type: map_at_5
value: 91.277
- type: mrr_at_1
value: 91.839
- type: mrr_at_10
value: 95.49
- type: mrr_at_100
value: 95.493
- type: mrr_at_1000
value: 95.493
- type: mrr_at_3
value: 95.345
- type: mrr_at_5
value: 95.47200000000001
- type: ndcg_at_1
value: 91.839
- type: ndcg_at_10
value: 93.806
- type: ndcg_at_100
value: 94.255
- type: ndcg_at_1000
value: 94.399
- type: ndcg_at_3
value: 93.027
- type: ndcg_at_5
value: 93.51
- type: precision_at_1
value: 91.839
- type: precision_at_10
value: 10.93
- type: precision_at_100
value: 1.1400000000000001
- type: precision_at_1000
value: 0.117
- type: precision_at_3
value: 34.873
- type: precision_at_5
value: 21.44
- type: recall_at_1
value: 85.378
- type: recall_at_10
value: 96.814
- type: recall_at_100
value: 98.386
- type: recall_at_1000
value: 99.21600000000001
- type: recall_at_3
value: 94.643
- type: recall_at_5
value: 95.976
- task:
type: Retrieval
dataset:
type: mteb/fiqa
name: MTEB FiQA2018
config: default
split: test
revision: 27a168819829fe9bcd655c2df245fb19452e8e06
metrics:
- type: map_at_1
value: 32.190000000000005
- type: map_at_10
value: 53.605000000000004
- type: map_at_100
value: 55.550999999999995
- type: map_at_1000
value: 55.665
- type: map_at_3
value: 46.62
- type: map_at_5
value: 50.517999999999994
- type: mrr_at_1
value: 60.34
- type: mrr_at_10
value: 70.775
- type: mrr_at_100
value: 71.238
- type: mrr_at_1000
value: 71.244
- type: mrr_at_3
value: 68.72399999999999
- type: mrr_at_5
value: 69.959
- type: ndcg_at_1
value: 60.34
- type: ndcg_at_10
value: 63.226000000000006
- type: ndcg_at_100
value: 68.60300000000001
- type: ndcg_at_1000
value: 69.901
- type: ndcg_at_3
value: 58.048
- type: ndcg_at_5
value: 59.789
- type: precision_at_1
value: 60.34
- type: precision_at_10
value: 17.130000000000003
- type: precision_at_100
value: 2.29
- type: precision_at_1000
value: 0.256
- type: precision_at_3
value: 38.323
- type: precision_at_5
value: 27.87
- type: recall_at_1
value: 32.190000000000005
- type: recall_at_10
value: 73.041
- type: recall_at_100
value: 91.31
- type: recall_at_1000
value: 98.104
- type: recall_at_3
value: 53.70399999999999
- type: recall_at_5
value: 62.358999999999995
- task:
type: Retrieval
dataset:
type: mteb/hotpotqa
name: MTEB HotpotQA
config: default
split: test
revision: ab518f4d6fcca38d87c25209f94beba119d02014
metrics:
- type: map_at_1
value: 43.511
- type: map_at_10
value: 58.15
- type: map_at_100
value: 58.95399999999999
- type: map_at_1000
value: 59.018
- type: map_at_3
value: 55.31700000000001
- type: map_at_5
value: 57.04900000000001
- type: mrr_at_1
value: 87.022
- type: mrr_at_10
value: 91.32000000000001
- type: mrr_at_100
value: 91.401
- type: mrr_at_1000
value: 91.403
- type: mrr_at_3
value: 90.77
- type: mrr_at_5
value: 91.156
- type: ndcg_at_1
value: 87.022
- type: ndcg_at_10
value: 68.183
- type: ndcg_at_100
value: 70.781
- type: ndcg_at_1000
value: 72.009
- type: ndcg_at_3
value: 64.334
- type: ndcg_at_5
value: 66.449
- type: precision_at_1
value: 87.022
- type: precision_at_10
value: 13.406
- type: precision_at_100
value: 1.542
- type: precision_at_1000
value: 0.17099999999999999
- type: precision_at_3
value: 39.023
- type: precision_at_5
value: 25.080000000000002
- type: recall_at_1
value: 43.511
- type: recall_at_10
value: 67.02900000000001
- type: recall_at_100
value: 77.11
- type: recall_at_1000
value: 85.294
- type: recall_at_3
value: 58.535000000000004
- type: recall_at_5
value: 62.70099999999999
- task:
type: Classification
dataset:
type: mteb/imdb
name: MTEB ImdbClassification
config: default
split: test
revision: 3d86128a09e091d6018b6d26cad27f2739fc2db7
metrics:
- type: accuracy
value: 92.0996
- type: ap
value: 87.86206089096373
- type: f1
value: 92.07554547510763
- task:
type: Retrieval
dataset:
type: mteb/msmarco
name: MTEB MSMARCO
config: default
split: dev
revision: c5a29a104738b98a9e76336939199e264163d4a0
metrics:
- type: map_at_1
value: 23.179
- type: map_at_10
value: 35.86
- type: map_at_100
value: 37.025999999999996
- type: map_at_1000
value: 37.068
- type: map_at_3
value: 31.921
- type: map_at_5
value: 34.172000000000004
- type: mrr_at_1
value: 23.926
- type: mrr_at_10
value: 36.525999999999996
- type: mrr_at_100
value: 37.627
- type: mrr_at_1000
value: 37.665
- type: mrr_at_3
value: 32.653
- type: mrr_at_5
value: 34.897
- type: ndcg_at_1
value: 23.910999999999998
- type: ndcg_at_10
value: 42.927
- type: ndcg_at_100
value: 48.464
- type: ndcg_at_1000
value: 49.533
- type: ndcg_at_3
value: 34.910000000000004
- type: ndcg_at_5
value: 38.937
- type: precision_at_1
value: 23.910999999999998
- type: precision_at_10
value: 6.758
- type: precision_at_100
value: 0.9520000000000001
- type: precision_at_1000
value: 0.104
- type: precision_at_3
value: 14.838000000000001
- type: precision_at_5
value: 10.934000000000001
- type: recall_at_1
value: 23.179
- type: recall_at_10
value: 64.622
- type: recall_at_100
value: 90.135
- type: recall_at_1000
value: 98.301
- type: recall_at_3
value: 42.836999999999996
- type: recall_at_5
value: 52.512
- task:
type: Classification
dataset:
type: mteb/mtop_domain
name: MTEB MTOPDomainClassification (en)
config: en
split: test
revision: d80d48c1eb48d3562165c59d59d0034df9fff0bf
metrics:
- type: accuracy
value: 96.59598723210215
- type: f1
value: 96.41913500001952
- task:
type: Classification
dataset:
type: mteb/mtop_intent
name: MTEB MTOPIntentClassification (en)
config: en
split: test
revision: ae001d0e6b1228650b7bd1c2c65fb50ad11a8aba
metrics:
- type: accuracy
value: 82.89557683538533
- type: f1
value: 63.379319722356264
- task:
type: Classification
dataset:
type: mteb/amazon_massive_intent
name: MTEB MassiveIntentClassification (en)
config: en
split: test
revision: 31efe3c427b0bae9c22cbb560b8f15491cc6bed7
metrics:
- type: accuracy
value: 78.93745796906524
- type: f1
value: 75.71616541785902
- task:
type: Classification
dataset:
type: mteb/amazon_massive_scenario
name: MTEB MassiveScenarioClassification (en)
config: en
split: test
revision: 7d571f92784cd94a019292a1f45445077d0ef634
metrics:
- type: accuracy
value: 81.41223940820443
- type: f1
value: 81.2877893719078
- task:
type: Clustering
dataset:
type: mteb/medrxiv-clustering-p2p
name: MTEB MedrxivClusteringP2P
config: default
split: test
revision: e7a26af6f3ae46b30dde8737f02c07b1505bcc73
metrics:
- type: v_measure
value: 35.03682528325662
- task:
type: Clustering
dataset:
type: mteb/medrxiv-clustering-s2s
name: MTEB MedrxivClusteringS2S
config: default
split: test
revision: 35191c8c0dca72d8ff3efcd72aa802307d469663
metrics:
- type: v_measure
value: 32.942529406124
- task:
type: Reranking
dataset:
type: mteb/mind_small
name: MTEB MindSmallReranking
config: default
split: test
revision: 3bdac13927fdc888b903db93b2ffdbd90b295a69
metrics:
- type: map
value: 31.459949660460317
- type: mrr
value: 32.70509582031616
- task:
type: Retrieval
dataset:
type: mteb/nfcorpus
name: MTEB NFCorpus
config: default
split: test
revision: ec0fa4fe99da2ff19ca1214b7966684033a58814
metrics:
- type: map_at_1
value: 6.497
- type: map_at_10
value: 13.843
- type: map_at_100
value: 17.713
- type: map_at_1000
value: 19.241
- type: map_at_3
value: 10.096
- type: map_at_5
value: 11.85
- type: mrr_at_1
value: 48.916
- type: mrr_at_10
value: 57.764
- type: mrr_at_100
value: 58.251
- type: mrr_at_1000
value: 58.282999999999994
- type: mrr_at_3
value: 55.623999999999995
- type: mrr_at_5
value: 57.018
- type: ndcg_at_1
value: 46.594
- type: ndcg_at_10
value: 36.945
- type: ndcg_at_100
value: 34.06
- type: ndcg_at_1000
value: 43.05
- type: ndcg_at_3
value: 41.738
- type: ndcg_at_5
value: 39.330999999999996
- type: precision_at_1
value: 48.916
- type: precision_at_10
value: 27.43
- type: precision_at_100
value: 8.616
- type: precision_at_1000
value: 2.155
- type: precision_at_3
value: 39.112
- type: precision_at_5
value: 33.808
- type: recall_at_1
value: 6.497
- type: recall_at_10
value: 18.163
- type: recall_at_100
value: 34.566
- type: recall_at_1000
value: 67.15
- type: recall_at_3
value: 11.100999999999999
- type: recall_at_5
value: 14.205000000000002
- task:
type: Retrieval
dataset:
type: mteb/nq
name: MTEB NQ
config: default
split: test
revision: b774495ed302d8c44a3a7ea25c90dbce03968f31
metrics:
- type: map_at_1
value: 31.916
- type: map_at_10
value: 48.123
- type: map_at_100
value: 49.103
- type: map_at_1000
value: 49.131
- type: map_at_3
value: 43.711
- type: map_at_5
value: 46.323
- type: mrr_at_1
value: 36.181999999999995
- type: mrr_at_10
value: 50.617999999999995
- type: mrr_at_100
value: 51.329
- type: mrr_at_1000
value: 51.348000000000006
- type: mrr_at_3
value: 47.010999999999996
- type: mrr_at_5
value: 49.175000000000004
- type: ndcg_at_1
value: 36.181999999999995
- type: ndcg_at_10
value: 56.077999999999996
- type: ndcg_at_100
value: 60.037
- type: ndcg_at_1000
value: 60.63499999999999
- type: ndcg_at_3
value: 47.859
- type: ndcg_at_5
value: 52.178999999999995
- type: precision_at_1
value: 36.181999999999995
- type: precision_at_10
value: 9.284
- type: precision_at_100
value: 1.149
- type: precision_at_1000
value: 0.121
- type: precision_at_3
value: 22.006999999999998
- type: precision_at_5
value: 15.695
- type: recall_at_1
value: 31.916
- type: recall_at_10
value: 77.771
- type: recall_at_100
value: 94.602
- type: recall_at_1000
value: 98.967
- type: recall_at_3
value: 56.528
- type: recall_at_5
value: 66.527
- task:
type: Retrieval
dataset:
type: mteb/quora
name: MTEB QuoraRetrieval
config: default
split: test
revision: None
metrics:
- type: map_at_1
value: 71.486
- type: map_at_10
value: 85.978
- type: map_at_100
value: 86.587
- type: map_at_1000
value: 86.598
- type: map_at_3
value: 83.04899999999999
- type: map_at_5
value: 84.857
- type: mrr_at_1
value: 82.32000000000001
- type: mrr_at_10
value: 88.64
- type: mrr_at_100
value: 88.702
- type: mrr_at_1000
value: 88.702
- type: mrr_at_3
value: 87.735
- type: mrr_at_5
value: 88.36
- type: ndcg_at_1
value: 82.34
- type: ndcg_at_10
value: 89.67
- type: ndcg_at_100
value: 90.642
- type: ndcg_at_1000
value: 90.688
- type: ndcg_at_3
value: 86.932
- type: ndcg_at_5
value: 88.408
- type: precision_at_1
value: 82.34
- type: precision_at_10
value: 13.675999999999998
- type: precision_at_100
value: 1.544
- type: precision_at_1000
value: 0.157
- type: precision_at_3
value: 38.24
- type: precision_at_5
value: 25.068
- type: recall_at_1
value: 71.486
- type: recall_at_10
value: 96.844
- type: recall_at_100
value: 99.843
- type: recall_at_1000
value: 99.996
- type: recall_at_3
value: 88.92099999999999
- type: recall_at_5
value: 93.215
- task:
type: Clustering
dataset:
type: mteb/reddit-clustering
name: MTEB RedditClustering
config: default
split: test
revision: 24640382cdbf8abc73003fb0fa6d111a705499eb
metrics:
- type: v_measure
value: 59.75758437908334
- task:
type: Clustering
dataset:
type: mteb/reddit-clustering-p2p
name: MTEB RedditClusteringP2P
config: default
split: test
revision: 282350215ef01743dc01b456c7f5241fa8937f16
metrics:
- type: v_measure
value: 68.03497914092789
- task:
type: Retrieval
dataset:
type: mteb/scidocs
name: MTEB SCIDOCS
config: default
split: test
revision: None
metrics:
- type: map_at_1
value: 5.808
- type: map_at_10
value: 16.059
- type: map_at_100
value: 19.048000000000002
- type: map_at_1000
value: 19.43
- type: map_at_3
value: 10.953
- type: map_at_5
value: 13.363
- type: mrr_at_1
value: 28.7
- type: mrr_at_10
value: 42.436
- type: mrr_at_100
value: 43.599
- type: mrr_at_1000
value: 43.62
- type: mrr_at_3
value: 38.45
- type: mrr_at_5
value: 40.89
- type: ndcg_at_1
value: 28.7
- type: ndcg_at_10
value: 26.346000000000004
- type: ndcg_at_100
value: 36.758
- type: ndcg_at_1000
value: 42.113
- type: ndcg_at_3
value: 24.254
- type: ndcg_at_5
value: 21.506
- type: precision_at_1
value: 28.7
- type: precision_at_10
value: 13.969999999999999
- type: precision_at_100
value: 2.881
- type: precision_at_1000
value: 0.414
- type: precision_at_3
value: 22.933
- type: precision_at_5
value: 19.220000000000002
- type: recall_at_1
value: 5.808
- type: recall_at_10
value: 28.310000000000002
- type: recall_at_100
value: 58.475
- type: recall_at_1000
value: 84.072
- type: recall_at_3
value: 13.957
- type: recall_at_5
value: 19.515
- task:
type: STS
dataset:
type: mteb/sickr-sts
name: MTEB SICK-R
config: default
split: test
revision: a6ea5a8cab320b040a23452cc28066d9beae2cee
metrics:
- type: cos_sim_pearson
value: 82.39274129958557
- type: cos_sim_spearman
value: 79.78021235170053
- type: euclidean_pearson
value: 79.35335401300166
- type: euclidean_spearman
value: 79.7271870968275
- type: manhattan_pearson
value: 79.35256263340601
- type: manhattan_spearman
value: 79.76036386976321
- task:
type: STS
dataset:
type: mteb/sts12-sts
name: MTEB STS12
config: default
split: test
revision: a0d554a64d88156834ff5ae9920b964011b16384
metrics:
- type: cos_sim_pearson
value: 83.99130429246708
- type: cos_sim_spearman
value: 73.88322811171203
- type: euclidean_pearson
value: 80.7569419170376
- type: euclidean_spearman
value: 73.82542155409597
- type: manhattan_pearson
value: 80.79468183847625
- type: manhattan_spearman
value: 73.87027144047784
- task:
type: STS
dataset:
type: mteb/sts13-sts
name: MTEB STS13
config: default
split: test
revision: 7e90230a92c190f1bf69ae9002b8cea547a64cca
metrics:
- type: cos_sim_pearson
value: 84.88548789489907
- type: cos_sim_spearman
value: 85.07535893847255
- type: euclidean_pearson
value: 84.6637222061494
- type: euclidean_spearman
value: 85.14200626702456
- type: manhattan_pearson
value: 84.75327892344734
- type: manhattan_spearman
value: 85.24406181838596
- task:
type: STS
dataset:
type: mteb/sts14-sts
name: MTEB STS14
config: default
split: test
revision: 6031580fec1f6af667f0bd2da0a551cf4f0b2375
metrics:
- type: cos_sim_pearson
value: 82.88140039325008
- type: cos_sim_spearman
value: 79.61211268112362
- type: euclidean_pearson
value: 81.29639728816458
- type: euclidean_spearman
value: 79.51284578041442
- type: manhattan_pearson
value: 81.3381797137111
- type: manhattan_spearman
value: 79.55683684039808
- task:
type: STS
dataset:
type: mteb/sts15-sts
name: MTEB STS15
config: default
split: test
revision: ae752c7c21bf194d8b67fd573edf7ae58183cbe3
metrics:
- type: cos_sim_pearson
value: 85.16716737270485
- type: cos_sim_spearman
value: 86.14823841857738
- type: euclidean_pearson
value: 85.36325733440725
- type: euclidean_spearman
value: 86.04919691402029
- type: manhattan_pearson
value: 85.3147511385052
- type: manhattan_spearman
value: 86.00676205857764
- task:
type: STS
dataset:
type: mteb/sts16-sts
name: MTEB STS16
config: default
split: test
revision: 4d8694f8f0e0100860b497b999b3dbed754a0513
metrics:
- type: cos_sim_pearson
value: 80.34266645861588
- type: cos_sim_spearman
value: 81.59914035005882
- type: euclidean_pearson
value: 81.15053076245988
- type: euclidean_spearman
value: 81.52776915798489
- type: manhattan_pearson
value: 81.1819647418673
- type: manhattan_spearman
value: 81.57479527353556
- task:
type: STS
dataset:
type: mteb/sts17-crosslingual-sts
name: MTEB STS17 (en-en)
config: en-en
split: test
revision: af5e6fb845001ecf41f4c1e033ce921939a2a68d
metrics:
- type: cos_sim_pearson
value: 89.38263326821439
- type: cos_sim_spearman
value: 89.10946308202642
- type: euclidean_pearson
value: 88.87831312540068
- type: euclidean_spearman
value: 89.03615865973664
- type: manhattan_pearson
value: 88.79835539970384
- type: manhattan_spearman
value: 88.9766156339753
- task:
type: STS
dataset:
type: mteb/sts22-crosslingual-sts
name: MTEB STS22 (en)
config: en
split: test
revision: eea2b4fe26a775864c896887d910b76a8098ad3f
metrics:
- type: cos_sim_pearson
value: 70.1574915581685
- type: cos_sim_spearman
value: 70.59144980004054
- type: euclidean_pearson
value: 71.43246306918755
- type: euclidean_spearman
value: 70.5544189562984
- type: manhattan_pearson
value: 71.4071414609503
- type: manhattan_spearman
value: 70.31799126163712
- task:
type: STS
dataset:
type: mteb/stsbenchmark-sts
name: MTEB STSBenchmark
config: default
split: test
revision: b0fddb56ed78048fa8b90373c8a3cfc37b684831
metrics:
- type: cos_sim_pearson
value: 83.36215796635351
- type: cos_sim_spearman
value: 83.07276756467208
- type: euclidean_pearson
value: 83.06690453635584
- type: euclidean_spearman
value: 82.9635366303289
- type: manhattan_pearson
value: 83.04994049700815
- type: manhattan_spearman
value: 82.98120125356036
- task:
type: Reranking
dataset:
type: mteb/scidocs-reranking
name: MTEB SciDocsRR
config: default
split: test
revision: d3c5e1fc0b855ab6097bf1cda04dd73947d7caab
metrics:
- type: map
value: 86.92530011616722
- type: mrr
value: 96.21826793395421
- task:
type: Retrieval
dataset:
type: mteb/scifact
name: MTEB SciFact
config: default
split: test
revision: 0228b52cf27578f30900b9e5271d331663a030d7
metrics:
- type: map_at_1
value: 65.75
- type: map_at_10
value: 77.701
- type: map_at_100
value: 78.005
- type: map_at_1000
value: 78.006
- type: map_at_3
value: 75.48
- type: map_at_5
value: 76.927
- type: mrr_at_1
value: 68.333
- type: mrr_at_10
value: 78.511
- type: mrr_at_100
value: 78.704
- type: mrr_at_1000
value: 78.704
- type: mrr_at_3
value: 77
- type: mrr_at_5
value: 78.083
- type: ndcg_at_1
value: 68.333
- type: ndcg_at_10
value: 82.42699999999999
- type: ndcg_at_100
value: 83.486
- type: ndcg_at_1000
value: 83.511
- type: ndcg_at_3
value: 78.96300000000001
- type: ndcg_at_5
value: 81.028
- type: precision_at_1
value: 68.333
- type: precision_at_10
value: 10.667
- type: precision_at_100
value: 1.127
- type: precision_at_1000
value: 0.11299999999999999
- type: precision_at_3
value: 31.333
- type: precision_at_5
value: 20.133000000000003
- type: recall_at_1
value: 65.75
- type: recall_at_10
value: 95.578
- type: recall_at_100
value: 99.833
- type: recall_at_1000
value: 100
- type: recall_at_3
value: 86.506
- type: recall_at_5
value: 91.75
- task:
type: PairClassification
dataset:
type: mteb/sprintduplicatequestions-pairclassification
name: MTEB SprintDuplicateQuestions
config: default
split: test
revision: d66bd1f72af766a5cc4b0ca5e00c162f89e8cc46
metrics:
- type: cos_sim_accuracy
value: 99.75247524752476
- type: cos_sim_ap
value: 94.16065078045173
- type: cos_sim_f1
value: 87.22986247544205
- type: cos_sim_precision
value: 85.71428571428571
- type: cos_sim_recall
value: 88.8
- type: dot_accuracy
value: 99.74554455445545
- type: dot_ap
value: 93.90633887037264
- type: dot_f1
value: 86.9873417721519
- type: dot_precision
value: 88.1025641025641
- type: dot_recall
value: 85.9
- type: euclidean_accuracy
value: 99.75247524752476
- type: euclidean_ap
value: 94.17466319018055
- type: euclidean_f1
value: 87.3405299313052
- type: euclidean_precision
value: 85.74181117533719
- type: euclidean_recall
value: 89
- type: manhattan_accuracy
value: 99.75445544554455
- type: manhattan_ap
value: 94.27688371923577
- type: manhattan_f1
value: 87.74002954209749
- type: manhattan_precision
value: 86.42095053346266
- type: manhattan_recall
value: 89.1
- type: max_accuracy
value: 99.75445544554455
- type: max_ap
value: 94.27688371923577
- type: max_f1
value: 87.74002954209749
- task:
type: Clustering
dataset:
type: mteb/stackexchange-clustering
name: MTEB StackExchangeClustering
config: default
split: test
revision: 6cbc1f7b2bc0622f2e39d2c77fa502909748c259
metrics:
- type: v_measure
value: 71.26500637517056
- task:
type: Clustering
dataset:
type: mteb/stackexchange-clustering-p2p
name: MTEB StackExchangeClusteringP2P
config: default
split: test
revision: 815ca46b2622cec33ccafc3735d572c266efdb44
metrics:
- type: v_measure
value: 39.17507906280528
- task:
type: Reranking
dataset:
type: mteb/stackoverflowdupquestions-reranking
name: MTEB StackOverflowDupQuestions
config: default
split: test
revision: e185fbe320c72810689fc5848eb6114e1ef5ec69
metrics:
- type: map
value: 52.4848744828509
- type: mrr
value: 53.33678168236992
- task:
type: Summarization
dataset:
type: mteb/summeval
name: MTEB SummEval
config: default
split: test
revision: cda12ad7615edc362dbf25a00fdd61d3b1eaf93c
metrics:
- type: cos_sim_pearson
value: 30.599864323827887
- type: cos_sim_spearman
value: 30.91116204665598
- type: dot_pearson
value: 30.82637894269936
- type: dot_spearman
value: 30.957573868416066
- task:
type: Retrieval
dataset:
type: mteb/trec-covid
name: MTEB TRECCOVID
config: default
split: test
revision: None
metrics:
- type: map_at_1
value: 0.23600000000000002
- type: map_at_10
value: 1.892
- type: map_at_100
value: 11.586
- type: map_at_1000
value: 27.761999999999997
- type: map_at_3
value: 0.653
- type: map_at_5
value: 1.028
- type: mrr_at_1
value: 88
- type: mrr_at_10
value: 94
- type: mrr_at_100
value: 94
- type: mrr_at_1000
value: 94
- type: mrr_at_3
value: 94
- type: mrr_at_5
value: 94
- type: ndcg_at_1
value: 82
- type: ndcg_at_10
value: 77.48899999999999
- type: ndcg_at_100
value: 60.141
- type: ndcg_at_1000
value: 54.228
- type: ndcg_at_3
value: 82.358
- type: ndcg_at_5
value: 80.449
- type: precision_at_1
value: 88
- type: precision_at_10
value: 82.19999999999999
- type: precision_at_100
value: 61.760000000000005
- type: precision_at_1000
value: 23.684
- type: precision_at_3
value: 88
- type: precision_at_5
value: 85.6
- type: recall_at_1
value: 0.23600000000000002
- type: recall_at_10
value: 2.117
- type: recall_at_100
value: 14.985000000000001
- type: recall_at_1000
value: 51.107
- type: recall_at_3
value: 0.688
- type: recall_at_5
value: 1.1039999999999999
- task:
type: Retrieval
dataset:
type: mteb/touche2020
name: MTEB Touche2020
config: default
split: test
revision: a34f9a33db75fa0cbb21bb5cfc3dae8dc8bec93f
metrics:
- type: map_at_1
value: 2.3040000000000003
- type: map_at_10
value: 9.025
- type: map_at_100
value: 15.312999999999999
- type: map_at_1000
value: 16.954
- type: map_at_3
value: 4.981
- type: map_at_5
value: 6.32
- type: mrr_at_1
value: 24.490000000000002
- type: mrr_at_10
value: 39.835
- type: mrr_at_100
value: 40.8
- type: mrr_at_1000
value: 40.8
- type: mrr_at_3
value: 35.034
- type: mrr_at_5
value: 37.687
- type: ndcg_at_1
value: 22.448999999999998
- type: ndcg_at_10
value: 22.545
- type: ndcg_at_100
value: 35.931999999999995
- type: ndcg_at_1000
value: 47.665
- type: ndcg_at_3
value: 23.311
- type: ndcg_at_5
value: 22.421
- type: precision_at_1
value: 24.490000000000002
- type: precision_at_10
value: 20.408
- type: precision_at_100
value: 7.815999999999999
- type: precision_at_1000
value: 1.553
- type: precision_at_3
value: 25.169999999999998
- type: precision_at_5
value: 23.265
- type: recall_at_1
value: 2.3040000000000003
- type: recall_at_10
value: 15.693999999999999
- type: recall_at_100
value: 48.917
- type: recall_at_1000
value: 84.964
- type: recall_at_3
value: 6.026
- type: recall_at_5
value: 9.066
- task:
type: Classification
dataset:
type: mteb/toxic_conversations_50k
name: MTEB ToxicConversationsClassification
config: default
split: test
revision: d7c0de2777da35d6aae2200a62c6e0e5af397c4c
metrics:
- type: accuracy
value: 82.6074
- type: ap
value: 23.187467098602013
- type: f1
value: 65.36829506379657
- task:
type: Classification
dataset:
type: mteb/tweet_sentiment_extraction
name: MTEB TweetSentimentExtractionClassification
config: default
split: test
revision: d604517c81ca91fe16a244d1248fc021f9ecee7a
metrics:
- type: accuracy
value: 63.16355404640635
- type: f1
value: 63.534725639863346
- task:
type: Clustering
dataset:
type: mteb/twentynewsgroups-clustering
name: MTEB TwentyNewsgroupsClustering
config: default
split: test
revision: 6125ec4e24fa026cec8a478383ee943acfbd5449
metrics:
- type: v_measure
value: 50.91004094411276
- task:
type: PairClassification
dataset:
type: mteb/twittersemeval2015-pairclassification
name: MTEB TwitterSemEval2015
config: default
split: test
revision: 70970daeab8776df92f5ea462b6173c0b46fd2d1
metrics:
- type: cos_sim_accuracy
value: 86.55301901412649
- type: cos_sim_ap
value: 75.25312618556728
- type: cos_sim_f1
value: 68.76561719140429
- type: cos_sim_precision
value: 65.3061224489796
- type: cos_sim_recall
value: 72.61213720316623
- type: dot_accuracy
value: 86.29671574178936
- type: dot_ap
value: 75.11910195501207
- type: dot_f1
value: 68.44048376830045
- type: dot_precision
value: 66.12546125461255
- type: dot_recall
value: 70.92348284960423
- type: euclidean_accuracy
value: 86.5828217202122
- type: euclidean_ap
value: 75.22986344900924
- type: euclidean_f1
value: 68.81267797449549
- type: euclidean_precision
value: 64.8238861674831
- type: euclidean_recall
value: 73.3245382585752
- type: manhattan_accuracy
value: 86.61262442629791
- type: manhattan_ap
value: 75.24401608557328
- type: manhattan_f1
value: 68.80473982483257
- type: manhattan_precision
value: 67.21187720181177
- type: manhattan_recall
value: 70.47493403693932
- type: max_accuracy
value: 86.61262442629791
- type: max_ap
value: 75.25312618556728
- type: max_f1
value: 68.81267797449549
- task:
type: PairClassification
dataset:
type: mteb/twitterurlcorpus-pairclassification
name: MTEB TwitterURLCorpus
config: default
split: test
revision: 8b6510b0b1fa4e4c4f879467980e9be563ec1cdf
metrics:
- type: cos_sim_accuracy
value: 88.10688089416696
- type: cos_sim_ap
value: 84.17862178779863
- type: cos_sim_f1
value: 76.17305208781748
- type: cos_sim_precision
value: 71.31246641590543
- type: cos_sim_recall
value: 81.74468740375731
- type: dot_accuracy
value: 88.1844995536927
- type: dot_ap
value: 84.33816725235876
- type: dot_f1
value: 76.43554032918746
- type: dot_precision
value: 74.01557767200346
- type: dot_recall
value: 79.0190945488143
- type: euclidean_accuracy
value: 88.07001203089223
- type: euclidean_ap
value: 84.12267000814985
- type: euclidean_f1
value: 76.12232600180778
- type: euclidean_precision
value: 74.50604541433205
- type: euclidean_recall
value: 77.81028641823221
- type: manhattan_accuracy
value: 88.06419063142779
- type: manhattan_ap
value: 84.11648917164187
- type: manhattan_f1
value: 76.20579953925474
- type: manhattan_precision
value: 72.56772755762935
- type: manhattan_recall
value: 80.22790267939637
- type: max_accuracy
value: 88.1844995536927
- type: max_ap
value: 84.33816725235876
- type: max_f1
value: 76.43554032918746
---
<!-- **English** | [中文](./README_zh.md) -->
# gte-large-en-v1.5
We introduce `gte-v1.5` series, upgraded `gte` embeddings that support the context length of up to **8192**, while further enhancing model performance.
The models are built upon the `transformer++` encoder [backbone](https://huggingface.co/Alibaba-NLP/new-impl) (BERT + RoPE + GLU).
The `gte-v1.5` series achieve state-of-the-art scores on the MTEB benchmark within the same model size category and prodvide competitive on the LoCo long-context retrieval tests (refer to [Evaluation](#evaluation)).
We also present the [`gte-Qwen1.5-7B-instruct`](https://huggingface.co/Alibaba-NLP/gte-Qwen1.5-7B-instruct),
a SOTA instruction-tuned multi-lingual embedding model that ranked 2nd in MTEB and 1st in C-MTEB.
<!-- Provide a longer summary of what this model is. -->
- **Developed by:** Institute for Intelligent Computing, Alibaba Group
- **Model type:** Text Embeddings
- **Paper:** [mGTE: Generalized Long-Context Text Representation and Reranking
Models for Multilingual Text Retrieval](https://arxiv.org/pdf/2407.19669)
<!-- - **Demo [optional]:** [More Information Needed] -->
### Model list
| Models | Language | Model Size | Max Seq. Length | Dimension | MTEB-en | LoCo |
|:-----: | :-----: |:-----: |:-----: |:-----: | :-----: | :-----: |
|[`gte-Qwen1.5-7B-instruct`](https://huggingface.co/Alibaba-NLP/gte-Qwen1.5-7B-instruct)| Multiple | 7720 | 32768 | 4096 | 67.34 | 87.57 |
|[`gte-large-en-v1.5`](https://huggingface.co/Alibaba-NLP/gte-large-en-v1.5) | English | 434 | 8192 | 1024 | 65.39 | 86.71 |
|[`gte-base-en-v1.5`](https://huggingface.co/Alibaba-NLP/gte-base-en-v1.5) | English | 137 | 8192 | 768 | 64.11 | 87.44 |
## How to Get Started with the Model
Use the code below to get started with the model.
```python
# Requires transformers>=4.36.0
import torch.nn.functional as F
from transformers import AutoModel, AutoTokenizer
input_texts = [
"what is the capital of China?",
"how to implement quick sort in python?",
"Beijing",
"sorting algorithms"
]
model_path = 'Alibaba-NLP/gte-large-en-v1.5'
tokenizer = AutoTokenizer.from_pretrained(model_path)
model = AutoModel.from_pretrained(model_path, trust_remote_code=True)
# Tokenize the input texts
batch_dict = tokenizer(input_texts, max_length=8192, padding=True, truncation=True, return_tensors='pt')
outputs = model(**batch_dict)
embeddings = outputs.last_hidden_state[:, 0]
# (Optionally) normalize embeddings
embeddings = F.normalize(embeddings, p=2, dim=1)
scores = (embeddings[:1] @ embeddings[1:].T) * 100
print(scores.tolist())
```
**It is recommended to install xformers and enable unpadding for acceleration, refer to [enable-unpadding-and-xformers](https://huggingface.co/Alibaba-NLP/new-impl#recommendation-enable-unpadding-and-acceleration-with-xformers).**
Use with sentence-transformers:
```python
# Requires sentence_transformers>=2.7.0
from sentence_transformers import SentenceTransformer
from sentence_transformers.util import cos_sim
sentences = ['That is a happy person', 'That is a very happy person']
model = SentenceTransformer('Alibaba-NLP/gte-large-en-v1.5', trust_remote_code=True)
embeddings = model.encode(sentences)
print(cos_sim(embeddings[0], embeddings[1]))
```
Use with `transformers.js`:
```js
// npm i @xenova/transformers
import { pipeline, dot } from '@xenova/transformers';
// Create feature extraction pipeline
const extractor = await pipeline('feature-extraction', 'Alibaba-NLP/gte-large-en-v1.5', {
quantized: false, // Comment out this line to use the quantized version
});
// Generate sentence embeddings
const sentences = [
"what is the capital of China?",
"how to implement quick sort in python?",
"Beijing",
"sorting algorithms"
]
const output = await extractor(sentences, { normalize: true, pooling: 'cls' });
// Compute similarity scores
const [source_embeddings, ...document_embeddings ] = output.tolist();
const similarities = document_embeddings.map(x => 100 * dot(source_embeddings, x));
console.log(similarities); // [41.86354093370361, 77.07076371259589, 37.02981979677899]
```
## Training Details
### Training Data
- Masked language modeling (MLM): `c4-en`
- Weak-supervised contrastive pre-training (CPT): [GTE](https://arxiv.org/pdf/2308.03281.pdf) pre-training data
- Supervised contrastive fine-tuning: [GTE](https://arxiv.org/pdf/2308.03281.pdf) fine-tuning data
### Training Procedure
To enable the backbone model to support a context length of 8192, we adopted a multi-stage training strategy.
The model first undergoes preliminary MLM pre-training on shorter lengths.
And then, we resample the data, reducing the proportion of short texts, and continue the MLM pre-training.
The entire training process is as follows:
- MLM-512: lr 2e-4, mlm_probability 0.3, batch_size 4096, num_steps 300000, rope_base 10000
- MLM-2048: lr 5e-5, mlm_probability 0.3, batch_size 4096, num_steps 30000, rope_base 10000
- [MLM-8192](https://huggingface.co/Alibaba-NLP/gte-en-mlm-large): lr 5e-5, mlm_probability 0.3, batch_size 1024, num_steps 30000, rope_base 160000
- CPT: max_len 512, lr 5e-5, batch_size 28672, num_steps 100000
- Fine-tuning: TODO
## Evaluation
### MTEB
The results of other models are retrieved from [MTEB leaderboard](https://huggingface.co/spaces/mteb/leaderboard).
The gte evaluation setting: `mteb==1.2.0, fp16 auto mix precision, max_length=8192`, and set ntk scaling factor to 2 (equivalent to rope_base * 2).
| Model Name | Param Size (M) | Dimension | Sequence Length | Average (56) | Class. (12) | Clust. (11) | Pair Class. (3) | Reran. (4) | Retr. (15) | STS (10) | Summ. (1) |
|:----:|:---:|:---:|:---:|:---:|:---:|:---:|:---:|:---:|:---:|:---:|:---:|
| [**gte-large-en-v1.5**](https://huggingface.co/Alibaba-NLP/gte-large-en-v1.5) | 409 | 1024 | 8192 | **65.39** | 77.75 | 47.95 | 84.63 | 58.50 | 57.91 | 81.43 | 30.91 |
| [mxbai-embed-large-v1](https://huggingface.co/mixedbread-ai/mxbai-embed-large-v1) | 335 | 1024 | 512 | 64.68 | 75.64 | 46.71 | 87.2 | 60.11 | 54.39 | 85 | 32.71 |
| [multilingual-e5-large-instruct](https://huggingface.co/intfloat/multilingual-e5-large-instruct) | 560 | 1024 | 514 | 64.41 | 77.56 | 47.1 | 86.19 | 58.58 | 52.47 | 84.78 | 30.39 |
| [bge-large-en-v1.5](https://huggingface.co/BAAI/bge-large-en-v1.5)| 335 | 1024 | 512 | 64.23 | 75.97 | 46.08 | 87.12 | 60.03 | 54.29 | 83.11 | 31.61 |
| [**gte-base-en-v1.5**](https://huggingface.co/Alibaba-NLP/gte-base-en-v1.5) | 137 | 768 | 8192 | **64.11** | 77.17 | 46.82 | 85.33 | 57.66 | 54.09 | 81.97 | 31.17 |
| [bge-base-en-v1.5](https://huggingface.co/BAAI/bge-base-en-v1.5)| 109 | 768 | 512 | 63.55 | 75.53 | 45.77 | 86.55 | 58.86 | 53.25 | 82.4 | 31.07 |
### LoCo
| Model Name | Dimension | Sequence Length | Average (5) | QsmsumRetrieval | SummScreenRetrieval | QasperAbastractRetrieval | QasperTitleRetrieval | GovReportRetrieval |
|:----:|:---:|:---:|:---:|:---:|:---:|:---:|:---:|:---:|
| [gte-qwen1.5-7b](https://huggingface.co/Alibaba-NLP/gte-qwen1.5-7b) | 4096 | 32768 | 87.57 | 49.37 | 93.10 | 99.67 | 97.54 | 98.21 |
| [gte-large-v1.5](https://huggingface.co/Alibaba-NLP/gte-large-v1.5) |1024 | 8192 | 86.71 | 44.55 | 92.61 | 99.82 | 97.81 | 98.74 |
| [gte-base-v1.5](https://huggingface.co/Alibaba-NLP/gte-base-v1.5) | 768 | 8192 | 87.44 | 49.91 | 91.78 | 99.82 | 97.13 | 98.58 |
## Citation
If you find our paper or models helpful, please consider citing them as follows:
```
@article{zhang2024mgte,
title={mGTE: Generalized Long-Context Text Representation and Reranking Models for Multilingual Text Retrieval},
author={Zhang, Xin and Zhang, Yanzhao and Long, Dingkun and Xie, Wen and Dai, Ziqi and Tang, Jialong and Lin, Huan and Yang, Baosong and Xie, Pengjun and Huang, Fei and others},
journal={arXiv preprint arXiv:2407.19669},
year={2024}
}
@article{li2023towards,
title={Towards general text embeddings with multi-stage contrastive learning},
author={Li, Zehan and Zhang, Xin and Zhang, Yanzhao and Long, Dingkun and Xie, Pengjun and Zhang, Meishan},
journal={arXiv preprint arXiv:2308.03281},
year={2023}
}
``` |
pyannote/speaker-diarization-3.0 | pyannote | "2024-05-10T19:37:39Z" | 2,686,530 | 167 | pyannote-audio | [
"pyannote-audio",
"pyannote",
"pyannote-audio-pipeline",
"audio",
"voice",
"speech",
"speaker",
"speaker-diarization",
"speaker-change-detection",
"voice-activity-detection",
"overlapped-speech-detection",
"automatic-speech-recognition",
"arxiv:2111.14448",
"arxiv:2012.01477",
"license:mit",
"region:us"
] | automatic-speech-recognition | "2023-09-22T13:40:36Z" | ---
tags:
- pyannote
- pyannote-audio
- pyannote-audio-pipeline
- audio
- voice
- speech
- speaker
- speaker-diarization
- speaker-change-detection
- voice-activity-detection
- overlapped-speech-detection
- automatic-speech-recognition
license: mit
extra_gated_prompt: "The collected information will help acquire a better knowledge of pyannote.audio userbase and help its maintainers improve it further. Though this pipeline uses MIT license and will always remain open-source, we will occasionnally email you about premium pipelines and paid services around pyannote."
extra_gated_fields:
Company/university: text
Website: text
---
Using this open-source model in production?
Consider switching to [pyannoteAI](https://www.pyannote.ai) for better and faster options.
# 🎹 Speaker diarization 3.0
This pipeline has been trained by Séverin Baroudi with [pyannote.audio](https://github.com/pyannote/pyannote-audio) `3.0.0` using a combination of the training sets of AISHELL, AliMeeting, AMI, AVA-AVD, DIHARD, Ego4D, MSDWild, REPERE, and VoxConverse.
It ingests mono audio sampled at 16kHz and outputs speaker diarization as an [`Annotation`](http://pyannote.github.io/pyannote-core/structure.html#annotation) instance:
* stereo or multi-channel audio files are automatically downmixed to mono by averaging the channels.
* audio files sampled at a different rate are resampled to 16kHz automatically upon loading.
## Requirements
1. Install [`pyannote.audio`](https://github.com/pyannote/pyannote-audio) `3.0` with `pip install pyannote.audio`
2. Accept [`pyannote/segmentation-3.0`](https://hf.co/pyannote/segmentation-3.0) user conditions
3. Accept [`pyannote/speaker-diarization-3.0`](https://hf.co/pyannote-speaker-diarization-3.0) user conditions
4. Create access token at [`hf.co/settings/tokens`](https://hf.co/settings/tokens).
## Usage
```python
# instantiate the pipeline
from pyannote.audio import Pipeline
pipeline = Pipeline.from_pretrained(
"pyannote/speaker-diarization-3.0",
use_auth_token="HUGGINGFACE_ACCESS_TOKEN_GOES_HERE")
# run the pipeline on an audio file
diarization = pipeline("audio.wav")
# dump the diarization output to disk using RTTM format
with open("audio.rttm", "w") as rttm:
diarization.write_rttm(rttm)
```
### Processing on GPU
`pyannote.audio` pipelines run on CPU by default.
You can send them to GPU with the following lines:
```python
import torch
pipeline.to(torch.device("cuda"))
```
Real-time factor is around 2.5% using one Nvidia Tesla V100 SXM2 GPU (for the neural inference part) and one Intel Cascade Lake 6248 CPU (for the clustering part).
In other words, it takes approximately 1.5 minutes to process a one hour conversation.
### Processing from memory
Pre-loading audio files in memory may result in faster processing:
```python
waveform, sample_rate = torchaudio.load("audio.wav")
diarization = pipeline({"waveform": waveform, "sample_rate": sample_rate})
```
### Monitoring progress
Hooks are available to monitor the progress of the pipeline:
```python
from pyannote.audio.pipelines.utils.hook import ProgressHook
with ProgressHook() as hook:
diarization = pipeline("audio.wav", hook=hook)
```
### Controlling the number of speakers
In case the number of speakers is known in advance, one can use the `num_speakers` option:
```python
diarization = pipeline("audio.wav", num_speakers=2)
```
One can also provide lower and/or upper bounds on the number of speakers using `min_speakers` and `max_speakers` options:
```python
diarization = pipeline("audio.wav", min_speakers=2, max_speakers=5)
```
## Benchmark
This pipeline has been benchmarked on a large collection of datasets.
Processing is fully automatic:
* no manual voice activity detection (as is sometimes the case in the literature)
* no manual number of speakers (though it is possible to provide it to the pipeline)
* no fine-tuning of the internal models nor tuning of the pipeline hyper-parameters to each dataset
... with the least forgiving diarization error rate (DER) setup (named *"Full"* in [this paper](https://doi.org/10.1016/j.csl.2021.101254)):
* no forgiveness collar
* evaluation of overlapped speech
| Benchmark | [DER%](. "Diarization error rate") | [FA%](. "False alarm rate") | [Miss%](. "Missed detection rate") | [Conf%](. "Speaker confusion rate") | Expected output | File-level evaluation |
| ------------------------------------------------------------------------------------------------------------------------------------------- | ---------------------------------- | --------------------------- | ---------------------------------- | ----------------------------------- | ----------------------------------------------------------------------------------------------------------------------------- | ----------------------------------------------------------------------------------------------------------------------------- |
| [AISHELL-4](http://www.openslr.org/111/) | 12.3 | 3.8 | 4.4 | 4.1 | [RTTM](https://huggingface.co/pyannote/speaker-diarization-3.0.0/blob/main/reproducible_research/AISHELL.SpeakerDiarization.Benchmark.test.rttm) | [eval](https://huggingface.co/pyannote/speaker-diarization-3.0.0/blob/main/reproducible_research/AISHELL.SpeakerDiarization.Benchmark.test.eval) |
| [AliMeeting (*channel 1*)](https://www.openslr.org/119/) | 24.3 | 4.4 | 10.0 | 9.9 | [RTTM](https://huggingface.co/pyannote/speaker-diarization-3.0.0/blob/main/reproducible_research/AliMeeting.SpeakerDiarization.Benchmark.test.rttm) | [eval](https://huggingface.co/pyannote/speaker-diarization-3.0.0/blob/main/reproducible_research/AliMeeting.SpeakerDiarization.Benchmark.test.eval) |
| [AMI (*headset mix,*](https://groups.inf.ed.ac.uk/ami/corpus/) [*only_words*)](https://github.com/BUTSpeechFIT/AMI-diarization-setup) | 19.0 | 3.6 | 9.5 | 5.9 | [RTTM](https://huggingface.co/pyannote/speaker-diarization-3.0.0/blob/main/reproducible_research/AMI.SpeakerDiarization.Benchmark.test.rttm) | [eval](https://huggingface.co/pyannote/speaker-diarization-3.0.0/blob/main/reproducible_research/AMI.SpeakerDiarization.Benchmark.test.eval) |
| [AMI (*array1, channel 1,*](https://groups.inf.ed.ac.uk/ami/corpus/) [*only_words)*](https://github.com/BUTSpeechFIT/AMI-diarization-setup) | 22.2 | 3.8 | 11.2 | 7.3 | [RTTM](https://huggingface.co/pyannote/speaker-diarization-3.0.0/blob/main/reproducible_research/AMI-SDM.SpeakerDiarization.Benchmark.test.rttm) | [eval](https://huggingface.co/pyannote/speaker-diarization-3.0.0/blob/main/reproducible_research/AMI-SDM.SpeakerDiarization.Benchmark.test.eval) |
| [AVA-AVD](https://arxiv.org/abs/2111.14448) | 49.1 | 10.8 | 15.7| 22.5 | [RTTM](https://huggingface.co/pyannote/speaker-diarization-3.0.0/blob/main/reproducible_research/AVA-AVD.SpeakerDiarization.Benchmark.test.rttm) | [eval](https://huggingface.co/pyannote/speaker-diarization-3.0.0/blob/main/reproducible_research/AVA-AVD.SpeakerDiarization.Benchmark.test.eval) |
| [DIHARD 3 (*Full*)](https://arxiv.org/abs/2012.01477) | 21.7 | 6.2 | 8.1 | 7.3 | [RTTM](https://huggingface.co/pyannote/speaker-diarization-3.0.0/blob/main/reproducible_research/DIHARD.SpeakerDiarization.Benchmark.test.rttm) | [eval](https://huggingface.co/pyannote/speaker-diarization-3.0.0/blob/main/reproducible_research/DIHARD.SpeakerDiarization.Benchmark.test.eval) |
| [MSDWild](https://x-lance.github.io/MSDWILD/) | 24.6 | 5.8 | 8.0 | 10.7 | [RTTM](https://huggingface.co/pyannote/speaker-diarization-3.0.0/blob/main/reproducible_research/MSDWILD.SpeakerDiarization.Benchmark.test.rttm) | [eval](https://huggingface.co/pyannote/speaker-diarization-3.0.0/blob/main/reproducible_research/MSDWILD.SpeakerDiarization.Benchmark.test.eval) |
| [REPERE (*phase 2*)](https://islrn.org/resources/360-758-359-485-0/) | 7.8 | 1.8 | 2.6 | 3.5 | [RTTM](https://huggingface.co/pyannote/speaker-diarization-3.0.0/blob/main/reproducible_research/REPERE.SpeakerDiarization.Benchmark.test.rttm) | [eval](https://huggingface.co/pyannote/speaker-diarization-3.0.0/blob/main/reproducible_research/REPERE.SpeakerDiarization.Benchmark.test.eval) |
| [VoxConverse (*v0.3*)](https://github.com/joonson/voxconverse) | 11.3 | 4.1 | 3.4 | 3.8 | [RTTM](https://huggingface.co/pyannote/speaker-diarization-3.0.0/blob/main/reproducible_research/VoxConverse.SpeakerDiarization.Benchmark.test.rttm) | [eval](https://huggingface.co/pyannote/speaker-diarization-3.0.0/blob/main/reproducible_research/VoxConverse.SpeakerDiarization.Benchmark.test.eval) |
## Citations
```bibtex
@inproceedings{Plaquet23,
author={Alexis Plaquet and Hervé Bredin},
title={{Powerset multi-class cross entropy loss for neural speaker diarization}},
year=2023,
booktitle={Proc. INTERSPEECH 2023},
}
```
```bibtex
@inproceedings{Bredin23,
author={Hervé Bredin},
title={{pyannote.audio 2.1 speaker diarization pipeline: principle, benchmark, and recipe}},
year=2023,
booktitle={Proc. INTERSPEECH 2023},
}
```
|
nesaorg/fc_1 | nesaorg | "2024-08-14T12:54:24Z" | 2,686,049 | 0 | null | [
"safetensors",
"model_hub_mixin",
"pytorch_model_hub_mixin",
"region:us"
] | null | "2024-08-14T12:53:48Z" | ---
tags:
- model_hub_mixin
- pytorch_model_hub_mixin
---
This model has been pushed to the Hub using the [PytorchModelHubMixin](https://huggingface.co/docs/huggingface_hub/package_reference/mixins#huggingface_hub.PyTorchModelHubMixin) integration:
- Library: [More Information Needed]
- Docs: [More Information Needed] |
prs-eth/marigold-normals-lcm-v0-1 | prs-eth | "2024-05-09T13:56:36Z" | 2,546,423 | 6 | diffusers | [
"diffusers",
"safetensors",
"monocular normals estimation",
"single image normals estimation",
"normals",
"in-the-wild",
"zero-shot",
"LCM",
"normals-estimation",
"en",
"arxiv:2312.02145",
"license:apache-2.0",
"diffusers:MarigoldPipeline",
"region:us"
] | null | "2024-04-26T13:27:15Z" | ---
license: apache-2.0
language:
- en
pipeline_tag: normals-estimation
tags:
- monocular normals estimation
- single image normals estimation
- normals
- in-the-wild
- zero-shot
- LCM
---
# Marigold Normals (LCM) Model Card
This model belongs to the family of diffusion-based Marigold models for solving various computer vision tasks.
The Marigold Normals model focuses on the surface normals task.
It takes an input image and computes surface normals in each pixel.
The LCM stands for Latent Consistency Models, which is a technique for making the diffusion model fast.
The Marigold Normals model is trained from Stable Diffusion with synthetic data, and the LCM model is further fine-tuned from it.
Thanks to the rich visual knowledge stored in Stable Diffusion, Marigold models possess deep scene understanding and excel at solving computer vision tasks.
Read more about Marigold in our paper titled "Repurposing Diffusion-Based Image Generators for Monocular Depth Estimation".
[](https://marigoldmonodepth.github.io)
[](https://github.com/prs-eth/Marigold)
[](https://arxiv.org/abs/2312.02145)
[](https://huggingface.co/spaces/toshas/marigold)
Developed by:
[Bingxin Ke](http://www.kebingxin.com/),
[Anton Obukhov](https://www.obukhov.ai/),
[Shengyu Huang](https://shengyuh.github.io/),
[Nando Metzger](https://nandometzger.github.io/),
[Rodrigo Caye Daudt](https://rcdaudt.github.io/),
[Konrad Schindler](https://scholar.google.com/citations?user=FZuNgqIAAAAJ&hl=en)

## 🎓 Citation
```bibtex
@InProceedings{ke2023repurposing,
title={Repurposing Diffusion-Based Image Generators for Monocular Depth Estimation},
author={Bingxin Ke and Anton Obukhov and Shengyu Huang and Nando Metzger and Rodrigo Caye Daudt and Konrad Schindler},
booktitle = {Proceedings of the IEEE/CVF Conference on Computer Vision and Pattern Recognition (CVPR)},
year={2024}
}
```
## 🎫 License
This work is licensed under the Apache License, Version 2.0 (as defined in the [LICENSE](LICENSE.txt)).
By downloading and using the code and model you agree to the terms in the [LICENSE](LICENSE.txt).
[](https://www.apache.org/licenses/LICENSE-2.0)
|
lxyuan/distilbert-base-multilingual-cased-sentiments-student | lxyuan | "2023-06-24T04:09:07Z" | 2,534,172 | 254 | transformers | [
"transformers",
"pytorch",
"safetensors",
"distilbert",
"text-classification",
"sentiment-analysis",
"zero-shot-distillation",
"distillation",
"zero-shot-classification",
"debarta-v3",
"en",
"ar",
"de",
"es",
"fr",
"ja",
"zh",
"id",
"hi",
"it",
"ms",
"pt",
"dataset:tyqiangz/multilingual-sentiments",
"doi:10.57967/hf/1422",
"license:apache-2.0",
"autotrain_compatible",
"endpoints_compatible",
"region:us"
] | text-classification | "2023-05-05T16:22:55Z" | ---
license: apache-2.0
tags:
- sentiment-analysis
- text-classification
- zero-shot-distillation
- distillation
- zero-shot-classification
- debarta-v3
model-index:
- name: distilbert-base-multilingual-cased-sentiments-student
results: []
datasets:
- tyqiangz/multilingual-sentiments
language:
- en
- ar
- de
- es
- fr
- ja
- zh
- id
- hi
- it
- ms
- pt
---
<!-- This model card has been generated automatically according to the information the Trainer had access to. You
should probably proofread and complete it, then remove this comment. -->
# distilbert-base-multilingual-cased-sentiments-student
This model is distilled from the zero-shot classification pipeline on the Multilingual Sentiment
dataset using this [script](https://github.com/huggingface/transformers/tree/main/examples/research_projects/zero-shot-distillation).
In reality the multilingual-sentiment dataset is annotated of course,
but we'll pretend and ignore the annotations for the sake of example.
Teacher model: MoritzLaurer/mDeBERTa-v3-base-mnli-xnli
Teacher hypothesis template: "The sentiment of this text is {}."
Student model: distilbert-base-multilingual-cased
## Inference example
```python
from transformers import pipeline
distilled_student_sentiment_classifier = pipeline(
model="lxyuan/distilbert-base-multilingual-cased-sentiments-student",
return_all_scores=True
)
# english
distilled_student_sentiment_classifier ("I love this movie and i would watch it again and again!")
>> [[{'label': 'positive', 'score': 0.9731044769287109},
{'label': 'neutral', 'score': 0.016910076141357422},
{'label': 'negative', 'score': 0.009985478594899178}]]
# malay
distilled_student_sentiment_classifier("Saya suka filem ini dan saya akan menontonnya lagi dan lagi!")
[[{'label': 'positive', 'score': 0.9760093688964844},
{'label': 'neutral', 'score': 0.01804516464471817},
{'label': 'negative', 'score': 0.005945465061813593}]]
# japanese
distilled_student_sentiment_classifier("私はこの映画が大好きで、何度も見ます!")
>> [[{'label': 'positive', 'score': 0.9342429041862488},
{'label': 'neutral', 'score': 0.040193185210227966},
{'label': 'negative', 'score': 0.025563929229974747}]]
```
## Training procedure
Notebook link: [here](https://github.com/LxYuan0420/nlp/blob/main/notebooks/Distilling_Zero_Shot_multilingual_distilbert_sentiments_student.ipynb)
### Training hyperparameters
Result can be reproduce using the following commands:
```bash
python transformers/examples/research_projects/zero-shot-distillation/distill_classifier.py \
--data_file ./multilingual-sentiments/train_unlabeled.txt \
--class_names_file ./multilingual-sentiments/class_names.txt \
--hypothesis_template "The sentiment of this text is {}." \
--teacher_name_or_path MoritzLaurer/mDeBERTa-v3-base-mnli-xnli \
--teacher_batch_size 32 \
--student_name_or_path distilbert-base-multilingual-cased \
--output_dir ./distilbert-base-multilingual-cased-sentiments-student \
--per_device_train_batch_size 16 \
--fp16
```
If you are training this model on Colab, make the following code changes to avoid Out-of-memory error message:
```bash
###### modify L78 to disable fast tokenizer
default=False,
###### update dataset map part at L313
dataset = dataset.map(tokenizer, input_columns="text", fn_kwargs={"padding": "max_length", "truncation": True, "max_length": 512})
###### add following lines to L213
del model
print(f"Manually deleted Teacher model, free some memory for student model.")
###### add following lines to L337
trainer.push_to_hub()
tokenizer.push_to_hub("distilbert-base-multilingual-cased-sentiments-student")
```
### Training log
```bash
Training completed. Do not forget to share your model on huggingface.co/models =)
{'train_runtime': 2009.8864, 'train_samples_per_second': 73.0, 'train_steps_per_second': 4.563, 'train_loss': 0.6473459283913797, 'epoch': 1.0}
100%|███████████████████████████████████████| 9171/9171 [33:29<00:00, 4.56it/s]
[INFO|trainer.py:762] 2023-05-06 10:56:18,555 >> The following columns in the evaluation set don't have a corresponding argument in `DistilBertForSequenceClassification.forward` and have been ignored: text. If text are not expected by `DistilBertForSequenceClassification.forward`, you can safely ignore this message.
[INFO|trainer.py:3129] 2023-05-06 10:56:18,557 >> ***** Running Evaluation *****
[INFO|trainer.py:3131] 2023-05-06 10:56:18,557 >> Num examples = 146721
[INFO|trainer.py:3134] 2023-05-06 10:56:18,557 >> Batch size = 128
100%|███████████████████████████████████████| 1147/1147 [08:59<00:00, 2.13it/s]
05/06/2023 11:05:18 - INFO - __main__ - Agreement of student and teacher predictions: 88.29%
[INFO|trainer.py:2868] 2023-05-06 11:05:18,251 >> Saving model checkpoint to ./distilbert-base-multilingual-cased-sentiments-student
[INFO|configuration_utils.py:457] 2023-05-06 11:05:18,251 >> Configuration saved in ./distilbert-base-multilingual-cased-sentiments-student/config.json
[INFO|modeling_utils.py:1847] 2023-05-06 11:05:18,905 >> Model weights saved in ./distilbert-base-multilingual-cased-sentiments-student/pytorch_model.bin
[INFO|tokenization_utils_base.py:2171] 2023-05-06 11:05:18,905 >> tokenizer config file saved in ./distilbert-base-multilingual-cased-sentiments-student/tokenizer_config.json
[INFO|tokenization_utils_base.py:2178] 2023-05-06 11:05:18,905 >> Special tokens file saved in ./distilbert-base-multilingual-cased-sentiments-student/special_tokens_map.json
```
### Framework versions
- Transformers 4.28.1
- Pytorch 2.0.0+cu118
- Datasets 2.11.0
- Tokenizers 0.13.3 |
Salesforce/blip-image-captioning-large | Salesforce | "2023-12-07T09:07:33Z" | 2,516,728 | 1,146 | transformers | [
"transformers",
"pytorch",
"tf",
"safetensors",
"blip",
"image-text-to-text",
"image-captioning",
"image-to-text",
"arxiv:2201.12086",
"license:bsd-3-clause",
"endpoints_compatible",
"region:us"
] | image-to-text | "2022-12-13T11:27:40Z" | ---
pipeline_tag: image-to-text
tags:
- image-captioning
languages:
- en
license: bsd-3-clause
---
# BLIP: Bootstrapping Language-Image Pre-training for Unified Vision-Language Understanding and Generation
Model card for image captioning pretrained on COCO dataset - base architecture (with ViT large backbone).
|  |
|:--:|
| <b> Pull figure from BLIP official repo | Image source: https://github.com/salesforce/BLIP </b>|
## TL;DR
Authors from the [paper](https://arxiv.org/abs/2201.12086) write in the abstract:
*Vision-Language Pre-training (VLP) has advanced the performance for many vision-language tasks. However, most existing pre-trained models only excel in either understanding-based tasks or generation-based tasks. Furthermore, performance improvement has been largely achieved by scaling up the dataset with noisy image-text pairs collected from the web, which is a suboptimal source of supervision. In this paper, we propose BLIP, a new VLP framework which transfers flexibly to both vision-language understanding and generation tasks. BLIP effectively utilizes the noisy web data by bootstrapping the captions, where a captioner generates synthetic captions and a filter removes the noisy ones. We achieve state-of-the-art results on a wide range of vision-language tasks, such as image-text retrieval (+2.7% in average recall@1), image captioning (+2.8% in CIDEr), and VQA (+1.6% in VQA score). BLIP also demonstrates strong generalization ability when directly transferred to videolanguage tasks in a zero-shot manner. Code, models, and datasets are released.*
## Usage
You can use this model for conditional and un-conditional image captioning
### Using the Pytorch model
#### Running the model on CPU
<details>
<summary> Click to expand </summary>
```python
import requests
from PIL import Image
from transformers import BlipProcessor, BlipForConditionalGeneration
processor = BlipProcessor.from_pretrained("Salesforce/blip-image-captioning-large")
model = BlipForConditionalGeneration.from_pretrained("Salesforce/blip-image-captioning-large")
img_url = 'https://storage.googleapis.com/sfr-vision-language-research/BLIP/demo.jpg'
raw_image = Image.open(requests.get(img_url, stream=True).raw).convert('RGB')
# conditional image captioning
text = "a photography of"
inputs = processor(raw_image, text, return_tensors="pt")
out = model.generate(**inputs)
print(processor.decode(out[0], skip_special_tokens=True))
# unconditional image captioning
inputs = processor(raw_image, return_tensors="pt")
out = model.generate(**inputs)
print(processor.decode(out[0], skip_special_tokens=True))
```
</details>
#### Running the model on GPU
##### In full precision
<details>
<summary> Click to expand </summary>
```python
import requests
from PIL import Image
from transformers import BlipProcessor, BlipForConditionalGeneration
processor = BlipProcessor.from_pretrained("Salesforce/blip-image-captioning-large")
model = BlipForConditionalGeneration.from_pretrained("Salesforce/blip-image-captioning-large").to("cuda")
img_url = 'https://storage.googleapis.com/sfr-vision-language-research/BLIP/demo.jpg'
raw_image = Image.open(requests.get(img_url, stream=True).raw).convert('RGB')
# conditional image captioning
text = "a photography of"
inputs = processor(raw_image, text, return_tensors="pt").to("cuda")
out = model.generate(**inputs)
print(processor.decode(out[0], skip_special_tokens=True))
# unconditional image captioning
inputs = processor(raw_image, return_tensors="pt").to("cuda")
out = model.generate(**inputs)
print(processor.decode(out[0], skip_special_tokens=True))
```
</details>
##### In half precision (`float16`)
<details>
<summary> Click to expand </summary>
```python
import torch
import requests
from PIL import Image
from transformers import BlipProcessor, BlipForConditionalGeneration
processor = BlipProcessor.from_pretrained("Salesforce/blip-image-captioning-large")
model = BlipForConditionalGeneration.from_pretrained("Salesforce/blip-image-captioning-large", torch_dtype=torch.float16).to("cuda")
img_url = 'https://storage.googleapis.com/sfr-vision-language-research/BLIP/demo.jpg'
raw_image = Image.open(requests.get(img_url, stream=True).raw).convert('RGB')
# conditional image captioning
text = "a photography of"
inputs = processor(raw_image, text, return_tensors="pt").to("cuda", torch.float16)
out = model.generate(**inputs)
print(processor.decode(out[0], skip_special_tokens=True))
# >>> a photography of a woman and her dog
# unconditional image captioning
inputs = processor(raw_image, return_tensors="pt").to("cuda", torch.float16)
out = model.generate(**inputs)
print(processor.decode(out[0], skip_special_tokens=True))
>>> a woman sitting on the beach with her dog
```
</details>
## BibTex and citation info
```
@misc{https://doi.org/10.48550/arxiv.2201.12086,
doi = {10.48550/ARXIV.2201.12086},
url = {https://arxiv.org/abs/2201.12086},
author = {Li, Junnan and Li, Dongxu and Xiong, Caiming and Hoi, Steven},
keywords = {Computer Vision and Pattern Recognition (cs.CV), FOS: Computer and information sciences, FOS: Computer and information sciences},
title = {BLIP: Bootstrapping Language-Image Pre-training for Unified Vision-Language Understanding and Generation},
publisher = {arXiv},
year = {2022},
copyright = {Creative Commons Attribution 4.0 International}
}
``` |
unslothai/repeat | unslothai | "2024-07-07T16:55:04Z" | 2,511,095 | 0 | transformers | [
"transformers",
"safetensors",
"llama",
"feature-extraction",
"text-generation-inference",
"endpoints_compatible",
"region:us"
] | feature-extraction | "2024-07-07T16:55:01Z" | ---
library_name: transformers
tags: []
---
|
intfloat/multilingual-e5-large | intfloat | "2024-02-15T07:12:38Z" | 2,510,643 | 784 | sentence-transformers | [
"sentence-transformers",
"pytorch",
"onnx",
"safetensors",
"xlm-roberta",
"mteb",
"Sentence Transformers",
"sentence-similarity",
"feature-extraction",
"multilingual",
"af",
"am",
"ar",
"as",
"az",
"be",
"bg",
"bn",
"br",
"bs",
"ca",
"cs",
"cy",
"da",
"de",
"el",
"en",
"eo",
"es",
"et",
"eu",
"fa",
"fi",
"fr",
"fy",
"ga",
"gd",
"gl",
"gu",
"ha",
"he",
"hi",
"hr",
"hu",
"hy",
"id",
"is",
"it",
"ja",
"jv",
"ka",
"kk",
"km",
"kn",
"ko",
"ku",
"ky",
"la",
"lo",
"lt",
"lv",
"mg",
"mk",
"ml",
"mn",
"mr",
"ms",
"my",
"ne",
"nl",
"no",
"om",
"or",
"pa",
"pl",
"ps",
"pt",
"ro",
"ru",
"sa",
"sd",
"si",
"sk",
"sl",
"so",
"sq",
"sr",
"su",
"sv",
"sw",
"ta",
"te",
"th",
"tl",
"tr",
"ug",
"uk",
"ur",
"uz",
"vi",
"xh",
"yi",
"zh",
"arxiv:2402.05672",
"arxiv:2108.08787",
"arxiv:2104.08663",
"arxiv:2210.07316",
"license:mit",
"model-index",
"autotrain_compatible",
"text-embeddings-inference",
"endpoints_compatible",
"region:us"
] | feature-extraction | "2023-06-30T07:38:19Z" | ---
tags:
- mteb
- Sentence Transformers
- sentence-similarity
- feature-extraction
- sentence-transformers
model-index:
- name: multilingual-e5-large
results:
- task:
type: Classification
dataset:
type: mteb/amazon_counterfactual
name: MTEB AmazonCounterfactualClassification (en)
config: en
split: test
revision: e8379541af4e31359cca9fbcf4b00f2671dba205
metrics:
- type: accuracy
value: 79.05970149253731
- type: ap
value: 43.486574390835635
- type: f1
value: 73.32700092140148
- task:
type: Classification
dataset:
type: mteb/amazon_counterfactual
name: MTEB AmazonCounterfactualClassification (de)
config: de
split: test
revision: e8379541af4e31359cca9fbcf4b00f2671dba205
metrics:
- type: accuracy
value: 71.22055674518201
- type: ap
value: 81.55756710830498
- type: f1
value: 69.28271787752661
- task:
type: Classification
dataset:
type: mteb/amazon_counterfactual
name: MTEB AmazonCounterfactualClassification (en-ext)
config: en-ext
split: test
revision: e8379541af4e31359cca9fbcf4b00f2671dba205
metrics:
- type: accuracy
value: 80.41979010494754
- type: ap
value: 29.34879922376344
- type: f1
value: 67.62475449011278
- task:
type: Classification
dataset:
type: mteb/amazon_counterfactual
name: MTEB AmazonCounterfactualClassification (ja)
config: ja
split: test
revision: e8379541af4e31359cca9fbcf4b00f2671dba205
metrics:
- type: accuracy
value: 77.8372591006424
- type: ap
value: 26.557560591210738
- type: f1
value: 64.96619417368707
- task:
type: Classification
dataset:
type: mteb/amazon_polarity
name: MTEB AmazonPolarityClassification
config: default
split: test
revision: e2d317d38cd51312af73b3d32a06d1a08b442046
metrics:
- type: accuracy
value: 93.489875
- type: ap
value: 90.98758636917603
- type: f1
value: 93.48554819717332
- task:
type: Classification
dataset:
type: mteb/amazon_reviews_multi
name: MTEB AmazonReviewsClassification (en)
config: en
split: test
revision: 1399c76144fd37290681b995c656ef9b2e06e26d
metrics:
- type: accuracy
value: 47.564
- type: f1
value: 46.75122173518047
- task:
type: Classification
dataset:
type: mteb/amazon_reviews_multi
name: MTEB AmazonReviewsClassification (de)
config: de
split: test
revision: 1399c76144fd37290681b995c656ef9b2e06e26d
metrics:
- type: accuracy
value: 45.400000000000006
- type: f1
value: 44.17195682400632
- task:
type: Classification
dataset:
type: mteb/amazon_reviews_multi
name: MTEB AmazonReviewsClassification (es)
config: es
split: test
revision: 1399c76144fd37290681b995c656ef9b2e06e26d
metrics:
- type: accuracy
value: 43.068
- type: f1
value: 42.38155696855596
- task:
type: Classification
dataset:
type: mteb/amazon_reviews_multi
name: MTEB AmazonReviewsClassification (fr)
config: fr
split: test
revision: 1399c76144fd37290681b995c656ef9b2e06e26d
metrics:
- type: accuracy
value: 41.89
- type: f1
value: 40.84407321682663
- task:
type: Classification
dataset:
type: mteb/amazon_reviews_multi
name: MTEB AmazonReviewsClassification (ja)
config: ja
split: test
revision: 1399c76144fd37290681b995c656ef9b2e06e26d
metrics:
- type: accuracy
value: 40.120000000000005
- type: f1
value: 39.522976223819114
- task:
type: Classification
dataset:
type: mteb/amazon_reviews_multi
name: MTEB AmazonReviewsClassification (zh)
config: zh
split: test
revision: 1399c76144fd37290681b995c656ef9b2e06e26d
metrics:
- type: accuracy
value: 38.832
- type: f1
value: 38.0392533394713
- task:
type: Retrieval
dataset:
type: arguana
name: MTEB ArguAna
config: default
split: test
revision: None
metrics:
- type: map_at_1
value: 30.725
- type: map_at_10
value: 46.055
- type: map_at_100
value: 46.900999999999996
- type: map_at_1000
value: 46.911
- type: map_at_3
value: 41.548
- type: map_at_5
value: 44.297
- type: mrr_at_1
value: 31.152
- type: mrr_at_10
value: 46.231
- type: mrr_at_100
value: 47.07
- type: mrr_at_1000
value: 47.08
- type: mrr_at_3
value: 41.738
- type: mrr_at_5
value: 44.468999999999994
- type: ndcg_at_1
value: 30.725
- type: ndcg_at_10
value: 54.379999999999995
- type: ndcg_at_100
value: 58.138
- type: ndcg_at_1000
value: 58.389
- type: ndcg_at_3
value: 45.156
- type: ndcg_at_5
value: 50.123
- type: precision_at_1
value: 30.725
- type: precision_at_10
value: 8.087
- type: precision_at_100
value: 0.9769999999999999
- type: precision_at_1000
value: 0.1
- type: precision_at_3
value: 18.54
- type: precision_at_5
value: 13.542000000000002
- type: recall_at_1
value: 30.725
- type: recall_at_10
value: 80.868
- type: recall_at_100
value: 97.653
- type: recall_at_1000
value: 99.57300000000001
- type: recall_at_3
value: 55.619
- type: recall_at_5
value: 67.71000000000001
- task:
type: Clustering
dataset:
type: mteb/arxiv-clustering-p2p
name: MTEB ArxivClusteringP2P
config: default
split: test
revision: a122ad7f3f0291bf49cc6f4d32aa80929df69d5d
metrics:
- type: v_measure
value: 44.30960650674069
- task:
type: Clustering
dataset:
type: mteb/arxiv-clustering-s2s
name: MTEB ArxivClusteringS2S
config: default
split: test
revision: f910caf1a6075f7329cdf8c1a6135696f37dbd53
metrics:
- type: v_measure
value: 38.427074197498996
- task:
type: Reranking
dataset:
type: mteb/askubuntudupquestions-reranking
name: MTEB AskUbuntuDupQuestions
config: default
split: test
revision: 2000358ca161889fa9c082cb41daa8dcfb161a54
metrics:
- type: map
value: 60.28270056031872
- type: mrr
value: 74.38332673789738
- task:
type: STS
dataset:
type: mteb/biosses-sts
name: MTEB BIOSSES
config: default
split: test
revision: d3fb88f8f02e40887cd149695127462bbcf29b4a
metrics:
- type: cos_sim_pearson
value: 84.05942144105269
- type: cos_sim_spearman
value: 82.51212105850809
- type: euclidean_pearson
value: 81.95639829909122
- type: euclidean_spearman
value: 82.3717564144213
- type: manhattan_pearson
value: 81.79273425468256
- type: manhattan_spearman
value: 82.20066817871039
- task:
type: BitextMining
dataset:
type: mteb/bucc-bitext-mining
name: MTEB BUCC (de-en)
config: de-en
split: test
revision: d51519689f32196a32af33b075a01d0e7c51e252
metrics:
- type: accuracy
value: 99.46764091858039
- type: f1
value: 99.37717466945023
- type: precision
value: 99.33194154488518
- type: recall
value: 99.46764091858039
- task:
type: BitextMining
dataset:
type: mteb/bucc-bitext-mining
name: MTEB BUCC (fr-en)
config: fr-en
split: test
revision: d51519689f32196a32af33b075a01d0e7c51e252
metrics:
- type: accuracy
value: 98.29407880255337
- type: f1
value: 98.11248073959938
- type: precision
value: 98.02443319392472
- type: recall
value: 98.29407880255337
- task:
type: BitextMining
dataset:
type: mteb/bucc-bitext-mining
name: MTEB BUCC (ru-en)
config: ru-en
split: test
revision: d51519689f32196a32af33b075a01d0e7c51e252
metrics:
- type: accuracy
value: 97.79009352268791
- type: f1
value: 97.5176076665512
- type: precision
value: 97.38136473848286
- type: recall
value: 97.79009352268791
- task:
type: BitextMining
dataset:
type: mteb/bucc-bitext-mining
name: MTEB BUCC (zh-en)
config: zh-en
split: test
revision: d51519689f32196a32af33b075a01d0e7c51e252
metrics:
- type: accuracy
value: 99.26276987888363
- type: f1
value: 99.20133403545726
- type: precision
value: 99.17500438827453
- type: recall
value: 99.26276987888363
- task:
type: Classification
dataset:
type: mteb/banking77
name: MTEB Banking77Classification
config: default
split: test
revision: 0fd18e25b25c072e09e0d92ab615fda904d66300
metrics:
- type: accuracy
value: 84.72727272727273
- type: f1
value: 84.67672206031433
- task:
type: Clustering
dataset:
type: mteb/biorxiv-clustering-p2p
name: MTEB BiorxivClusteringP2P
config: default
split: test
revision: 65b79d1d13f80053f67aca9498d9402c2d9f1f40
metrics:
- type: v_measure
value: 35.34220182511161
- task:
type: Clustering
dataset:
type: mteb/biorxiv-clustering-s2s
name: MTEB BiorxivClusteringS2S
config: default
split: test
revision: 258694dd0231531bc1fd9de6ceb52a0853c6d908
metrics:
- type: v_measure
value: 33.4987096128766
- task:
type: Retrieval
dataset:
type: BeIR/cqadupstack
name: MTEB CQADupstackRetrieval
config: default
split: test
revision: None
metrics:
- type: map_at_1
value: 25.558249999999997
- type: map_at_10
value: 34.44425000000001
- type: map_at_100
value: 35.59833333333333
- type: map_at_1000
value: 35.706916666666665
- type: map_at_3
value: 31.691749999999995
- type: map_at_5
value: 33.252916666666664
- type: mrr_at_1
value: 30.252666666666666
- type: mrr_at_10
value: 38.60675
- type: mrr_at_100
value: 39.42666666666666
- type: mrr_at_1000
value: 39.48408333333334
- type: mrr_at_3
value: 36.17441666666665
- type: mrr_at_5
value: 37.56275
- type: ndcg_at_1
value: 30.252666666666666
- type: ndcg_at_10
value: 39.683
- type: ndcg_at_100
value: 44.68541666666667
- type: ndcg_at_1000
value: 46.94316666666668
- type: ndcg_at_3
value: 34.961749999999995
- type: ndcg_at_5
value: 37.215666666666664
- type: precision_at_1
value: 30.252666666666666
- type: precision_at_10
value: 6.904166666666667
- type: precision_at_100
value: 1.0989999999999995
- type: precision_at_1000
value: 0.14733333333333334
- type: precision_at_3
value: 16.037666666666667
- type: precision_at_5
value: 11.413583333333333
- type: recall_at_1
value: 25.558249999999997
- type: recall_at_10
value: 51.13341666666666
- type: recall_at_100
value: 73.08366666666667
- type: recall_at_1000
value: 88.79483333333334
- type: recall_at_3
value: 37.989083333333326
- type: recall_at_5
value: 43.787833333333325
- task:
type: Retrieval
dataset:
type: climate-fever
name: MTEB ClimateFEVER
config: default
split: test
revision: None
metrics:
- type: map_at_1
value: 10.338
- type: map_at_10
value: 18.360000000000003
- type: map_at_100
value: 19.942
- type: map_at_1000
value: 20.134
- type: map_at_3
value: 15.174000000000001
- type: map_at_5
value: 16.830000000000002
- type: mrr_at_1
value: 23.257
- type: mrr_at_10
value: 33.768
- type: mrr_at_100
value: 34.707
- type: mrr_at_1000
value: 34.766000000000005
- type: mrr_at_3
value: 30.977
- type: mrr_at_5
value: 32.528
- type: ndcg_at_1
value: 23.257
- type: ndcg_at_10
value: 25.733
- type: ndcg_at_100
value: 32.288
- type: ndcg_at_1000
value: 35.992000000000004
- type: ndcg_at_3
value: 20.866
- type: ndcg_at_5
value: 22.612
- type: precision_at_1
value: 23.257
- type: precision_at_10
value: 8.124
- type: precision_at_100
value: 1.518
- type: precision_at_1000
value: 0.219
- type: precision_at_3
value: 15.679000000000002
- type: precision_at_5
value: 12.117
- type: recall_at_1
value: 10.338
- type: recall_at_10
value: 31.154
- type: recall_at_100
value: 54.161
- type: recall_at_1000
value: 75.21900000000001
- type: recall_at_3
value: 19.427
- type: recall_at_5
value: 24.214
- task:
type: Retrieval
dataset:
type: dbpedia-entity
name: MTEB DBPedia
config: default
split: test
revision: None
metrics:
- type: map_at_1
value: 8.498
- type: map_at_10
value: 19.103
- type: map_at_100
value: 27.375
- type: map_at_1000
value: 28.981
- type: map_at_3
value: 13.764999999999999
- type: map_at_5
value: 15.950000000000001
- type: mrr_at_1
value: 65.5
- type: mrr_at_10
value: 74.53800000000001
- type: mrr_at_100
value: 74.71799999999999
- type: mrr_at_1000
value: 74.725
- type: mrr_at_3
value: 72.792
- type: mrr_at_5
value: 73.554
- type: ndcg_at_1
value: 53.37499999999999
- type: ndcg_at_10
value: 41.286
- type: ndcg_at_100
value: 45.972
- type: ndcg_at_1000
value: 53.123
- type: ndcg_at_3
value: 46.172999999999995
- type: ndcg_at_5
value: 43.033
- type: precision_at_1
value: 65.5
- type: precision_at_10
value: 32.725
- type: precision_at_100
value: 10.683
- type: precision_at_1000
value: 1.978
- type: precision_at_3
value: 50
- type: precision_at_5
value: 41.349999999999994
- type: recall_at_1
value: 8.498
- type: recall_at_10
value: 25.070999999999998
- type: recall_at_100
value: 52.383
- type: recall_at_1000
value: 74.91499999999999
- type: recall_at_3
value: 15.207999999999998
- type: recall_at_5
value: 18.563
- task:
type: Classification
dataset:
type: mteb/emotion
name: MTEB EmotionClassification
config: default
split: test
revision: 4f58c6b202a23cf9a4da393831edf4f9183cad37
metrics:
- type: accuracy
value: 46.5
- type: f1
value: 41.93833713984145
- task:
type: Retrieval
dataset:
type: fever
name: MTEB FEVER
config: default
split: test
revision: None
metrics:
- type: map_at_1
value: 67.914
- type: map_at_10
value: 78.10000000000001
- type: map_at_100
value: 78.333
- type: map_at_1000
value: 78.346
- type: map_at_3
value: 76.626
- type: map_at_5
value: 77.627
- type: mrr_at_1
value: 72.74199999999999
- type: mrr_at_10
value: 82.414
- type: mrr_at_100
value: 82.511
- type: mrr_at_1000
value: 82.513
- type: mrr_at_3
value: 81.231
- type: mrr_at_5
value: 82.065
- type: ndcg_at_1
value: 72.74199999999999
- type: ndcg_at_10
value: 82.806
- type: ndcg_at_100
value: 83.677
- type: ndcg_at_1000
value: 83.917
- type: ndcg_at_3
value: 80.305
- type: ndcg_at_5
value: 81.843
- type: precision_at_1
value: 72.74199999999999
- type: precision_at_10
value: 10.24
- type: precision_at_100
value: 1.089
- type: precision_at_1000
value: 0.11299999999999999
- type: precision_at_3
value: 31.268
- type: precision_at_5
value: 19.706000000000003
- type: recall_at_1
value: 67.914
- type: recall_at_10
value: 92.889
- type: recall_at_100
value: 96.42699999999999
- type: recall_at_1000
value: 97.92
- type: recall_at_3
value: 86.21
- type: recall_at_5
value: 90.036
- task:
type: Retrieval
dataset:
type: fiqa
name: MTEB FiQA2018
config: default
split: test
revision: None
metrics:
- type: map_at_1
value: 22.166
- type: map_at_10
value: 35.57
- type: map_at_100
value: 37.405
- type: map_at_1000
value: 37.564
- type: map_at_3
value: 30.379
- type: map_at_5
value: 33.324
- type: mrr_at_1
value: 43.519000000000005
- type: mrr_at_10
value: 51.556000000000004
- type: mrr_at_100
value: 52.344
- type: mrr_at_1000
value: 52.373999999999995
- type: mrr_at_3
value: 48.868
- type: mrr_at_5
value: 50.319
- type: ndcg_at_1
value: 43.519000000000005
- type: ndcg_at_10
value: 43.803
- type: ndcg_at_100
value: 50.468999999999994
- type: ndcg_at_1000
value: 53.111
- type: ndcg_at_3
value: 38.893
- type: ndcg_at_5
value: 40.653
- type: precision_at_1
value: 43.519000000000005
- type: precision_at_10
value: 12.253
- type: precision_at_100
value: 1.931
- type: precision_at_1000
value: 0.242
- type: precision_at_3
value: 25.617
- type: precision_at_5
value: 19.383
- type: recall_at_1
value: 22.166
- type: recall_at_10
value: 51.6
- type: recall_at_100
value: 76.574
- type: recall_at_1000
value: 92.192
- type: recall_at_3
value: 34.477999999999994
- type: recall_at_5
value: 41.835
- task:
type: Retrieval
dataset:
type: hotpotqa
name: MTEB HotpotQA
config: default
split: test
revision: None
metrics:
- type: map_at_1
value: 39.041
- type: map_at_10
value: 62.961999999999996
- type: map_at_100
value: 63.79899999999999
- type: map_at_1000
value: 63.854
- type: map_at_3
value: 59.399
- type: map_at_5
value: 61.669
- type: mrr_at_1
value: 78.082
- type: mrr_at_10
value: 84.321
- type: mrr_at_100
value: 84.49600000000001
- type: mrr_at_1000
value: 84.502
- type: mrr_at_3
value: 83.421
- type: mrr_at_5
value: 83.977
- type: ndcg_at_1
value: 78.082
- type: ndcg_at_10
value: 71.229
- type: ndcg_at_100
value: 74.10900000000001
- type: ndcg_at_1000
value: 75.169
- type: ndcg_at_3
value: 66.28699999999999
- type: ndcg_at_5
value: 69.084
- type: precision_at_1
value: 78.082
- type: precision_at_10
value: 14.993
- type: precision_at_100
value: 1.7239999999999998
- type: precision_at_1000
value: 0.186
- type: precision_at_3
value: 42.737
- type: precision_at_5
value: 27.843
- type: recall_at_1
value: 39.041
- type: recall_at_10
value: 74.96300000000001
- type: recall_at_100
value: 86.199
- type: recall_at_1000
value: 93.228
- type: recall_at_3
value: 64.105
- type: recall_at_5
value: 69.608
- task:
type: Classification
dataset:
type: mteb/imdb
name: MTEB ImdbClassification
config: default
split: test
revision: 3d86128a09e091d6018b6d26cad27f2739fc2db7
metrics:
- type: accuracy
value: 90.23160000000001
- type: ap
value: 85.5674856808308
- type: f1
value: 90.18033354786317
- task:
type: Retrieval
dataset:
type: msmarco
name: MTEB MSMARCO
config: default
split: dev
revision: None
metrics:
- type: map_at_1
value: 24.091
- type: map_at_10
value: 36.753
- type: map_at_100
value: 37.913000000000004
- type: map_at_1000
value: 37.958999999999996
- type: map_at_3
value: 32.818999999999996
- type: map_at_5
value: 35.171
- type: mrr_at_1
value: 24.742
- type: mrr_at_10
value: 37.285000000000004
- type: mrr_at_100
value: 38.391999999999996
- type: mrr_at_1000
value: 38.431
- type: mrr_at_3
value: 33.440999999999995
- type: mrr_at_5
value: 35.75
- type: ndcg_at_1
value: 24.742
- type: ndcg_at_10
value: 43.698
- type: ndcg_at_100
value: 49.145
- type: ndcg_at_1000
value: 50.23800000000001
- type: ndcg_at_3
value: 35.769
- type: ndcg_at_5
value: 39.961999999999996
- type: precision_at_1
value: 24.742
- type: precision_at_10
value: 6.7989999999999995
- type: precision_at_100
value: 0.95
- type: precision_at_1000
value: 0.104
- type: precision_at_3
value: 15.096000000000002
- type: precision_at_5
value: 11.183
- type: recall_at_1
value: 24.091
- type: recall_at_10
value: 65.068
- type: recall_at_100
value: 89.899
- type: recall_at_1000
value: 98.16
- type: recall_at_3
value: 43.68
- type: recall_at_5
value: 53.754999999999995
- task:
type: Classification
dataset:
type: mteb/mtop_domain
name: MTEB MTOPDomainClassification (en)
config: en
split: test
revision: d80d48c1eb48d3562165c59d59d0034df9fff0bf
metrics:
- type: accuracy
value: 93.66621067031465
- type: f1
value: 93.49622853272142
- task:
type: Classification
dataset:
type: mteb/mtop_domain
name: MTEB MTOPDomainClassification (de)
config: de
split: test
revision: d80d48c1eb48d3562165c59d59d0034df9fff0bf
metrics:
- type: accuracy
value: 91.94702733164272
- type: f1
value: 91.17043441745282
- task:
type: Classification
dataset:
type: mteb/mtop_domain
name: MTEB MTOPDomainClassification (es)
config: es
split: test
revision: d80d48c1eb48d3562165c59d59d0034df9fff0bf
metrics:
- type: accuracy
value: 92.20146764509674
- type: f1
value: 91.98359080555608
- task:
type: Classification
dataset:
type: mteb/mtop_domain
name: MTEB MTOPDomainClassification (fr)
config: fr
split: test
revision: d80d48c1eb48d3562165c59d59d0034df9fff0bf
metrics:
- type: accuracy
value: 88.99780770435328
- type: f1
value: 89.19746342724068
- task:
type: Classification
dataset:
type: mteb/mtop_domain
name: MTEB MTOPDomainClassification (hi)
config: hi
split: test
revision: d80d48c1eb48d3562165c59d59d0034df9fff0bf
metrics:
- type: accuracy
value: 89.78486912871998
- type: f1
value: 89.24578823628642
- task:
type: Classification
dataset:
type: mteb/mtop_domain
name: MTEB MTOPDomainClassification (th)
config: th
split: test
revision: d80d48c1eb48d3562165c59d59d0034df9fff0bf
metrics:
- type: accuracy
value: 88.74502712477394
- type: f1
value: 89.00297573881542
- task:
type: Classification
dataset:
type: mteb/mtop_intent
name: MTEB MTOPIntentClassification (en)
config: en
split: test
revision: ae001d0e6b1228650b7bd1c2c65fb50ad11a8aba
metrics:
- type: accuracy
value: 77.9046967624259
- type: f1
value: 59.36787125785957
- task:
type: Classification
dataset:
type: mteb/mtop_intent
name: MTEB MTOPIntentClassification (de)
config: de
split: test
revision: ae001d0e6b1228650b7bd1c2c65fb50ad11a8aba
metrics:
- type: accuracy
value: 74.5280360664976
- type: f1
value: 57.17723440888718
- task:
type: Classification
dataset:
type: mteb/mtop_intent
name: MTEB MTOPIntentClassification (es)
config: es
split: test
revision: ae001d0e6b1228650b7bd1c2c65fb50ad11a8aba
metrics:
- type: accuracy
value: 75.44029352901934
- type: f1
value: 54.052855531072964
- task:
type: Classification
dataset:
type: mteb/mtop_intent
name: MTEB MTOPIntentClassification (fr)
config: fr
split: test
revision: ae001d0e6b1228650b7bd1c2c65fb50ad11a8aba
metrics:
- type: accuracy
value: 70.5606013153774
- type: f1
value: 52.62215934386531
- task:
type: Classification
dataset:
type: mteb/mtop_intent
name: MTEB MTOPIntentClassification (hi)
config: hi
split: test
revision: ae001d0e6b1228650b7bd1c2c65fb50ad11a8aba
metrics:
- type: accuracy
value: 73.11581211903908
- type: f1
value: 52.341291845645465
- task:
type: Classification
dataset:
type: mteb/mtop_intent
name: MTEB MTOPIntentClassification (th)
config: th
split: test
revision: ae001d0e6b1228650b7bd1c2c65fb50ad11a8aba
metrics:
- type: accuracy
value: 74.28933092224233
- type: f1
value: 57.07918745504911
- task:
type: Classification
dataset:
type: mteb/amazon_massive_intent
name: MTEB MassiveIntentClassification (af)
config: af
split: test
revision: 31efe3c427b0bae9c22cbb560b8f15491cc6bed7
metrics:
- type: accuracy
value: 62.38063214525892
- type: f1
value: 59.46463723443009
- task:
type: Classification
dataset:
type: mteb/amazon_massive_intent
name: MTEB MassiveIntentClassification (am)
config: am
split: test
revision: 31efe3c427b0bae9c22cbb560b8f15491cc6bed7
metrics:
- type: accuracy
value: 56.06926698049766
- type: f1
value: 52.49084283283562
- task:
type: Classification
dataset:
type: mteb/amazon_massive_intent
name: MTEB MassiveIntentClassification (ar)
config: ar
split: test
revision: 31efe3c427b0bae9c22cbb560b8f15491cc6bed7
metrics:
- type: accuracy
value: 60.74983187626093
- type: f1
value: 56.960640620165904
- task:
type: Classification
dataset:
type: mteb/amazon_massive_intent
name: MTEB MassiveIntentClassification (az)
config: az
split: test
revision: 31efe3c427b0bae9c22cbb560b8f15491cc6bed7
metrics:
- type: accuracy
value: 64.86550100874243
- type: f1
value: 62.47370548140688
- task:
type: Classification
dataset:
type: mteb/amazon_massive_intent
name: MTEB MassiveIntentClassification (bn)
config: bn
split: test
revision: 31efe3c427b0bae9c22cbb560b8f15491cc6bed7
metrics:
- type: accuracy
value: 63.971082716879636
- type: f1
value: 61.03812421957381
- task:
type: Classification
dataset:
type: mteb/amazon_massive_intent
name: MTEB MassiveIntentClassification (cy)
config: cy
split: test
revision: 31efe3c427b0bae9c22cbb560b8f15491cc6bed7
metrics:
- type: accuracy
value: 54.98318762609282
- type: f1
value: 51.51207916008392
- task:
type: Classification
dataset:
type: mteb/amazon_massive_intent
name: MTEB MassiveIntentClassification (da)
config: da
split: test
revision: 31efe3c427b0bae9c22cbb560b8f15491cc6bed7
metrics:
- type: accuracy
value: 69.45527908540686
- type: f1
value: 66.16631905400318
- task:
type: Classification
dataset:
type: mteb/amazon_massive_intent
name: MTEB MassiveIntentClassification (de)
config: de
split: test
revision: 31efe3c427b0bae9c22cbb560b8f15491cc6bed7
metrics:
- type: accuracy
value: 69.32750504371216
- type: f1
value: 66.16755288646591
- task:
type: Classification
dataset:
type: mteb/amazon_massive_intent
name: MTEB MassiveIntentClassification (el)
config: el
split: test
revision: 31efe3c427b0bae9c22cbb560b8f15491cc6bed7
metrics:
- type: accuracy
value: 69.09213180901143
- type: f1
value: 66.95654394661507
- task:
type: Classification
dataset:
type: mteb/amazon_massive_intent
name: MTEB MassiveIntentClassification (en)
config: en
split: test
revision: 31efe3c427b0bae9c22cbb560b8f15491cc6bed7
metrics:
- type: accuracy
value: 73.75588433086752
- type: f1
value: 71.79973779656923
- task:
type: Classification
dataset:
type: mteb/amazon_massive_intent
name: MTEB MassiveIntentClassification (es)
config: es
split: test
revision: 31efe3c427b0bae9c22cbb560b8f15491cc6bed7
metrics:
- type: accuracy
value: 70.49428379287154
- type: f1
value: 68.37494379215734
- task:
type: Classification
dataset:
type: mteb/amazon_massive_intent
name: MTEB MassiveIntentClassification (fa)
config: fa
split: test
revision: 31efe3c427b0bae9c22cbb560b8f15491cc6bed7
metrics:
- type: accuracy
value: 69.90921318090115
- type: f1
value: 66.79517376481645
- task:
type: Classification
dataset:
type: mteb/amazon_massive_intent
name: MTEB MassiveIntentClassification (fi)
config: fi
split: test
revision: 31efe3c427b0bae9c22cbb560b8f15491cc6bed7
metrics:
- type: accuracy
value: 70.12104909213181
- type: f1
value: 67.29448842879584
- task:
type: Classification
dataset:
type: mteb/amazon_massive_intent
name: MTEB MassiveIntentClassification (fr)
config: fr
split: test
revision: 31efe3c427b0bae9c22cbb560b8f15491cc6bed7
metrics:
- type: accuracy
value: 69.34095494283793
- type: f1
value: 67.01134288992947
- task:
type: Classification
dataset:
type: mteb/amazon_massive_intent
name: MTEB MassiveIntentClassification (he)
config: he
split: test
revision: 31efe3c427b0bae9c22cbb560b8f15491cc6bed7
metrics:
- type: accuracy
value: 67.61264290517822
- type: f1
value: 64.68730512660757
- task:
type: Classification
dataset:
type: mteb/amazon_massive_intent
name: MTEB MassiveIntentClassification (hi)
config: hi
split: test
revision: 31efe3c427b0bae9c22cbb560b8f15491cc6bed7
metrics:
- type: accuracy
value: 67.79757901815738
- type: f1
value: 65.24938539425598
- task:
type: Classification
dataset:
type: mteb/amazon_massive_intent
name: MTEB MassiveIntentClassification (hu)
config: hu
split: test
revision: 31efe3c427b0bae9c22cbb560b8f15491cc6bed7
metrics:
- type: accuracy
value: 69.68728984532616
- type: f1
value: 67.0487169762553
- task:
type: Classification
dataset:
type: mteb/amazon_massive_intent
name: MTEB MassiveIntentClassification (hy)
config: hy
split: test
revision: 31efe3c427b0bae9c22cbb560b8f15491cc6bed7
metrics:
- type: accuracy
value: 62.07464694014795
- type: f1
value: 59.183532276789286
- task:
type: Classification
dataset:
type: mteb/amazon_massive_intent
name: MTEB MassiveIntentClassification (id)
config: id
split: test
revision: 31efe3c427b0bae9c22cbb560b8f15491cc6bed7
metrics:
- type: accuracy
value: 70.04707464694015
- type: f1
value: 67.66829629003848
- task:
type: Classification
dataset:
type: mteb/amazon_massive_intent
name: MTEB MassiveIntentClassification (is)
config: is
split: test
revision: 31efe3c427b0bae9c22cbb560b8f15491cc6bed7
metrics:
- type: accuracy
value: 62.42434431741762
- type: f1
value: 59.01617226544757
- task:
type: Classification
dataset:
type: mteb/amazon_massive_intent
name: MTEB MassiveIntentClassification (it)
config: it
split: test
revision: 31efe3c427b0bae9c22cbb560b8f15491cc6bed7
metrics:
- type: accuracy
value: 70.53127101546738
- type: f1
value: 68.10033760906255
- task:
type: Classification
dataset:
type: mteb/amazon_massive_intent
name: MTEB MassiveIntentClassification (ja)
config: ja
split: test
revision: 31efe3c427b0bae9c22cbb560b8f15491cc6bed7
metrics:
- type: accuracy
value: 72.50504371217215
- type: f1
value: 69.74931103158923
- task:
type: Classification
dataset:
type: mteb/amazon_massive_intent
name: MTEB MassiveIntentClassification (jv)
config: jv
split: test
revision: 31efe3c427b0bae9c22cbb560b8f15491cc6bed7
metrics:
- type: accuracy
value: 57.91190316072628
- type: f1
value: 54.05551136648796
- task:
type: Classification
dataset:
type: mteb/amazon_massive_intent
name: MTEB MassiveIntentClassification (ka)
config: ka
split: test
revision: 31efe3c427b0bae9c22cbb560b8f15491cc6bed7
metrics:
- type: accuracy
value: 51.78211163416275
- type: f1
value: 49.874888544058535
- task:
type: Classification
dataset:
type: mteb/amazon_massive_intent
name: MTEB MassiveIntentClassification (km)
config: km
split: test
revision: 31efe3c427b0bae9c22cbb560b8f15491cc6bed7
metrics:
- type: accuracy
value: 47.017484868863484
- type: f1
value: 44.53364263352014
- task:
type: Classification
dataset:
type: mteb/amazon_massive_intent
name: MTEB MassiveIntentClassification (kn)
config: kn
split: test
revision: 31efe3c427b0bae9c22cbb560b8f15491cc6bed7
metrics:
- type: accuracy
value: 62.16207128446537
- type: f1
value: 59.01185692320829
- task:
type: Classification
dataset:
type: mteb/amazon_massive_intent
name: MTEB MassiveIntentClassification (ko)
config: ko
split: test
revision: 31efe3c427b0bae9c22cbb560b8f15491cc6bed7
metrics:
- type: accuracy
value: 69.42501681237391
- type: f1
value: 67.13169450166086
- task:
type: Classification
dataset:
type: mteb/amazon_massive_intent
name: MTEB MassiveIntentClassification (lv)
config: lv
split: test
revision: 31efe3c427b0bae9c22cbb560b8f15491cc6bed7
metrics:
- type: accuracy
value: 67.0780094149294
- type: f1
value: 64.41720167850707
- task:
type: Classification
dataset:
type: mteb/amazon_massive_intent
name: MTEB MassiveIntentClassification (ml)
config: ml
split: test
revision: 31efe3c427b0bae9c22cbb560b8f15491cc6bed7
metrics:
- type: accuracy
value: 65.57162071284466
- type: f1
value: 62.414138683804424
- task:
type: Classification
dataset:
type: mteb/amazon_massive_intent
name: MTEB MassiveIntentClassification (mn)
config: mn
split: test
revision: 31efe3c427b0bae9c22cbb560b8f15491cc6bed7
metrics:
- type: accuracy
value: 61.71149966375252
- type: f1
value: 58.594805125087234
- task:
type: Classification
dataset:
type: mteb/amazon_massive_intent
name: MTEB MassiveIntentClassification (ms)
config: ms
split: test
revision: 31efe3c427b0bae9c22cbb560b8f15491cc6bed7
metrics:
- type: accuracy
value: 66.03900470746471
- type: f1
value: 63.87937257883887
- task:
type: Classification
dataset:
type: mteb/amazon_massive_intent
name: MTEB MassiveIntentClassification (my)
config: my
split: test
revision: 31efe3c427b0bae9c22cbb560b8f15491cc6bed7
metrics:
- type: accuracy
value: 60.8776059179556
- type: f1
value: 57.48587618059131
- task:
type: Classification
dataset:
type: mteb/amazon_massive_intent
name: MTEB MassiveIntentClassification (nb)
config: nb
split: test
revision: 31efe3c427b0bae9c22cbb560b8f15491cc6bed7
metrics:
- type: accuracy
value: 69.87895090786819
- type: f1
value: 66.8141299430347
- task:
type: Classification
dataset:
type: mteb/amazon_massive_intent
name: MTEB MassiveIntentClassification (nl)
config: nl
split: test
revision: 31efe3c427b0bae9c22cbb560b8f15491cc6bed7
metrics:
- type: accuracy
value: 70.45057162071285
- type: f1
value: 67.46444039673516
- task:
type: Classification
dataset:
type: mteb/amazon_massive_intent
name: MTEB MassiveIntentClassification (pl)
config: pl
split: test
revision: 31efe3c427b0bae9c22cbb560b8f15491cc6bed7
metrics:
- type: accuracy
value: 71.546738399462
- type: f1
value: 68.63640876702655
- task:
type: Classification
dataset:
type: mteb/amazon_massive_intent
name: MTEB MassiveIntentClassification (pt)
config: pt
split: test
revision: 31efe3c427b0bae9c22cbb560b8f15491cc6bed7
metrics:
- type: accuracy
value: 70.72965702757229
- type: f1
value: 68.54119560379115
- task:
type: Classification
dataset:
type: mteb/amazon_massive_intent
name: MTEB MassiveIntentClassification (ro)
config: ro
split: test
revision: 31efe3c427b0bae9c22cbb560b8f15491cc6bed7
metrics:
- type: accuracy
value: 68.35574983187625
- type: f1
value: 65.88844917691927
- task:
type: Classification
dataset:
type: mteb/amazon_massive_intent
name: MTEB MassiveIntentClassification (ru)
config: ru
split: test
revision: 31efe3c427b0bae9c22cbb560b8f15491cc6bed7
metrics:
- type: accuracy
value: 71.70477471418964
- type: f1
value: 69.19665697061978
- task:
type: Classification
dataset:
type: mteb/amazon_massive_intent
name: MTEB MassiveIntentClassification (sl)
config: sl
split: test
revision: 31efe3c427b0bae9c22cbb560b8f15491cc6bed7
metrics:
- type: accuracy
value: 67.0880968392737
- type: f1
value: 64.76962317666086
- task:
type: Classification
dataset:
type: mteb/amazon_massive_intent
name: MTEB MassiveIntentClassification (sq)
config: sq
split: test
revision: 31efe3c427b0bae9c22cbb560b8f15491cc6bed7
metrics:
- type: accuracy
value: 65.18493611297916
- type: f1
value: 62.49984559035371
- task:
type: Classification
dataset:
type: mteb/amazon_massive_intent
name: MTEB MassiveIntentClassification (sv)
config: sv
split: test
revision: 31efe3c427b0bae9c22cbb560b8f15491cc6bed7
metrics:
- type: accuracy
value: 71.75857431069265
- type: f1
value: 69.20053687623418
- task:
type: Classification
dataset:
type: mteb/amazon_massive_intent
name: MTEB MassiveIntentClassification (sw)
config: sw
split: test
revision: 31efe3c427b0bae9c22cbb560b8f15491cc6bed7
metrics:
- type: accuracy
value: 58.500336247478145
- type: f1
value: 55.2972398687929
- task:
type: Classification
dataset:
type: mteb/amazon_massive_intent
name: MTEB MassiveIntentClassification (ta)
config: ta
split: test
revision: 31efe3c427b0bae9c22cbb560b8f15491cc6bed7
metrics:
- type: accuracy
value: 62.68997982515132
- type: f1
value: 59.36848202755348
- task:
type: Classification
dataset:
type: mteb/amazon_massive_intent
name: MTEB MassiveIntentClassification (te)
config: te
split: test
revision: 31efe3c427b0bae9c22cbb560b8f15491cc6bed7
metrics:
- type: accuracy
value: 63.01950235373235
- type: f1
value: 60.09351954625423
- task:
type: Classification
dataset:
type: mteb/amazon_massive_intent
name: MTEB MassiveIntentClassification (th)
config: th
split: test
revision: 31efe3c427b0bae9c22cbb560b8f15491cc6bed7
metrics:
- type: accuracy
value: 68.29186281102892
- type: f1
value: 67.57860496703447
- task:
type: Classification
dataset:
type: mteb/amazon_massive_intent
name: MTEB MassiveIntentClassification (tl)
config: tl
split: test
revision: 31efe3c427b0bae9c22cbb560b8f15491cc6bed7
metrics:
- type: accuracy
value: 64.77471418964357
- type: f1
value: 61.913983147713836
- task:
type: Classification
dataset:
type: mteb/amazon_massive_intent
name: MTEB MassiveIntentClassification (tr)
config: tr
split: test
revision: 31efe3c427b0bae9c22cbb560b8f15491cc6bed7
metrics:
- type: accuracy
value: 69.87222595830532
- type: f1
value: 66.03679033708141
- task:
type: Classification
dataset:
type: mteb/amazon_massive_intent
name: MTEB MassiveIntentClassification (ur)
config: ur
split: test
revision: 31efe3c427b0bae9c22cbb560b8f15491cc6bed7
metrics:
- type: accuracy
value: 64.04505716207127
- type: f1
value: 61.28569169817908
- task:
type: Classification
dataset:
type: mteb/amazon_massive_intent
name: MTEB MassiveIntentClassification (vi)
config: vi
split: test
revision: 31efe3c427b0bae9c22cbb560b8f15491cc6bed7
metrics:
- type: accuracy
value: 69.38466711499663
- type: f1
value: 67.20532357036844
- task:
type: Classification
dataset:
type: mteb/amazon_massive_intent
name: MTEB MassiveIntentClassification (zh-CN)
config: zh-CN
split: test
revision: 31efe3c427b0bae9c22cbb560b8f15491cc6bed7
metrics:
- type: accuracy
value: 71.12306657700067
- type: f1
value: 68.91251226588182
- task:
type: Classification
dataset:
type: mteb/amazon_massive_intent
name: MTEB MassiveIntentClassification (zh-TW)
config: zh-TW
split: test
revision: 31efe3c427b0bae9c22cbb560b8f15491cc6bed7
metrics:
- type: accuracy
value: 66.20040349697378
- type: f1
value: 66.02657347714175
- task:
type: Classification
dataset:
type: mteb/amazon_massive_scenario
name: MTEB MassiveScenarioClassification (af)
config: af
split: test
revision: 7d571f92784cd94a019292a1f45445077d0ef634
metrics:
- type: accuracy
value: 68.73907195696032
- type: f1
value: 66.98484521791418
- task:
type: Classification
dataset:
type: mteb/amazon_massive_scenario
name: MTEB MassiveScenarioClassification (am)
config: am
split: test
revision: 7d571f92784cd94a019292a1f45445077d0ef634
metrics:
- type: accuracy
value: 60.58843308675185
- type: f1
value: 58.95591723092005
- task:
type: Classification
dataset:
type: mteb/amazon_massive_scenario
name: MTEB MassiveScenarioClassification (ar)
config: ar
split: test
revision: 7d571f92784cd94a019292a1f45445077d0ef634
metrics:
- type: accuracy
value: 66.22730329522528
- type: f1
value: 66.0894499712115
- task:
type: Classification
dataset:
type: mteb/amazon_massive_scenario
name: MTEB MassiveScenarioClassification (az)
config: az
split: test
revision: 7d571f92784cd94a019292a1f45445077d0ef634
metrics:
- type: accuracy
value: 66.48285137861465
- type: f1
value: 65.21963176785157
- task:
type: Classification
dataset:
type: mteb/amazon_massive_scenario
name: MTEB MassiveScenarioClassification (bn)
config: bn
split: test
revision: 7d571f92784cd94a019292a1f45445077d0ef634
metrics:
- type: accuracy
value: 67.74714189643578
- type: f1
value: 66.8212192745412
- task:
type: Classification
dataset:
type: mteb/amazon_massive_scenario
name: MTEB MassiveScenarioClassification (cy)
config: cy
split: test
revision: 7d571f92784cd94a019292a1f45445077d0ef634
metrics:
- type: accuracy
value: 59.09213180901143
- type: f1
value: 56.70735546356339
- task:
type: Classification
dataset:
type: mteb/amazon_massive_scenario
name: MTEB MassiveScenarioClassification (da)
config: da
split: test
revision: 7d571f92784cd94a019292a1f45445077d0ef634
metrics:
- type: accuracy
value: 75.05716207128448
- type: f1
value: 74.8413712365364
- task:
type: Classification
dataset:
type: mteb/amazon_massive_scenario
name: MTEB MassiveScenarioClassification (de)
config: de
split: test
revision: 7d571f92784cd94a019292a1f45445077d0ef634
metrics:
- type: accuracy
value: 74.69737726967047
- type: f1
value: 74.7664341963
- task:
type: Classification
dataset:
type: mteb/amazon_massive_scenario
name: MTEB MassiveScenarioClassification (el)
config: el
split: test
revision: 7d571f92784cd94a019292a1f45445077d0ef634
metrics:
- type: accuracy
value: 73.90383322125084
- type: f1
value: 73.59201554448323
- task:
type: Classification
dataset:
type: mteb/amazon_massive_scenario
name: MTEB MassiveScenarioClassification (en)
config: en
split: test
revision: 7d571f92784cd94a019292a1f45445077d0ef634
metrics:
- type: accuracy
value: 77.51176866173503
- type: f1
value: 77.46104434577758
- task:
type: Classification
dataset:
type: mteb/amazon_massive_scenario
name: MTEB MassiveScenarioClassification (es)
config: es
split: test
revision: 7d571f92784cd94a019292a1f45445077d0ef634
metrics:
- type: accuracy
value: 74.31069266980496
- type: f1
value: 74.61048660675635
- task:
type: Classification
dataset:
type: mteb/amazon_massive_scenario
name: MTEB MassiveScenarioClassification (fa)
config: fa
split: test
revision: 7d571f92784cd94a019292a1f45445077d0ef634
metrics:
- type: accuracy
value: 72.95225285810356
- type: f1
value: 72.33160006574627
- task:
type: Classification
dataset:
type: mteb/amazon_massive_scenario
name: MTEB MassiveScenarioClassification (fi)
config: fi
split: test
revision: 7d571f92784cd94a019292a1f45445077d0ef634
metrics:
- type: accuracy
value: 73.12373907195696
- type: f1
value: 73.20921012557481
- task:
type: Classification
dataset:
type: mteb/amazon_massive_scenario
name: MTEB MassiveScenarioClassification (fr)
config: fr
split: test
revision: 7d571f92784cd94a019292a1f45445077d0ef634
metrics:
- type: accuracy
value: 73.86684599865501
- type: f1
value: 73.82348774610831
- task:
type: Classification
dataset:
type: mteb/amazon_massive_scenario
name: MTEB MassiveScenarioClassification (he)
config: he
split: test
revision: 7d571f92784cd94a019292a1f45445077d0ef634
metrics:
- type: accuracy
value: 71.40215198386012
- type: f1
value: 71.11945183971858
- task:
type: Classification
dataset:
type: mteb/amazon_massive_scenario
name: MTEB MassiveScenarioClassification (hi)
config: hi
split: test
revision: 7d571f92784cd94a019292a1f45445077d0ef634
metrics:
- type: accuracy
value: 72.12844653665098
- type: f1
value: 71.34450495911766
- task:
type: Classification
dataset:
type: mteb/amazon_massive_scenario
name: MTEB MassiveScenarioClassification (hu)
config: hu
split: test
revision: 7d571f92784cd94a019292a1f45445077d0ef634
metrics:
- type: accuracy
value: 74.52252858103566
- type: f1
value: 73.98878711342999
- task:
type: Classification
dataset:
type: mteb/amazon_massive_scenario
name: MTEB MassiveScenarioClassification (hy)
config: hy
split: test
revision: 7d571f92784cd94a019292a1f45445077d0ef634
metrics:
- type: accuracy
value: 64.93611297915265
- type: f1
value: 63.723200467653385
- task:
type: Classification
dataset:
type: mteb/amazon_massive_scenario
name: MTEB MassiveScenarioClassification (id)
config: id
split: test
revision: 7d571f92784cd94a019292a1f45445077d0ef634
metrics:
- type: accuracy
value: 74.11903160726295
- type: f1
value: 73.82138439467096
- task:
type: Classification
dataset:
type: mteb/amazon_massive_scenario
name: MTEB MassiveScenarioClassification (is)
config: is
split: test
revision: 7d571f92784cd94a019292a1f45445077d0ef634
metrics:
- type: accuracy
value: 67.15198386012105
- type: f1
value: 66.02172193802167
- task:
type: Classification
dataset:
type: mteb/amazon_massive_scenario
name: MTEB MassiveScenarioClassification (it)
config: it
split: test
revision: 7d571f92784cd94a019292a1f45445077d0ef634
metrics:
- type: accuracy
value: 74.32414256893072
- type: f1
value: 74.30943421170574
- task:
type: Classification
dataset:
type: mteb/amazon_massive_scenario
name: MTEB MassiveScenarioClassification (ja)
config: ja
split: test
revision: 7d571f92784cd94a019292a1f45445077d0ef634
metrics:
- type: accuracy
value: 77.46805648957633
- type: f1
value: 77.62808409298209
- task:
type: Classification
dataset:
type: mteb/amazon_massive_scenario
name: MTEB MassiveScenarioClassification (jv)
config: jv
split: test
revision: 7d571f92784cd94a019292a1f45445077d0ef634
metrics:
- type: accuracy
value: 63.318762609280434
- type: f1
value: 62.094284066075076
- task:
type: Classification
dataset:
type: mteb/amazon_massive_scenario
name: MTEB MassiveScenarioClassification (ka)
config: ka
split: test
revision: 7d571f92784cd94a019292a1f45445077d0ef634
metrics:
- type: accuracy
value: 58.34902488231338
- type: f1
value: 57.12893860987984
- task:
type: Classification
dataset:
type: mteb/amazon_massive_scenario
name: MTEB MassiveScenarioClassification (km)
config: km
split: test
revision: 7d571f92784cd94a019292a1f45445077d0ef634
metrics:
- type: accuracy
value: 50.88433086751849
- type: f1
value: 48.2272350802058
- task:
type: Classification
dataset:
type: mteb/amazon_massive_scenario
name: MTEB MassiveScenarioClassification (kn)
config: kn
split: test
revision: 7d571f92784cd94a019292a1f45445077d0ef634
metrics:
- type: accuracy
value: 66.4425016812374
- type: f1
value: 64.61463095996173
- task:
type: Classification
dataset:
type: mteb/amazon_massive_scenario
name: MTEB MassiveScenarioClassification (ko)
config: ko
split: test
revision: 7d571f92784cd94a019292a1f45445077d0ef634
metrics:
- type: accuracy
value: 75.04707464694015
- type: f1
value: 75.05099199098998
- task:
type: Classification
dataset:
type: mteb/amazon_massive_scenario
name: MTEB MassiveScenarioClassification (lv)
config: lv
split: test
revision: 7d571f92784cd94a019292a1f45445077d0ef634
metrics:
- type: accuracy
value: 70.50437121721586
- type: f1
value: 69.83397721096314
- task:
type: Classification
dataset:
type: mteb/amazon_massive_scenario
name: MTEB MassiveScenarioClassification (ml)
config: ml
split: test
revision: 7d571f92784cd94a019292a1f45445077d0ef634
metrics:
- type: accuracy
value: 69.94283792871553
- type: f1
value: 68.8704663703913
- task:
type: Classification
dataset:
type: mteb/amazon_massive_scenario
name: MTEB MassiveScenarioClassification (mn)
config: mn
split: test
revision: 7d571f92784cd94a019292a1f45445077d0ef634
metrics:
- type: accuracy
value: 64.79488903833222
- type: f1
value: 63.615424063345436
- task:
type: Classification
dataset:
type: mteb/amazon_massive_scenario
name: MTEB MassiveScenarioClassification (ms)
config: ms
split: test
revision: 7d571f92784cd94a019292a1f45445077d0ef634
metrics:
- type: accuracy
value: 69.88231338264963
- type: f1
value: 68.57892302593237
- task:
type: Classification
dataset:
type: mteb/amazon_massive_scenario
name: MTEB MassiveScenarioClassification (my)
config: my
split: test
revision: 7d571f92784cd94a019292a1f45445077d0ef634
metrics:
- type: accuracy
value: 63.248150638870214
- type: f1
value: 61.06680605338809
- task:
type: Classification
dataset:
type: mteb/amazon_massive_scenario
name: MTEB MassiveScenarioClassification (nb)
config: nb
split: test
revision: 7d571f92784cd94a019292a1f45445077d0ef634
metrics:
- type: accuracy
value: 74.84196368527236
- type: f1
value: 74.52566464968763
- task:
type: Classification
dataset:
type: mteb/amazon_massive_scenario
name: MTEB MassiveScenarioClassification (nl)
config: nl
split: test
revision: 7d571f92784cd94a019292a1f45445077d0ef634
metrics:
- type: accuracy
value: 74.8285137861466
- type: f1
value: 74.8853197608802
- task:
type: Classification
dataset:
type: mteb/amazon_massive_scenario
name: MTEB MassiveScenarioClassification (pl)
config: pl
split: test
revision: 7d571f92784cd94a019292a1f45445077d0ef634
metrics:
- type: accuracy
value: 74.13248150638869
- type: f1
value: 74.3982040999179
- task:
type: Classification
dataset:
type: mteb/amazon_massive_scenario
name: MTEB MassiveScenarioClassification (pt)
config: pt
split: test
revision: 7d571f92784cd94a019292a1f45445077d0ef634
metrics:
- type: accuracy
value: 73.49024882313383
- type: f1
value: 73.82153848368573
- task:
type: Classification
dataset:
type: mteb/amazon_massive_scenario
name: MTEB MassiveScenarioClassification (ro)
config: ro
split: test
revision: 7d571f92784cd94a019292a1f45445077d0ef634
metrics:
- type: accuracy
value: 71.72158708809684
- type: f1
value: 71.85049433180541
- task:
type: Classification
dataset:
type: mteb/amazon_massive_scenario
name: MTEB MassiveScenarioClassification (ru)
config: ru
split: test
revision: 7d571f92784cd94a019292a1f45445077d0ef634
metrics:
- type: accuracy
value: 75.137861466039
- type: f1
value: 75.37628348188467
- task:
type: Classification
dataset:
type: mteb/amazon_massive_scenario
name: MTEB MassiveScenarioClassification (sl)
config: sl
split: test
revision: 7d571f92784cd94a019292a1f45445077d0ef634
metrics:
- type: accuracy
value: 71.86953597848016
- type: f1
value: 71.87537624521661
- task:
type: Classification
dataset:
type: mteb/amazon_massive_scenario
name: MTEB MassiveScenarioClassification (sq)
config: sq
split: test
revision: 7d571f92784cd94a019292a1f45445077d0ef634
metrics:
- type: accuracy
value: 70.27572293207801
- type: f1
value: 68.80017302344231
- task:
type: Classification
dataset:
type: mteb/amazon_massive_scenario
name: MTEB MassiveScenarioClassification (sv)
config: sv
split: test
revision: 7d571f92784cd94a019292a1f45445077d0ef634
metrics:
- type: accuracy
value: 76.09952925353059
- type: f1
value: 76.07992707688408
- task:
type: Classification
dataset:
type: mteb/amazon_massive_scenario
name: MTEB MassiveScenarioClassification (sw)
config: sw
split: test
revision: 7d571f92784cd94a019292a1f45445077d0ef634
metrics:
- type: accuracy
value: 63.140551445864155
- type: f1
value: 61.73855010331415
- task:
type: Classification
dataset:
type: mteb/amazon_massive_scenario
name: MTEB MassiveScenarioClassification (ta)
config: ta
split: test
revision: 7d571f92784cd94a019292a1f45445077d0ef634
metrics:
- type: accuracy
value: 66.27774041694687
- type: f1
value: 64.83664868894539
- task:
type: Classification
dataset:
type: mteb/amazon_massive_scenario
name: MTEB MassiveScenarioClassification (te)
config: te
split: test
revision: 7d571f92784cd94a019292a1f45445077d0ef634
metrics:
- type: accuracy
value: 66.69468728984533
- type: f1
value: 64.76239666920868
- task:
type: Classification
dataset:
type: mteb/amazon_massive_scenario
name: MTEB MassiveScenarioClassification (th)
config: th
split: test
revision: 7d571f92784cd94a019292a1f45445077d0ef634
metrics:
- type: accuracy
value: 73.44653665097512
- type: f1
value: 73.14646052013873
- task:
type: Classification
dataset:
type: mteb/amazon_massive_scenario
name: MTEB MassiveScenarioClassification (tl)
config: tl
split: test
revision: 7d571f92784cd94a019292a1f45445077d0ef634
metrics:
- type: accuracy
value: 67.71351714862139
- type: f1
value: 66.67212180163382
- task:
type: Classification
dataset:
type: mteb/amazon_massive_scenario
name: MTEB MassiveScenarioClassification (tr)
config: tr
split: test
revision: 7d571f92784cd94a019292a1f45445077d0ef634
metrics:
- type: accuracy
value: 73.9946200403497
- type: f1
value: 73.87348793725525
- task:
type: Classification
dataset:
type: mteb/amazon_massive_scenario
name: MTEB MassiveScenarioClassification (ur)
config: ur
split: test
revision: 7d571f92784cd94a019292a1f45445077d0ef634
metrics:
- type: accuracy
value: 68.15400134498992
- type: f1
value: 67.09433241421094
- task:
type: Classification
dataset:
type: mteb/amazon_massive_scenario
name: MTEB MassiveScenarioClassification (vi)
config: vi
split: test
revision: 7d571f92784cd94a019292a1f45445077d0ef634
metrics:
- type: accuracy
value: 73.11365164761264
- type: f1
value: 73.59502539433753
- task:
type: Classification
dataset:
type: mteb/amazon_massive_scenario
name: MTEB MassiveScenarioClassification (zh-CN)
config: zh-CN
split: test
revision: 7d571f92784cd94a019292a1f45445077d0ef634
metrics:
- type: accuracy
value: 76.82582380632145
- type: f1
value: 76.89992945316313
- task:
type: Classification
dataset:
type: mteb/amazon_massive_scenario
name: MTEB MassiveScenarioClassification (zh-TW)
config: zh-TW
split: test
revision: 7d571f92784cd94a019292a1f45445077d0ef634
metrics:
- type: accuracy
value: 71.81237390719569
- type: f1
value: 72.36499770986265
- task:
type: Clustering
dataset:
type: mteb/medrxiv-clustering-p2p
name: MTEB MedrxivClusteringP2P
config: default
split: test
revision: e7a26af6f3ae46b30dde8737f02c07b1505bcc73
metrics:
- type: v_measure
value: 31.480506569594695
- task:
type: Clustering
dataset:
type: mteb/medrxiv-clustering-s2s
name: MTEB MedrxivClusteringS2S
config: default
split: test
revision: 35191c8c0dca72d8ff3efcd72aa802307d469663
metrics:
- type: v_measure
value: 29.71252128004552
- task:
type: Reranking
dataset:
type: mteb/mind_small
name: MTEB MindSmallReranking
config: default
split: test
revision: 3bdac13927fdc888b903db93b2ffdbd90b295a69
metrics:
- type: map
value: 31.421396787056548
- type: mrr
value: 32.48155274872267
- task:
type: Retrieval
dataset:
type: nfcorpus
name: MTEB NFCorpus
config: default
split: test
revision: None
metrics:
- type: map_at_1
value: 5.595
- type: map_at_10
value: 12.642000000000001
- type: map_at_100
value: 15.726
- type: map_at_1000
value: 17.061999999999998
- type: map_at_3
value: 9.125
- type: map_at_5
value: 10.866000000000001
- type: mrr_at_1
value: 43.344
- type: mrr_at_10
value: 52.227999999999994
- type: mrr_at_100
value: 52.898999999999994
- type: mrr_at_1000
value: 52.944
- type: mrr_at_3
value: 49.845
- type: mrr_at_5
value: 51.115
- type: ndcg_at_1
value: 41.949999999999996
- type: ndcg_at_10
value: 33.995
- type: ndcg_at_100
value: 30.869999999999997
- type: ndcg_at_1000
value: 39.487
- type: ndcg_at_3
value: 38.903999999999996
- type: ndcg_at_5
value: 37.236999999999995
- type: precision_at_1
value: 43.344
- type: precision_at_10
value: 25.480000000000004
- type: precision_at_100
value: 7.672
- type: precision_at_1000
value: 2.028
- type: precision_at_3
value: 36.636
- type: precision_at_5
value: 32.632
- type: recall_at_1
value: 5.595
- type: recall_at_10
value: 16.466
- type: recall_at_100
value: 31.226
- type: recall_at_1000
value: 62.778999999999996
- type: recall_at_3
value: 9.931
- type: recall_at_5
value: 12.884
- task:
type: Retrieval
dataset:
type: nq
name: MTEB NQ
config: default
split: test
revision: None
metrics:
- type: map_at_1
value: 40.414
- type: map_at_10
value: 56.754000000000005
- type: map_at_100
value: 57.457
- type: map_at_1000
value: 57.477999999999994
- type: map_at_3
value: 52.873999999999995
- type: map_at_5
value: 55.175
- type: mrr_at_1
value: 45.278
- type: mrr_at_10
value: 59.192
- type: mrr_at_100
value: 59.650000000000006
- type: mrr_at_1000
value: 59.665
- type: mrr_at_3
value: 56.141
- type: mrr_at_5
value: 57.998000000000005
- type: ndcg_at_1
value: 45.278
- type: ndcg_at_10
value: 64.056
- type: ndcg_at_100
value: 66.89
- type: ndcg_at_1000
value: 67.364
- type: ndcg_at_3
value: 56.97
- type: ndcg_at_5
value: 60.719
- type: precision_at_1
value: 45.278
- type: precision_at_10
value: 9.994
- type: precision_at_100
value: 1.165
- type: precision_at_1000
value: 0.121
- type: precision_at_3
value: 25.512
- type: precision_at_5
value: 17.509
- type: recall_at_1
value: 40.414
- type: recall_at_10
value: 83.596
- type: recall_at_100
value: 95.72
- type: recall_at_1000
value: 99.24
- type: recall_at_3
value: 65.472
- type: recall_at_5
value: 74.039
- task:
type: Retrieval
dataset:
type: quora
name: MTEB QuoraRetrieval
config: default
split: test
revision: None
metrics:
- type: map_at_1
value: 70.352
- type: map_at_10
value: 84.369
- type: map_at_100
value: 85.02499999999999
- type: map_at_1000
value: 85.04
- type: map_at_3
value: 81.42399999999999
- type: map_at_5
value: 83.279
- type: mrr_at_1
value: 81.05
- type: mrr_at_10
value: 87.401
- type: mrr_at_100
value: 87.504
- type: mrr_at_1000
value: 87.505
- type: mrr_at_3
value: 86.443
- type: mrr_at_5
value: 87.10799999999999
- type: ndcg_at_1
value: 81.04
- type: ndcg_at_10
value: 88.181
- type: ndcg_at_100
value: 89.411
- type: ndcg_at_1000
value: 89.507
- type: ndcg_at_3
value: 85.28099999999999
- type: ndcg_at_5
value: 86.888
- type: precision_at_1
value: 81.04
- type: precision_at_10
value: 13.406
- type: precision_at_100
value: 1.5350000000000001
- type: precision_at_1000
value: 0.157
- type: precision_at_3
value: 37.31
- type: precision_at_5
value: 24.54
- type: recall_at_1
value: 70.352
- type: recall_at_10
value: 95.358
- type: recall_at_100
value: 99.541
- type: recall_at_1000
value: 99.984
- type: recall_at_3
value: 87.111
- type: recall_at_5
value: 91.643
- task:
type: Clustering
dataset:
type: mteb/reddit-clustering
name: MTEB RedditClustering
config: default
split: test
revision: 24640382cdbf8abc73003fb0fa6d111a705499eb
metrics:
- type: v_measure
value: 46.54068723291946
- task:
type: Clustering
dataset:
type: mteb/reddit-clustering-p2p
name: MTEB RedditClusteringP2P
config: default
split: test
revision: 282350215ef01743dc01b456c7f5241fa8937f16
metrics:
- type: v_measure
value: 63.216287629895994
- task:
type: Retrieval
dataset:
type: scidocs
name: MTEB SCIDOCS
config: default
split: test
revision: None
metrics:
- type: map_at_1
value: 4.023000000000001
- type: map_at_10
value: 10.071
- type: map_at_100
value: 11.892
- type: map_at_1000
value: 12.196
- type: map_at_3
value: 7.234
- type: map_at_5
value: 8.613999999999999
- type: mrr_at_1
value: 19.900000000000002
- type: mrr_at_10
value: 30.516
- type: mrr_at_100
value: 31.656000000000002
- type: mrr_at_1000
value: 31.723000000000003
- type: mrr_at_3
value: 27.400000000000002
- type: mrr_at_5
value: 29.270000000000003
- type: ndcg_at_1
value: 19.900000000000002
- type: ndcg_at_10
value: 17.474
- type: ndcg_at_100
value: 25.020999999999997
- type: ndcg_at_1000
value: 30.728
- type: ndcg_at_3
value: 16.588
- type: ndcg_at_5
value: 14.498
- type: precision_at_1
value: 19.900000000000002
- type: precision_at_10
value: 9.139999999999999
- type: precision_at_100
value: 2.011
- type: precision_at_1000
value: 0.33899999999999997
- type: precision_at_3
value: 15.667
- type: precision_at_5
value: 12.839999999999998
- type: recall_at_1
value: 4.023000000000001
- type: recall_at_10
value: 18.497
- type: recall_at_100
value: 40.8
- type: recall_at_1000
value: 68.812
- type: recall_at_3
value: 9.508
- type: recall_at_5
value: 12.983
- task:
type: STS
dataset:
type: mteb/sickr-sts
name: MTEB SICK-R
config: default
split: test
revision: a6ea5a8cab320b040a23452cc28066d9beae2cee
metrics:
- type: cos_sim_pearson
value: 83.967008785134
- type: cos_sim_spearman
value: 80.23142141101837
- type: euclidean_pearson
value: 81.20166064704539
- type: euclidean_spearman
value: 80.18961335654585
- type: manhattan_pearson
value: 81.13925443187625
- type: manhattan_spearman
value: 80.07948723044424
- task:
type: STS
dataset:
type: mteb/sts12-sts
name: MTEB STS12
config: default
split: test
revision: a0d554a64d88156834ff5ae9920b964011b16384
metrics:
- type: cos_sim_pearson
value: 86.94262461316023
- type: cos_sim_spearman
value: 80.01596278563865
- type: euclidean_pearson
value: 83.80799622922581
- type: euclidean_spearman
value: 79.94984954947103
- type: manhattan_pearson
value: 83.68473841756281
- type: manhattan_spearman
value: 79.84990707951822
- task:
type: STS
dataset:
type: mteb/sts13-sts
name: MTEB STS13
config: default
split: test
revision: 7e90230a92c190f1bf69ae9002b8cea547a64cca
metrics:
- type: cos_sim_pearson
value: 80.57346443146068
- type: cos_sim_spearman
value: 81.54689837570866
- type: euclidean_pearson
value: 81.10909881516007
- type: euclidean_spearman
value: 81.56746243261762
- type: manhattan_pearson
value: 80.87076036186582
- type: manhattan_spearman
value: 81.33074987964402
- task:
type: STS
dataset:
type: mteb/sts14-sts
name: MTEB STS14
config: default
split: test
revision: 6031580fec1f6af667f0bd2da0a551cf4f0b2375
metrics:
- type: cos_sim_pearson
value: 79.54733787179849
- type: cos_sim_spearman
value: 77.72202105610411
- type: euclidean_pearson
value: 78.9043595478849
- type: euclidean_spearman
value: 77.93422804309435
- type: manhattan_pearson
value: 78.58115121621368
- type: manhattan_spearman
value: 77.62508135122033
- task:
type: STS
dataset:
type: mteb/sts15-sts
name: MTEB STS15
config: default
split: test
revision: ae752c7c21bf194d8b67fd573edf7ae58183cbe3
metrics:
- type: cos_sim_pearson
value: 88.59880017237558
- type: cos_sim_spearman
value: 89.31088630824758
- type: euclidean_pearson
value: 88.47069261564656
- type: euclidean_spearman
value: 89.33581971465233
- type: manhattan_pearson
value: 88.40774264100956
- type: manhattan_spearman
value: 89.28657485627835
- task:
type: STS
dataset:
type: mteb/sts16-sts
name: MTEB STS16
config: default
split: test
revision: 4d8694f8f0e0100860b497b999b3dbed754a0513
metrics:
- type: cos_sim_pearson
value: 84.08055117917084
- type: cos_sim_spearman
value: 85.78491813080304
- type: euclidean_pearson
value: 84.99329155500392
- type: euclidean_spearman
value: 85.76728064677287
- type: manhattan_pearson
value: 84.87947428989587
- type: manhattan_spearman
value: 85.62429454917464
- task:
type: STS
dataset:
type: mteb/sts17-crosslingual-sts
name: MTEB STS17 (ko-ko)
config: ko-ko
split: test
revision: af5e6fb845001ecf41f4c1e033ce921939a2a68d
metrics:
- type: cos_sim_pearson
value: 82.14190939287384
- type: cos_sim_spearman
value: 82.27331573306041
- type: euclidean_pearson
value: 81.891896953716
- type: euclidean_spearman
value: 82.37695542955998
- type: manhattan_pearson
value: 81.73123869460504
- type: manhattan_spearman
value: 82.19989168441421
- task:
type: STS
dataset:
type: mteb/sts17-crosslingual-sts
name: MTEB STS17 (ar-ar)
config: ar-ar
split: test
revision: af5e6fb845001ecf41f4c1e033ce921939a2a68d
metrics:
- type: cos_sim_pearson
value: 76.84695301843362
- type: cos_sim_spearman
value: 77.87790986014461
- type: euclidean_pearson
value: 76.91981583106315
- type: euclidean_spearman
value: 77.88154772749589
- type: manhattan_pearson
value: 76.94953277451093
- type: manhattan_spearman
value: 77.80499230728604
- task:
type: STS
dataset:
type: mteb/sts17-crosslingual-sts
name: MTEB STS17 (en-ar)
config: en-ar
split: test
revision: af5e6fb845001ecf41f4c1e033ce921939a2a68d
metrics:
- type: cos_sim_pearson
value: 75.44657840482016
- type: cos_sim_spearman
value: 75.05531095119674
- type: euclidean_pearson
value: 75.88161755829299
- type: euclidean_spearman
value: 74.73176238219332
- type: manhattan_pearson
value: 75.63984765635362
- type: manhattan_spearman
value: 74.86476440770737
- task:
type: STS
dataset:
type: mteb/sts17-crosslingual-sts
name: MTEB STS17 (en-de)
config: en-de
split: test
revision: af5e6fb845001ecf41f4c1e033ce921939a2a68d
metrics:
- type: cos_sim_pearson
value: 85.64700140524133
- type: cos_sim_spearman
value: 86.16014210425672
- type: euclidean_pearson
value: 86.49086860843221
- type: euclidean_spearman
value: 86.09729326815614
- type: manhattan_pearson
value: 86.43406265125513
- type: manhattan_spearman
value: 86.17740150939994
- task:
type: STS
dataset:
type: mteb/sts17-crosslingual-sts
name: MTEB STS17 (en-en)
config: en-en
split: test
revision: af5e6fb845001ecf41f4c1e033ce921939a2a68d
metrics:
- type: cos_sim_pearson
value: 87.91170098764921
- type: cos_sim_spearman
value: 88.12437004058931
- type: euclidean_pearson
value: 88.81828254494437
- type: euclidean_spearman
value: 88.14831794572122
- type: manhattan_pearson
value: 88.93442183448961
- type: manhattan_spearman
value: 88.15254630778304
- task:
type: STS
dataset:
type: mteb/sts17-crosslingual-sts
name: MTEB STS17 (en-tr)
config: en-tr
split: test
revision: af5e6fb845001ecf41f4c1e033ce921939a2a68d
metrics:
- type: cos_sim_pearson
value: 72.91390577997292
- type: cos_sim_spearman
value: 71.22979457536074
- type: euclidean_pearson
value: 74.40314008106749
- type: euclidean_spearman
value: 72.54972136083246
- type: manhattan_pearson
value: 73.85687539530218
- type: manhattan_spearman
value: 72.09500771742637
- task:
type: STS
dataset:
type: mteb/sts17-crosslingual-sts
name: MTEB STS17 (es-en)
config: es-en
split: test
revision: af5e6fb845001ecf41f4c1e033ce921939a2a68d
metrics:
- type: cos_sim_pearson
value: 80.9301067983089
- type: cos_sim_spearman
value: 80.74989828346473
- type: euclidean_pearson
value: 81.36781301814257
- type: euclidean_spearman
value: 80.9448819964426
- type: manhattan_pearson
value: 81.0351322685609
- type: manhattan_spearman
value: 80.70192121844177
- task:
type: STS
dataset:
type: mteb/sts17-crosslingual-sts
name: MTEB STS17 (es-es)
config: es-es
split: test
revision: af5e6fb845001ecf41f4c1e033ce921939a2a68d
metrics:
- type: cos_sim_pearson
value: 87.13820465980005
- type: cos_sim_spearman
value: 86.73532498758757
- type: euclidean_pearson
value: 87.21329451846637
- type: euclidean_spearman
value: 86.57863198601002
- type: manhattan_pearson
value: 87.06973713818554
- type: manhattan_spearman
value: 86.47534918791499
- task:
type: STS
dataset:
type: mteb/sts17-crosslingual-sts
name: MTEB STS17 (fr-en)
config: fr-en
split: test
revision: af5e6fb845001ecf41f4c1e033ce921939a2a68d
metrics:
- type: cos_sim_pearson
value: 85.48720108904415
- type: cos_sim_spearman
value: 85.62221757068387
- type: euclidean_pearson
value: 86.1010129512749
- type: euclidean_spearman
value: 85.86580966509942
- type: manhattan_pearson
value: 86.26800938808971
- type: manhattan_spearman
value: 85.88902721678429
- task:
type: STS
dataset:
type: mteb/sts17-crosslingual-sts
name: MTEB STS17 (it-en)
config: it-en
split: test
revision: af5e6fb845001ecf41f4c1e033ce921939a2a68d
metrics:
- type: cos_sim_pearson
value: 83.98021347333516
- type: cos_sim_spearman
value: 84.53806553803501
- type: euclidean_pearson
value: 84.61483347248364
- type: euclidean_spearman
value: 85.14191408011702
- type: manhattan_pearson
value: 84.75297588825967
- type: manhattan_spearman
value: 85.33176753669242
- task:
type: STS
dataset:
type: mteb/sts17-crosslingual-sts
name: MTEB STS17 (nl-en)
config: nl-en
split: test
revision: af5e6fb845001ecf41f4c1e033ce921939a2a68d
metrics:
- type: cos_sim_pearson
value: 84.51856644893233
- type: cos_sim_spearman
value: 85.27510748506413
- type: euclidean_pearson
value: 85.09886861540977
- type: euclidean_spearman
value: 85.62579245860887
- type: manhattan_pearson
value: 84.93017860464607
- type: manhattan_spearman
value: 85.5063988898453
- task:
type: STS
dataset:
type: mteb/sts22-crosslingual-sts
name: MTEB STS22 (en)
config: en
split: test
revision: 6d1ba47164174a496b7fa5d3569dae26a6813b80
metrics:
- type: cos_sim_pearson
value: 62.581573200584195
- type: cos_sim_spearman
value: 63.05503590247928
- type: euclidean_pearson
value: 63.652564812602094
- type: euclidean_spearman
value: 62.64811520876156
- type: manhattan_pearson
value: 63.506842893061076
- type: manhattan_spearman
value: 62.51289573046917
- task:
type: STS
dataset:
type: mteb/sts22-crosslingual-sts
name: MTEB STS22 (de)
config: de
split: test
revision: 6d1ba47164174a496b7fa5d3569dae26a6813b80
metrics:
- type: cos_sim_pearson
value: 48.2248801729127
- type: cos_sim_spearman
value: 56.5936604678561
- type: euclidean_pearson
value: 43.98149464089
- type: euclidean_spearman
value: 56.108561882423615
- type: manhattan_pearson
value: 43.86880305903564
- type: manhattan_spearman
value: 56.04671150510166
- task:
type: STS
dataset:
type: mteb/sts22-crosslingual-sts
name: MTEB STS22 (es)
config: es
split: test
revision: 6d1ba47164174a496b7fa5d3569dae26a6813b80
metrics:
- type: cos_sim_pearson
value: 55.17564527009831
- type: cos_sim_spearman
value: 64.57978560979488
- type: euclidean_pearson
value: 58.8818330154583
- type: euclidean_spearman
value: 64.99214839071281
- type: manhattan_pearson
value: 58.72671436121381
- type: manhattan_spearman
value: 65.10713416616109
- task:
type: STS
dataset:
type: mteb/sts22-crosslingual-sts
name: MTEB STS22 (pl)
config: pl
split: test
revision: 6d1ba47164174a496b7fa5d3569dae26a6813b80
metrics:
- type: cos_sim_pearson
value: 26.772131864023297
- type: cos_sim_spearman
value: 34.68200792408681
- type: euclidean_pearson
value: 16.68082419005441
- type: euclidean_spearman
value: 34.83099932652166
- type: manhattan_pearson
value: 16.52605949659529
- type: manhattan_spearman
value: 34.82075801399475
- task:
type: STS
dataset:
type: mteb/sts22-crosslingual-sts
name: MTEB STS22 (tr)
config: tr
split: test
revision: 6d1ba47164174a496b7fa5d3569dae26a6813b80
metrics:
- type: cos_sim_pearson
value: 54.42415189043831
- type: cos_sim_spearman
value: 63.54594264576758
- type: euclidean_pearson
value: 57.36577498297745
- type: euclidean_spearman
value: 63.111466379158074
- type: manhattan_pearson
value: 57.584543715873885
- type: manhattan_spearman
value: 63.22361054139183
- task:
type: STS
dataset:
type: mteb/sts22-crosslingual-sts
name: MTEB STS22 (ar)
config: ar
split: test
revision: 6d1ba47164174a496b7fa5d3569dae26a6813b80
metrics:
- type: cos_sim_pearson
value: 47.55216762405518
- type: cos_sim_spearman
value: 56.98670142896412
- type: euclidean_pearson
value: 50.15318757562699
- type: euclidean_spearman
value: 56.524941926541906
- type: manhattan_pearson
value: 49.955618528674904
- type: manhattan_spearman
value: 56.37102209240117
- task:
type: STS
dataset:
type: mteb/sts22-crosslingual-sts
name: MTEB STS22 (ru)
config: ru
split: test
revision: 6d1ba47164174a496b7fa5d3569dae26a6813b80
metrics:
- type: cos_sim_pearson
value: 49.20540980338571
- type: cos_sim_spearman
value: 59.9009453504406
- type: euclidean_pearson
value: 49.557749853620535
- type: euclidean_spearman
value: 59.76631621172456
- type: manhattan_pearson
value: 49.62340591181147
- type: manhattan_spearman
value: 59.94224880322436
- task:
type: STS
dataset:
type: mteb/sts22-crosslingual-sts
name: MTEB STS22 (zh)
config: zh
split: test
revision: 6d1ba47164174a496b7fa5d3569dae26a6813b80
metrics:
- type: cos_sim_pearson
value: 51.508169956576985
- type: cos_sim_spearman
value: 66.82461565306046
- type: euclidean_pearson
value: 56.2274426480083
- type: euclidean_spearman
value: 66.6775323848333
- type: manhattan_pearson
value: 55.98277796300661
- type: manhattan_spearman
value: 66.63669848497175
- task:
type: STS
dataset:
type: mteb/sts22-crosslingual-sts
name: MTEB STS22 (fr)
config: fr
split: test
revision: 6d1ba47164174a496b7fa5d3569dae26a6813b80
metrics:
- type: cos_sim_pearson
value: 72.86478788045507
- type: cos_sim_spearman
value: 76.7946552053193
- type: euclidean_pearson
value: 75.01598530490269
- type: euclidean_spearman
value: 76.83618917858281
- type: manhattan_pearson
value: 74.68337628304332
- type: manhattan_spearman
value: 76.57480204017773
- task:
type: STS
dataset:
type: mteb/sts22-crosslingual-sts
name: MTEB STS22 (de-en)
config: de-en
split: test
revision: 6d1ba47164174a496b7fa5d3569dae26a6813b80
metrics:
- type: cos_sim_pearson
value: 55.922619099401984
- type: cos_sim_spearman
value: 56.599362477240774
- type: euclidean_pearson
value: 56.68307052369783
- type: euclidean_spearman
value: 54.28760436777401
- type: manhattan_pearson
value: 56.67763566500681
- type: manhattan_spearman
value: 53.94619541711359
- task:
type: STS
dataset:
type: mteb/sts22-crosslingual-sts
name: MTEB STS22 (es-en)
config: es-en
split: test
revision: 6d1ba47164174a496b7fa5d3569dae26a6813b80
metrics:
- type: cos_sim_pearson
value: 66.74357206710913
- type: cos_sim_spearman
value: 72.5208244925311
- type: euclidean_pearson
value: 67.49254562186032
- type: euclidean_spearman
value: 72.02469076238683
- type: manhattan_pearson
value: 67.45251772238085
- type: manhattan_spearman
value: 72.05538819984538
- task:
type: STS
dataset:
type: mteb/sts22-crosslingual-sts
name: MTEB STS22 (it)
config: it
split: test
revision: 6d1ba47164174a496b7fa5d3569dae26a6813b80
metrics:
- type: cos_sim_pearson
value: 71.25734330033191
- type: cos_sim_spearman
value: 76.98349083946823
- type: euclidean_pearson
value: 73.71642838667736
- type: euclidean_spearman
value: 77.01715504651384
- type: manhattan_pearson
value: 73.61712711868105
- type: manhattan_spearman
value: 77.01392571153896
- task:
type: STS
dataset:
type: mteb/sts22-crosslingual-sts
name: MTEB STS22 (pl-en)
config: pl-en
split: test
revision: 6d1ba47164174a496b7fa5d3569dae26a6813b80
metrics:
- type: cos_sim_pearson
value: 63.18215462781212
- type: cos_sim_spearman
value: 65.54373266117607
- type: euclidean_pearson
value: 64.54126095439005
- type: euclidean_spearman
value: 65.30410369102711
- type: manhattan_pearson
value: 63.50332221148234
- type: manhattan_spearman
value: 64.3455878104313
- task:
type: STS
dataset:
type: mteb/sts22-crosslingual-sts
name: MTEB STS22 (zh-en)
config: zh-en
split: test
revision: 6d1ba47164174a496b7fa5d3569dae26a6813b80
metrics:
- type: cos_sim_pearson
value: 62.30509221440029
- type: cos_sim_spearman
value: 65.99582704642478
- type: euclidean_pearson
value: 63.43818859884195
- type: euclidean_spearman
value: 66.83172582815764
- type: manhattan_pearson
value: 63.055779168508764
- type: manhattan_spearman
value: 65.49585020501449
- task:
type: STS
dataset:
type: mteb/sts22-crosslingual-sts
name: MTEB STS22 (es-it)
config: es-it
split: test
revision: 6d1ba47164174a496b7fa5d3569dae26a6813b80
metrics:
- type: cos_sim_pearson
value: 59.587830825340404
- type: cos_sim_spearman
value: 68.93467614588089
- type: euclidean_pearson
value: 62.3073527367404
- type: euclidean_spearman
value: 69.69758171553175
- type: manhattan_pearson
value: 61.9074580815789
- type: manhattan_spearman
value: 69.57696375597865
- task:
type: STS
dataset:
type: mteb/sts22-crosslingual-sts
name: MTEB STS22 (de-fr)
config: de-fr
split: test
revision: 6d1ba47164174a496b7fa5d3569dae26a6813b80
metrics:
- type: cos_sim_pearson
value: 57.143220125577066
- type: cos_sim_spearman
value: 67.78857859159226
- type: euclidean_pearson
value: 55.58225107923733
- type: euclidean_spearman
value: 67.80662907184563
- type: manhattan_pearson
value: 56.24953502726514
- type: manhattan_spearman
value: 67.98262125431616
- task:
type: STS
dataset:
type: mteb/sts22-crosslingual-sts
name: MTEB STS22 (de-pl)
config: de-pl
split: test
revision: 6d1ba47164174a496b7fa5d3569dae26a6813b80
metrics:
- type: cos_sim_pearson
value: 21.826928900322066
- type: cos_sim_spearman
value: 49.578506634400405
- type: euclidean_pearson
value: 27.939890138843214
- type: euclidean_spearman
value: 52.71950519136242
- type: manhattan_pearson
value: 26.39878683847546
- type: manhattan_spearman
value: 47.54609580342499
- task:
type: STS
dataset:
type: mteb/sts22-crosslingual-sts
name: MTEB STS22 (fr-pl)
config: fr-pl
split: test
revision: 6d1ba47164174a496b7fa5d3569dae26a6813b80
metrics:
- type: cos_sim_pearson
value: 57.27603854632001
- type: cos_sim_spearman
value: 50.709255283710995
- type: euclidean_pearson
value: 59.5419024445929
- type: euclidean_spearman
value: 50.709255283710995
- type: manhattan_pearson
value: 59.03256832438492
- type: manhattan_spearman
value: 61.97797868009122
- task:
type: STS
dataset:
type: mteb/stsbenchmark-sts
name: MTEB STSBenchmark
config: default
split: test
revision: b0fddb56ed78048fa8b90373c8a3cfc37b684831
metrics:
- type: cos_sim_pearson
value: 85.00757054859712
- type: cos_sim_spearman
value: 87.29283629622222
- type: euclidean_pearson
value: 86.54824171775536
- type: euclidean_spearman
value: 87.24364730491402
- type: manhattan_pearson
value: 86.5062156915074
- type: manhattan_spearman
value: 87.15052170378574
- task:
type: Reranking
dataset:
type: mteb/scidocs-reranking
name: MTEB SciDocsRR
config: default
split: test
revision: d3c5e1fc0b855ab6097bf1cda04dd73947d7caab
metrics:
- type: map
value: 82.03549357197389
- type: mrr
value: 95.05437645143527
- task:
type: Retrieval
dataset:
type: scifact
name: MTEB SciFact
config: default
split: test
revision: None
metrics:
- type: map_at_1
value: 57.260999999999996
- type: map_at_10
value: 66.259
- type: map_at_100
value: 66.884
- type: map_at_1000
value: 66.912
- type: map_at_3
value: 63.685
- type: map_at_5
value: 65.35499999999999
- type: mrr_at_1
value: 60.333000000000006
- type: mrr_at_10
value: 67.5
- type: mrr_at_100
value: 68.013
- type: mrr_at_1000
value: 68.038
- type: mrr_at_3
value: 65.61099999999999
- type: mrr_at_5
value: 66.861
- type: ndcg_at_1
value: 60.333000000000006
- type: ndcg_at_10
value: 70.41
- type: ndcg_at_100
value: 73.10600000000001
- type: ndcg_at_1000
value: 73.846
- type: ndcg_at_3
value: 66.133
- type: ndcg_at_5
value: 68.499
- type: precision_at_1
value: 60.333000000000006
- type: precision_at_10
value: 9.232999999999999
- type: precision_at_100
value: 1.0630000000000002
- type: precision_at_1000
value: 0.11299999999999999
- type: precision_at_3
value: 25.667
- type: precision_at_5
value: 17.067
- type: recall_at_1
value: 57.260999999999996
- type: recall_at_10
value: 81.94399999999999
- type: recall_at_100
value: 93.867
- type: recall_at_1000
value: 99.667
- type: recall_at_3
value: 70.339
- type: recall_at_5
value: 76.25
- task:
type: PairClassification
dataset:
type: mteb/sprintduplicatequestions-pairclassification
name: MTEB SprintDuplicateQuestions
config: default
split: test
revision: d66bd1f72af766a5cc4b0ca5e00c162f89e8cc46
metrics:
- type: cos_sim_accuracy
value: 99.74356435643564
- type: cos_sim_ap
value: 93.13411948212683
- type: cos_sim_f1
value: 86.80521991300147
- type: cos_sim_precision
value: 84.00374181478017
- type: cos_sim_recall
value: 89.8
- type: dot_accuracy
value: 99.67920792079208
- type: dot_ap
value: 89.27277565444479
- type: dot_f1
value: 83.9276990718124
- type: dot_precision
value: 82.04393505253104
- type: dot_recall
value: 85.9
- type: euclidean_accuracy
value: 99.74257425742574
- type: euclidean_ap
value: 93.17993008259062
- type: euclidean_f1
value: 86.69396110542476
- type: euclidean_precision
value: 88.78406708595388
- type: euclidean_recall
value: 84.7
- type: manhattan_accuracy
value: 99.74257425742574
- type: manhattan_ap
value: 93.14413755550099
- type: manhattan_f1
value: 86.82483594144371
- type: manhattan_precision
value: 87.66564729867483
- type: manhattan_recall
value: 86
- type: max_accuracy
value: 99.74356435643564
- type: max_ap
value: 93.17993008259062
- type: max_f1
value: 86.82483594144371
- task:
type: Clustering
dataset:
type: mteb/stackexchange-clustering
name: MTEB StackExchangeClustering
config: default
split: test
revision: 6cbc1f7b2bc0622f2e39d2c77fa502909748c259
metrics:
- type: v_measure
value: 57.525863806168566
- task:
type: Clustering
dataset:
type: mteb/stackexchange-clustering-p2p
name: MTEB StackExchangeClusteringP2P
config: default
split: test
revision: 815ca46b2622cec33ccafc3735d572c266efdb44
metrics:
- type: v_measure
value: 32.68850574423839
- task:
type: Reranking
dataset:
type: mteb/stackoverflowdupquestions-reranking
name: MTEB StackOverflowDupQuestions
config: default
split: test
revision: e185fbe320c72810689fc5848eb6114e1ef5ec69
metrics:
- type: map
value: 49.71580650644033
- type: mrr
value: 50.50971903913081
- task:
type: Summarization
dataset:
type: mteb/summeval
name: MTEB SummEval
config: default
split: test
revision: cda12ad7615edc362dbf25a00fdd61d3b1eaf93c
metrics:
- type: cos_sim_pearson
value: 29.152190498799484
- type: cos_sim_spearman
value: 29.686180371952727
- type: dot_pearson
value: 27.248664793816342
- type: dot_spearman
value: 28.37748983721745
- task:
type: Retrieval
dataset:
type: trec-covid
name: MTEB TRECCOVID
config: default
split: test
revision: None
metrics:
- type: map_at_1
value: 0.20400000000000001
- type: map_at_10
value: 1.6209999999999998
- type: map_at_100
value: 9.690999999999999
- type: map_at_1000
value: 23.733
- type: map_at_3
value: 0.575
- type: map_at_5
value: 0.885
- type: mrr_at_1
value: 78
- type: mrr_at_10
value: 86.56700000000001
- type: mrr_at_100
value: 86.56700000000001
- type: mrr_at_1000
value: 86.56700000000001
- type: mrr_at_3
value: 85.667
- type: mrr_at_5
value: 86.56700000000001
- type: ndcg_at_1
value: 76
- type: ndcg_at_10
value: 71.326
- type: ndcg_at_100
value: 54.208999999999996
- type: ndcg_at_1000
value: 49.252
- type: ndcg_at_3
value: 74.235
- type: ndcg_at_5
value: 73.833
- type: precision_at_1
value: 78
- type: precision_at_10
value: 74.8
- type: precision_at_100
value: 55.50000000000001
- type: precision_at_1000
value: 21.836
- type: precision_at_3
value: 78
- type: precision_at_5
value: 78
- type: recall_at_1
value: 0.20400000000000001
- type: recall_at_10
value: 1.894
- type: recall_at_100
value: 13.245999999999999
- type: recall_at_1000
value: 46.373
- type: recall_at_3
value: 0.613
- type: recall_at_5
value: 0.991
- task:
type: BitextMining
dataset:
type: mteb/tatoeba-bitext-mining
name: MTEB Tatoeba (sqi-eng)
config: sqi-eng
split: test
revision: 9080400076fbadbb4c4dcb136ff4eddc40b42553
metrics:
- type: accuracy
value: 95.89999999999999
- type: f1
value: 94.69999999999999
- type: precision
value: 94.11666666666667
- type: recall
value: 95.89999999999999
- task:
type: BitextMining
dataset:
type: mteb/tatoeba-bitext-mining
name: MTEB Tatoeba (fry-eng)
config: fry-eng
split: test
revision: 9080400076fbadbb4c4dcb136ff4eddc40b42553
metrics:
- type: accuracy
value: 68.20809248554913
- type: f1
value: 63.431048720066066
- type: precision
value: 61.69143958161298
- type: recall
value: 68.20809248554913
- task:
type: BitextMining
dataset:
type: mteb/tatoeba-bitext-mining
name: MTEB Tatoeba (kur-eng)
config: kur-eng
split: test
revision: 9080400076fbadbb4c4dcb136ff4eddc40b42553
metrics:
- type: accuracy
value: 71.21951219512195
- type: f1
value: 66.82926829268293
- type: precision
value: 65.1260162601626
- type: recall
value: 71.21951219512195
- task:
type: BitextMining
dataset:
type: mteb/tatoeba-bitext-mining
name: MTEB Tatoeba (tur-eng)
config: tur-eng
split: test
revision: 9080400076fbadbb4c4dcb136ff4eddc40b42553
metrics:
- type: accuracy
value: 97.2
- type: f1
value: 96.26666666666667
- type: precision
value: 95.8
- type: recall
value: 97.2
- task:
type: BitextMining
dataset:
type: mteb/tatoeba-bitext-mining
name: MTEB Tatoeba (deu-eng)
config: deu-eng
split: test
revision: 9080400076fbadbb4c4dcb136ff4eddc40b42553
metrics:
- type: accuracy
value: 99.3
- type: f1
value: 99.06666666666666
- type: precision
value: 98.95
- type: recall
value: 99.3
- task:
type: BitextMining
dataset:
type: mteb/tatoeba-bitext-mining
name: MTEB Tatoeba (nld-eng)
config: nld-eng
split: test
revision: 9080400076fbadbb4c4dcb136ff4eddc40b42553
metrics:
- type: accuracy
value: 97.39999999999999
- type: f1
value: 96.63333333333333
- type: precision
value: 96.26666666666668
- type: recall
value: 97.39999999999999
- task:
type: BitextMining
dataset:
type: mteb/tatoeba-bitext-mining
name: MTEB Tatoeba (ron-eng)
config: ron-eng
split: test
revision: 9080400076fbadbb4c4dcb136ff4eddc40b42553
metrics:
- type: accuracy
value: 96
- type: f1
value: 94.86666666666666
- type: precision
value: 94.31666666666668
- type: recall
value: 96
- task:
type: BitextMining
dataset:
type: mteb/tatoeba-bitext-mining
name: MTEB Tatoeba (ang-eng)
config: ang-eng
split: test
revision: 9080400076fbadbb4c4dcb136ff4eddc40b42553
metrics:
- type: accuracy
value: 47.01492537313433
- type: f1
value: 40.178867566927266
- type: precision
value: 38.179295828549556
- type: recall
value: 47.01492537313433
- task:
type: BitextMining
dataset:
type: mteb/tatoeba-bitext-mining
name: MTEB Tatoeba (ido-eng)
config: ido-eng
split: test
revision: 9080400076fbadbb4c4dcb136ff4eddc40b42553
metrics:
- type: accuracy
value: 86.5
- type: f1
value: 83.62537480063796
- type: precision
value: 82.44555555555554
- type: recall
value: 86.5
- task:
type: BitextMining
dataset:
type: mteb/tatoeba-bitext-mining
name: MTEB Tatoeba (jav-eng)
config: jav-eng
split: test
revision: 9080400076fbadbb4c4dcb136ff4eddc40b42553
metrics:
- type: accuracy
value: 80.48780487804879
- type: f1
value: 75.45644599303138
- type: precision
value: 73.37398373983739
- type: recall
value: 80.48780487804879
- task:
type: BitextMining
dataset:
type: mteb/tatoeba-bitext-mining
name: MTEB Tatoeba (isl-eng)
config: isl-eng
split: test
revision: 9080400076fbadbb4c4dcb136ff4eddc40b42553
metrics:
- type: accuracy
value: 93.7
- type: f1
value: 91.95666666666666
- type: precision
value: 91.125
- type: recall
value: 93.7
- task:
type: BitextMining
dataset:
type: mteb/tatoeba-bitext-mining
name: MTEB Tatoeba (slv-eng)
config: slv-eng
split: test
revision: 9080400076fbadbb4c4dcb136ff4eddc40b42553
metrics:
- type: accuracy
value: 91.73754556500607
- type: f1
value: 89.65168084244632
- type: precision
value: 88.73025516403402
- type: recall
value: 91.73754556500607
- task:
type: BitextMining
dataset:
type: mteb/tatoeba-bitext-mining
name: MTEB Tatoeba (cym-eng)
config: cym-eng
split: test
revision: 9080400076fbadbb4c4dcb136ff4eddc40b42553
metrics:
- type: accuracy
value: 81.04347826086956
- type: f1
value: 76.2128364389234
- type: precision
value: 74.2
- type: recall
value: 81.04347826086956
- task:
type: BitextMining
dataset:
type: mteb/tatoeba-bitext-mining
name: MTEB Tatoeba (kaz-eng)
config: kaz-eng
split: test
revision: 9080400076fbadbb4c4dcb136ff4eddc40b42553
metrics:
- type: accuracy
value: 83.65217391304348
- type: f1
value: 79.4376811594203
- type: precision
value: 77.65797101449274
- type: recall
value: 83.65217391304348
- task:
type: BitextMining
dataset:
type: mteb/tatoeba-bitext-mining
name: MTEB Tatoeba (est-eng)
config: est-eng
split: test
revision: 9080400076fbadbb4c4dcb136ff4eddc40b42553
metrics:
- type: accuracy
value: 87.5
- type: f1
value: 85.02690476190476
- type: precision
value: 83.96261904761904
- type: recall
value: 87.5
- task:
type: BitextMining
dataset:
type: mteb/tatoeba-bitext-mining
name: MTEB Tatoeba (heb-eng)
config: heb-eng
split: test
revision: 9080400076fbadbb4c4dcb136ff4eddc40b42553
metrics:
- type: accuracy
value: 89.3
- type: f1
value: 86.52333333333333
- type: precision
value: 85.22833333333332
- type: recall
value: 89.3
- task:
type: BitextMining
dataset:
type: mteb/tatoeba-bitext-mining
name: MTEB Tatoeba (gla-eng)
config: gla-eng
split: test
revision: 9080400076fbadbb4c4dcb136ff4eddc40b42553
metrics:
- type: accuracy
value: 65.01809408926418
- type: f1
value: 59.00594446432805
- type: precision
value: 56.827215807915444
- type: recall
value: 65.01809408926418
- task:
type: BitextMining
dataset:
type: mteb/tatoeba-bitext-mining
name: MTEB Tatoeba (mar-eng)
config: mar-eng
split: test
revision: 9080400076fbadbb4c4dcb136ff4eddc40b42553
metrics:
- type: accuracy
value: 91.2
- type: f1
value: 88.58
- type: precision
value: 87.33333333333334
- type: recall
value: 91.2
- task:
type: BitextMining
dataset:
type: mteb/tatoeba-bitext-mining
name: MTEB Tatoeba (lat-eng)
config: lat-eng
split: test
revision: 9080400076fbadbb4c4dcb136ff4eddc40b42553
metrics:
- type: accuracy
value: 59.199999999999996
- type: f1
value: 53.299166276284915
- type: precision
value: 51.3383908045977
- type: recall
value: 59.199999999999996
- task:
type: BitextMining
dataset:
type: mteb/tatoeba-bitext-mining
name: MTEB Tatoeba (bel-eng)
config: bel-eng
split: test
revision: 9080400076fbadbb4c4dcb136ff4eddc40b42553
metrics:
- type: accuracy
value: 93.2
- type: f1
value: 91.2
- type: precision
value: 90.25
- type: recall
value: 93.2
- task:
type: BitextMining
dataset:
type: mteb/tatoeba-bitext-mining
name: MTEB Tatoeba (pms-eng)
config: pms-eng
split: test
revision: 9080400076fbadbb4c4dcb136ff4eddc40b42553
metrics:
- type: accuracy
value: 64.76190476190476
- type: f1
value: 59.867110667110666
- type: precision
value: 58.07390192653351
- type: recall
value: 64.76190476190476
- task:
type: BitextMining
dataset:
type: mteb/tatoeba-bitext-mining
name: MTEB Tatoeba (gle-eng)
config: gle-eng
split: test
revision: 9080400076fbadbb4c4dcb136ff4eddc40b42553
metrics:
- type: accuracy
value: 76.2
- type: f1
value: 71.48147546897547
- type: precision
value: 69.65409090909091
- type: recall
value: 76.2
- task:
type: BitextMining
dataset:
type: mteb/tatoeba-bitext-mining
name: MTEB Tatoeba (pes-eng)
config: pes-eng
split: test
revision: 9080400076fbadbb4c4dcb136ff4eddc40b42553
metrics:
- type: accuracy
value: 93.8
- type: f1
value: 92.14
- type: precision
value: 91.35833333333333
- type: recall
value: 93.8
- task:
type: BitextMining
dataset:
type: mteb/tatoeba-bitext-mining
name: MTEB Tatoeba (nob-eng)
config: nob-eng
split: test
revision: 9080400076fbadbb4c4dcb136ff4eddc40b42553
metrics:
- type: accuracy
value: 97.89999999999999
- type: f1
value: 97.2
- type: precision
value: 96.85000000000001
- type: recall
value: 97.89999999999999
- task:
type: BitextMining
dataset:
type: mteb/tatoeba-bitext-mining
name: MTEB Tatoeba (bul-eng)
config: bul-eng
split: test
revision: 9080400076fbadbb4c4dcb136ff4eddc40b42553
metrics:
- type: accuracy
value: 94.6
- type: f1
value: 92.93333333333334
- type: precision
value: 92.13333333333333
- type: recall
value: 94.6
- task:
type: BitextMining
dataset:
type: mteb/tatoeba-bitext-mining
name: MTEB Tatoeba (cbk-eng)
config: cbk-eng
split: test
revision: 9080400076fbadbb4c4dcb136ff4eddc40b42553
metrics:
- type: accuracy
value: 74.1
- type: f1
value: 69.14817460317461
- type: precision
value: 67.2515873015873
- type: recall
value: 74.1
- task:
type: BitextMining
dataset:
type: mteb/tatoeba-bitext-mining
name: MTEB Tatoeba (hun-eng)
config: hun-eng
split: test
revision: 9080400076fbadbb4c4dcb136ff4eddc40b42553
metrics:
- type: accuracy
value: 95.19999999999999
- type: f1
value: 94.01333333333335
- type: precision
value: 93.46666666666667
- type: recall
value: 95.19999999999999
- task:
type: BitextMining
dataset:
type: mteb/tatoeba-bitext-mining
name: MTEB Tatoeba (uig-eng)
config: uig-eng
split: test
revision: 9080400076fbadbb4c4dcb136ff4eddc40b42553
metrics:
- type: accuracy
value: 76.9
- type: f1
value: 72.07523809523809
- type: precision
value: 70.19777777777779
- type: recall
value: 76.9
- task:
type: BitextMining
dataset:
type: mteb/tatoeba-bitext-mining
name: MTEB Tatoeba (rus-eng)
config: rus-eng
split: test
revision: 9080400076fbadbb4c4dcb136ff4eddc40b42553
metrics:
- type: accuracy
value: 94.1
- type: f1
value: 92.31666666666666
- type: precision
value: 91.43333333333332
- type: recall
value: 94.1
- task:
type: BitextMining
dataset:
type: mteb/tatoeba-bitext-mining
name: MTEB Tatoeba (spa-eng)
config: spa-eng
split: test
revision: 9080400076fbadbb4c4dcb136ff4eddc40b42553
metrics:
- type: accuracy
value: 97.8
- type: f1
value: 97.1
- type: precision
value: 96.76666666666668
- type: recall
value: 97.8
- task:
type: BitextMining
dataset:
type: mteb/tatoeba-bitext-mining
name: MTEB Tatoeba (hye-eng)
config: hye-eng
split: test
revision: 9080400076fbadbb4c4dcb136ff4eddc40b42553
metrics:
- type: accuracy
value: 92.85714285714286
- type: f1
value: 90.92093441150045
- type: precision
value: 90.00449236298293
- type: recall
value: 92.85714285714286
- task:
type: BitextMining
dataset:
type: mteb/tatoeba-bitext-mining
name: MTEB Tatoeba (tel-eng)
config: tel-eng
split: test
revision: 9080400076fbadbb4c4dcb136ff4eddc40b42553
metrics:
- type: accuracy
value: 93.16239316239316
- type: f1
value: 91.33903133903132
- type: precision
value: 90.56267806267806
- type: recall
value: 93.16239316239316
- task:
type: BitextMining
dataset:
type: mteb/tatoeba-bitext-mining
name: MTEB Tatoeba (afr-eng)
config: afr-eng
split: test
revision: 9080400076fbadbb4c4dcb136ff4eddc40b42553
metrics:
- type: accuracy
value: 92.4
- type: f1
value: 90.25666666666666
- type: precision
value: 89.25833333333334
- type: recall
value: 92.4
- task:
type: BitextMining
dataset:
type: mteb/tatoeba-bitext-mining
name: MTEB Tatoeba (mon-eng)
config: mon-eng
split: test
revision: 9080400076fbadbb4c4dcb136ff4eddc40b42553
metrics:
- type: accuracy
value: 90.22727272727272
- type: f1
value: 87.53030303030303
- type: precision
value: 86.37121212121211
- type: recall
value: 90.22727272727272
- task:
type: BitextMining
dataset:
type: mteb/tatoeba-bitext-mining
name: MTEB Tatoeba (arz-eng)
config: arz-eng
split: test
revision: 9080400076fbadbb4c4dcb136ff4eddc40b42553
metrics:
- type: accuracy
value: 79.03563941299791
- type: f1
value: 74.7349505840072
- type: precision
value: 72.9035639412998
- type: recall
value: 79.03563941299791
- task:
type: BitextMining
dataset:
type: mteb/tatoeba-bitext-mining
name: MTEB Tatoeba (hrv-eng)
config: hrv-eng
split: test
revision: 9080400076fbadbb4c4dcb136ff4eddc40b42553
metrics:
- type: accuracy
value: 97
- type: f1
value: 96.15
- type: precision
value: 95.76666666666668
- type: recall
value: 97
- task:
type: BitextMining
dataset:
type: mteb/tatoeba-bitext-mining
name: MTEB Tatoeba (nov-eng)
config: nov-eng
split: test
revision: 9080400076fbadbb4c4dcb136ff4eddc40b42553
metrics:
- type: accuracy
value: 76.26459143968872
- type: f1
value: 71.55642023346303
- type: precision
value: 69.7544932369835
- type: recall
value: 76.26459143968872
- task:
type: BitextMining
dataset:
type: mteb/tatoeba-bitext-mining
name: MTEB Tatoeba (gsw-eng)
config: gsw-eng
split: test
revision: 9080400076fbadbb4c4dcb136ff4eddc40b42553
metrics:
- type: accuracy
value: 58.119658119658126
- type: f1
value: 51.65242165242165
- type: precision
value: 49.41768108434775
- type: recall
value: 58.119658119658126
- task:
type: BitextMining
dataset:
type: mteb/tatoeba-bitext-mining
name: MTEB Tatoeba (nds-eng)
config: nds-eng
split: test
revision: 9080400076fbadbb4c4dcb136ff4eddc40b42553
metrics:
- type: accuracy
value: 74.3
- type: f1
value: 69.52055555555555
- type: precision
value: 67.7574938949939
- type: recall
value: 74.3
- task:
type: BitextMining
dataset:
type: mteb/tatoeba-bitext-mining
name: MTEB Tatoeba (ukr-eng)
config: ukr-eng
split: test
revision: 9080400076fbadbb4c4dcb136ff4eddc40b42553
metrics:
- type: accuracy
value: 94.8
- type: f1
value: 93.31666666666666
- type: precision
value: 92.60000000000001
- type: recall
value: 94.8
- task:
type: BitextMining
dataset:
type: mteb/tatoeba-bitext-mining
name: MTEB Tatoeba (uzb-eng)
config: uzb-eng
split: test
revision: 9080400076fbadbb4c4dcb136ff4eddc40b42553
metrics:
- type: accuracy
value: 76.63551401869158
- type: f1
value: 72.35202492211837
- type: precision
value: 70.60358255451713
- type: recall
value: 76.63551401869158
- task:
type: BitextMining
dataset:
type: mteb/tatoeba-bitext-mining
name: MTEB Tatoeba (lit-eng)
config: lit-eng
split: test
revision: 9080400076fbadbb4c4dcb136ff4eddc40b42553
metrics:
- type: accuracy
value: 90.4
- type: f1
value: 88.4811111111111
- type: precision
value: 87.7452380952381
- type: recall
value: 90.4
- task:
type: BitextMining
dataset:
type: mteb/tatoeba-bitext-mining
name: MTEB Tatoeba (ina-eng)
config: ina-eng
split: test
revision: 9080400076fbadbb4c4dcb136ff4eddc40b42553
metrics:
- type: accuracy
value: 95
- type: f1
value: 93.60666666666667
- type: precision
value: 92.975
- type: recall
value: 95
- task:
type: BitextMining
dataset:
type: mteb/tatoeba-bitext-mining
name: MTEB Tatoeba (lfn-eng)
config: lfn-eng
split: test
revision: 9080400076fbadbb4c4dcb136ff4eddc40b42553
metrics:
- type: accuracy
value: 67.2
- type: f1
value: 63.01595782872099
- type: precision
value: 61.596587301587306
- type: recall
value: 67.2
- task:
type: BitextMining
dataset:
type: mteb/tatoeba-bitext-mining
name: MTEB Tatoeba (zsm-eng)
config: zsm-eng
split: test
revision: 9080400076fbadbb4c4dcb136ff4eddc40b42553
metrics:
- type: accuracy
value: 95.7
- type: f1
value: 94.52999999999999
- type: precision
value: 94
- type: recall
value: 95.7
- task:
type: BitextMining
dataset:
type: mteb/tatoeba-bitext-mining
name: MTEB Tatoeba (ita-eng)
config: ita-eng
split: test
revision: 9080400076fbadbb4c4dcb136ff4eddc40b42553
metrics:
- type: accuracy
value: 94.6
- type: f1
value: 93.28999999999999
- type: precision
value: 92.675
- type: recall
value: 94.6
- task:
type: BitextMining
dataset:
type: mteb/tatoeba-bitext-mining
name: MTEB Tatoeba (cmn-eng)
config: cmn-eng
split: test
revision: 9080400076fbadbb4c4dcb136ff4eddc40b42553
metrics:
- type: accuracy
value: 96.39999999999999
- type: f1
value: 95.28333333333333
- type: precision
value: 94.75
- type: recall
value: 96.39999999999999
- task:
type: BitextMining
dataset:
type: mteb/tatoeba-bitext-mining
name: MTEB Tatoeba (lvs-eng)
config: lvs-eng
split: test
revision: 9080400076fbadbb4c4dcb136ff4eddc40b42553
metrics:
- type: accuracy
value: 91.9
- type: f1
value: 89.83
- type: precision
value: 88.92
- type: recall
value: 91.9
- task:
type: BitextMining
dataset:
type: mteb/tatoeba-bitext-mining
name: MTEB Tatoeba (glg-eng)
config: glg-eng
split: test
revision: 9080400076fbadbb4c4dcb136ff4eddc40b42553
metrics:
- type: accuracy
value: 94.69999999999999
- type: f1
value: 93.34222222222223
- type: precision
value: 92.75416666666668
- type: recall
value: 94.69999999999999
- task:
type: BitextMining
dataset:
type: mteb/tatoeba-bitext-mining
name: MTEB Tatoeba (ceb-eng)
config: ceb-eng
split: test
revision: 9080400076fbadbb4c4dcb136ff4eddc40b42553
metrics:
- type: accuracy
value: 60.333333333333336
- type: f1
value: 55.31203703703703
- type: precision
value: 53.39971108326371
- type: recall
value: 60.333333333333336
- task:
type: BitextMining
dataset:
type: mteb/tatoeba-bitext-mining
name: MTEB Tatoeba (bre-eng)
config: bre-eng
split: test
revision: 9080400076fbadbb4c4dcb136ff4eddc40b42553
metrics:
- type: accuracy
value: 12.9
- type: f1
value: 11.099861903031458
- type: precision
value: 10.589187932631877
- type: recall
value: 12.9
- task:
type: BitextMining
dataset:
type: mteb/tatoeba-bitext-mining
name: MTEB Tatoeba (ben-eng)
config: ben-eng
split: test
revision: 9080400076fbadbb4c4dcb136ff4eddc40b42553
metrics:
- type: accuracy
value: 86.7
- type: f1
value: 83.0152380952381
- type: precision
value: 81.37833333333333
- type: recall
value: 86.7
- task:
type: BitextMining
dataset:
type: mteb/tatoeba-bitext-mining
name: MTEB Tatoeba (swg-eng)
config: swg-eng
split: test
revision: 9080400076fbadbb4c4dcb136ff4eddc40b42553
metrics:
- type: accuracy
value: 63.39285714285714
- type: f1
value: 56.832482993197274
- type: precision
value: 54.56845238095237
- type: recall
value: 63.39285714285714
- task:
type: BitextMining
dataset:
type: mteb/tatoeba-bitext-mining
name: MTEB Tatoeba (arq-eng)
config: arq-eng
split: test
revision: 9080400076fbadbb4c4dcb136ff4eddc40b42553
metrics:
- type: accuracy
value: 48.73765093304062
- type: f1
value: 41.555736920720456
- type: precision
value: 39.06874531737319
- type: recall
value: 48.73765093304062
- task:
type: BitextMining
dataset:
type: mteb/tatoeba-bitext-mining
name: MTEB Tatoeba (kab-eng)
config: kab-eng
split: test
revision: 9080400076fbadbb4c4dcb136ff4eddc40b42553
metrics:
- type: accuracy
value: 41.099999999999994
- type: f1
value: 36.540165945165946
- type: precision
value: 35.05175685425686
- type: recall
value: 41.099999999999994
- task:
type: BitextMining
dataset:
type: mteb/tatoeba-bitext-mining
name: MTEB Tatoeba (fra-eng)
config: fra-eng
split: test
revision: 9080400076fbadbb4c4dcb136ff4eddc40b42553
metrics:
- type: accuracy
value: 94.89999999999999
- type: f1
value: 93.42333333333333
- type: precision
value: 92.75833333333333
- type: recall
value: 94.89999999999999
- task:
type: BitextMining
dataset:
type: mteb/tatoeba-bitext-mining
name: MTEB Tatoeba (por-eng)
config: por-eng
split: test
revision: 9080400076fbadbb4c4dcb136ff4eddc40b42553
metrics:
- type: accuracy
value: 94.89999999999999
- type: f1
value: 93.63333333333334
- type: precision
value: 93.01666666666665
- type: recall
value: 94.89999999999999
- task:
type: BitextMining
dataset:
type: mteb/tatoeba-bitext-mining
name: MTEB Tatoeba (tat-eng)
config: tat-eng
split: test
revision: 9080400076fbadbb4c4dcb136ff4eddc40b42553
metrics:
- type: accuracy
value: 77.9
- type: f1
value: 73.64833333333334
- type: precision
value: 71.90282106782105
- type: recall
value: 77.9
- task:
type: BitextMining
dataset:
type: mteb/tatoeba-bitext-mining
name: MTEB Tatoeba (oci-eng)
config: oci-eng
split: test
revision: 9080400076fbadbb4c4dcb136ff4eddc40b42553
metrics:
- type: accuracy
value: 59.4
- type: f1
value: 54.90521367521367
- type: precision
value: 53.432840025471606
- type: recall
value: 59.4
- task:
type: BitextMining
dataset:
type: mteb/tatoeba-bitext-mining
name: MTEB Tatoeba (pol-eng)
config: pol-eng
split: test
revision: 9080400076fbadbb4c4dcb136ff4eddc40b42553
metrics:
- type: accuracy
value: 97.39999999999999
- type: f1
value: 96.6
- type: precision
value: 96.2
- type: recall
value: 97.39999999999999
- task:
type: BitextMining
dataset:
type: mteb/tatoeba-bitext-mining
name: MTEB Tatoeba (war-eng)
config: war-eng
split: test
revision: 9080400076fbadbb4c4dcb136ff4eddc40b42553
metrics:
- type: accuracy
value: 67.2
- type: f1
value: 62.25926129426129
- type: precision
value: 60.408376623376626
- type: recall
value: 67.2
- task:
type: BitextMining
dataset:
type: mteb/tatoeba-bitext-mining
name: MTEB Tatoeba (aze-eng)
config: aze-eng
split: test
revision: 9080400076fbadbb4c4dcb136ff4eddc40b42553
metrics:
- type: accuracy
value: 90.2
- type: f1
value: 87.60666666666667
- type: precision
value: 86.45277777777778
- type: recall
value: 90.2
- task:
type: BitextMining
dataset:
type: mteb/tatoeba-bitext-mining
name: MTEB Tatoeba (vie-eng)
config: vie-eng
split: test
revision: 9080400076fbadbb4c4dcb136ff4eddc40b42553
metrics:
- type: accuracy
value: 97.7
- type: f1
value: 97
- type: precision
value: 96.65
- type: recall
value: 97.7
- task:
type: BitextMining
dataset:
type: mteb/tatoeba-bitext-mining
name: MTEB Tatoeba (nno-eng)
config: nno-eng
split: test
revision: 9080400076fbadbb4c4dcb136ff4eddc40b42553
metrics:
- type: accuracy
value: 93.2
- type: f1
value: 91.39746031746031
- type: precision
value: 90.6125
- type: recall
value: 93.2
- task:
type: BitextMining
dataset:
type: mteb/tatoeba-bitext-mining
name: MTEB Tatoeba (cha-eng)
config: cha-eng
split: test
revision: 9080400076fbadbb4c4dcb136ff4eddc40b42553
metrics:
- type: accuracy
value: 32.11678832116788
- type: f1
value: 27.210415386260234
- type: precision
value: 26.20408990846947
- type: recall
value: 32.11678832116788
- task:
type: BitextMining
dataset:
type: mteb/tatoeba-bitext-mining
name: MTEB Tatoeba (mhr-eng)
config: mhr-eng
split: test
revision: 9080400076fbadbb4c4dcb136ff4eddc40b42553
metrics:
- type: accuracy
value: 8.5
- type: f1
value: 6.787319277832475
- type: precision
value: 6.3452094433344435
- type: recall
value: 8.5
- task:
type: BitextMining
dataset:
type: mteb/tatoeba-bitext-mining
name: MTEB Tatoeba (dan-eng)
config: dan-eng
split: test
revision: 9080400076fbadbb4c4dcb136ff4eddc40b42553
metrics:
- type: accuracy
value: 96.1
- type: f1
value: 95.08
- type: precision
value: 94.61666666666667
- type: recall
value: 96.1
- task:
type: BitextMining
dataset:
type: mteb/tatoeba-bitext-mining
name: MTEB Tatoeba (ell-eng)
config: ell-eng
split: test
revision: 9080400076fbadbb4c4dcb136ff4eddc40b42553
metrics:
- type: accuracy
value: 95.3
- type: f1
value: 93.88333333333333
- type: precision
value: 93.18333333333332
- type: recall
value: 95.3
- task:
type: BitextMining
dataset:
type: mteb/tatoeba-bitext-mining
name: MTEB Tatoeba (amh-eng)
config: amh-eng
split: test
revision: 9080400076fbadbb4c4dcb136ff4eddc40b42553
metrics:
- type: accuracy
value: 85.11904761904762
- type: f1
value: 80.69444444444444
- type: precision
value: 78.72023809523809
- type: recall
value: 85.11904761904762
- task:
type: BitextMining
dataset:
type: mteb/tatoeba-bitext-mining
name: MTEB Tatoeba (pam-eng)
config: pam-eng
split: test
revision: 9080400076fbadbb4c4dcb136ff4eddc40b42553
metrics:
- type: accuracy
value: 11.1
- type: f1
value: 9.276381801735853
- type: precision
value: 8.798174603174601
- type: recall
value: 11.1
- task:
type: BitextMining
dataset:
type: mteb/tatoeba-bitext-mining
name: MTEB Tatoeba (hsb-eng)
config: hsb-eng
split: test
revision: 9080400076fbadbb4c4dcb136ff4eddc40b42553
metrics:
- type: accuracy
value: 63.56107660455487
- type: f1
value: 58.70433569191332
- type: precision
value: 56.896926581464015
- type: recall
value: 63.56107660455487
- task:
type: BitextMining
dataset:
type: mteb/tatoeba-bitext-mining
name: MTEB Tatoeba (srp-eng)
config: srp-eng
split: test
revision: 9080400076fbadbb4c4dcb136ff4eddc40b42553
metrics:
- type: accuracy
value: 94.69999999999999
- type: f1
value: 93.10000000000001
- type: precision
value: 92.35
- type: recall
value: 94.69999999999999
- task:
type: BitextMining
dataset:
type: mteb/tatoeba-bitext-mining
name: MTEB Tatoeba (epo-eng)
config: epo-eng
split: test
revision: 9080400076fbadbb4c4dcb136ff4eddc40b42553
metrics:
- type: accuracy
value: 96.8
- type: f1
value: 96.01222222222222
- type: precision
value: 95.67083333333332
- type: recall
value: 96.8
- task:
type: BitextMining
dataset:
type: mteb/tatoeba-bitext-mining
name: MTEB Tatoeba (kzj-eng)
config: kzj-eng
split: test
revision: 9080400076fbadbb4c4dcb136ff4eddc40b42553
metrics:
- type: accuracy
value: 9.2
- type: f1
value: 7.911555250305249
- type: precision
value: 7.631246556216846
- type: recall
value: 9.2
- task:
type: BitextMining
dataset:
type: mteb/tatoeba-bitext-mining
name: MTEB Tatoeba (awa-eng)
config: awa-eng
split: test
revision: 9080400076fbadbb4c4dcb136ff4eddc40b42553
metrics:
- type: accuracy
value: 77.48917748917748
- type: f1
value: 72.27375798804371
- type: precision
value: 70.14430014430013
- type: recall
value: 77.48917748917748
- task:
type: BitextMining
dataset:
type: mteb/tatoeba-bitext-mining
name: MTEB Tatoeba (fao-eng)
config: fao-eng
split: test
revision: 9080400076fbadbb4c4dcb136ff4eddc40b42553
metrics:
- type: accuracy
value: 77.09923664122137
- type: f1
value: 72.61541257724463
- type: precision
value: 70.8998380754106
- type: recall
value: 77.09923664122137
- task:
type: BitextMining
dataset:
type: mteb/tatoeba-bitext-mining
name: MTEB Tatoeba (mal-eng)
config: mal-eng
split: test
revision: 9080400076fbadbb4c4dcb136ff4eddc40b42553
metrics:
- type: accuracy
value: 98.2532751091703
- type: f1
value: 97.69529354682193
- type: precision
value: 97.42843279961184
- type: recall
value: 98.2532751091703
- task:
type: BitextMining
dataset:
type: mteb/tatoeba-bitext-mining
name: MTEB Tatoeba (ile-eng)
config: ile-eng
split: test
revision: 9080400076fbadbb4c4dcb136ff4eddc40b42553
metrics:
- type: accuracy
value: 82.8
- type: f1
value: 79.14672619047619
- type: precision
value: 77.59489247311828
- type: recall
value: 82.8
- task:
type: BitextMining
dataset:
type: mteb/tatoeba-bitext-mining
name: MTEB Tatoeba (bos-eng)
config: bos-eng
split: test
revision: 9080400076fbadbb4c4dcb136ff4eddc40b42553
metrics:
- type: accuracy
value: 94.35028248587571
- type: f1
value: 92.86252354048965
- type: precision
value: 92.2080979284369
- type: recall
value: 94.35028248587571
- task:
type: BitextMining
dataset:
type: mteb/tatoeba-bitext-mining
name: MTEB Tatoeba (cor-eng)
config: cor-eng
split: test
revision: 9080400076fbadbb4c4dcb136ff4eddc40b42553
metrics:
- type: accuracy
value: 8.5
- type: f1
value: 6.282429263935621
- type: precision
value: 5.783274240739785
- type: recall
value: 8.5
- task:
type: BitextMining
dataset:
type: mteb/tatoeba-bitext-mining
name: MTEB Tatoeba (cat-eng)
config: cat-eng
split: test
revision: 9080400076fbadbb4c4dcb136ff4eddc40b42553
metrics:
- type: accuracy
value: 92.7
- type: f1
value: 91.025
- type: precision
value: 90.30428571428571
- type: recall
value: 92.7
- task:
type: BitextMining
dataset:
type: mteb/tatoeba-bitext-mining
name: MTEB Tatoeba (eus-eng)
config: eus-eng
split: test
revision: 9080400076fbadbb4c4dcb136ff4eddc40b42553
metrics:
- type: accuracy
value: 81
- type: f1
value: 77.8232380952381
- type: precision
value: 76.60194444444444
- type: recall
value: 81
- task:
type: BitextMining
dataset:
type: mteb/tatoeba-bitext-mining
name: MTEB Tatoeba (yue-eng)
config: yue-eng
split: test
revision: 9080400076fbadbb4c4dcb136ff4eddc40b42553
metrics:
- type: accuracy
value: 91
- type: f1
value: 88.70857142857142
- type: precision
value: 87.7
- type: recall
value: 91
- task:
type: BitextMining
dataset:
type: mteb/tatoeba-bitext-mining
name: MTEB Tatoeba (swe-eng)
config: swe-eng
split: test
revision: 9080400076fbadbb4c4dcb136ff4eddc40b42553
metrics:
- type: accuracy
value: 96.39999999999999
- type: f1
value: 95.3
- type: precision
value: 94.76666666666667
- type: recall
value: 96.39999999999999
- task:
type: BitextMining
dataset:
type: mteb/tatoeba-bitext-mining
name: MTEB Tatoeba (dtp-eng)
config: dtp-eng
split: test
revision: 9080400076fbadbb4c4dcb136ff4eddc40b42553
metrics:
- type: accuracy
value: 8.1
- type: f1
value: 7.001008218834307
- type: precision
value: 6.708329562594269
- type: recall
value: 8.1
- task:
type: BitextMining
dataset:
type: mteb/tatoeba-bitext-mining
name: MTEB Tatoeba (kat-eng)
config: kat-eng
split: test
revision: 9080400076fbadbb4c4dcb136ff4eddc40b42553
metrics:
- type: accuracy
value: 87.1313672922252
- type: f1
value: 84.09070598748882
- type: precision
value: 82.79171454104429
- type: recall
value: 87.1313672922252
- task:
type: BitextMining
dataset:
type: mteb/tatoeba-bitext-mining
name: MTEB Tatoeba (jpn-eng)
config: jpn-eng
split: test
revision: 9080400076fbadbb4c4dcb136ff4eddc40b42553
metrics:
- type: accuracy
value: 96.39999999999999
- type: f1
value: 95.28333333333333
- type: precision
value: 94.73333333333332
- type: recall
value: 96.39999999999999
- task:
type: BitextMining
dataset:
type: mteb/tatoeba-bitext-mining
name: MTEB Tatoeba (csb-eng)
config: csb-eng
split: test
revision: 9080400076fbadbb4c4dcb136ff4eddc40b42553
metrics:
- type: accuracy
value: 42.29249011857708
- type: f1
value: 36.981018542283365
- type: precision
value: 35.415877813576024
- type: recall
value: 42.29249011857708
- task:
type: BitextMining
dataset:
type: mteb/tatoeba-bitext-mining
name: MTEB Tatoeba (xho-eng)
config: xho-eng
split: test
revision: 9080400076fbadbb4c4dcb136ff4eddc40b42553
metrics:
- type: accuracy
value: 83.80281690140845
- type: f1
value: 80.86854460093896
- type: precision
value: 79.60093896713614
- type: recall
value: 83.80281690140845
- task:
type: BitextMining
dataset:
type: mteb/tatoeba-bitext-mining
name: MTEB Tatoeba (orv-eng)
config: orv-eng
split: test
revision: 9080400076fbadbb4c4dcb136ff4eddc40b42553
metrics:
- type: accuracy
value: 45.26946107784431
- type: f1
value: 39.80235464678088
- type: precision
value: 38.14342660001342
- type: recall
value: 45.26946107784431
- task:
type: BitextMining
dataset:
type: mteb/tatoeba-bitext-mining
name: MTEB Tatoeba (ind-eng)
config: ind-eng
split: test
revision: 9080400076fbadbb4c4dcb136ff4eddc40b42553
metrics:
- type: accuracy
value: 94.3
- type: f1
value: 92.9
- type: precision
value: 92.26666666666668
- type: recall
value: 94.3
- task:
type: BitextMining
dataset:
type: mteb/tatoeba-bitext-mining
name: MTEB Tatoeba (tuk-eng)
config: tuk-eng
split: test
revision: 9080400076fbadbb4c4dcb136ff4eddc40b42553
metrics:
- type: accuracy
value: 37.93103448275862
- type: f1
value: 33.15192743764172
- type: precision
value: 31.57456528146183
- type: recall
value: 37.93103448275862
- task:
type: BitextMining
dataset:
type: mteb/tatoeba-bitext-mining
name: MTEB Tatoeba (max-eng)
config: max-eng
split: test
revision: 9080400076fbadbb4c4dcb136ff4eddc40b42553
metrics:
- type: accuracy
value: 69.01408450704226
- type: f1
value: 63.41549295774648
- type: precision
value: 61.342778895595806
- type: recall
value: 69.01408450704226
- task:
type: BitextMining
dataset:
type: mteb/tatoeba-bitext-mining
name: MTEB Tatoeba (swh-eng)
config: swh-eng
split: test
revision: 9080400076fbadbb4c4dcb136ff4eddc40b42553
metrics:
- type: accuracy
value: 76.66666666666667
- type: f1
value: 71.60705960705961
- type: precision
value: 69.60683760683762
- type: recall
value: 76.66666666666667
- task:
type: BitextMining
dataset:
type: mteb/tatoeba-bitext-mining
name: MTEB Tatoeba (hin-eng)
config: hin-eng
split: test
revision: 9080400076fbadbb4c4dcb136ff4eddc40b42553
metrics:
- type: accuracy
value: 95.8
- type: f1
value: 94.48333333333333
- type: precision
value: 93.83333333333333
- type: recall
value: 95.8
- task:
type: BitextMining
dataset:
type: mteb/tatoeba-bitext-mining
name: MTEB Tatoeba (dsb-eng)
config: dsb-eng
split: test
revision: 9080400076fbadbb4c4dcb136ff4eddc40b42553
metrics:
- type: accuracy
value: 52.81837160751566
- type: f1
value: 48.435977731384824
- type: precision
value: 47.11291973845539
- type: recall
value: 52.81837160751566
- task:
type: BitextMining
dataset:
type: mteb/tatoeba-bitext-mining
name: MTEB Tatoeba (ber-eng)
config: ber-eng
split: test
revision: 9080400076fbadbb4c4dcb136ff4eddc40b42553
metrics:
- type: accuracy
value: 44.9
- type: f1
value: 38.88962621607783
- type: precision
value: 36.95936507936508
- type: recall
value: 44.9
- task:
type: BitextMining
dataset:
type: mteb/tatoeba-bitext-mining
name: MTEB Tatoeba (tam-eng)
config: tam-eng
split: test
revision: 9080400076fbadbb4c4dcb136ff4eddc40b42553
metrics:
- type: accuracy
value: 90.55374592833876
- type: f1
value: 88.22553125484721
- type: precision
value: 87.26927252985884
- type: recall
value: 90.55374592833876
- task:
type: BitextMining
dataset:
type: mteb/tatoeba-bitext-mining
name: MTEB Tatoeba (slk-eng)
config: slk-eng
split: test
revision: 9080400076fbadbb4c4dcb136ff4eddc40b42553
metrics:
- type: accuracy
value: 94.6
- type: f1
value: 93.13333333333333
- type: precision
value: 92.45333333333333
- type: recall
value: 94.6
- task:
type: BitextMining
dataset:
type: mteb/tatoeba-bitext-mining
name: MTEB Tatoeba (tgl-eng)
config: tgl-eng
split: test
revision: 9080400076fbadbb4c4dcb136ff4eddc40b42553
metrics:
- type: accuracy
value: 93.7
- type: f1
value: 91.99666666666667
- type: precision
value: 91.26666666666668
- type: recall
value: 93.7
- task:
type: BitextMining
dataset:
type: mteb/tatoeba-bitext-mining
name: MTEB Tatoeba (ast-eng)
config: ast-eng
split: test
revision: 9080400076fbadbb4c4dcb136ff4eddc40b42553
metrics:
- type: accuracy
value: 85.03937007874016
- type: f1
value: 81.75853018372703
- type: precision
value: 80.34120734908137
- type: recall
value: 85.03937007874016
- task:
type: BitextMining
dataset:
type: mteb/tatoeba-bitext-mining
name: MTEB Tatoeba (mkd-eng)
config: mkd-eng
split: test
revision: 9080400076fbadbb4c4dcb136ff4eddc40b42553
metrics:
- type: accuracy
value: 88.3
- type: f1
value: 85.5
- type: precision
value: 84.25833333333334
- type: recall
value: 88.3
- task:
type: BitextMining
dataset:
type: mteb/tatoeba-bitext-mining
name: MTEB Tatoeba (khm-eng)
config: khm-eng
split: test
revision: 9080400076fbadbb4c4dcb136ff4eddc40b42553
metrics:
- type: accuracy
value: 65.51246537396122
- type: f1
value: 60.02297410192148
- type: precision
value: 58.133467727289236
- type: recall
value: 65.51246537396122
- task:
type: BitextMining
dataset:
type: mteb/tatoeba-bitext-mining
name: MTEB Tatoeba (ces-eng)
config: ces-eng
split: test
revision: 9080400076fbadbb4c4dcb136ff4eddc40b42553
metrics:
- type: accuracy
value: 96
- type: f1
value: 94.89
- type: precision
value: 94.39166666666667
- type: recall
value: 96
- task:
type: BitextMining
dataset:
type: mteb/tatoeba-bitext-mining
name: MTEB Tatoeba (tzl-eng)
config: tzl-eng
split: test
revision: 9080400076fbadbb4c4dcb136ff4eddc40b42553
metrics:
- type: accuracy
value: 57.692307692307686
- type: f1
value: 53.162393162393165
- type: precision
value: 51.70673076923077
- type: recall
value: 57.692307692307686
- task:
type: BitextMining
dataset:
type: mteb/tatoeba-bitext-mining
name: MTEB Tatoeba (urd-eng)
config: urd-eng
split: test
revision: 9080400076fbadbb4c4dcb136ff4eddc40b42553
metrics:
- type: accuracy
value: 91.60000000000001
- type: f1
value: 89.21190476190475
- type: precision
value: 88.08666666666667
- type: recall
value: 91.60000000000001
- task:
type: BitextMining
dataset:
type: mteb/tatoeba-bitext-mining
name: MTEB Tatoeba (ara-eng)
config: ara-eng
split: test
revision: 9080400076fbadbb4c4dcb136ff4eddc40b42553
metrics:
- type: accuracy
value: 88
- type: f1
value: 85.47
- type: precision
value: 84.43266233766234
- type: recall
value: 88
- task:
type: BitextMining
dataset:
type: mteb/tatoeba-bitext-mining
name: MTEB Tatoeba (kor-eng)
config: kor-eng
split: test
revision: 9080400076fbadbb4c4dcb136ff4eddc40b42553
metrics:
- type: accuracy
value: 92.7
- type: f1
value: 90.64999999999999
- type: precision
value: 89.68333333333332
- type: recall
value: 92.7
- task:
type: BitextMining
dataset:
type: mteb/tatoeba-bitext-mining
name: MTEB Tatoeba (yid-eng)
config: yid-eng
split: test
revision: 9080400076fbadbb4c4dcb136ff4eddc40b42553
metrics:
- type: accuracy
value: 80.30660377358491
- type: f1
value: 76.33044137466307
- type: precision
value: 74.78970125786164
- type: recall
value: 80.30660377358491
- task:
type: BitextMining
dataset:
type: mteb/tatoeba-bitext-mining
name: MTEB Tatoeba (fin-eng)
config: fin-eng
split: test
revision: 9080400076fbadbb4c4dcb136ff4eddc40b42553
metrics:
- type: accuracy
value: 96.39999999999999
- type: f1
value: 95.44
- type: precision
value: 94.99166666666666
- type: recall
value: 96.39999999999999
- task:
type: BitextMining
dataset:
type: mteb/tatoeba-bitext-mining
name: MTEB Tatoeba (tha-eng)
config: tha-eng
split: test
revision: 9080400076fbadbb4c4dcb136ff4eddc40b42553
metrics:
- type: accuracy
value: 96.53284671532847
- type: f1
value: 95.37712895377129
- type: precision
value: 94.7992700729927
- type: recall
value: 96.53284671532847
- task:
type: BitextMining
dataset:
type: mteb/tatoeba-bitext-mining
name: MTEB Tatoeba (wuu-eng)
config: wuu-eng
split: test
revision: 9080400076fbadbb4c4dcb136ff4eddc40b42553
metrics:
- type: accuracy
value: 89
- type: f1
value: 86.23190476190476
- type: precision
value: 85.035
- type: recall
value: 89
- task:
type: Retrieval
dataset:
type: webis-touche2020
name: MTEB Touche2020
config: default
split: test
revision: None
metrics:
- type: map_at_1
value: 2.585
- type: map_at_10
value: 9.012
- type: map_at_100
value: 14.027000000000001
- type: map_at_1000
value: 15.565000000000001
- type: map_at_3
value: 5.032
- type: map_at_5
value: 6.657
- type: mrr_at_1
value: 28.571
- type: mrr_at_10
value: 45.377
- type: mrr_at_100
value: 46.119
- type: mrr_at_1000
value: 46.127
- type: mrr_at_3
value: 41.156
- type: mrr_at_5
value: 42.585
- type: ndcg_at_1
value: 27.551
- type: ndcg_at_10
value: 23.395
- type: ndcg_at_100
value: 33.342
- type: ndcg_at_1000
value: 45.523
- type: ndcg_at_3
value: 25.158
- type: ndcg_at_5
value: 23.427
- type: precision_at_1
value: 28.571
- type: precision_at_10
value: 21.429000000000002
- type: precision_at_100
value: 6.714
- type: precision_at_1000
value: 1.473
- type: precision_at_3
value: 27.211000000000002
- type: precision_at_5
value: 24.490000000000002
- type: recall_at_1
value: 2.585
- type: recall_at_10
value: 15.418999999999999
- type: recall_at_100
value: 42.485
- type: recall_at_1000
value: 79.536
- type: recall_at_3
value: 6.239999999999999
- type: recall_at_5
value: 8.996
- task:
type: Classification
dataset:
type: mteb/toxic_conversations_50k
name: MTEB ToxicConversationsClassification
config: default
split: test
revision: d7c0de2777da35d6aae2200a62c6e0e5af397c4c
metrics:
- type: accuracy
value: 71.3234
- type: ap
value: 14.361688653847423
- type: f1
value: 54.819068624319044
- task:
type: Classification
dataset:
type: mteb/tweet_sentiment_extraction
name: MTEB TweetSentimentExtractionClassification
config: default
split: test
revision: d604517c81ca91fe16a244d1248fc021f9ecee7a
metrics:
- type: accuracy
value: 61.97792869269949
- type: f1
value: 62.28965628513728
- task:
type: Clustering
dataset:
type: mteb/twentynewsgroups-clustering
name: MTEB TwentyNewsgroupsClustering
config: default
split: test
revision: 6125ec4e24fa026cec8a478383ee943acfbd5449
metrics:
- type: v_measure
value: 38.90540145385218
- task:
type: PairClassification
dataset:
type: mteb/twittersemeval2015-pairclassification
name: MTEB TwitterSemEval2015
config: default
split: test
revision: 70970daeab8776df92f5ea462b6173c0b46fd2d1
metrics:
- type: cos_sim_accuracy
value: 86.53513739047506
- type: cos_sim_ap
value: 75.27741586677557
- type: cos_sim_f1
value: 69.18792902473774
- type: cos_sim_precision
value: 67.94708725515136
- type: cos_sim_recall
value: 70.47493403693932
- type: dot_accuracy
value: 84.7052512368123
- type: dot_ap
value: 69.36075482849378
- type: dot_f1
value: 64.44688376631296
- type: dot_precision
value: 59.92288500793831
- type: dot_recall
value: 69.70976253298153
- type: euclidean_accuracy
value: 86.60666388508076
- type: euclidean_ap
value: 75.47512772621097
- type: euclidean_f1
value: 69.413872536473
- type: euclidean_precision
value: 67.39562624254472
- type: euclidean_recall
value: 71.55672823218997
- type: manhattan_accuracy
value: 86.52917684925792
- type: manhattan_ap
value: 75.34000110496703
- type: manhattan_f1
value: 69.28489190226429
- type: manhattan_precision
value: 67.24608889992551
- type: manhattan_recall
value: 71.45118733509234
- type: max_accuracy
value: 86.60666388508076
- type: max_ap
value: 75.47512772621097
- type: max_f1
value: 69.413872536473
- task:
type: PairClassification
dataset:
type: mteb/twitterurlcorpus-pairclassification
name: MTEB TwitterURLCorpus
config: default
split: test
revision: 8b6510b0b1fa4e4c4f879467980e9be563ec1cdf
metrics:
- type: cos_sim_accuracy
value: 89.01695967710637
- type: cos_sim_ap
value: 85.8298270742901
- type: cos_sim_f1
value: 78.46988128389272
- type: cos_sim_precision
value: 74.86017897091722
- type: cos_sim_recall
value: 82.44533415460425
- type: dot_accuracy
value: 88.19420188613343
- type: dot_ap
value: 83.82679165901324
- type: dot_f1
value: 76.55833777304208
- type: dot_precision
value: 75.6884875846501
- type: dot_recall
value: 77.44841392054204
- type: euclidean_accuracy
value: 89.03054294252338
- type: euclidean_ap
value: 85.89089555185325
- type: euclidean_f1
value: 78.62997658079624
- type: euclidean_precision
value: 74.92329149232914
- type: euclidean_recall
value: 82.72251308900523
- type: manhattan_accuracy
value: 89.0266620095471
- type: manhattan_ap
value: 85.86458997929147
- type: manhattan_f1
value: 78.50685331000291
- type: manhattan_precision
value: 74.5499861534201
- type: manhattan_recall
value: 82.90729904527257
- type: max_accuracy
value: 89.03054294252338
- type: max_ap
value: 85.89089555185325
- type: max_f1
value: 78.62997658079624
language:
- multilingual
- af
- am
- ar
- as
- az
- be
- bg
- bn
- br
- bs
- ca
- cs
- cy
- da
- de
- el
- en
- eo
- es
- et
- eu
- fa
- fi
- fr
- fy
- ga
- gd
- gl
- gu
- ha
- he
- hi
- hr
- hu
- hy
- id
- is
- it
- ja
- jv
- ka
- kk
- km
- kn
- ko
- ku
- ky
- la
- lo
- lt
- lv
- mg
- mk
- ml
- mn
- mr
- ms
- my
- ne
- nl
- 'no'
- om
- or
- pa
- pl
- ps
- pt
- ro
- ru
- sa
- sd
- si
- sk
- sl
- so
- sq
- sr
- su
- sv
- sw
- ta
- te
- th
- tl
- tr
- ug
- uk
- ur
- uz
- vi
- xh
- yi
- zh
license: mit
---
## Multilingual-E5-large
[Multilingual E5 Text Embeddings: A Technical Report](https://arxiv.org/pdf/2402.05672).
Liang Wang, Nan Yang, Xiaolong Huang, Linjun Yang, Rangan Majumder, Furu Wei, arXiv 2024
This model has 24 layers and the embedding size is 1024.
## Usage
Below is an example to encode queries and passages from the MS-MARCO passage ranking dataset.
```python
import torch.nn.functional as F
from torch import Tensor
from transformers import AutoTokenizer, AutoModel
def average_pool(last_hidden_states: Tensor,
attention_mask: Tensor) -> Tensor:
last_hidden = last_hidden_states.masked_fill(~attention_mask[..., None].bool(), 0.0)
return last_hidden.sum(dim=1) / attention_mask.sum(dim=1)[..., None]
# Each input text should start with "query: " or "passage: ", even for non-English texts.
# For tasks other than retrieval, you can simply use the "query: " prefix.
input_texts = ['query: how much protein should a female eat',
'query: 南瓜的家常做法',
"passage: As a general guideline, the CDC's average requirement of protein for women ages 19 to 70 is 46 grams per day. But, as you can see from this chart, you'll need to increase that if you're expecting or training for a marathon. Check out the chart below to see how much protein you should be eating each day.",
"passage: 1.清炒南瓜丝 原料:嫩南瓜半个 调料:葱、盐、白糖、鸡精 做法: 1、南瓜用刀薄薄的削去表面一层皮,用勺子刮去瓤 2、擦成细丝(没有擦菜板就用刀慢慢切成细丝) 3、锅烧热放油,入葱花煸出香味 4、入南瓜丝快速翻炒一分钟左右,放盐、一点白糖和鸡精调味出锅 2.香葱炒南瓜 原料:南瓜1只 调料:香葱、蒜末、橄榄油、盐 做法: 1、将南瓜去皮,切成片 2、油锅8成热后,将蒜末放入爆香 3、爆香后,将南瓜片放入,翻炒 4、在翻炒的同时,可以不时地往锅里加水,但不要太多 5、放入盐,炒匀 6、南瓜差不多软和绵了之后,就可以关火 7、撒入香葱,即可出锅"]
tokenizer = AutoTokenizer.from_pretrained('intfloat/multilingual-e5-large')
model = AutoModel.from_pretrained('intfloat/multilingual-e5-large')
# Tokenize the input texts
batch_dict = tokenizer(input_texts, max_length=512, padding=True, truncation=True, return_tensors='pt')
outputs = model(**batch_dict)
embeddings = average_pool(outputs.last_hidden_state, batch_dict['attention_mask'])
# normalize embeddings
embeddings = F.normalize(embeddings, p=2, dim=1)
scores = (embeddings[:2] @ embeddings[2:].T) * 100
print(scores.tolist())
```
## Supported Languages
This model is initialized from [xlm-roberta-large](https://huggingface.co/xlm-roberta-large)
and continually trained on a mixture of multilingual datasets.
It supports 100 languages from xlm-roberta,
but low-resource languages may see performance degradation.
## Training Details
**Initialization**: [xlm-roberta-large](https://huggingface.co/xlm-roberta-large)
**First stage**: contrastive pre-training with weak supervision
| Dataset | Weak supervision | # of text pairs |
|--------------------------------------------------------------------------------------------------------|---------------------------------------|-----------------|
| Filtered [mC4](https://huggingface.co/datasets/mc4) | (title, page content) | 1B |
| [CC News](https://huggingface.co/datasets/intfloat/multilingual_cc_news) | (title, news content) | 400M |
| [NLLB](https://huggingface.co/datasets/allenai/nllb) | translation pairs | 2.4B |
| [Wikipedia](https://huggingface.co/datasets/intfloat/wikipedia) | (hierarchical section title, passage) | 150M |
| Filtered [Reddit](https://www.reddit.com/) | (comment, response) | 800M |
| [S2ORC](https://github.com/allenai/s2orc) | (title, abstract) and citation pairs | 100M |
| [Stackexchange](https://stackexchange.com/) | (question, answer) | 50M |
| [xP3](https://huggingface.co/datasets/bigscience/xP3) | (input prompt, response) | 80M |
| [Miscellaneous unsupervised SBERT data](https://huggingface.co/sentence-transformers/all-MiniLM-L6-v2) | - | 10M |
**Second stage**: supervised fine-tuning
| Dataset | Language | # of text pairs |
|----------------------------------------------------------------------------------------|--------------|-----------------|
| [MS MARCO](https://microsoft.github.io/msmarco/) | English | 500k |
| [NQ](https://github.com/facebookresearch/DPR) | English | 70k |
| [Trivia QA](https://github.com/facebookresearch/DPR) | English | 60k |
| [NLI from SimCSE](https://github.com/princeton-nlp/SimCSE) | English | <300k |
| [ELI5](https://huggingface.co/datasets/eli5) | English | 500k |
| [DuReader Retrieval](https://github.com/baidu/DuReader/tree/master/DuReader-Retrieval) | Chinese | 86k |
| [KILT Fever](https://huggingface.co/datasets/kilt_tasks) | English | 70k |
| [KILT HotpotQA](https://huggingface.co/datasets/kilt_tasks) | English | 70k |
| [SQuAD](https://huggingface.co/datasets/squad) | English | 87k |
| [Quora](https://huggingface.co/datasets/quora) | English | 150k |
| [Mr. TyDi](https://huggingface.co/datasets/castorini/mr-tydi) | 11 languages | 50k |
| [MIRACL](https://huggingface.co/datasets/miracl/miracl) | 16 languages | 40k |
For all labeled datasets, we only use its training set for fine-tuning.
For other training details, please refer to our paper at [https://arxiv.org/pdf/2402.05672](https://arxiv.org/pdf/2402.05672).
## Benchmark Results on [Mr. TyDi](https://arxiv.org/abs/2108.08787)
| Model | Avg MRR@10 | | ar | bn | en | fi | id | ja | ko | ru | sw | te | th |
|-----------------------|------------|-------|------| --- | --- | --- | --- | --- | --- | --- |------| --- | --- |
| BM25 | 33.3 | | 36.7 | 41.3 | 15.1 | 28.8 | 38.2 | 21.7 | 28.1 | 32.9 | 39.6 | 42.4 | 41.7 |
| mDPR | 16.7 | | 26.0 | 25.8 | 16.2 | 11.3 | 14.6 | 18.1 | 21.9 | 18.5 | 7.3 | 10.6 | 13.5 |
| BM25 + mDPR | 41.7 | | 49.1 | 53.5 | 28.4 | 36.5 | 45.5 | 35.5 | 36.2 | 42.7 | 40.5 | 42.0 | 49.2 |
| | |
| multilingual-e5-small | 64.4 | | 71.5 | 66.3 | 54.5 | 57.7 | 63.2 | 55.4 | 54.3 | 60.8 | 65.4 | 89.1 | 70.1 |
| multilingual-e5-base | 65.9 | | 72.3 | 65.0 | 58.5 | 60.8 | 64.9 | 56.6 | 55.8 | 62.7 | 69.0 | 86.6 | 72.7 |
| multilingual-e5-large | **70.5** | | 77.5 | 73.2 | 60.8 | 66.8 | 68.5 | 62.5 | 61.6 | 65.8 | 72.7 | 90.2 | 76.2 |
## MTEB Benchmark Evaluation
Check out [unilm/e5](https://github.com/microsoft/unilm/tree/master/e5) to reproduce evaluation results
on the [BEIR](https://arxiv.org/abs/2104.08663) and [MTEB benchmark](https://arxiv.org/abs/2210.07316).
## Support for Sentence Transformers
Below is an example for usage with sentence_transformers.
```python
from sentence_transformers import SentenceTransformer
model = SentenceTransformer('intfloat/multilingual-e5-large')
input_texts = [
'query: how much protein should a female eat',
'query: 南瓜的家常做法',
"passage: As a general guideline, the CDC's average requirement of protein for women ages 19 to 70 i s 46 grams per day. But, as you can see from this chart, you'll need to increase that if you're expecting or traini ng for a marathon. Check out the chart below to see how much protein you should be eating each day.",
"passage: 1.清炒南瓜丝 原料:嫩南瓜半个 调料:葱、盐、白糖、鸡精 做法: 1、南瓜用刀薄薄的削去表面一层皮 ,用勺子刮去瓤 2、擦成细丝(没有擦菜板就用刀慢慢切成细丝) 3、锅烧热放油,入葱花煸出香味 4、入南瓜丝快速翻炒一分钟左右, 放盐、一点白糖和鸡精调味出锅 2.香葱炒南瓜 原料:南瓜1只 调料:香葱、蒜末、橄榄油、盐 做法: 1、将南瓜去皮,切成片 2、油 锅8成热后,将蒜末放入爆香 3、爆香后,将南瓜片放入,翻炒 4、在翻炒的同时,可以不时地往锅里加水,但不要太多 5、放入盐,炒匀 6、南瓜差不多软和绵了之后,就可以关火 7、撒入香葱,即可出锅"
]
embeddings = model.encode(input_texts, normalize_embeddings=True)
```
Package requirements
`pip install sentence_transformers~=2.2.2`
Contributors: [michaelfeil](https://huggingface.co/michaelfeil)
## FAQ
**1. Do I need to add the prefix "query: " and "passage: " to input texts?**
Yes, this is how the model is trained, otherwise you will see a performance degradation.
Here are some rules of thumb:
- Use "query: " and "passage: " correspondingly for asymmetric tasks such as passage retrieval in open QA, ad-hoc information retrieval.
- Use "query: " prefix for symmetric tasks such as semantic similarity, bitext mining, paraphrase retrieval.
- Use "query: " prefix if you want to use embeddings as features, such as linear probing classification, clustering.
**2. Why are my reproduced results slightly different from reported in the model card?**
Different versions of `transformers` and `pytorch` could cause negligible but non-zero performance differences.
**3. Why does the cosine similarity scores distribute around 0.7 to 1.0?**
This is a known and expected behavior as we use a low temperature 0.01 for InfoNCE contrastive loss.
For text embedding tasks like text retrieval or semantic similarity,
what matters is the relative order of the scores instead of the absolute values,
so this should not be an issue.
## Citation
If you find our paper or models helpful, please consider cite as follows:
```
@article{wang2024multilingual,
title={Multilingual E5 Text Embeddings: A Technical Report},
author={Wang, Liang and Yang, Nan and Huang, Xiaolong and Yang, Linjun and Majumder, Rangan and Wei, Furu},
journal={arXiv preprint arXiv:2402.05672},
year={2024}
}
```
## Limitations
Long texts will be truncated to at most 512 tokens.
|
google-t5/t5-base | google-t5 | "2024-02-14T17:21:55Z" | 2,492,337 | 615 | transformers | [
"transformers",
"pytorch",
"tf",
"jax",
"rust",
"safetensors",
"t5",
"text2text-generation",
"summarization",
"translation",
"en",
"fr",
"ro",
"de",
"dataset:c4",
"arxiv:1805.12471",
"arxiv:1708.00055",
"arxiv:1704.05426",
"arxiv:1606.05250",
"arxiv:1808.09121",
"arxiv:1810.12885",
"arxiv:1905.10044",
"arxiv:1910.09700",
"license:apache-2.0",
"autotrain_compatible",
"text-generation-inference",
"endpoints_compatible",
"region:us"
] | translation | "2022-03-02T23:29:04Z" | ---
pipeline_tag: translation
language:
- en
- fr
- ro
- de
datasets:
- c4
tags:
- summarization
- translation
license: apache-2.0
---
# Model Card for T5 Base

# Table of Contents
1. [Model Details](#model-details)
2. [Uses](#uses)
3. [Bias, Risks, and Limitations](#bias-risks-and-limitations)
4. [Training Details](#training-details)
5. [Evaluation](#evaluation)
6. [Environmental Impact](#environmental-impact)
7. [Citation](#citation)
8. [Model Card Authors](#model-card-authors)
9. [How To Get Started With the Model](#how-to-get-started-with-the-model)
# Model Details
## Model Description
The developers of the Text-To-Text Transfer Transformer (T5) [write](https://ai.googleblog.com/2020/02/exploring-transfer-learning-with-t5.html):
> With T5, we propose reframing all NLP tasks into a unified text-to-text-format where the input and output are always text strings, in contrast to BERT-style models that can only output either a class label or a span of the input. Our text-to-text framework allows us to use the same model, loss function, and hyperparameters on any NLP task.
T5-Base is the checkpoint with 220 million parameters.
- **Developed by:** Colin Raffel, Noam Shazeer, Adam Roberts, Katherine Lee, Sharan Narang, Michael Matena, Yanqi Zhou, Wei Li, Peter J. Liu. See [associated paper](https://jmlr.org/papers/volume21/20-074/20-074.pdf) and [GitHub repo](https://github.com/google-research/text-to-text-transfer-transformer#released-model-checkpoints)
- **Model type:** Language model
- **Language(s) (NLP):** English, French, Romanian, German
- **License:** Apache 2.0
- **Related Models:** [All T5 Checkpoints](https://huggingface.co/models?search=t5)
- **Resources for more information:**
- [Research paper](https://jmlr.org/papers/volume21/20-074/20-074.pdf)
- [Google's T5 Blog Post](https://ai.googleblog.com/2020/02/exploring-transfer-learning-with-t5.html)
- [GitHub Repo](https://github.com/google-research/text-to-text-transfer-transformer)
- [Hugging Face T5 Docs](https://huggingface.co/docs/transformers/model_doc/t5)
# Uses
## Direct Use and Downstream Use
The developers write in a [blog post](https://ai.googleblog.com/2020/02/exploring-transfer-learning-with-t5.html) that the model:
> Our text-to-text framework allows us to use the same model, loss function, and hyperparameters on any NLP task, including machine translation, document summarization, question answering, and classification tasks (e.g., sentiment analysis). We can even apply T5 to regression tasks by training it to predict the string representation of a number instead of the number itself.
See the [blog post](https://ai.googleblog.com/2020/02/exploring-transfer-learning-with-t5.html) and [research paper](https://jmlr.org/papers/volume21/20-074/20-074.pdf) for further details.
## Out-of-Scope Use
More information needed.
# Bias, Risks, and Limitations
More information needed.
## Recommendations
More information needed.
# Training Details
## Training Data
The model is pre-trained on the [Colossal Clean Crawled Corpus (C4)](https://www.tensorflow.org/datasets/catalog/c4), which was developed and released in the context of the same [research paper](https://jmlr.org/papers/volume21/20-074/20-074.pdf) as T5.
The model was pre-trained on a on a **multi-task mixture of unsupervised (1.) and supervised tasks (2.)**.
Thereby, the following datasets were being used for (1.) and (2.):
1. **Datasets used for Unsupervised denoising objective**:
- [C4](https://huggingface.co/datasets/c4)
- [Wiki-DPR](https://huggingface.co/datasets/wiki_dpr)
2. **Datasets used for Supervised text-to-text language modeling objective**
- Sentence acceptability judgment
- CoLA [Warstadt et al., 2018](https://arxiv.org/abs/1805.12471)
- Sentiment analysis
- SST-2 [Socher et al., 2013](https://nlp.stanford.edu/~socherr/EMNLP2013_RNTN.pdf)
- Paraphrasing/sentence similarity
- MRPC [Dolan and Brockett, 2005](https://aclanthology.org/I05-5002)
- STS-B [Ceret al., 2017](https://arxiv.org/abs/1708.00055)
- QQP [Iyer et al., 2017](https://quoradata.quora.com/First-Quora-Dataset-Release-Question-Pairs)
- Natural language inference
- MNLI [Williams et al., 2017](https://arxiv.org/abs/1704.05426)
- QNLI [Rajpurkar et al.,2016](https://arxiv.org/abs/1606.05250)
- RTE [Dagan et al., 2005](https://link.springer.com/chapter/10.1007/11736790_9)
- CB [De Marneff et al., 2019](https://semanticsarchive.net/Archive/Tg3ZGI2M/Marneffe.pdf)
- Sentence completion
- COPA [Roemmele et al., 2011](https://www.researchgate.net/publication/221251392_Choice_of_Plausible_Alternatives_An_Evaluation_of_Commonsense_Causal_Reasoning)
- Word sense disambiguation
- WIC [Pilehvar and Camacho-Collados, 2018](https://arxiv.org/abs/1808.09121)
- Question answering
- MultiRC [Khashabi et al., 2018](https://aclanthology.org/N18-1023)
- ReCoRD [Zhang et al., 2018](https://arxiv.org/abs/1810.12885)
- BoolQ [Clark et al., 2019](https://arxiv.org/abs/1905.10044)
## Training Procedure
In their [abstract](https://jmlr.org/papers/volume21/20-074/20-074.pdf), the model developers write:
> In this paper, we explore the landscape of transfer learning techniques for NLP by introducing a unified framework that converts every language problem into a text-to-text format. Our systematic study compares pre-training objectives, architectures, unlabeled datasets, transfer approaches, and other factors on dozens of language understanding tasks.
The framework introduced, the T5 framework, involves a training procedure that brings together the approaches studied in the paper. See the [research paper](https://jmlr.org/papers/volume21/20-074/20-074.pdf) for further details.
# Evaluation
## Testing Data, Factors & Metrics
The developers evaluated the model on 24 tasks, see the [research paper](https://jmlr.org/papers/volume21/20-074/20-074.pdf) for full details.
## Results
For full results for T5-Base, see the [research paper](https://jmlr.org/papers/volume21/20-074/20-074.pdf), Table 14.
# Environmental Impact
Carbon emissions can be estimated using the [Machine Learning Impact calculator](https://mlco2.github.io/impact#compute) presented in [Lacoste et al. (2019)](https://arxiv.org/abs/1910.09700).
- **Hardware Type:** Google Cloud TPU Pods
- **Hours used:** More information needed
- **Cloud Provider:** GCP
- **Compute Region:** More information needed
- **Carbon Emitted:** More information needed
# Citation
**BibTeX:**
```bibtex
@article{2020t5,
author = {Colin Raffel and Noam Shazeer and Adam Roberts and Katherine Lee and Sharan Narang and Michael Matena and Yanqi Zhou and Wei Li and Peter J. Liu},
title = {Exploring the Limits of Transfer Learning with a Unified Text-to-Text Transformer},
journal = {Journal of Machine Learning Research},
year = {2020},
volume = {21},
number = {140},
pages = {1-67},
url = {http://jmlr.org/papers/v21/20-074.html}
}
```
**APA:**
- Raffel, C., Shazeer, N., Roberts, A., Lee, K., Narang, S., Matena, M., ... & Liu, P. J. (2020). Exploring the limits of transfer learning with a unified text-to-text transformer. J. Mach. Learn. Res., 21(140), 1-67.
# Model Card Authors
This model card was written by the team at Hugging Face.
# How to Get Started with the Model
Use the code below to get started with the model.
<details>
<summary> Click to expand </summary>
```python
from transformers import T5Tokenizer, T5Model
tokenizer = T5Tokenizer.from_pretrained("t5-base")
model = T5Model.from_pretrained("t5-base")
input_ids = tokenizer(
"Studies have been shown that owning a dog is good for you", return_tensors="pt"
).input_ids # Batch size 1
decoder_input_ids = tokenizer("Studies show that", return_tensors="pt").input_ids # Batch size 1
# forward pass
outputs = model(input_ids=input_ids, decoder_input_ids=decoder_input_ids)
last_hidden_states = outputs.last_hidden_state
```
See the [Hugging Face T5](https://huggingface.co/docs/transformers/model_doc/t5#transformers.T5Model) docs and a [Colab Notebook](https://colab.research.google.com/github/google-research/text-to-text-transfer-transformer/blob/main/notebooks/t5-trivia.ipynb) created by the model developers for more examples.
</details> |
meta-llama/Llama-3.2-11B-Vision-Instruct | meta-llama | "2024-09-30T03:03:34Z" | 2,388,364 | 915 | transformers | [
"transformers",
"safetensors",
"mllama",
"image-text-to-text",
"facebook",
"meta",
"pytorch",
"llama",
"llama-3",
"conversational",
"en",
"de",
"fr",
"it",
"pt",
"hi",
"es",
"th",
"arxiv:2204.05149",
"license:llama3.2",
"text-generation-inference",
"endpoints_compatible",
"region:us"
] | image-text-to-text | "2024-09-18T16:47:28Z" | ---
language:
- en
- de
- fr
- it
- pt
- hi
- es
- th
library_name: transformers
pipeline_tag: image-text-to-text
tags:
- facebook
- meta
- pytorch
- llama
- llama-3
widget:
- example_title: rococo art
messages:
- role: user
content:
- type: text
text: Which era does this piece belong to? Give details about the era.
- type: image_url
image_url:
url: https://huggingface.co/datasets/huggingface/release-assets/resolve/main/rococo.jpg
- role: assistant
content: |
The piece is a painting of a woman in a red dress, surrounded by gold and white ornate details. The woman is depicted in mid-air, with her arms outstretched and her legs bent at the knees. She is holding a bouquet of flowers in her right hand and a fruit in her left hand.
The background of the painting is a light blue sky with pink clouds, and there are also some pink flowers and green leaves surrounding the woman. The overall atmosphere of the painting is one of joy and celebration, as if the woman is dancing or celebrating something.
This piece belongs to the Rococo era, which was a style of art and architecture that emerged in Europe in the 18th century. The Rococo style is characterized by its use of pastel colors, curved lines, and ornate details. It was popularized during the reign of King Louis XV of France, who ruled from 1715 to 1774.
- example_title: diagram understanding
messages:
- role: user
content:
- type: text
text: Where do the droughts happen according to this diagram?
- type: image_url
image_url:
url: https://huggingface.co/datasets/huggingface/release-assets/resolve/main/weather_events.png
- role: assistant
content: |
# Drought Regions in 2016
According to the diagram, the regions that experienced severe droughts in 2016 are:
- **Eastern and Southern Africa**: This region was severely affected by droughts, with significant impacts on agriculture and water resources.
- **Western and Southern Africa**: Droughts also occurred in this region, leading to food insecurity and other challenges.
These regions were particularly vulnerable to droughts in 2016, highlighting the need for effective water management strategies and climate resilience measures.
- example_title: document qa
messages:
- role: user
content:
- type: text
text: How long does it take from invoice date to due date? Be short and concise.
- type: image_url
image_url:
url: https://huggingface.co/datasets/huggingface/release-assets/resolve/main/invoice.png
- role: assistant
content: |
To determine the time gap between the invoice date and the due date, we need to calculate the difference between these two dates.
Invoice Date: February 11, 2019
Due Date: February 26, 2019
Step 1: Calculate the Difference in Days
February 26 - February 11 = 15 days
Therefore, the time gap between the invoice date and the due date is 15 days.
license: llama3.2
extra_gated_prompt: >-
### LLAMA 3.2 COMMUNITY LICENSE AGREEMENT
Llama 3.2 Version Release Date: September 25, 2024
“Agreement” means the terms and conditions for use, reproduction, distribution
and modification of the Llama Materials set forth herein.
“Documentation” means the specifications, manuals and documentation accompanying Llama 3.2
distributed by Meta at https://llama.meta.com/doc/overview.
“Licensee” or “you” means you, or your employer or any other person or entity (if you are
entering into this Agreement on such person or entity’s behalf), of the age required under
applicable laws, rules or regulations to provide legal consent and that has legal authority
to bind your employer or such other person or entity if you are entering in this Agreement
on their behalf.
“Llama 3.2” means the foundational large language models and software and algorithms, including
machine-learning model code, trained model weights, inference-enabling code, training-enabling code,
fine-tuning enabling code and other elements of the foregoing distributed by Meta at
https://www.llama.com/llama-downloads.
“Llama Materials” means, collectively, Meta’s proprietary Llama 3.2 and Documentation (and
any portion thereof) made available under this Agreement.
“Meta” or “we” means Meta Platforms Ireland Limited (if you are located in or,
if you are an entity, your principal place of business is in the EEA or Switzerland)
and Meta Platforms, Inc. (if you are located outside of the EEA or Switzerland).
By clicking “I Accept” below or by using or distributing any portion or element of the Llama Materials,
you agree to be bound by this Agreement.
1. License Rights and Redistribution.
a. Grant of Rights. You are granted a non-exclusive, worldwide,
non-transferable and royalty-free limited license under Meta’s intellectual property or other rights
owned by Meta embodied in the Llama Materials to use, reproduce, distribute, copy, create derivative works
of, and make modifications to the Llama Materials.
b. Redistribution and Use.
i. If you distribute or make available the Llama Materials (or any derivative works thereof),
or a product or service (including another AI model) that contains any of them, you shall (A) provide
a copy of this Agreement with any such Llama Materials; and (B) prominently display “Built with Llama”
on a related website, user interface, blogpost, about page, or product documentation. If you use the
Llama Materials or any outputs or results of the Llama Materials to create, train, fine tune, or
otherwise improve an AI model, which is distributed or made available, you shall also include “Llama”
at the beginning of any such AI model name.
ii. If you receive Llama Materials, or any derivative works thereof, from a Licensee as part
of an integrated end user product, then Section 2 of this Agreement will not apply to you.
iii. You must retain in all copies of the Llama Materials that you distribute the
following attribution notice within a “Notice” text file distributed as a part of such copies:
“Llama 3.2 is licensed under the Llama 3.2 Community License, Copyright © Meta Platforms,
Inc. All Rights Reserved.”
iv. Your use of the Llama Materials must comply with applicable laws and regulations
(including trade compliance laws and regulations) and adhere to the Acceptable Use Policy for
the Llama Materials (available at https://www.llama.com/llama3_2/use-policy), which is hereby
incorporated by reference into this Agreement.
2. Additional Commercial Terms. If, on the Llama 3.2 version release date, the monthly active users
of the products or services made available by or for Licensee, or Licensee’s affiliates,
is greater than 700 million monthly active users in the preceding calendar month, you must request
a license from Meta, which Meta may grant to you in its sole discretion, and you are not authorized to
exercise any of the rights under this Agreement unless or until Meta otherwise expressly grants you such rights.
3. Disclaimer of Warranty. UNLESS REQUIRED BY APPLICABLE LAW, THE LLAMA MATERIALS AND ANY OUTPUT AND
RESULTS THEREFROM ARE PROVIDED ON AN “AS IS” BASIS, WITHOUT WARRANTIES OF ANY KIND, AND META DISCLAIMS
ALL WARRANTIES OF ANY KIND, BOTH EXPRESS AND IMPLIED, INCLUDING, WITHOUT LIMITATION, ANY WARRANTIES
OF TITLE, NON-INFRINGEMENT, MERCHANTABILITY, OR FITNESS FOR A PARTICULAR PURPOSE. YOU ARE SOLELY RESPONSIBLE
FOR DETERMINING THE APPROPRIATENESS OF USING OR REDISTRIBUTING THE LLAMA MATERIALS AND ASSUME ANY RISKS ASSOCIATED
WITH YOUR USE OF THE LLAMA MATERIALS AND ANY OUTPUT AND RESULTS.
4. Limitation of Liability. IN NO EVENT WILL META OR ITS AFFILIATES BE LIABLE UNDER ANY THEORY OF LIABILITY,
WHETHER IN CONTRACT, TORT, NEGLIGENCE, PRODUCTS LIABILITY, OR OTHERWISE, ARISING OUT OF THIS AGREEMENT,
FOR ANY LOST PROFITS OR ANY INDIRECT, SPECIAL, CONSEQUENTIAL, INCIDENTAL, EXEMPLARY OR PUNITIVE DAMAGES, EVEN
IF META OR ITS AFFILIATES HAVE BEEN ADVISED OF THE POSSIBILITY OF ANY OF THE FOREGOING.
5. Intellectual Property.
a. No trademark licenses are granted under this Agreement, and in connection with the Llama Materials,
neither Meta nor Licensee may use any name or mark owned by or associated with the other or any of its affiliates,
except as required for reasonable and customary use in describing and redistributing the Llama Materials or as
set forth in this Section 5(a). Meta hereby grants you a license to use “Llama” (the “Mark”) solely as required
to comply with the last sentence of Section 1.b.i. You will comply with Meta’s brand guidelines (currently accessible
at https://about.meta.com/brand/resources/meta/company-brand/). All goodwill arising out of your use of the Mark
will inure to the benefit of Meta.
b. Subject to Meta’s ownership of Llama Materials and derivatives made by or for Meta, with respect to any
derivative works and modifications of the Llama Materials that are made by you, as between you and Meta,
you are and will be the owner of such derivative works and modifications.
c. If you institute litigation or other proceedings against Meta or any entity (including a cross-claim or
counterclaim in a lawsuit) alleging that the Llama Materials or Llama 3.2 outputs or results, or any portion
of any of the foregoing, constitutes infringement of intellectual property or other rights owned or licensable
by you, then any licenses granted to you under this Agreement shall terminate as of the date such litigation or
claim is filed or instituted. You will indemnify and hold harmless Meta from and against any claim by any third
party arising out of or related to your use or distribution of the Llama Materials.
6. Term and Termination. The term of this Agreement will commence upon your acceptance of this Agreement or access
to the Llama Materials and will continue in full force and effect until terminated in accordance with the terms
and conditions herein. Meta may terminate this Agreement if you are in breach of any term or condition of this
Agreement. Upon termination of this Agreement, you shall delete and cease use of the Llama Materials. Sections 3,
4 and 7 shall survive the termination of this Agreement.
7. Governing Law and Jurisdiction. This Agreement will be governed and construed under the laws of the State of
California without regard to choice of law principles, and the UN Convention on Contracts for the International
Sale of Goods does not apply to this Agreement. The courts of California shall have exclusive jurisdiction of
any dispute arising out of this Agreement.
### Llama 3.2 Acceptable Use Policy
Meta is committed to promoting safe and fair use of its tools and features, including Llama 3.2.
If you access or use Llama 3.2, you agree to this Acceptable Use Policy (“**Policy**”).
The most recent copy of this policy can be found at
[https://www.llama.com/llama3_2/use-policy](https://www.llama.com/llama3_2/use-policy).
#### Prohibited Uses
We want everyone to use Llama 3.2 safely and responsibly. You agree you will not use, or allow others to use, Llama 3.2 to:
1. Violate the law or others’ rights, including to:
1. Engage in, promote, generate, contribute to, encourage, plan, incite, or further illegal or unlawful activity or content, such as:
1. Violence or terrorism
2. Exploitation or harm to children, including the solicitation, creation, acquisition, or dissemination of child exploitative content or failure to report Child Sexual Abuse Material
3. Human trafficking, exploitation, and sexual violence
4. The illegal distribution of information or materials to minors, including obscene materials, or failure to employ legally required age-gating in connection with such information or materials.
5. Sexual solicitation
6. Any other criminal activity
1. Engage in, promote, incite, or facilitate the harassment, abuse, threatening, or bullying of individuals or groups of individuals
2. Engage in, promote, incite, or facilitate discrimination or other unlawful or harmful conduct in the provision of employment, employment benefits, credit, housing, other economic benefits, or other essential goods and services
3. Engage in the unauthorized or unlicensed practice of any profession including, but not limited to, financial, legal, medical/health, or related professional practices
4. Collect, process, disclose, generate, or infer private or sensitive information about individuals, including information about individuals’ identity, health, or demographic information, unless you have obtained the right to do so in accordance with applicable law
5. Engage in or facilitate any action or generate any content that infringes, misappropriates, or otherwise violates any third-party rights, including the outputs or results of any products or services using the Llama Materials
6. Create, generate, or facilitate the creation of malicious code, malware, computer viruses or do anything else that could disable, overburden, interfere with or impair the proper working, integrity, operation or appearance of a website or computer system
7. Engage in any action, or facilitate any action, to intentionally circumvent or remove usage restrictions or other safety measures, or to enable functionality disabled by Meta
2. Engage in, promote, incite, facilitate, or assist in the planning or development of activities that present a risk of death or bodily harm to individuals, including use of Llama 3.2 related to the following:
8. Military, warfare, nuclear industries or applications, espionage, use for materials or activities that are subject to the International Traffic Arms Regulations (ITAR) maintained by the United States Department of State or to the U.S. Biological Weapons Anti-Terrorism Act of 1989 or the Chemical Weapons Convention Implementation Act of 1997
9. Guns and illegal weapons (including weapon development)
10. Illegal drugs and regulated/controlled substances
11. Operation of critical infrastructure, transportation technologies, or heavy machinery
12. Self-harm or harm to others, including suicide, cutting, and eating disorders
13. Any content intended to incite or promote violence, abuse, or any infliction of bodily harm to an individual
3. Intentionally deceive or mislead others, including use of Llama 3.2 related to the following:
14. Generating, promoting, or furthering fraud or the creation or promotion of disinformation
15. Generating, promoting, or furthering defamatory content, including the creation of defamatory statements, images, or other content
16. Generating, promoting, or further distributing spam
17. Impersonating another individual without consent, authorization, or legal right
18. Representing that the use of Llama 3.2 or outputs are human-generated
19. Generating or facilitating false online engagement, including fake reviews and other means of fake online engagement
4. Fail to appropriately disclose to end users any known dangers of your AI system
5. Interact with third party tools, models, or software designed to generate unlawful content or engage in unlawful or harmful conduct and/or represent that the outputs of such tools, models, or software are associated with Meta or Llama 3.2
With respect to any multimodal models included in Llama 3.2, the rights granted under Section 1(a) of the Llama 3.2 Community License Agreement are not being granted to you if you are an individual domiciled in, or a company with a principal place of business in, the European Union. This restriction does not apply to end users of a product or service that incorporates any such multimodal models.
Please report any violation of this Policy, software “bug,” or other problems that could lead to a violation of this Policy through one of the following means:
* Reporting issues with the model: [https://github.com/meta-llama/llama-models/issues](https://l.workplace.com/l.php?u=https%3A%2F%2Fgithub.com%2Fmeta-llama%2Fllama-models%2Fissues&h=AT0qV8W9BFT6NwihiOHRuKYQM_UnkzN_NmHMy91OT55gkLpgi4kQupHUl0ssR4dQsIQ8n3tfd0vtkobvsEvt1l4Ic6GXI2EeuHV8N08OG2WnbAmm0FL4ObkazC6G_256vN0lN9DsykCvCqGZ)
* Reporting risky content generated by the model: [developers.facebook.com/llama_output_feedback](http://developers.facebook.com/llama_output_feedback)
* Reporting bugs and security concerns: [facebook.com/whitehat/info](http://facebook.com/whitehat/info)
* Reporting violations of the Acceptable Use Policy or unlicensed uses of Llama 3.2: [email protected]
extra_gated_fields:
First Name: text
Last Name: text
Date of birth: date_picker
Country: country
Affiliation: text
Job title:
type: select
options:
- Student
- Research Graduate
- AI researcher
- AI developer/engineer
- Reporter
- Other
geo: ip_location
By clicking Submit below I accept the terms of the license and acknowledge that the information I provide will be collected stored processed and shared in accordance with the Meta Privacy Policy: checkbox
extra_gated_description: >-
The information you provide will be collected, stored, processed and shared in
accordance with the [Meta Privacy
Policy](https://www.facebook.com/privacy/policy/).
extra_gated_button_content: Submit
extra_gated_eu_disallowed: true
---
## Model Information
The Llama 3.2-Vision collection of multimodal large language models (LLMs) is a collection of pretrained and instruction-tuned image reasoning generative models in 11B and 90B sizes (text \+ images in / text out). The Llama 3.2-Vision instruction-tuned models are optimized for visual recognition, image reasoning, captioning, and answering general questions about an image. The models outperform many of the available open source and closed multimodal models on common industry benchmarks.
**Model Developer**: Meta
**Model Architecture:** Llama 3.2-Vision is built on top of Llama 3.1 text-only model, which is an auto-regressive language model that uses an optimized transformer architecture. The tuned versions use supervised fine-tuning (SFT) and reinforcement learning with human feedback (RLHF) to align with human preferences for helpfulness and safety. To support image recognition tasks, the Llama 3.2-Vision model uses a separately trained vision adapter that integrates with the pre-trained Llama 3.1 language model. The adapter consists of a series of cross-attention layers that feed image encoder representations into the core LLM.
| | Training Data | Params | Input modalities | Output modalities | Context length | GQA | Data volume | Knowledge cutoff |
| :---- | :---- | :---- | :---- | :---- | :---- | :---- | :---- | :---- |
| Llama 3.2-Vision | (Image, text) pairs | 11B (10.6) | Text \+ Image | Text | 128k | Yes | 6B (image, text) pairs | December 2023 |
| Llama 3.2-Vision | (Image, text) pairs | 90B (88.8) | Text \+ Image | Text | 128k | Yes | 6B (image, text) pairs | December 2023 |
**Supported Languages:** For text only tasks, English, German, French, Italian, Portuguese, Hindi, Spanish, and Thai are officially supported. Llama 3.2 has been trained on a broader collection of languages than these 8 supported languages. Note for image+text applications, English is the only language supported.
Developers may fine-tune Llama 3.2 models for languages beyond these supported languages, provided they comply with the Llama 3.2 Community License and the Acceptable Use Policy. Developers are always expected to ensure that their deployments, including those that involve additional languages, are completed safely and responsibly.
**Llama 3.2 Model Family:** Token counts refer to pretraining data only. All model versions use Grouped-Query Attention (GQA) for improved inference scalability.
**Model Release Date:** Sept 25, 2024
**Status:** This is a static model trained on an offline dataset. Future versions may be released that improve model capabilities and safety.
**License:** Use of Llama 3.2 is governed by the [Llama 3.2 Community License](https://github.com/meta-llama/llama-models/blob/main/models/llama3_2/LICENSE) (a custom, commercial license agreement).
**Feedback:** Where to send questions or comments about the model Instructions on how to provide feedback or comments on the model can be found in the model [README](https://github.com/meta-llama/llama-models/tree/main/models/llama3_2). For more technical information about generation parameters and recipes for how to use Llama 3.2-Vision in applications, please go [here](https://github.com/meta-llama/llama-recipes).
## Intended Use
**Intended Use Cases:** Llama 3.2-Vision is intended for commercial and research use. Instruction tuned models are intended for visual recognition, image reasoning, captioning, and assistant-like chat with images, whereas pretrained models can be adapted for a variety of image reasoning tasks. Additionally, because of Llama 3.2-Vision’s ability to take images and text as inputs, additional use cases could include:
1. Visual Question Answering (VQA) and Visual Reasoning: Imagine a machine that looks at a picture and understands your questions about it.
2. Document Visual Question Answering (DocVQA): Imagine a computer understanding both the text and layout of a document, like a map or contract, and then answering questions about it directly from the image.
3. Image Captioning: Image captioning bridges the gap between vision and language, extracting details, understanding the scene, and then crafting a sentence or two that tells the story.
4. Image-Text Retrieval: Image-text retrieval is like a matchmaker for images and their descriptions. Similar to a search engine but one that understands both pictures and words.
5. Visual Grounding: Visual grounding is like connecting the dots between what we see and say. It’s about understanding how language references specific parts of an image, allowing AI models to pinpoint objects or regions based on natural language descriptions.
The Llama 3.2 model collection also supports the ability to leverage the outputs of its models to improve other models including synthetic data generation and distillation. The Llama 3.2 Community License allows for these use cases.
**Out of Scope:** Use in any manner that violates applicable laws or regulations (including trade compliance laws). Use in any other way that is prohibited by the Acceptable Use Policy and Llama 3.2 Community License. Use in languages beyond those explicitly referenced as supported in this model card.
## How to use
This repository contains two versions of Llama-3.2-11B-Vision-Instruct, for use with transformers and with the original `llama` codebase.
### Use with transformers
Starting with transformers >= 4.45.0 onward, you can run inference using conversational messages that may include an image you can query about.
Make sure to update your transformers installation via `pip install --upgrade transformers`.
```python
import requests
import torch
from PIL import Image
from transformers import MllamaForConditionalGeneration, AutoProcessor
model_id = "meta-llama/Llama-3.2-11B-Vision-Instruct"
model = MllamaForConditionalGeneration.from_pretrained(
model_id,
torch_dtype=torch.bfloat16,
device_map="auto",
)
processor = AutoProcessor.from_pretrained(model_id)
url = "https://huggingface.co/datasets/huggingface/documentation-images/resolve/0052a70beed5bf71b92610a43a52df6d286cd5f3/diffusers/rabbit.jpg"
image = Image.open(requests.get(url, stream=True).raw)
messages = [
{"role": "user", "content": [
{"type": "image"},
{"type": "text", "text": "If I had to write a haiku for this one, it would be: "}
]}
]
input_text = processor.apply_chat_template(messages, add_generation_prompt=True)
inputs = processor(
image,
input_text,
add_special_tokens=False,
return_tensors="pt"
).to(model.device)
output = model.generate(**inputs, max_new_tokens=30)
print(processor.decode(output[0]))
```
### Use with `llama`
Please, follow the instructions in the [repository](https://github.com/meta-llama/llama).
To download the original checkpoints, you can use `huggingface-cli` as follows:
```
huggingface-cli download meta-llama/Llama-3.2-11B-Vision-Instruct --include "original/*" --local-dir Llama-3.2-11B-Vision-Instruct
```
## Hardware and Software
**Training Factors:** We used custom training libraries, Meta's custom built GPU cluster, and production infrastructure for pretraining. Fine-tuning, annotation, and evaluation were also performed on production infrastructure.
**Training Energy Use:** Training utilized a cumulative of **2.02M** GPU hours of computation on H100-80GB (TDP of 700W) type hardware, per the table below. Training time is the total GPU time required for training each model and power consumption is the peak power capacity per GPU device used, adjusted for power usage efficiency.
##
**Training Greenhouse Gas Emissions:** Estimated total location-based greenhouse gas emissions were **584** tons CO2eq for training. Since 2020, Meta has maintained net zero greenhouse gas emissions in its global operations and matched 100% of its electricity use with renewable energy, therefore the total market-based greenhouse gas emissions for training were 0 tons CO2eq.
| | Training Time (GPU hours) | Training Power Consumption (W) | Training Location-Based Greenhouse Gas Emissions (tons CO2eq) | Training Market-Based Greenhouse Gas Emissions (tons CO2eq) |
| :---- | :---: | :---: | :---: | :---: |
| Llama 3.2-vision 11B | Stage 1 pretraining: 147K H100 hours Stage 2 annealing: 98K H100 hours SFT: 896 H100 hours RLHF: 224 H100 hours | 700 | 71 | 0 |
| Llama 3.2-vision 90B | Stage 1 pretraining: 885K H100 hours Stage 2 annealing: 885K H100 hours SFT: 3072 H100 hours RLHF: 2048 H100 hours | 700 | 513 | 0 |
| Total | 2.02M | | 584 | 0 |
The methodology used to determine training energy use and greenhouse gas emissions can be found [here](https://arxiv.org/pdf/2204.05149). Since Meta is openly releasing these models, the training energy use and greenhouse gas emissions will not be incurred by others.
## Training Data
**Overview:** Llama 3.2-Vision was pretrained on 6B image and text pairs. The instruction tuning data includes publicly available vision instruction datasets, as well as over 3M synthetically generated examples.
**Data Freshness:** The pretraining data has a cutoff of December 2023\.
## Benchmarks \- Image Reasoning
In this section, we report the results for Llama 3.2-Vision models on standard automatic benchmarks. For all these evaluations, we used our internal evaluations library.
### Base Pretrained Models
| Category | Benchmark | \# Shots | Metric | Llama 3.2 11B | Llama 3.2 90B |
| ----- | ----- | ----- | ----- | ----- | ----- |
| Image Understanding | VQAv2 (val) | 0 | Accuracy | 66.8 | 73.6 |
| | Text VQA (val) | 0 | Relaxed accuracy | 73.1 | 73.5 |
| | DocVQA (val, unseen) | 0 | ANLS | 62.3 | 70.7 |
| Visual Reasoning | MMMU (val, 0-shot) | 0 | Micro average accuracy | 41.7 | 49.3 |
| | ChartQA (test) | 0 | Accuracy | 39.4 | 54.2 |
| | InfographicsQA (val, unseen) | 0 | ANLS | 43.2 | 56.8 |
| | AI2 Diagram (test) | 0 | Accuracy | 62.4 | 75.3 |
### Instruction Tuned Models
| Modality | Capability | Benchmark | \# Shots | Metric | Llama 3.2 11B | Llama 3.2 90B |
| ----- | :---: | ----- | :---: | :---: | ----- | ----- |
| Image | College-level Problems and Mathematical Reasoning | MMMU (val, CoT) | 0 | Micro average accuracy | 50.7 | 60.3 |
| | | MMMU-Pro, Standard (10 opts, test) | 0 | Accuracy | 33.0 | 45.2 |
| | | MMMU-Pro, Vision (test) | 0 | Accuracy | 23.7 | 33.8 |
| | | MathVista (testmini) | 0 | Accuracy | 51.5 | 57.3 |
| | Charts and Diagram Understanding | ChartQA (test, CoT) | 0 | Relaxed accuracy | 83.4 | 85.5 |
| | | AI2 Diagram (test) | 0 | Accuracy | 91.1 | 92.3 |
| | | DocVQA (test) | 0 | ANLS | 88.4 | 90.1 |
| | General Visual Question Answering | VQAv2 (test) | 0 | Accuracy | 75.2 | 78.1 |
| | | | | | | |
| Text | General | MMLU (CoT) | 0 | Macro\_avg/acc | 73.0 | 86.0 |
| | Math | MATH (CoT) | 0 | Final\_em | 51.9 | 68.0 |
| | Reasoning | GPQA | 0 | Accuracy | 32.8 | 46.7 |
| | Multilingual | MGSM (CoT) | 0 | em | 68.9 | 86.9 |
## Responsibility & Safety
As part of our Responsible release approach, we followed a three-pronged strategy to managing trust & safety risks:
1. Enable developers to deploy helpful, safe and flexible experiences for their target audience and for the use cases supported by Llama.
2. Protect developers against adversarial users aiming to exploit Llama capabilities to potentially cause harm.
3. Provide protections for the community to help prevent the misuse of our models.
### Responsible Deployment
**Approach:** Llama is a foundational technology designed to be used in a variety of use cases, examples on how Meta’s Llama models have been responsibly deployed can be found in our [Community Stories webpage](https://llama.meta.com/community-stories/). Our approach is to build the most helpful models enabling the world to benefit from the technology power, by aligning our model safety for the generic use cases addressing a standard set of harms. Developers are then in the driver seat to tailor safety for their use case, defining their own policy and deploying the models with the necessary safeguards in their Llama systems. Llama 3.2 was developed following the best practices outlined in our Responsible Use Guide, you can refer to the [Responsible Use Guide](https://llama.meta.com/responsible-use-guide/) to learn more.
#### Llama 3.2 Instruct
**Objective:** Our main objectives for conducting safety fine-tuning are to provide the research community with a valuable resource for studying the robustness of safety fine-tuning, as well as to offer developers a readily available, safe, and powerful model for various applications to reduce the developer workload to deploy safe AI systems. We implemented the same set of safety mitigations as in Llama 3, and you can learn more about these in the Llama 3 [paper](https://ai.meta.com/research/publications/the-llama-3-herd-of-models/).
**Fine-Tuning Data:** We employ a multi-faceted approach to data collection, combining human-generated data from our vendors with synthetic data to mitigate potential safety risks. We’ve developed many large language model (LLM)-based classifiers that enable us to thoughtfully select high-quality prompts and responses, enhancing data quality control.
**Refusals and Tone:** Building on the work we started with Llama 3, we put a great emphasis on model refusals to benign prompts as well as refusal tone. We included both borderline and adversarial prompts in our safety data strategy, and modified our safety data responses to follow tone guidelines.
#### Llama 3.2 Systems
**Safety as a System:** Large language models, including Llama 3.2, **are not designed to be deployed in isolation** but instead should be deployed as part of an overall AI system with additional safety guardrails as required. Developers are expected to deploy system safeguards when building agentic systems. Safeguards are key to achieve the right helpfulness-safety alignment as well as mitigating safety and security risks inherent to the system and any integration of the model or system with external tools. As part of our responsible release approach, we provide the community with [safeguards](https://llama.meta.com/trust-and-safety/) that developers should deploy with Llama models or other LLMs, including Llama Guard, Prompt Guard and Code Shield. All our [reference implementations](https://github.com/meta-llama/llama-agentic-system) demos contain these safeguards by default so developers can benefit from system-level safety out-of-the-box.
### New Capabilities and Use Cases
**Technological Advancement:** Llama releases usually introduce new capabilities that require specific considerations in addition to the best practices that generally apply across all Generative AI use cases. For prior release capabilities also supported by Llama 3.2, see [Llama 3.1 Model Card](https://github.com/meta-llama/llama-models/blob/main/models/llama3_1/MODEL_CARD.md), as the same considerations apply here as well.,
**Image Reasoning:** Llama 3.2-Vision models come with multimodal (text and image) input capabilities enabling image reasoning applications. As part of our responsible release process, we took dedicated measures including evaluations and mitigations to address the risk of the models uniquely identifying individuals in images. As with other LLM risks, models may not always be robust to adversarial prompts, and developers should evaluate identification and other applicable risks in the context of their applications as well as consider deploying Llama Guard 3-11B-Vision as part of their system or other mitigations as appropriate to detect and mitigate such risks.
### Evaluations
**Scaled Evaluations:** We built dedicated, adversarial evaluation datasets and evaluated systems composed of Llama models and Purple Llama safeguards to filter input prompt and output response. It is important to evaluate applications in context, and we recommend building dedicated evaluation dataset for your use case.
**Red teaming:** We conducted recurring red teaming exercises with the goal of discovering risks via adversarial prompting and we used the learnings to improve our benchmarks and safety tuning datasets. We partnered early with subject-matter experts in critical risk areas to understand the nature of these real-world harms and how such models may lead to unintended harm for society. Based on these conversations, we derived a set of adversarial goals for the red team to attempt to achieve, such as extracting harmful information or reprogramming the model to act in a potentially harmful capacity. The red team consisted of experts in cybersecurity, adversarial machine learning, responsible AI, and integrity in addition to multilingual content specialists with background in integrity issues in specific geographic markets.
### Critical Risks
In addition to our safety work above, we took extra care on measuring and/or mitigating the following critical risk areas:
**1\. CBRNE (Chemical, Biological, Radiological, Nuclear, and Explosive Weapons):** For Llama 3.1, to assess risks related to proliferation of chemical and biological weapons, we performed uplift testing designed to assess whether use of Llama 3.1 models could meaningfully increase the capabilities of malicious actors to plan or carry out attacks using these types of weapons. For Llama 3.2-Vision models, we conducted additional targeted evaluations and found that it was unlikely Llama 3.2 presented an increase in scientific capabilities due to its added image understanding capability as compared to Llama 3.1.
**2\. Child Safety:** Child Safety risk assessments were conducted using a team of experts, to assess the model’s capability to produce outputs that could result in Child Safety risks and inform on any necessary and appropriate risk mitigations via fine tuning. We leveraged those expert red teaming sessions to expand the coverage of our evaluation benchmarks through Llama 3 model development. For Llama 3, we conducted new in-depth sessions using objective based methodologies to assess the model risks along multiple attack vectors including the additional languages Llama 3 is trained on. We also partnered with content specialists to perform red teaming exercises assessing potentially violating content while taking account of market specific nuances or experiences.
**3\. Cyber Attacks:** For Llama 3.1 405B, our cyber attack uplift study investigated whether LLMs can enhance human capabilities in hacking tasks, both in terms of skill level and speed.
Our attack automation study focused on evaluating the capabilities of LLMs when used as autonomous agents in cyber offensive operations, specifically in the context of ransomware attacks. This evaluation was distinct from previous studies that considered LLMs as interactive assistants. The primary objective was to assess whether these models could effectively function as independent agents in executing complex cyber-attacks without human intervention. Because Llama 3.2’s vision capabilities are not generally germane to cyber uplift, we believe that the testing conducted for Llama 3.1 also applies to Llama 3.2.
### Community
**Industry Partnerships:** Generative AI safety requires expertise and tooling, and we believe in the strength of the open community to accelerate its progress. We are active members of open consortiums, including the AI Alliance, Partnership on AI and MLCommons, actively contributing to safety standardization and transparency. We encourage the community to adopt taxonomies like the MLCommons Proof of Concept evaluation to facilitate collaboration and transparency on safety and content evaluations. Our Purple Llama tools are open sourced for the community to use and widely distributed across ecosystem partners including cloud service providers. We encourage community contributions to our [Github repository](https://github.com/meta-llama/PurpleLlama).
**Grants:** We also set up the [Llama Impact Grants](https://llama.meta.com/llama-impact-grants/) program to identify and support the most compelling applications of Meta’s Llama model for societal benefit across three categories: education, climate and open innovation. The 20 finalists from the hundreds of applications can be found [here](https://llama.meta.com/llama-impact-grants/#finalists).
**Reporting:** Finally, we put in place a set of resources including an [output reporting mechanism](https://developers.facebook.com/llama_output_feedback) and [bug bounty program](https://www.facebook.com/whitehat) to continuously improve the Llama technology with the help of the community.
## Ethical Considerations and Limitations
**Values:** The core values of Llama 3.2 are openness, inclusivity and helpfulness. It is meant to serve everyone, and to work for a wide range of use cases. It is thus designed to be accessible to people across many different backgrounds, experiences and perspectives. Llama 3.2 addresses users and their needs as they are, without insertion unnecessary judgment or normativity, while reflecting the understanding that even content that may appear problematic in some cases can serve valuable purposes in others. It respects the dignity and autonomy of all users, especially in terms of the values of free thought and expression that power innovation and progress.
**Testing:** But Llama 3.2 is a new technology, and like any new technology, there are risks associated with its use. Testing conducted to date has not covered, nor could it cover, all scenarios. For these reasons, as with all LLMs, Llama 3.2’s potential outputs cannot be predicted in advance, and the model may in some instances produce inaccurate, biased or other objectionable responses to user prompts. Therefore, before deploying any applications of Llama 3.2 models, developers should perform safety testing and tuning tailored to their specific applications of the model. Please refer to available resources including our [Responsible Use Guide](https://llama.meta.com/responsible-use-guide), [Trust and Safety](https://llama.meta.com/trust-and-safety/) solutions, and other [resources](https://llama.meta.com/docs/get-started/) to learn more about responsible development.
|
tohoku-nlp/bert-base-japanese | tohoku-nlp | "2024-02-22T00:57:00Z" | 2,355,899 | 34 | transformers | [
"transformers",
"pytorch",
"tf",
"jax",
"bert",
"fill-mask",
"ja",
"dataset:wikipedia",
"license:cc-by-sa-4.0",
"autotrain_compatible",
"endpoints_compatible",
"region:us"
] | fill-mask | "2022-03-02T23:29:05Z" | ---
language: ja
license: cc-by-sa-4.0
datasets:
- wikipedia
widget:
- text: 東北大学で[MASK]の研究をしています。
---
# BERT base Japanese (IPA dictionary)
This is a [BERT](https://github.com/google-research/bert) model pretrained on texts in the Japanese language.
This version of the model processes input texts with word-level tokenization based on the IPA dictionary, followed by the WordPiece subword tokenization.
The codes for the pretraining are available at [cl-tohoku/bert-japanese](https://github.com/cl-tohoku/bert-japanese/tree/v1.0).
## Model architecture
The model architecture is the same as the original BERT base model; 12 layers, 768 dimensions of hidden states, and 12 attention heads.
## Training Data
The model is trained on Japanese Wikipedia as of September 1, 2019.
To generate the training corpus, [WikiExtractor](https://github.com/attardi/wikiextractor) is used to extract plain texts from a dump file of Wikipedia articles.
The text files used for the training are 2.6GB in size, consisting of approximately 17M sentences.
## Tokenization
The texts are first tokenized by [MeCab](https://taku910.github.io/mecab/) morphological parser with the IPA dictionary and then split into subwords by the WordPiece algorithm.
The vocabulary size is 32000.
## Training
The model is trained with the same configuration as the original BERT; 512 tokens per instance, 256 instances per batch, and 1M training steps.
## Licenses
The pretrained models are distributed under the terms of the [Creative Commons Attribution-ShareAlike 3.0](https://creativecommons.org/licenses/by-sa/3.0/).
## Acknowledgments
For training models, we used Cloud TPUs provided by [TensorFlow Research Cloud](https://www.tensorflow.org/tfrc/) program.
|
microsoft/table-transformer-detection | microsoft | "2023-09-06T14:49:09Z" | 2,353,122 | 300 | transformers | [
"transformers",
"pytorch",
"safetensors",
"table-transformer",
"object-detection",
"arxiv:2110.00061",
"license:mit",
"endpoints_compatible",
"region:us"
] | object-detection | "2022-10-14T09:14:13Z" | ---
license: mit
widget:
- src: https://www.invoicesimple.com/wp-content/uploads/2018/06/Sample-Invoice-printable.png
example_title: Invoice
---
# Table Transformer (fine-tuned for Table Detection)
Table Transformer (DETR) model trained on PubTables1M. It was introduced in the paper [PubTables-1M: Towards Comprehensive Table Extraction From Unstructured Documents](https://arxiv.org/abs/2110.00061) by Smock et al. and first released in [this repository](https://github.com/microsoft/table-transformer).
Disclaimer: The team releasing Table Transformer did not write a model card for this model so this model card has been written by the Hugging Face team.
## Model description
The Table Transformer is equivalent to [DETR](https://huggingface.co/docs/transformers/model_doc/detr), a Transformer-based object detection model. Note that the authors decided to use the "normalize before" setting of DETR, which means that layernorm is applied before self- and cross-attention.
## Usage
You can use the raw model for detecting tables in documents. See the [documentation](https://huggingface.co/docs/transformers/main/en/model_doc/table-transformer) for more info. |
jhgan/ko-sroberta-multitask | jhgan | "2022-06-13T16:34:48Z" | 2,337,590 | 95 | sentence-transformers | [
"sentence-transformers",
"pytorch",
"tf",
"roberta",
"feature-extraction",
"sentence-similarity",
"transformers",
"ko",
"autotrain_compatible",
"text-embeddings-inference",
"endpoints_compatible",
"region:us"
] | sentence-similarity | "2022-03-02T23:29:05Z" | ---
pipeline_tag: sentence-similarity
tags:
- sentence-transformers
- feature-extraction
- sentence-similarity
- transformers
language: ko
---
# ko-sroberta-multitask
This is a [sentence-transformers](https://www.SBERT.net) model: It maps sentences & paragraphs to a 768 dimensional dense vector space and can be used for tasks like clustering or semantic search.
<!--- Describe your model here -->
## Usage (Sentence-Transformers)
Using this model becomes easy when you have [sentence-transformers](https://www.SBERT.net) installed:
```
pip install -U sentence-transformers
```
Then you can use the model like this:
```python
from sentence_transformers import SentenceTransformer
sentences = ["안녕하세요?", "한국어 문장 임베딩을 위한 버트 모델입니다."]
model = SentenceTransformer('jhgan/ko-sroberta-multitask')
embeddings = model.encode(sentences)
print(embeddings)
```
## Usage (HuggingFace Transformers)
Without [sentence-transformers](https://www.SBERT.net), you can use the model like this: First, you pass your input through the transformer model, then you have to apply the right pooling-operation on-top of the contextualized word embeddings.
```python
from transformers import AutoTokenizer, AutoModel
import torch
#Mean Pooling - Take attention mask into account for correct averaging
def mean_pooling(model_output, attention_mask):
token_embeddings = model_output[0] #First element of model_output contains all token embeddings
input_mask_expanded = attention_mask.unsqueeze(-1).expand(token_embeddings.size()).float()
return torch.sum(token_embeddings * input_mask_expanded, 1) / torch.clamp(input_mask_expanded.sum(1), min=1e-9)
# Sentences we want sentence embeddings for
sentences = ['This is an example sentence', 'Each sentence is converted']
# Load model from HuggingFace Hub
tokenizer = AutoTokenizer.from_pretrained('jhgan/ko-sroberta-multitask')
model = AutoModel.from_pretrained('jhgan/ko-sroberta-multitask')
# Tokenize sentences
encoded_input = tokenizer(sentences, padding=True, truncation=True, return_tensors='pt')
# Compute token embeddings
with torch.no_grad():
model_output = model(**encoded_input)
# Perform pooling. In this case, mean pooling.
sentence_embeddings = mean_pooling(model_output, encoded_input['attention_mask'])
print("Sentence embeddings:")
print(sentence_embeddings)
```
## Evaluation Results
<!--- Describe how your model was evaluated -->
KorSTS, KorNLI 학습 데이터셋으로 멀티 태스크 학습을 진행한 후 KorSTS 평가 데이터셋으로 평가한 결과입니다.
- Cosine Pearson: 84.77
- Cosine Spearman: 85.60
- Euclidean Pearson: 83.71
- Euclidean Spearman: 84.40
- Manhattan Pearson: 83.70
- Manhattan Spearman: 84.38
- Dot Pearson: 82.42
- Dot Spearman: 82.33
## Training
The model was trained with the parameters:
**DataLoader**:
`sentence_transformers.datasets.NoDuplicatesDataLoader.NoDuplicatesDataLoader` of length 8885 with parameters:
```
{'batch_size': 64}
```
**Loss**:
`sentence_transformers.losses.MultipleNegativesRankingLoss.MultipleNegativesRankingLoss` with parameters:
```
{'scale': 20.0, 'similarity_fct': 'cos_sim'}
```
**DataLoader**:
`torch.utils.data.dataloader.DataLoader` of length 719 with parameters:
```
{'batch_size': 8, 'sampler': 'torch.utils.data.sampler.RandomSampler', 'batch_sampler': 'torch.utils.data.sampler.BatchSampler'}
```
**Loss**:
`sentence_transformers.losses.CosineSimilarityLoss.CosineSimilarityLoss`
Parameters of the fit()-Method:
```
{
"epochs": 5,
"evaluation_steps": 1000,
"evaluator": "sentence_transformers.evaluation.EmbeddingSimilarityEvaluator.EmbeddingSimilarityEvaluator",
"max_grad_norm": 1,
"optimizer_class": "<class 'transformers.optimization.AdamW'>",
"optimizer_params": {
"lr": 2e-05
},
"scheduler": "WarmupLinear",
"steps_per_epoch": null,
"warmup_steps": 360,
"weight_decay": 0.01
}
```
## Full Model Architecture
```
SentenceTransformer(
(0): Transformer({'max_seq_length': 128, 'do_lower_case': False}) with Transformer model: RobertaModel
(1): Pooling({'word_embedding_dimension': 768, 'pooling_mode_cls_token': False, 'pooling_mode_mean_tokens': True, 'pooling_mode_max_tokens': False, 'pooling_mode_mean_sqrt_len_tokens': False})
)
```
## Citing & Authors
<!--- Describe where people can find more information -->
- Ham, J., Choe, Y. J., Park, K., Choi, I., & Soh, H. (2020). Kornli and korsts: New benchmark datasets for korean natural language understanding. arXiv
preprint arXiv:2004.03289
- Reimers, Nils and Iryna Gurevych. “Sentence-BERT: Sentence Embeddings using Siamese BERT-Networks.” ArXiv abs/1908.10084 (2019)
- Reimers, Nils and Iryna Gurevych. “Making Monolingual Sentence Embeddings Multilingual Using Knowledge Distillation.” EMNLP (2020).
|
FacebookAI/xlm-roberta-large-finetuned-conll03-english | FacebookAI | "2024-02-19T12:48:53Z" | 2,327,498 | 144 | transformers | [
"transformers",
"pytorch",
"rust",
"onnx",
"safetensors",
"xlm-roberta",
"token-classification",
"multilingual",
"af",
"am",
"ar",
"as",
"az",
"be",
"bg",
"bn",
"br",
"bs",
"ca",
"cs",
"cy",
"da",
"de",
"el",
"en",
"eo",
"es",
"et",
"eu",
"fa",
"fi",
"fr",
"fy",
"ga",
"gd",
"gl",
"gu",
"ha",
"he",
"hi",
"hr",
"hu",
"hy",
"id",
"is",
"it",
"ja",
"jv",
"ka",
"kk",
"km",
"kn",
"ko",
"ku",
"ky",
"la",
"lo",
"lt",
"lv",
"mg",
"mk",
"ml",
"mn",
"mr",
"ms",
"my",
"ne",
"nl",
"no",
"om",
"or",
"pa",
"pl",
"ps",
"pt",
"ro",
"ru",
"sa",
"sd",
"si",
"sk",
"sl",
"so",
"sq",
"sr",
"su",
"sv",
"sw",
"ta",
"te",
"th",
"tl",
"tr",
"ug",
"uk",
"ur",
"uz",
"vi",
"xh",
"yi",
"zh",
"arxiv:1911.02116",
"arxiv:2008.03415",
"arxiv:1910.09700",
"autotrain_compatible",
"endpoints_compatible",
"region:us"
] | token-classification | "2022-03-02T23:29:04Z" | ---
language:
- multilingual
- af
- am
- ar
- as
- az
- be
- bg
- bn
- br
- bs
- ca
- cs
- cy
- da
- de
- el
- en
- eo
- es
- et
- eu
- fa
- fi
- fr
- fy
- ga
- gd
- gl
- gu
- ha
- he
- hi
- hr
- hu
- hy
- id
- is
- it
- ja
- jv
- ka
- kk
- km
- kn
- ko
- ku
- ky
- la
- lo
- lt
- lv
- mg
- mk
- ml
- mn
- mr
- ms
- my
- ne
- nl
- no
- om
- or
- pa
- pl
- ps
- pt
- ro
- ru
- sa
- sd
- si
- sk
- sl
- so
- sq
- sr
- su
- sv
- sw
- ta
- te
- th
- tl
- tr
- ug
- uk
- ur
- uz
- vi
- xh
- yi
- zh
---
# xlm-roberta-large-finetuned-conll03-english
# Table of Contents
1. [Model Details](#model-details)
2. [Uses](#uses)
3. [Bias, Risks, and Limitations](#bias-risks-and-limitations)
4. [Training](#training)
5. [Evaluation](#evaluation)
6. [Environmental Impact](#environmental-impact)
7. [Technical Specifications](#technical-specifications)
8. [Citation](#citation)
9. [Model Card Authors](#model-card-authors)
10. [How To Get Started With the Model](#how-to-get-started-with-the-model)
# Model Details
## Model Description
The XLM-RoBERTa model was proposed in [Unsupervised Cross-lingual Representation Learning at Scale](https://arxiv.org/abs/1911.02116) by Alexis Conneau, Kartikay Khandelwal, Naman Goyal, Vishrav Chaudhary, Guillaume Wenzek, Francisco Guzmán, Edouard Grave, Myle Ott, Luke Zettlemoyer and Veselin Stoyanov. It is based on Facebook's RoBERTa model released in 2019. It is a large multi-lingual language model, trained on 2.5TB of filtered CommonCrawl data. This model is [XLM-RoBERTa-large](https://huggingface.co/xlm-roberta-large) fine-tuned with the [conll2003](https://huggingface.co/datasets/conll2003) dataset in English.
- **Developed by:** See [associated paper](https://arxiv.org/abs/1911.02116)
- **Model type:** Multi-lingual language model
- **Language(s) (NLP) or Countries (images):** XLM-RoBERTa is a multilingual model trained on 100 different languages; see [GitHub Repo](https://github.com/facebookresearch/fairseq/tree/main/examples/xlmr) for full list; model is fine-tuned on a dataset in English
- **License:** More information needed
- **Related Models:** [RoBERTa](https://huggingface.co/roberta-base), [XLM](https://huggingface.co/docs/transformers/model_doc/xlm)
- **Parent Model:** [XLM-RoBERTa-large](https://huggingface.co/xlm-roberta-large)
- **Resources for more information:**
-[GitHub Repo](https://github.com/facebookresearch/fairseq/tree/main/examples/xlmr)
-[Associated Paper](https://arxiv.org/abs/1911.02116)
# Uses
## Direct Use
The model is a language model. The model can be used for token classification, a natural language understanding task in which a label is assigned to some tokens in a text.
## Downstream Use
Potential downstream use cases include Named Entity Recognition (NER) and Part-of-Speech (PoS) tagging. To learn more about token classification and other potential downstream use cases, see the Hugging Face [token classification docs](https://huggingface.co/tasks/token-classification).
## Out-of-Scope Use
The model should not be used to intentionally create hostile or alienating environments for people.
# Bias, Risks, and Limitations
**CONTENT WARNING: Readers should be made aware that language generated by this model may be disturbing or offensive to some and may propagate historical and current stereotypes.**
Significant research has explored bias and fairness issues with language models (see, e.g., [Sheng et al. (2021)](https://aclanthology.org/2021.acl-long.330.pdf) and [Bender et al. (2021)](https://dl.acm.org/doi/pdf/10.1145/3442188.3445922)). In the context of tasks relevant to this model, [Mishra et al. (2020)](https://arxiv.org/pdf/2008.03415.pdf) explore social biases in NER systems for English and find that there is systematic bias in existing NER systems in that they fail to identify named entities from different demographic groups (though this paper did not look at BERT). For example, using a sample sentence from [Mishra et al. (2020)](https://arxiv.org/pdf/2008.03415.pdf):
```python
>>> from transformers import pipeline
>>> tokenizer = AutoTokenizer.from_pretrained("xlm-roberta-large-finetuned-conll03-english")
>>> model = AutoModelForTokenClassification.from_pretrained("xlm-roberta-large-finetuned-conll03-english")
>>> classifier = pipeline("ner", model=model, tokenizer=tokenizer)
>>> classifier("Alya told Jasmine that Andrew could pay with cash..")
[{'end': 2,
'entity': 'I-PER',
'index': 1,
'score': 0.9997861,
'start': 0,
'word': '▁Al'},
{'end': 4,
'entity': 'I-PER',
'index': 2,
'score': 0.9998591,
'start': 2,
'word': 'ya'},
{'end': 16,
'entity': 'I-PER',
'index': 4,
'score': 0.99995816,
'start': 10,
'word': '▁Jasmin'},
{'end': 17,
'entity': 'I-PER',
'index': 5,
'score': 0.9999584,
'start': 16,
'word': 'e'},
{'end': 29,
'entity': 'I-PER',
'index': 7,
'score': 0.99998057,
'start': 23,
'word': '▁Andrew'}]
```
## Recommendations
Users (both direct and downstream) should be made aware of the risks, biases and limitations of the model.
# Training
See the following resources for training data and training procedure details:
- [XLM-RoBERTa-large model card](https://huggingface.co/xlm-roberta-large)
- [CoNLL-2003 data card](https://huggingface.co/datasets/conll2003)
- [Associated paper](https://arxiv.org/pdf/1911.02116.pdf)
# Evaluation
See the [associated paper](https://arxiv.org/pdf/1911.02116.pdf) for evaluation details.
# Environmental Impact
Carbon emissions can be estimated using the [Machine Learning Impact calculator](https://mlco2.github.io/impact#compute) presented in [Lacoste et al. (2019)](https://arxiv.org/abs/1910.09700).
- **Hardware Type:** 500 32GB Nvidia V100 GPUs (from the [associated paper](https://arxiv.org/pdf/1911.02116.pdf))
- **Hours used:** More information needed
- **Cloud Provider:** More information needed
- **Compute Region:** More information needed
- **Carbon Emitted:** More information needed
# Technical Specifications
See the [associated paper](https://arxiv.org/pdf/1911.02116.pdf) for further details.
# Citation
**BibTeX:**
```bibtex
@article{conneau2019unsupervised,
title={Unsupervised Cross-lingual Representation Learning at Scale},
author={Conneau, Alexis and Khandelwal, Kartikay and Goyal, Naman and Chaudhary, Vishrav and Wenzek, Guillaume and Guzm{\'a}n, Francisco and Grave, Edouard and Ott, Myle and Zettlemoyer, Luke and Stoyanov, Veselin},
journal={arXiv preprint arXiv:1911.02116},
year={2019}
}
```
**APA:**
- Conneau, A., Khandelwal, K., Goyal, N., Chaudhary, V., Wenzek, G., Guzmán, F., ... & Stoyanov, V. (2019). Unsupervised cross-lingual representation learning at scale. arXiv preprint arXiv:1911.02116.
# Model Card Authors
This model card was written by the team at Hugging Face.
# How to Get Started with the Model
Use the code below to get started with the model. You can use this model directly within a pipeline for NER.
<details>
<summary> Click to expand </summary>
```python
>>> from transformers import AutoTokenizer, AutoModelForTokenClassification
>>> from transformers import pipeline
>>> tokenizer = AutoTokenizer.from_pretrained("xlm-roberta-large-finetuned-conll03-english")
>>> model = AutoModelForTokenClassification.from_pretrained("xlm-roberta-large-finetuned-conll03-english")
>>> classifier = pipeline("ner", model=model, tokenizer=tokenizer)
>>> classifier("Hello I'm Omar and I live in Zürich.")
[{'end': 14,
'entity': 'I-PER',
'index': 5,
'score': 0.9999175,
'start': 10,
'word': '▁Omar'},
{'end': 35,
'entity': 'I-LOC',
'index': 10,
'score': 0.9999906,
'start': 29,
'word': '▁Zürich'}]
```
</details> |
nlpconnect/vit-gpt2-image-captioning | nlpconnect | "2023-02-27T15:00:09Z" | 2,291,428 | 831 | transformers | [
"transformers",
"pytorch",
"vision-encoder-decoder",
"image-text-to-text",
"image-to-text",
"image-captioning",
"doi:10.57967/hf/0222",
"license:apache-2.0",
"endpoints_compatible",
"region:us"
] | image-to-text | "2022-03-02T23:29:05Z" | ---
tags:
- image-to-text
- image-captioning
license: apache-2.0
widget:
- src: https://huggingface.co/datasets/mishig/sample_images/resolve/main/savanna.jpg
example_title: Savanna
- src: https://huggingface.co/datasets/mishig/sample_images/resolve/main/football-match.jpg
example_title: Football Match
- src: https://huggingface.co/datasets/mishig/sample_images/resolve/main/airport.jpg
example_title: Airport
---
# nlpconnect/vit-gpt2-image-captioning
This is an image captioning model trained by @ydshieh in [flax ](https://github.com/huggingface/transformers/tree/main/examples/flax/image-captioning) this is pytorch version of [this](https://huggingface.co/ydshieh/vit-gpt2-coco-en-ckpts).
# The Illustrated Image Captioning using transformers
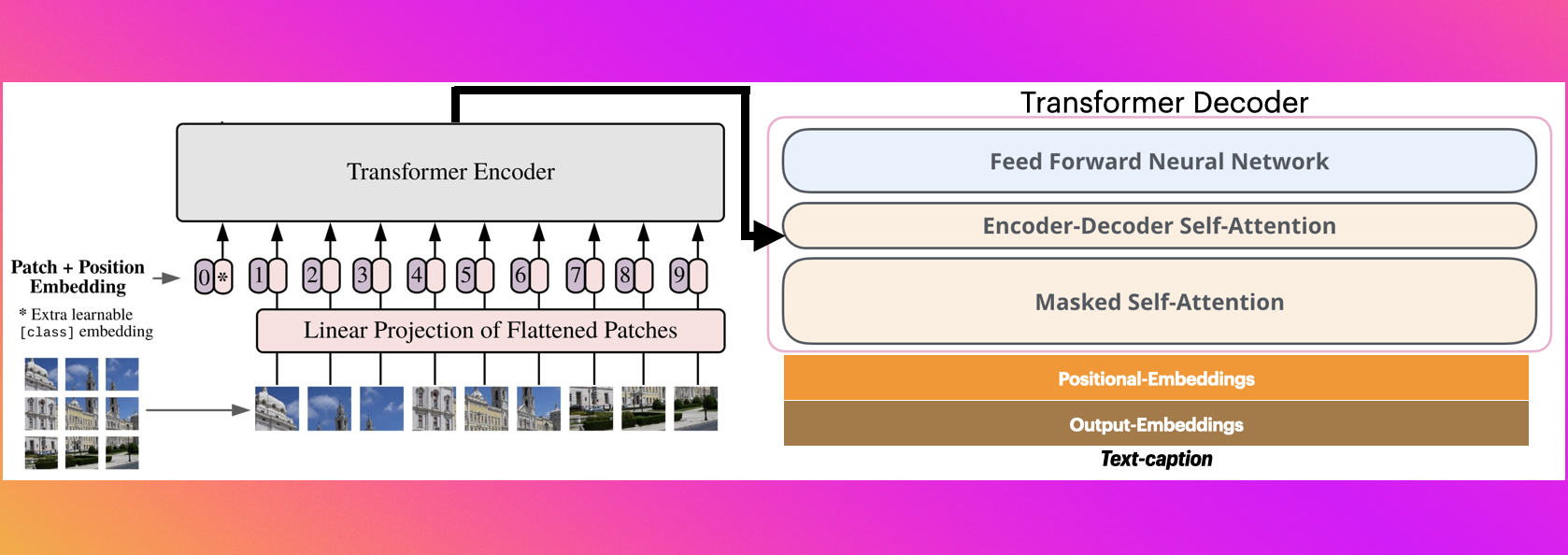
* https://ankur3107.github.io/blogs/the-illustrated-image-captioning-using-transformers/
# Sample running code
```python
from transformers import VisionEncoderDecoderModel, ViTImageProcessor, AutoTokenizer
import torch
from PIL import Image
model = VisionEncoderDecoderModel.from_pretrained("nlpconnect/vit-gpt2-image-captioning")
feature_extractor = ViTImageProcessor.from_pretrained("nlpconnect/vit-gpt2-image-captioning")
tokenizer = AutoTokenizer.from_pretrained("nlpconnect/vit-gpt2-image-captioning")
device = torch.device("cuda" if torch.cuda.is_available() else "cpu")
model.to(device)
max_length = 16
num_beams = 4
gen_kwargs = {"max_length": max_length, "num_beams": num_beams}
def predict_step(image_paths):
images = []
for image_path in image_paths:
i_image = Image.open(image_path)
if i_image.mode != "RGB":
i_image = i_image.convert(mode="RGB")
images.append(i_image)
pixel_values = feature_extractor(images=images, return_tensors="pt").pixel_values
pixel_values = pixel_values.to(device)
output_ids = model.generate(pixel_values, **gen_kwargs)
preds = tokenizer.batch_decode(output_ids, skip_special_tokens=True)
preds = [pred.strip() for pred in preds]
return preds
predict_step(['doctor.e16ba4e4.jpg']) # ['a woman in a hospital bed with a woman in a hospital bed']
```
# Sample running code using transformers pipeline
```python
from transformers import pipeline
image_to_text = pipeline("image-to-text", model="nlpconnect/vit-gpt2-image-captioning")
image_to_text("https://ankur3107.github.io/assets/images/image-captioning-example.png")
# [{'generated_text': 'a soccer game with a player jumping to catch the ball '}]
```
# Contact for any help
* https://huggingface.co/ankur310794
* https://twitter.com/ankur310794
* http://github.com/ankur3107
* https://www.linkedin.com/in/ankur310794 |
cardiffnlp/twitter-xlm-roberta-base-sentiment-multilingual | cardiffnlp | "2024-03-24T06:10:17Z" | 2,288,262 | 19 | transformers | [
"transformers",
"pytorch",
"xlm-roberta",
"text-classification",
"dataset:cardiffnlp/tweet_sentiment_multilingual",
"model-index",
"autotrain_compatible",
"endpoints_compatible",
"region:us"
] | text-classification | "2022-12-01T00:32:11Z" | ---
datasets:
- cardiffnlp/tweet_sentiment_multilingual
metrics:
- f1
- accuracy
model-index:
- name: cardiffnlp/twitter-xlm-roberta-base-sentiment-multilingual
results:
- task:
type: text-classification
name: Text Classification
dataset:
name: cardiffnlp/tweet_sentiment_multilingual
type: all
split: test
metrics:
- name: Micro F1 (cardiffnlp/tweet_sentiment_multilingual/all)
type: micro_f1_cardiffnlp/tweet_sentiment_multilingual/all
value: 0.6931034482758621
- name: Macro F1 (cardiffnlp/tweet_sentiment_multilingual/all)
type: micro_f1_cardiffnlp/tweet_sentiment_multilingual/all
value: 0.692628774202147
- name: Accuracy (cardiffnlp/tweet_sentiment_multilingual/all)
type: accuracy_cardiffnlp/tweet_sentiment_multilingual/all
value: 0.6931034482758621
pipeline_tag: text-classification
widget:
- text: Get the all-analog Classic Vinyl Edition of "Takin Off" Album from {@herbiehancock@} via {@bluenoterecords@} link below {{URL}}
example_title: "topic_classification 1"
- text: Yes, including Medicare and social security saving👍
example_title: "sentiment 1"
- text: All two of them taste like ass.
example_title: "offensive 1"
- text: If you wanna look like a badass, have drama on social media
example_title: "irony 1"
- text: Whoever just unfollowed me you a bitch
example_title: "hate 1"
- text: I love swimming for the same reason I love meditating...the feeling of weightlessness.
example_title: "emotion 1"
- text: Beautiful sunset last night from the pontoon @TupperLakeNY
example_title: "emoji 1"
---
# cardiffnlp/twitter-xlm-roberta-base-sentiment-multilingual
This model is a fine-tuned version of [cardiffnlp/twitter-xlm-roberta-base](https://huggingface.co/cardiffnlp/twitter-xlm-roberta-base) on the
[`cardiffnlp/tweet_sentiment_multilingual (all)`](https://huggingface.co/datasets/cardiffnlp/tweet_sentiment_multilingual)
via [`tweetnlp`](https://github.com/cardiffnlp/tweetnlp).
Training split is `train` and parameters have been tuned on the validation split `validation`.
Following metrics are achieved on the test split `test` ([link](https://huggingface.co/cardiffnlp/twitter-xlm-roberta-base-sentiment-multilingual/raw/main/metric.json)).
- F1 (micro): 0.6931034482758621
- F1 (macro): 0.692628774202147
- Accuracy: 0.6931034482758621
### Usage
Install tweetnlp via pip.
```shell
pip install tweetnlp
```
Load the model in python.
```python
import tweetnlp
model = tweetnlp.Classifier("cardiffnlp/twitter-xlm-roberta-base-sentiment-multilingual", max_length=128)
model.predict('Get the all-analog Classic Vinyl Edition of "Takin Off" Album from {@herbiehancock@} via {@bluenoterecords@} link below {{URL}}')
```
### Reference
```
@inproceedings{camacho-collados-etal-2022-tweetnlp,
title = "{T}weet{NLP}: Cutting-Edge Natural Language Processing for Social Media",
author = "Camacho-collados, Jose and
Rezaee, Kiamehr and
Riahi, Talayeh and
Ushio, Asahi and
Loureiro, Daniel and
Antypas, Dimosthenis and
Boisson, Joanne and
Espinosa Anke, Luis and
Liu, Fangyu and
Mart{\'\i}nez C{\'a}mara, Eugenio" and others,
booktitle = "Proceedings of the 2022 Conference on Empirical Methods in Natural Language Processing: System Demonstrations",
month = dec,
year = "2022",
address = "Abu Dhabi, UAE",
publisher = "Association for Computational Linguistics",
url = "https://aclanthology.org/2022.emnlp-demos.5",
pages = "38--49"
}
```
|
papluca/xlm-roberta-base-language-detection | papluca | "2023-12-28T13:54:18Z" | 2,253,942 | 279 | transformers | [
"transformers",
"pytorch",
"tf",
"safetensors",
"xlm-roberta",
"text-classification",
"generated_from_trainer",
"multilingual",
"ar",
"bg",
"de",
"el",
"en",
"es",
"fr",
"hi",
"it",
"ja",
"nl",
"pl",
"pt",
"ru",
"sw",
"th",
"tr",
"ur",
"vi",
"zh",
"dataset:papluca/language-identification",
"arxiv:1911.02116",
"base_model:FacebookAI/xlm-roberta-base",
"base_model:finetune:FacebookAI/xlm-roberta-base",
"doi:10.57967/hf/2064",
"license:mit",
"autotrain_compatible",
"endpoints_compatible",
"region:us"
] | text-classification | "2022-03-02T23:29:05Z" | ---
language:
- multilingual
- ar
- bg
- de
- el
- en
- es
- fr
- hi
- it
- ja
- nl
- pl
- pt
- ru
- sw
- th
- tr
- ur
- vi
- zh
license: mit
tags:
- generated_from_trainer
datasets: papluca/language-identification
metrics:
- accuracy
- f1
base_model: xlm-roberta-base
model-index:
- name: xlm-roberta-base-language-detection
results: []
---
# xlm-roberta-base-language-detection
This model is a fine-tuned version of [xlm-roberta-base](https://huggingface.co/xlm-roberta-base) on the [Language Identification](https://huggingface.co/datasets/papluca/language-identification#additional-information) dataset.
## Model description
This model is an XLM-RoBERTa transformer model with a classification head on top (i.e. a linear layer on top of the pooled output).
For additional information please refer to the [xlm-roberta-base](https://huggingface.co/xlm-roberta-base) model card or to the paper [Unsupervised Cross-lingual Representation Learning at Scale](https://arxiv.org/abs/1911.02116) by Conneau et al.
## Intended uses & limitations
You can directly use this model as a language detector, i.e. for sequence classification tasks. Currently, it supports the following 20 languages:
`arabic (ar), bulgarian (bg), german (de), modern greek (el), english (en), spanish (es), french (fr), hindi (hi), italian (it), japanese (ja), dutch (nl), polish (pl), portuguese (pt), russian (ru), swahili (sw), thai (th), turkish (tr), urdu (ur), vietnamese (vi), and chinese (zh)`
## Training and evaluation data
The model was fine-tuned on the [Language Identification](https://huggingface.co/datasets/papluca/language-identification#additional-information) dataset, which consists of text sequences in 20 languages. The training set contains 70k samples, while the validation and test sets 10k each. The average accuracy on the test set is **99.6%** (this matches the average macro/weighted F1-score being the test set perfectly balanced). A more detailed evaluation is provided by the following table.
| Language | Precision | Recall | F1-score | support |
|:--------:|:---------:|:------:|:--------:|:-------:|
|ar |0.998 |0.996 |0.997 |500 |
|bg |0.998 |0.964 |0.981 |500 |
|de |0.998 |0.996 |0.997 |500 |
|el |0.996 |1.000 |0.998 |500 |
|en |1.000 |1.000 |1.000 |500 |
|es |0.967 |1.000 |0.983 |500 |
|fr |1.000 |1.000 |1.000 |500 |
|hi |0.994 |0.992 |0.993 |500 |
|it |1.000 |0.992 |0.996 |500 |
|ja |0.996 |0.996 |0.996 |500 |
|nl |1.000 |1.000 |1.000 |500 |
|pl |1.000 |1.000 |1.000 |500 |
|pt |0.988 |1.000 |0.994 |500 |
|ru |1.000 |0.994 |0.997 |500 |
|sw |1.000 |1.000 |1.000 |500 |
|th |1.000 |0.998 |0.999 |500 |
|tr |0.994 |0.992 |0.993 |500 |
|ur |1.000 |1.000 |1.000 |500 |
|vi |0.992 |1.000 |0.996 |500 |
|zh |1.000 |1.000 |1.000 |500 |
### Benchmarks
As a baseline to compare `xlm-roberta-base-language-detection` against, we have used the Python [langid](https://github.com/saffsd/langid.py) library. Since it comes pre-trained on 97 languages, we have used its `.set_languages()` method to constrain the language set to our 20 languages. The average accuracy of langid on the test set is **98.5%**. More details are provided by the table below.
| Language | Precision | Recall | F1-score | support |
|:--------:|:---------:|:------:|:--------:|:-------:|
|ar |0.990 |0.970 |0.980 |500 |
|bg |0.998 |0.964 |0.981 |500 |
|de |0.992 |0.944 |0.967 |500 |
|el |1.000 |0.998 |0.999 |500 |
|en |1.000 |1.000 |1.000 |500 |
|es |1.000 |0.968 |0.984 |500 |
|fr |0.996 |1.000 |0.998 |500 |
|hi |0.949 |0.976 |0.963 |500 |
|it |0.990 |0.980 |0.985 |500 |
|ja |0.927 |0.988 |0.956 |500 |
|nl |0.980 |1.000 |0.990 |500 |
|pl |0.986 |0.996 |0.991 |500 |
|pt |0.950 |0.996 |0.973 |500 |
|ru |0.996 |0.974 |0.985 |500 |
|sw |1.000 |1.000 |1.000 |500 |
|th |1.000 |0.996 |0.998 |500 |
|tr |0.990 |0.968 |0.979 |500 |
|ur |0.998 |0.996 |0.997 |500 |
|vi |0.971 |0.990 |0.980 |500 |
|zh |1.000 |1.000 |1.000 |500 |
## How to get started with the model
The easiest way to use the model is via the high-level `pipeline` API:
```python
from transformers import pipeline
text = [
"Brevity is the soul of wit.",
"Amor, ch'a nullo amato amar perdona."
]
model_ckpt = "papluca/xlm-roberta-base-language-detection"
pipe = pipeline("text-classification", model=model_ckpt)
pipe(text, top_k=1, truncation=True)
```
Or one can proceed with the tokenizer and model separately:
```python
import torch
from transformers import AutoModelForSequenceClassification, AutoTokenizer
text = [
"Brevity is the soul of wit.",
"Amor, ch'a nullo amato amar perdona."
]
model_ckpt = "papluca/xlm-roberta-base-language-detection"
tokenizer = AutoTokenizer.from_pretrained(model_ckpt)
model = AutoModelForSequenceClassification.from_pretrained(model_ckpt)
inputs = tokenizer(text, padding=True, truncation=True, return_tensors="pt")
with torch.no_grad():
logits = model(**inputs).logits
preds = torch.softmax(logits, dim=-1)
# Map raw predictions to languages
id2lang = model.config.id2label
vals, idxs = torch.max(preds, dim=1)
{id2lang[k.item()]: v.item() for k, v in zip(idxs, vals)}
```
## Training procedure
Fine-tuning was done via the `Trainer` API. Here is the [Colab notebook](https://colab.research.google.com/drive/15LJTckS6gU3RQOmjLqxVNBmbsBdnUEvl?usp=sharing) with the training code.
### Training hyperparameters
The following hyperparameters were used during training:
- learning_rate: 2e-05
- train_batch_size: 64
- eval_batch_size: 128
- seed: 42
- optimizer: Adam with betas=(0.9,0.999) and epsilon=1e-08
- lr_scheduler_type: linear
- num_epochs: 2
- mixed_precision_training: Native AMP
### Training results
The validation results on the `valid` split of the Language Identification dataset are summarised here below.
| Training Loss | Epoch | Step | Validation Loss | Accuracy | F1 |
|:-------------:|:-----:|:----:|:---------------:|:--------:|:------:|
| 0.2492 | 1.0 | 1094 | 0.0149 | 0.9969 | 0.9969 |
| 0.0101 | 2.0 | 2188 | 0.0103 | 0.9977 | 0.9977 |
In short, it achieves the following results on the validation set:
- Loss: 0.0101
- Accuracy: 0.9977
- F1: 0.9977
### Framework versions
- Transformers 4.12.5
- Pytorch 1.10.0+cu111
- Datasets 1.15.1
- Tokenizers 0.10.3
|
MIT/ast-finetuned-audioset-10-10-0.4593 | MIT | "2023-09-06T14:49:15Z" | 2,206,869 | 276 | transformers | [
"transformers",
"pytorch",
"safetensors",
"audio-spectrogram-transformer",
"audio-classification",
"arxiv:2104.01778",
"license:bsd-3-clause",
"endpoints_compatible",
"region:us"
] | audio-classification | "2022-11-14T18:41:48Z" | ---
license: bsd-3-clause
tags:
- audio-classification
---
# Audio Spectrogram Transformer (fine-tuned on AudioSet)
Audio Spectrogram Transformer (AST) model fine-tuned on AudioSet. It was introduced in the paper [AST: Audio Spectrogram Transformer](https://arxiv.org/abs/2104.01778) by Gong et al. and first released in [this repository](https://github.com/YuanGongND/ast).
Disclaimer: The team releasing Audio Spectrogram Transformer did not write a model card for this model so this model card has been written by the Hugging Face team.
## Model description
The Audio Spectrogram Transformer is equivalent to [ViT](https://huggingface.co/docs/transformers/model_doc/vit), but applied on audio. Audio is first turned into an image (as a spectrogram), after which a Vision Transformer is applied. The model gets state-of-the-art results on several audio classification benchmarks.
## Usage
You can use the raw model for classifying audio into one of the AudioSet classes. See the [documentation](https://huggingface.co/docs/transformers/main/en/model_doc/audio-spectrogram-transformer#transformers.ASTForAudioClassification.forward.example) for more info. |
lengyue233/content-vec-best | lengyue233 | "2023-03-31T08:02:09Z" | 2,201,437 | 15 | transformers | [
"transformers",
"pytorch",
"hubert",
"doi:10.57967/hf/0479",
"license:mit",
"endpoints_compatible",
"region:us"
] | null | "2023-03-25T04:33:59Z" | ---
license: mit
---
# Content Vec Best
Official Repo: [ContentVec](https://github.com/auspicious3000/contentvec)
This repo brings fairseq ContentVec model to HuggingFace Transformers.
## How to use
To use this model, you need to define
```python
class HubertModelWithFinalProj(HubertModel):
def __init__(self, config):
super().__init__(config)
# The final projection layer is only used for backward compatibility.
# Following https://github.com/auspicious3000/contentvec/issues/6
# Remove this layer is necessary to achieve the desired outcome.
self.final_proj = nn.Linear(config.hidden_size, config.classifier_proj_size)
```
and then load the model with
```python
model = HubertModelWithFinalProj.from_pretrained("lengyue233/content-vec-best")
x = model(audio)["last_hidden_state"]
```
## How to convert
You need to download the ContentVec_legacy model from the official repo, and then run
```bash
python convert.py
```
|
meta-llama/Meta-Llama-3-8B-Instruct | meta-llama | "2024-09-27T15:52:39Z" | 2,183,805 | 3,607 | transformers | [
"transformers",
"safetensors",
"llama",
"text-generation",
"facebook",
"meta",
"pytorch",
"llama-3",
"conversational",
"en",
"license:llama3",
"autotrain_compatible",
"text-generation-inference",
"endpoints_compatible",
"region:us"
] | text-generation | "2024-04-17T09:35:12Z" | ---
language:
- en
pipeline_tag: text-generation
tags:
- facebook
- meta
- pytorch
- llama
- llama-3
license: llama3
new_version: meta-llama/Llama-3.1-8B-Instruct
extra_gated_prompt: >-
### META LLAMA 3 COMMUNITY LICENSE AGREEMENT
Meta Llama 3 Version Release Date: April 18, 2024
"Agreement" means the terms and conditions for use, reproduction, distribution and modification of the
Llama Materials set forth herein.
"Documentation" means the specifications, manuals and documentation accompanying Meta Llama 3
distributed by Meta at https://llama.meta.com/get-started/.
"Licensee" or "you" means you, or your employer or any other person or entity (if you are entering into
this Agreement on such person or entity’s behalf), of the age required under applicable laws, rules or
regulations to provide legal consent and that has legal authority to bind your employer or such other
person or entity if you are entering in this Agreement on their behalf.
"Meta Llama 3" means the foundational large language models and software and algorithms, including
machine-learning model code, trained model weights, inference-enabling code, training-enabling code,
fine-tuning enabling code and other elements of the foregoing distributed by Meta at
https://llama.meta.com/llama-downloads.
"Llama Materials" means, collectively, Meta’s proprietary Meta Llama 3 and Documentation (and any
portion thereof) made available under this Agreement.
"Meta" or "we" means Meta Platforms Ireland Limited (if you are located in or, if you are an entity, your
principal place of business is in the EEA or Switzerland) and Meta Platforms, Inc. (if you are located
outside of the EEA or Switzerland).
1. License Rights and Redistribution.
a. Grant of Rights. You are granted a non-exclusive, worldwide, non-transferable and royalty-free
limited license under Meta’s intellectual property or other rights owned by Meta embodied in the Llama
Materials to use, reproduce, distribute, copy, create derivative works of, and make modifications to the
Llama Materials.
b. Redistribution and Use.
i. If you distribute or make available the Llama Materials (or any derivative works
thereof), or a product or service that uses any of them, including another AI model, you shall (A) provide
a copy of this Agreement with any such Llama Materials; and (B) prominently display “Built with Meta
Llama 3” on a related website, user interface, blogpost, about page, or product documentation. If you
use the Llama Materials to create, train, fine tune, or otherwise improve an AI model, which is
distributed or made available, you shall also include “Llama 3” at the beginning of any such AI model
name.
ii. If you receive Llama Materials, or any derivative works thereof, from a Licensee as part
of an integrated end user product, then Section 2 of this Agreement will not apply to you.
iii. You must retain in all copies of the Llama Materials that you distribute the following
attribution notice within a “Notice” text file distributed as a part of such copies: “Meta Llama 3 is
licensed under the Meta Llama 3 Community License, Copyright © Meta Platforms, Inc. All Rights
Reserved.”
iv. Your use of the Llama Materials must comply with applicable laws and regulations
(including trade compliance laws and regulations) and adhere to the Acceptable Use Policy for the Llama
Materials (available at https://llama.meta.com/llama3/use-policy), which is hereby incorporated by
reference into this Agreement.
v. You will not use the Llama Materials or any output or results of the Llama Materials to
improve any other large language model (excluding Meta Llama 3 or derivative works thereof).
2. Additional Commercial Terms. If, on the Meta Llama 3 version release date, the monthly active users
of the products or services made available by or for Licensee, or Licensee’s affiliates, is greater than 700
million monthly active users in the preceding calendar month, you must request a license from Meta,
which Meta may grant to you in its sole discretion, and you are not authorized to exercise any of the
rights under this Agreement unless or until Meta otherwise expressly grants you such rights.
3. Disclaimer of Warranty. UNLESS REQUIRED BY APPLICABLE LAW, THE LLAMA MATERIALS AND ANY
OUTPUT AND RESULTS THEREFROM ARE PROVIDED ON AN “AS IS” BASIS, WITHOUT WARRANTIES OF
ANY KIND, AND META DISCLAIMS ALL WARRANTIES OF ANY KIND, BOTH EXPRESS AND IMPLIED,
INCLUDING, WITHOUT LIMITATION, ANY WARRANTIES OF TITLE, NON-INFRINGEMENT,
MERCHANTABILITY, OR FITNESS FOR A PARTICULAR PURPOSE. YOU ARE SOLELY RESPONSIBLE FOR
DETERMINING THE APPROPRIATENESS OF USING OR REDISTRIBUTING THE LLAMA MATERIALS AND
ASSUME ANY RISKS ASSOCIATED WITH YOUR USE OF THE LLAMA MATERIALS AND ANY OUTPUT AND
RESULTS.
4. Limitation of Liability. IN NO EVENT WILL META OR ITS AFFILIATES BE LIABLE UNDER ANY THEORY OF
LIABILITY, WHETHER IN CONTRACT, TORT, NEGLIGENCE, PRODUCTS LIABILITY, OR OTHERWISE, ARISING
OUT OF THIS AGREEMENT, FOR ANY LOST PROFITS OR ANY INDIRECT, SPECIAL, CONSEQUENTIAL,
INCIDENTAL, EXEMPLARY OR PUNITIVE DAMAGES, EVEN IF META OR ITS AFFILIATES HAVE BEEN ADVISED
OF THE POSSIBILITY OF ANY OF THE FOREGOING.
5. Intellectual Property.
a. No trademark licenses are granted under this Agreement, and in connection with the Llama
Materials, neither Meta nor Licensee may use any name or mark owned by or associated with the other
or any of its affiliates, except as required for reasonable and customary use in describing and
redistributing the Llama Materials or as set forth in this Section 5(a). Meta hereby grants you a license to
use “Llama 3” (the “Mark”) solely as required to comply with the last sentence of Section 1.b.i. You will
comply with Meta’s brand guidelines (currently accessible at
https://about.meta.com/brand/resources/meta/company-brand/ ). All goodwill arising out of your use
of the Mark will inure to the benefit of Meta.
b. Subject to Meta’s ownership of Llama Materials and derivatives made by or for Meta, with
respect to any derivative works and modifications of the Llama Materials that are made by you, as
between you and Meta, you are and will be the owner of such derivative works and modifications.
c. If you institute litigation or other proceedings against Meta or any entity (including a
cross-claim or counterclaim in a lawsuit) alleging that the Llama Materials or Meta Llama 3 outputs or
results, or any portion of any of the foregoing, constitutes infringement of intellectual property or other
rights owned or licensable by you, then any licenses granted to you under this Agreement shall
terminate as of the date such litigation or claim is filed or instituted. You will indemnify and hold
harmless Meta from and against any claim by any third party arising out of or related to your use or
distribution of the Llama Materials.
6. Term and Termination. The term of this Agreement will commence upon your acceptance of this
Agreement or access to the Llama Materials and will continue in full force and effect until terminated in
accordance with the terms and conditions herein. Meta may terminate this Agreement if you are in
breach of any term or condition of this Agreement. Upon termination of this Agreement, you shall delete
and cease use of the Llama Materials. Sections 3, 4 and 7 shall survive the termination of this
Agreement.
7. Governing Law and Jurisdiction. This Agreement will be governed and construed under the laws of
the State of California without regard to choice of law principles, and the UN Convention on Contracts
for the International Sale of Goods does not apply to this Agreement. The courts of California shall have
exclusive jurisdiction of any dispute arising out of this Agreement.
### Meta Llama 3 Acceptable Use Policy
Meta is committed to promoting safe and fair use of its tools and features, including Meta Llama 3. If you
access or use Meta Llama 3, you agree to this Acceptable Use Policy (“Policy”). The most recent copy of
this policy can be found at [https://llama.meta.com/llama3/use-policy](https://llama.meta.com/llama3/use-policy)
#### Prohibited Uses
We want everyone to use Meta Llama 3 safely and responsibly. You agree you will not use, or allow
others to use, Meta Llama 3 to:
1. Violate the law or others’ rights, including to:
1. Engage in, promote, generate, contribute to, encourage, plan, incite, or further illegal or unlawful activity or content, such as:
1. Violence or terrorism
2. Exploitation or harm to children, including the solicitation, creation, acquisition, or dissemination of child exploitative content or failure to report Child Sexual Abuse Material
3. Human trafficking, exploitation, and sexual violence
4. The illegal distribution of information or materials to minors, including obscene materials, or failure to employ legally required age-gating in connection with such information or materials.
5. Sexual solicitation
6. Any other criminal activity
2. Engage in, promote, incite, or facilitate the harassment, abuse, threatening, or bullying of individuals or groups of individuals
3. Engage in, promote, incite, or facilitate discrimination or other unlawful or harmful conduct in the provision of employment, employment benefits, credit, housing, other economic benefits, or other essential goods and services
4. Engage in the unauthorized or unlicensed practice of any profession including, but not limited to, financial, legal, medical/health, or related professional practices
5. Collect, process, disclose, generate, or infer health, demographic, or other sensitive personal or private information about individuals without rights and consents required by applicable laws
6. Engage in or facilitate any action or generate any content that infringes, misappropriates, or otherwise violates any third-party rights, including the outputs or results of any products or services using the Llama Materials
7. Create, generate, or facilitate the creation of malicious code, malware, computer viruses or do anything else that could disable, overburden, interfere with or impair the proper working, integrity, operation or appearance of a website or computer system
2. Engage in, promote, incite, facilitate, or assist in the planning or development of activities that present a risk of death or bodily harm to individuals, including use of Meta Llama 3 related to the following:
1. Military, warfare, nuclear industries or applications, espionage, use for materials or activities that are subject to the International Traffic Arms Regulations (ITAR) maintained by the United States Department of State
2. Guns and illegal weapons (including weapon development)
3. Illegal drugs and regulated/controlled substances
4. Operation of critical infrastructure, transportation technologies, or heavy machinery
5. Self-harm or harm to others, including suicide, cutting, and eating disorders
6. Any content intended to incite or promote violence, abuse, or any infliction of bodily harm to an individual
3. Intentionally deceive or mislead others, including use of Meta Llama 3 related to the following:
1. Generating, promoting, or furthering fraud or the creation or promotion of disinformation
2. Generating, promoting, or furthering defamatory content, including the creation of defamatory statements, images, or other content
3. Generating, promoting, or further distributing spam
4. Impersonating another individual without consent, authorization, or legal right
5. Representing that the use of Meta Llama 3 or outputs are human-generated
6. Generating or facilitating false online engagement, including fake reviews and other means of fake online engagement
4. Fail to appropriately disclose to end users any known dangers of your AI system
Please report any violation of this Policy, software “bug,” or other problems that could lead to a violation
of this Policy through one of the following means:
* Reporting issues with the model: [https://github.com/meta-llama/llama3](https://github.com/meta-llama/llama3)
* Reporting risky content generated by the model:
developers.facebook.com/llama_output_feedback
* Reporting bugs and security concerns: facebook.com/whitehat/info
* Reporting violations of the Acceptable Use Policy or unlicensed uses of Meta Llama 3: [email protected]
extra_gated_fields:
First Name: text
Last Name: text
Date of birth: date_picker
Country: country
Affiliation: text
geo: ip_location
By clicking Submit below I accept the terms of the license and acknowledge that the information I provide will be collected stored processed and shared in accordance with the Meta Privacy Policy: checkbox
extra_gated_description: The information you provide will be collected, stored, processed and shared in accordance with the [Meta Privacy Policy](https://www.facebook.com/privacy/policy/).
extra_gated_button_content: Submit
widget:
- example_title: Hello
messages:
- role: user
content: Hey my name is Julien! How are you?
- example_title: Winter holidays
messages:
- role: system
content: You are a helpful and honest assistant. Please, respond concisely and truthfully.
- role: user
content: Can you recommend a good destination for Winter holidays?
- example_title: Programming assistant
messages:
- role: system
content: You are a helpful and honest code and programming assistant. Please, respond concisely and truthfully.
- role: user
content: Write a function that computes the nth fibonacci number.
inference:
parameters:
max_new_tokens: 300
stop:
- <|end_of_text|>
- <|eot_id|>
---
## Model Details
Meta developed and released the Meta Llama 3 family of large language models (LLMs), a collection of pretrained and instruction tuned generative text models in 8 and 70B sizes. The Llama 3 instruction tuned models are optimized for dialogue use cases and outperform many of the available open source chat models on common industry benchmarks. Further, in developing these models, we took great care to optimize helpfulness and safety.
**Model developers** Meta
**Variations** Llama 3 comes in two sizes — 8B and 70B parameters — in pre-trained and instruction tuned variants.
**Input** Models input text only.
**Output** Models generate text and code only.
**Model Architecture** Llama 3 is an auto-regressive language model that uses an optimized transformer architecture. The tuned versions use supervised fine-tuning (SFT) and reinforcement learning with human feedback (RLHF) to align with human preferences for helpfulness and safety.
<table>
<tr>
<td>
</td>
<td><strong>Training Data</strong>
</td>
<td><strong>Params</strong>
</td>
<td><strong>Context length</strong>
</td>
<td><strong>GQA</strong>
</td>
<td><strong>Token count</strong>
</td>
<td><strong>Knowledge cutoff</strong>
</td>
</tr>
<tr>
<td rowspan="2" >Llama 3
</td>
<td rowspan="2" >A new mix of publicly available online data.
</td>
<td>8B
</td>
<td>8k
</td>
<td>Yes
</td>
<td rowspan="2" >15T+
</td>
<td>March, 2023
</td>
</tr>
<tr>
<td>70B
</td>
<td>8k
</td>
<td>Yes
</td>
<td>December, 2023
</td>
</tr>
</table>
**Llama 3 family of models**. Token counts refer to pretraining data only. Both the 8 and 70B versions use Grouped-Query Attention (GQA) for improved inference scalability.
**Model Release Date** April 18, 2024.
**Status** This is a static model trained on an offline dataset. Future versions of the tuned models will be released as we improve model safety with community feedback.
**License** A custom commercial license is available at: [https://llama.meta.com/llama3/license](https://llama.meta.com/llama3/license)
Where to send questions or comments about the model Instructions on how to provide feedback or comments on the model can be found in the model [README](https://github.com/meta-llama/llama3). For more technical information about generation parameters and recipes for how to use Llama 3 in applications, please go [here](https://github.com/meta-llama/llama-recipes).
## Intended Use
**Intended Use Cases** Llama 3 is intended for commercial and research use in English. Instruction tuned models are intended for assistant-like chat, whereas pretrained models can be adapted for a variety of natural language generation tasks.
**Out-of-scope** Use in any manner that violates applicable laws or regulations (including trade compliance laws). Use in any other way that is prohibited by the Acceptable Use Policy and Llama 3 Community License. Use in languages other than English**.
**Note: Developers may fine-tune Llama 3 models for languages beyond English provided they comply with the Llama 3 Community License and the Acceptable Use Policy.
## How to use
This repository contains two versions of Meta-Llama-3-8B-Instruct, for use with transformers and with the original `llama3` codebase.
### Use with transformers
You can run conversational inference using the Transformers pipeline abstraction, or by leveraging the Auto classes with the `generate()` function. Let's see examples of both.
#### Transformers pipeline
```python
import transformers
import torch
model_id = "meta-llama/Meta-Llama-3-8B-Instruct"
pipeline = transformers.pipeline(
"text-generation",
model=model_id,
model_kwargs={"torch_dtype": torch.bfloat16},
device_map="auto",
)
messages = [
{"role": "system", "content": "You are a pirate chatbot who always responds in pirate speak!"},
{"role": "user", "content": "Who are you?"},
]
terminators = [
pipeline.tokenizer.eos_token_id,
pipeline.tokenizer.convert_tokens_to_ids("<|eot_id|>")
]
outputs = pipeline(
messages,
max_new_tokens=256,
eos_token_id=terminators,
do_sample=True,
temperature=0.6,
top_p=0.9,
)
print(outputs[0]["generated_text"][-1])
```
#### Transformers AutoModelForCausalLM
```python
from transformers import AutoTokenizer, AutoModelForCausalLM
import torch
model_id = "meta-llama/Meta-Llama-3-8B-Instruct"
tokenizer = AutoTokenizer.from_pretrained(model_id)
model = AutoModelForCausalLM.from_pretrained(
model_id,
torch_dtype=torch.bfloat16,
device_map="auto",
)
messages = [
{"role": "system", "content": "You are a pirate chatbot who always responds in pirate speak!"},
{"role": "user", "content": "Who are you?"},
]
input_ids = tokenizer.apply_chat_template(
messages,
add_generation_prompt=True,
return_tensors="pt"
).to(model.device)
terminators = [
tokenizer.eos_token_id,
tokenizer.convert_tokens_to_ids("<|eot_id|>")
]
outputs = model.generate(
input_ids,
max_new_tokens=256,
eos_token_id=terminators,
do_sample=True,
temperature=0.6,
top_p=0.9,
)
response = outputs[0][input_ids.shape[-1]:]
print(tokenizer.decode(response, skip_special_tokens=True))
```
### Use with `llama3`
Please, follow the instructions in the [repository](https://github.com/meta-llama/llama3)
To download Original checkpoints, see the example command below leveraging `huggingface-cli`:
```
huggingface-cli download meta-llama/Meta-Llama-3-8B-Instruct --include "original/*" --local-dir Meta-Llama-3-8B-Instruct
```
For Hugging Face support, we recommend using transformers or TGI, but a similar command works.
## Hardware and Software
**Training Factors** We used custom training libraries, Meta's Research SuperCluster, and production clusters for pretraining. Fine-tuning, annotation, and evaluation were also performed on third-party cloud compute.
**Carbon Footprint Pretraining utilized a cumulative** 7.7M GPU hours of computation on hardware of type H100-80GB (TDP of 700W). Estimated total emissions were 2290 tCO2eq, 100% of which were offset by Meta’s sustainability program.
<table>
<tr>
<td>
</td>
<td><strong>Time (GPU hours)</strong>
</td>
<td><strong>Power Consumption (W)</strong>
</td>
<td><strong>Carbon Emitted(tCO2eq)</strong>
</td>
</tr>
<tr>
<td>Llama 3 8B
</td>
<td>1.3M
</td>
<td>700
</td>
<td>390
</td>
</tr>
<tr>
<td>Llama 3 70B
</td>
<td>6.4M
</td>
<td>700
</td>
<td>1900
</td>
</tr>
<tr>
<td>Total
</td>
<td>7.7M
</td>
<td>
</td>
<td>2290
</td>
</tr>
</table>
**CO2 emissions during pre-training**. Time: total GPU time required for training each model. Power Consumption: peak power capacity per GPU device for the GPUs used adjusted for power usage efficiency. 100% of the emissions are directly offset by Meta's sustainability program, and because we are openly releasing these models, the pretraining costs do not need to be incurred by others.
## Training Data
**Overview** Llama 3 was pretrained on over 15 trillion tokens of data from publicly available sources. The fine-tuning data includes publicly available instruction datasets, as well as over 10M human-annotated examples. Neither the pretraining nor the fine-tuning datasets include Meta user data.
**Data Freshness** The pretraining data has a cutoff of March 2023 for the 8B and December 2023 for the 70B models respectively.
## Benchmarks
In this section, we report the results for Llama 3 models on standard automatic benchmarks. For all the evaluations, we use our internal evaluations library. For details on the methodology see [here](https://github.com/meta-llama/llama3/blob/main/eval_methodology.md).
### Base pretrained models
<table>
<tr>
<td><strong>Category</strong>
</td>
<td><strong>Benchmark</strong>
</td>
<td><strong>Llama 3 8B</strong>
</td>
<td><strong>Llama2 7B</strong>
</td>
<td><strong>Llama2 13B</strong>
</td>
<td><strong>Llama 3 70B</strong>
</td>
<td><strong>Llama2 70B</strong>
</td>
</tr>
<tr>
<td rowspan="6" >General
</td>
<td>MMLU (5-shot)
</td>
<td>66.6
</td>
<td>45.7
</td>
<td>53.8
</td>
<td>79.5
</td>
<td>69.7
</td>
</tr>
<tr>
<td>AGIEval English (3-5 shot)
</td>
<td>45.9
</td>
<td>28.8
</td>
<td>38.7
</td>
<td>63.0
</td>
<td>54.8
</td>
</tr>
<tr>
<td>CommonSenseQA (7-shot)
</td>
<td>72.6
</td>
<td>57.6
</td>
<td>67.6
</td>
<td>83.8
</td>
<td>78.7
</td>
</tr>
<tr>
<td>Winogrande (5-shot)
</td>
<td>76.1
</td>
<td>73.3
</td>
<td>75.4
</td>
<td>83.1
</td>
<td>81.8
</td>
</tr>
<tr>
<td>BIG-Bench Hard (3-shot, CoT)
</td>
<td>61.1
</td>
<td>38.1
</td>
<td>47.0
</td>
<td>81.3
</td>
<td>65.7
</td>
</tr>
<tr>
<td>ARC-Challenge (25-shot)
</td>
<td>78.6
</td>
<td>53.7
</td>
<td>67.6
</td>
<td>93.0
</td>
<td>85.3
</td>
</tr>
<tr>
<td>Knowledge reasoning
</td>
<td>TriviaQA-Wiki (5-shot)
</td>
<td>78.5
</td>
<td>72.1
</td>
<td>79.6
</td>
<td>89.7
</td>
<td>87.5
</td>
</tr>
<tr>
<td rowspan="4" >Reading comprehension
</td>
<td>SQuAD (1-shot)
</td>
<td>76.4
</td>
<td>72.2
</td>
<td>72.1
</td>
<td>85.6
</td>
<td>82.6
</td>
</tr>
<tr>
<td>QuAC (1-shot, F1)
</td>
<td>44.4
</td>
<td>39.6
</td>
<td>44.9
</td>
<td>51.1
</td>
<td>49.4
</td>
</tr>
<tr>
<td>BoolQ (0-shot)
</td>
<td>75.7
</td>
<td>65.5
</td>
<td>66.9
</td>
<td>79.0
</td>
<td>73.1
</td>
</tr>
<tr>
<td>DROP (3-shot, F1)
</td>
<td>58.4
</td>
<td>37.9
</td>
<td>49.8
</td>
<td>79.7
</td>
<td>70.2
</td>
</tr>
</table>
### Instruction tuned models
<table>
<tr>
<td><strong>Benchmark</strong>
</td>
<td><strong>Llama 3 8B</strong>
</td>
<td><strong>Llama 2 7B</strong>
</td>
<td><strong>Llama 2 13B</strong>
</td>
<td><strong>Llama 3 70B</strong>
</td>
<td><strong>Llama 2 70B</strong>
</td>
</tr>
<tr>
<td>MMLU (5-shot)
</td>
<td>68.4
</td>
<td>34.1
</td>
<td>47.8
</td>
<td>82.0
</td>
<td>52.9
</td>
</tr>
<tr>
<td>GPQA (0-shot)
</td>
<td>34.2
</td>
<td>21.7
</td>
<td>22.3
</td>
<td>39.5
</td>
<td>21.0
</td>
</tr>
<tr>
<td>HumanEval (0-shot)
</td>
<td>62.2
</td>
<td>7.9
</td>
<td>14.0
</td>
<td>81.7
</td>
<td>25.6
</td>
</tr>
<tr>
<td>GSM-8K (8-shot, CoT)
</td>
<td>79.6
</td>
<td>25.7
</td>
<td>77.4
</td>
<td>93.0
</td>
<td>57.5
</td>
</tr>
<tr>
<td>MATH (4-shot, CoT)
</td>
<td>30.0
</td>
<td>3.8
</td>
<td>6.7
</td>
<td>50.4
</td>
<td>11.6
</td>
</tr>
</table>
### Responsibility & Safety
We believe that an open approach to AI leads to better, safer products, faster innovation, and a bigger overall market. We are committed to Responsible AI development and took a series of steps to limit misuse and harm and support the open source community.
Foundation models are widely capable technologies that are built to be used for a diverse range of applications. They are not designed to meet every developer preference on safety levels for all use cases, out-of-the-box, as those by their nature will differ across different applications.
Rather, responsible LLM-application deployment is achieved by implementing a series of safety best practices throughout the development of such applications, from the model pre-training, fine-tuning and the deployment of systems composed of safeguards to tailor the safety needs specifically to the use case and audience.
As part of the Llama 3 release, we updated our [Responsible Use Guide](https://llama.meta.com/responsible-use-guide/) to outline the steps and best practices for developers to implement model and system level safety for their application. We also provide a set of resources including [Meta Llama Guard 2](https://llama.meta.com/purple-llama/) and [Code Shield](https://llama.meta.com/purple-llama/) safeguards. These tools have proven to drastically reduce residual risks of LLM Systems, while maintaining a high level of helpfulness. We encourage developers to tune and deploy these safeguards according to their needs and we provide a [reference implementation](https://github.com/meta-llama/llama-recipes/tree/main/recipes/responsible_ai) to get you started.
#### Llama 3-Instruct
As outlined in the Responsible Use Guide, some trade-off between model helpfulness and model alignment is likely unavoidable. Developers should exercise discretion about how to weigh the benefits of alignment and helpfulness for their specific use case and audience. Developers should be mindful of residual risks when using Llama models and leverage additional safety tools as needed to reach the right safety bar for their use case.
<span style="text-decoration:underline;">Safety</span>
For our instruction tuned model, we conducted extensive red teaming exercises, performed adversarial evaluations and implemented safety mitigations techniques to lower residual risks. As with any Large Language Model, residual risks will likely remain and we recommend that developers assess these risks in the context of their use case. In parallel, we are working with the community to make AI safety benchmark standards transparent, rigorous and interpretable.
<span style="text-decoration:underline;">Refusals</span>
In addition to residual risks, we put a great emphasis on model refusals to benign prompts. Over-refusing not only can impact the user experience but could even be harmful in certain contexts as well. We’ve heard the feedback from the developer community and improved our fine tuning to ensure that Llama 3 is significantly less likely to falsely refuse to answer prompts than Llama 2.
We built internal benchmarks and developed mitigations to limit false refusals making Llama 3 our most helpful model to date.
#### Responsible release
In addition to responsible use considerations outlined above, we followed a rigorous process that requires us to take extra measures against misuse and critical risks before we make our release decision.
Misuse
If you access or use Llama 3, you agree to the Acceptable Use Policy. The most recent copy of this policy can be found at [https://llama.meta.com/llama3/use-policy/](https://llama.meta.com/llama3/use-policy/).
#### Critical risks
<span style="text-decoration:underline;">CBRNE</span> (Chemical, Biological, Radiological, Nuclear, and high yield Explosives)
We have conducted a two fold assessment of the safety of the model in this area:
* Iterative testing during model training to assess the safety of responses related to CBRNE threats and other adversarial risks.
* Involving external CBRNE experts to conduct an uplift test assessing the ability of the model to accurately provide expert knowledge and reduce barriers to potential CBRNE misuse, by reference to what can be achieved using web search (without the model).
### <span style="text-decoration:underline;">Cyber Security </span>
We have evaluated Llama 3 with CyberSecEval, Meta’s cybersecurity safety eval suite, measuring Llama 3’s propensity to suggest insecure code when used as a coding assistant, and Llama 3’s propensity to comply with requests to help carry out cyber attacks, where attacks are defined by the industry standard MITRE ATT&CK cyber attack ontology. On our insecure coding and cyber attacker helpfulness tests, Llama 3 behaved in the same range or safer than models of [equivalent coding capability](https://huggingface.co/spaces/facebook/CyberSecEval).
### <span style="text-decoration:underline;">Child Safety</span>
Child Safety risk assessments were conducted using a team of experts, to assess the model’s capability to produce outputs that could result in Child Safety risks and inform on any necessary and appropriate risk mitigations via fine tuning. We leveraged those expert red teaming sessions to expand the coverage of our evaluation benchmarks through Llama 3 model development. For Llama 3, we conducted new in-depth sessions using objective based methodologies to assess the model risks along multiple attack vectors. We also partnered with content specialists to perform red teaming exercises assessing potentially violating content while taking account of market specific nuances or experiences.
### Community
Generative AI safety requires expertise and tooling, and we believe in the strength of the open community to accelerate its progress. We are active members of open consortiums, including the AI Alliance, Partnership in AI and MLCommons, actively contributing to safety standardization and transparency. We encourage the community to adopt taxonomies like the MLCommons Proof of Concept evaluation to facilitate collaboration and transparency on safety and content evaluations. Our Purple Llama tools are open sourced for the community to use and widely distributed across ecosystem partners including cloud service providers. We encourage community contributions to our [Github repository](https://github.com/meta-llama/PurpleLlama).
Finally, we put in place a set of resources including an [output reporting mechanism](https://developers.facebook.com/llama_output_feedback) and [bug bounty program](https://www.facebook.com/whitehat) to continuously improve the Llama technology with the help of the community.
## Ethical Considerations and Limitations
The core values of Llama 3 are openness, inclusivity and helpfulness. It is meant to serve everyone, and to work for a wide range of use cases. It is thus designed to be accessible to people across many different backgrounds, experiences and perspectives. Llama 3 addresses users and their needs as they are, without insertion unnecessary judgment or normativity, while reflecting the understanding that even content that may appear problematic in some cases can serve valuable purposes in others. It respects the dignity and autonomy of all users, especially in terms of the values of free thought and expression that power innovation and progress.
But Llama 3 is a new technology, and like any new technology, there are risks associated with its use. Testing conducted to date has been in English, and has not covered, nor could it cover, all scenarios. For these reasons, as with all LLMs, Llama 3’s potential outputs cannot be predicted in advance, and the model may in some instances produce inaccurate, biased or other objectionable responses to user prompts. Therefore, before deploying any applications of Llama 3 models, developers should perform safety testing and tuning tailored to their specific applications of the model. As outlined in the Responsible Use Guide, we recommend incorporating [Purple Llama](https://github.com/facebookresearch/PurpleLlama) solutions into your workflows and specifically [Llama Guard](https://ai.meta.com/research/publications/llama-guard-llm-based-input-output-safeguard-for-human-ai-conversations/) which provides a base model to filter input and output prompts to layer system-level safety on top of model-level safety.
Please see the Responsible Use Guide available at [http://llama.meta.com/responsible-use-guide](http://llama.meta.com/responsible-use-guide)
## Citation instructions
@article{llama3modelcard,
title={Llama 3 Model Card},
author={AI@Meta},
year={2024},
url = {https://github.com/meta-llama/llama3/blob/main/MODEL_CARD.md}
}
## Contributors
Aaditya Singh; Aaron Grattafiori; Abhimanyu Dubey; Abhinav Jauhri; Abhinav Pandey; Abhishek Kadian; Adam Kelsey; Adi Gangidi; Ahmad Al-Dahle; Ahuva Goldstand; Aiesha Letman; Ajay Menon; Akhil Mathur; Alan Schelten; Alex Vaughan; Amy Yang; Andrei Lupu; Andres Alvarado; Andrew Gallagher; Andrew Gu; Andrew Ho; Andrew Poulton; Andrew Ryan; Angela Fan; Ankit Ramchandani; Anthony Hartshorn; Archi Mitra; Archie Sravankumar; Artem Korenev; Arun Rao; Ashley Gabriel; Ashwin Bharambe; Assaf Eisenman; Aston Zhang; Aurelien Rodriguez; Austen Gregerson; Ava Spataru; Baptiste Roziere; Ben Maurer; Benjamin Leonhardi; Bernie Huang; Bhargavi Paranjape; Bing Liu; Binh Tang; Bobbie Chern; Brani Stojkovic; Brian Fuller; Catalina Mejia Arenas; Chao Zhou; Charlotte Caucheteux; Chaya Nayak; Ching-Hsiang Chu; Chloe Bi; Chris Cai; Chris Cox; Chris Marra; Chris McConnell; Christian Keller; Christoph Feichtenhofer; Christophe Touret; Chunyang Wu; Corinne Wong; Cristian Canton Ferrer; Damien Allonsius; Daniel Kreymer; Daniel Haziza; Daniel Li; Danielle Pintz; Danny Livshits; Danny Wyatt; David Adkins; David Esiobu; David Xu; Davide Testuggine; Delia David; Devi Parikh; Dhruv Choudhary; Dhruv Mahajan; Diana Liskovich; Diego Garcia-Olano; Diego Perino; Dieuwke Hupkes; Dingkang Wang; Dustin Holland; Egor Lakomkin; Elina Lobanova; Xiaoqing Ellen Tan; Emily Dinan; Eric Smith; Erik Brinkman; Esteban Arcaute; Filip Radenovic; Firat Ozgenel; Francesco Caggioni; Frank Seide; Frank Zhang; Gabriel Synnaeve; Gabriella Schwarz; Gabrielle Lee; Gada Badeer; Georgia Anderson; Graeme Nail; Gregoire Mialon; Guan Pang; Guillem Cucurell; Hailey Nguyen; Hannah Korevaar; Hannah Wang; Haroun Habeeb; Harrison Rudolph; Henry Aspegren; Hu Xu; Hugo Touvron; Iga Kozlowska; Igor Molybog; Igor Tufanov; Iliyan Zarov; Imanol Arrieta Ibarra; Irina-Elena Veliche; Isabel Kloumann; Ishan Misra; Ivan Evtimov; Jacob Xu; Jade Copet; Jake Weissman; Jan Geffert; Jana Vranes; Japhet Asher; Jason Park; Jay Mahadeokar; Jean-Baptiste Gaya; Jeet Shah; Jelmer van der Linde; Jennifer Chan; Jenny Hong; Jenya Lee; Jeremy Fu; Jeremy Teboul; Jianfeng Chi; Jianyu Huang; Jie Wang; Jiecao Yu; Joanna Bitton; Joe Spisak; Joelle Pineau; Jon Carvill; Jongsoo Park; Joseph Rocca; Joshua Johnstun; Junteng Jia; Kalyan Vasuden Alwala; Kam Hou U; Kate Plawiak; Kartikeya Upasani; Kaushik Veeraraghavan; Ke Li; Kenneth Heafield; Kevin Stone; Khalid El-Arini; Krithika Iyer; Kshitiz Malik; Kuenley Chiu; Kunal Bhalla; Kyle Huang; Lakshya Garg; Lauren Rantala-Yeary; Laurens van der Maaten; Lawrence Chen; Leandro Silva; Lee Bell; Lei Zhang; Liang Tan; Louis Martin; Lovish Madaan; Luca Wehrstedt; Lukas Blecher; Luke de Oliveira; Madeline Muzzi; Madian Khabsa; Manav Avlani; Mannat Singh; Manohar Paluri; Mark Zuckerberg; Marcin Kardas; Martynas Mankus; Mathew Oldham; Mathieu Rita; Matthew Lennie; Maya Pavlova; Meghan Keneally; Melanie Kambadur; Mihir Patel; Mikayel Samvelyan; Mike Clark; Mike Lewis; Min Si; Mitesh Kumar Singh; Mo Metanat; Mona Hassan; Naman Goyal; Narjes Torabi; Nicolas Usunier; Nikolay Bashlykov; Nikolay Bogoychev; Niladri Chatterji; Ning Dong; Oliver Aobo Yang; Olivier Duchenne; Onur Celebi; Parth Parekh; Patrick Alrassy; Paul Saab; Pavan Balaji; Pedro Rittner; Pengchuan Zhang; Pengwei Li; Petar Vasic; Peter Weng; Polina Zvyagina; Prajjwal Bhargava; Pratik Dubal; Praveen Krishnan; Punit Singh Koura; Qing He; Rachel Rodriguez; Ragavan Srinivasan; Rahul Mitra; Ramon Calderer; Raymond Li; Robert Stojnic; Roberta Raileanu; Robin Battey; Rocky Wang; Rohit Girdhar; Rohit Patel; Romain Sauvestre; Ronnie Polidoro; Roshan Sumbaly; Ross Taylor; Ruan Silva; Rui Hou; Rui Wang; Russ Howes; Ruty Rinott; Saghar Hosseini; Sai Jayesh Bondu; Samyak Datta; Sanjay Singh; Sara Chugh; Sargun Dhillon; Satadru Pan; Sean Bell; Sergey Edunov; Shaoliang Nie; Sharan Narang; Sharath Raparthy; Shaun Lindsay; Sheng Feng; Sheng Shen; Shenghao Lin; Shiva Shankar; Shruti Bhosale; Shun Zhang; Simon Vandenhende; Sinong Wang; Seohyun Sonia Kim; Soumya Batra; Sten Sootla; Steve Kehoe; Suchin Gururangan; Sumit Gupta; Sunny Virk; Sydney Borodinsky; Tamar Glaser; Tamar Herman; Tamara Best; Tara Fowler; Thomas Georgiou; Thomas Scialom; Tianhe Li; Todor Mihaylov; Tong Xiao; Ujjwal Karn; Vedanuj Goswami; Vibhor Gupta; Vignesh Ramanathan; Viktor Kerkez; Vinay Satish Kumar; Vincent Gonguet; Vish Vogeti; Vlad Poenaru; Vlad Tiberiu Mihailescu; Vladan Petrovic; Vladimir Ivanov; Wei Li; Weiwei Chu; Wenhan Xiong; Wenyin Fu; Wes Bouaziz; Whitney Meers; Will Constable; Xavier Martinet; Xiaojian Wu; Xinbo Gao; Xinfeng Xie; Xuchao Jia; Yaelle Goldschlag; Yann LeCun; Yashesh Gaur; Yasmine Babaei; Ye Qi; Yenda Li; Yi Wen; Yiwen Song; Youngjin Nam; Yuchen Hao; Yuchen Zhang; Yun Wang; Yuning Mao; Yuzi He; Zacharie Delpierre Coudert; Zachary DeVito; Zahra Hankir; Zhaoduo Wen; Zheng Yan; Zhengxing Chen; Zhenyu Yang; Zoe Papakipos
|
timm/resnet18.a1_in1k | timm | "2024-02-10T21:14:05Z" | 2,162,890 | 10 | timm | [
"timm",
"pytorch",
"safetensors",
"image-classification",
"arxiv:2110.00476",
"arxiv:1512.03385",
"license:apache-2.0",
"region:us"
] | image-classification | "2023-04-05T18:02:50Z" | ---
tags:
- image-classification
- timm
license: apache-2.0
library_name: timm
---
# Model card for resnet18.a1_in1k
A ResNet-B image classification model.
This model features:
* ReLU activations
* single layer 7x7 convolution with pooling
* 1x1 convolution shortcut downsample
Trained on ImageNet-1k in `timm` using recipe template described below.
Recipe details:
* ResNet Strikes Back `A1` recipe
* LAMB optimizer with BCE loss
* Cosine LR schedule with warmup
## Model Details
- **Model Type:** Image classification / feature backbone
- **Model Stats:**
- Params (M): 11.7
- GMACs: 1.8
- Activations (M): 2.5
- Image size: train = 224 x 224, test = 288 x 288
- **Papers:**
- ResNet strikes back: An improved training procedure in timm: https://arxiv.org/abs/2110.00476
- Deep Residual Learning for Image Recognition: https://arxiv.org/abs/1512.03385
- **Original:** https://github.com/huggingface/pytorch-image-models
## Model Usage
### Image Classification
```python
from urllib.request import urlopen
from PIL import Image
import timm
img = Image.open(urlopen(
'https://huggingface.co/datasets/huggingface/documentation-images/resolve/main/beignets-task-guide.png'
))
model = timm.create_model('resnet18.a1_in1k', pretrained=True)
model = model.eval()
# get model specific transforms (normalization, resize)
data_config = timm.data.resolve_model_data_config(model)
transforms = timm.data.create_transform(**data_config, is_training=False)
output = model(transforms(img).unsqueeze(0)) # unsqueeze single image into batch of 1
top5_probabilities, top5_class_indices = torch.topk(output.softmax(dim=1) * 100, k=5)
```
### Feature Map Extraction
```python
from urllib.request import urlopen
from PIL import Image
import timm
img = Image.open(urlopen(
'https://huggingface.co/datasets/huggingface/documentation-images/resolve/main/beignets-task-guide.png'
))
model = timm.create_model(
'resnet18.a1_in1k',
pretrained=True,
features_only=True,
)
model = model.eval()
# get model specific transforms (normalization, resize)
data_config = timm.data.resolve_model_data_config(model)
transforms = timm.data.create_transform(**data_config, is_training=False)
output = model(transforms(img).unsqueeze(0)) # unsqueeze single image into batch of 1
for o in output:
# print shape of each feature map in output
# e.g.:
# torch.Size([1, 64, 112, 112])
# torch.Size([1, 64, 56, 56])
# torch.Size([1, 128, 28, 28])
# torch.Size([1, 256, 14, 14])
# torch.Size([1, 512, 7, 7])
print(o.shape)
```
### Image Embeddings
```python
from urllib.request import urlopen
from PIL import Image
import timm
img = Image.open(urlopen(
'https://huggingface.co/datasets/huggingface/documentation-images/resolve/main/beignets-task-guide.png'
))
model = timm.create_model(
'resnet18.a1_in1k',
pretrained=True,
num_classes=0, # remove classifier nn.Linear
)
model = model.eval()
# get model specific transforms (normalization, resize)
data_config = timm.data.resolve_model_data_config(model)
transforms = timm.data.create_transform(**data_config, is_training=False)
output = model(transforms(img).unsqueeze(0)) # output is (batch_size, num_features) shaped tensor
# or equivalently (without needing to set num_classes=0)
output = model.forward_features(transforms(img).unsqueeze(0))
# output is unpooled, a (1, 512, 7, 7) shaped tensor
output = model.forward_head(output, pre_logits=True)
# output is a (1, num_features) shaped tensor
```
## Model Comparison
Explore the dataset and runtime metrics of this model in timm [model results](https://github.com/huggingface/pytorch-image-models/tree/main/results).
|model |img_size|top1 |top5 |param_count|gmacs|macts|img/sec|
|------------------------------------------|--------|-----|-----|-----------|-----|-----|-------|
|[seresnextaa101d_32x8d.sw_in12k_ft_in1k_288](https://huggingface.co/timm/seresnextaa101d_32x8d.sw_in12k_ft_in1k_288)|320 |86.72|98.17|93.6 |35.2 |69.7 |451 |
|[seresnextaa101d_32x8d.sw_in12k_ft_in1k_288](https://huggingface.co/timm/seresnextaa101d_32x8d.sw_in12k_ft_in1k_288)|288 |86.51|98.08|93.6 |28.5 |56.4 |560 |
|[seresnextaa101d_32x8d.sw_in12k_ft_in1k](https://huggingface.co/timm/seresnextaa101d_32x8d.sw_in12k_ft_in1k)|288 |86.49|98.03|93.6 |28.5 |56.4 |557 |
|[seresnextaa101d_32x8d.sw_in12k_ft_in1k](https://huggingface.co/timm/seresnextaa101d_32x8d.sw_in12k_ft_in1k)|224 |85.96|97.82|93.6 |17.2 |34.2 |923 |
|[resnext101_32x32d.fb_wsl_ig1b_ft_in1k](https://huggingface.co/timm/resnext101_32x32d.fb_wsl_ig1b_ft_in1k)|224 |85.11|97.44|468.5 |87.3 |91.1 |254 |
|[resnetrs420.tf_in1k](https://huggingface.co/timm/resnetrs420.tf_in1k)|416 |85.0 |97.12|191.9 |108.4|213.8|134 |
|[ecaresnet269d.ra2_in1k](https://huggingface.co/timm/ecaresnet269d.ra2_in1k)|352 |84.96|97.22|102.1 |50.2 |101.2|291 |
|[ecaresnet269d.ra2_in1k](https://huggingface.co/timm/ecaresnet269d.ra2_in1k)|320 |84.73|97.18|102.1 |41.5 |83.7 |353 |
|[resnetrs350.tf_in1k](https://huggingface.co/timm/resnetrs350.tf_in1k)|384 |84.71|96.99|164.0 |77.6 |154.7|183 |
|[seresnextaa101d_32x8d.ah_in1k](https://huggingface.co/timm/seresnextaa101d_32x8d.ah_in1k)|288 |84.57|97.08|93.6 |28.5 |56.4 |557 |
|[resnetrs200.tf_in1k](https://huggingface.co/timm/resnetrs200.tf_in1k)|320 |84.45|97.08|93.2 |31.5 |67.8 |446 |
|[resnetrs270.tf_in1k](https://huggingface.co/timm/resnetrs270.tf_in1k)|352 |84.43|96.97|129.9 |51.1 |105.5|280 |
|[seresnext101d_32x8d.ah_in1k](https://huggingface.co/timm/seresnext101d_32x8d.ah_in1k)|288 |84.36|96.92|93.6 |27.6 |53.0 |595 |
|[seresnet152d.ra2_in1k](https://huggingface.co/timm/seresnet152d.ra2_in1k)|320 |84.35|97.04|66.8 |24.1 |47.7 |610 |
|[resnetrs350.tf_in1k](https://huggingface.co/timm/resnetrs350.tf_in1k)|288 |84.3 |96.94|164.0 |43.7 |87.1 |333 |
|[resnext101_32x8d.fb_swsl_ig1b_ft_in1k](https://huggingface.co/timm/resnext101_32x8d.fb_swsl_ig1b_ft_in1k)|224 |84.28|97.17|88.8 |16.5 |31.2 |1100 |
|[resnetrs420.tf_in1k](https://huggingface.co/timm/resnetrs420.tf_in1k)|320 |84.24|96.86|191.9 |64.2 |126.6|228 |
|[seresnext101_32x8d.ah_in1k](https://huggingface.co/timm/seresnext101_32x8d.ah_in1k)|288 |84.19|96.87|93.6 |27.2 |51.6 |613 |
|[resnext101_32x16d.fb_wsl_ig1b_ft_in1k](https://huggingface.co/timm/resnext101_32x16d.fb_wsl_ig1b_ft_in1k)|224 |84.18|97.19|194.0 |36.3 |51.2 |581 |
|[resnetaa101d.sw_in12k_ft_in1k](https://huggingface.co/timm/resnetaa101d.sw_in12k_ft_in1k)|288 |84.11|97.11|44.6 |15.1 |29.0 |1144 |
|[resnet200d.ra2_in1k](https://huggingface.co/timm/resnet200d.ra2_in1k)|320 |83.97|96.82|64.7 |31.2 |67.3 |518 |
|[resnetrs200.tf_in1k](https://huggingface.co/timm/resnetrs200.tf_in1k)|256 |83.87|96.75|93.2 |20.2 |43.4 |692 |
|[seresnextaa101d_32x8d.ah_in1k](https://huggingface.co/timm/seresnextaa101d_32x8d.ah_in1k)|224 |83.86|96.65|93.6 |17.2 |34.2 |923 |
|[resnetrs152.tf_in1k](https://huggingface.co/timm/resnetrs152.tf_in1k)|320 |83.72|96.61|86.6 |24.3 |48.1 |617 |
|[seresnet152d.ra2_in1k](https://huggingface.co/timm/seresnet152d.ra2_in1k)|256 |83.69|96.78|66.8 |15.4 |30.6 |943 |
|[seresnext101d_32x8d.ah_in1k](https://huggingface.co/timm/seresnext101d_32x8d.ah_in1k)|224 |83.68|96.61|93.6 |16.7 |32.0 |986 |
|[resnet152d.ra2_in1k](https://huggingface.co/timm/resnet152d.ra2_in1k)|320 |83.67|96.74|60.2 |24.1 |47.7 |706 |
|[resnetrs270.tf_in1k](https://huggingface.co/timm/resnetrs270.tf_in1k)|256 |83.59|96.61|129.9 |27.1 |55.8 |526 |
|[seresnext101_32x8d.ah_in1k](https://huggingface.co/timm/seresnext101_32x8d.ah_in1k)|224 |83.58|96.4 |93.6 |16.5 |31.2 |1013 |
|[resnetaa101d.sw_in12k_ft_in1k](https://huggingface.co/timm/resnetaa101d.sw_in12k_ft_in1k)|224 |83.54|96.83|44.6 |9.1 |17.6 |1864 |
|[resnet152.a1h_in1k](https://huggingface.co/timm/resnet152.a1h_in1k)|288 |83.46|96.54|60.2 |19.1 |37.3 |904 |
|[resnext101_32x16d.fb_swsl_ig1b_ft_in1k](https://huggingface.co/timm/resnext101_32x16d.fb_swsl_ig1b_ft_in1k)|224 |83.35|96.85|194.0 |36.3 |51.2 |582 |
|[resnet200d.ra2_in1k](https://huggingface.co/timm/resnet200d.ra2_in1k)|256 |83.23|96.53|64.7 |20.0 |43.1 |809 |
|[resnext101_32x4d.fb_swsl_ig1b_ft_in1k](https://huggingface.co/timm/resnext101_32x4d.fb_swsl_ig1b_ft_in1k)|224 |83.22|96.75|44.2 |8.0 |21.2 |1814 |
|[resnext101_64x4d.c1_in1k](https://huggingface.co/timm/resnext101_64x4d.c1_in1k)|288 |83.16|96.38|83.5 |25.7 |51.6 |590 |
|[resnet152d.ra2_in1k](https://huggingface.co/timm/resnet152d.ra2_in1k)|256 |83.14|96.38|60.2 |15.4 |30.5 |1096 |
|[resnet101d.ra2_in1k](https://huggingface.co/timm/resnet101d.ra2_in1k)|320 |83.02|96.45|44.6 |16.5 |34.8 |992 |
|[ecaresnet101d.miil_in1k](https://huggingface.co/timm/ecaresnet101d.miil_in1k)|288 |82.98|96.54|44.6 |13.4 |28.2 |1077 |
|[resnext101_64x4d.tv_in1k](https://huggingface.co/timm/resnext101_64x4d.tv_in1k)|224 |82.98|96.25|83.5 |15.5 |31.2 |989 |
|[resnetrs152.tf_in1k](https://huggingface.co/timm/resnetrs152.tf_in1k)|256 |82.86|96.28|86.6 |15.6 |30.8 |951 |
|[resnext101_32x8d.tv2_in1k](https://huggingface.co/timm/resnext101_32x8d.tv2_in1k)|224 |82.83|96.22|88.8 |16.5 |31.2 |1099 |
|[resnet152.a1h_in1k](https://huggingface.co/timm/resnet152.a1h_in1k)|224 |82.8 |96.13|60.2 |11.6 |22.6 |1486 |
|[resnet101.a1h_in1k](https://huggingface.co/timm/resnet101.a1h_in1k)|288 |82.8 |96.32|44.6 |13.0 |26.8 |1291 |
|[resnet152.a1_in1k](https://huggingface.co/timm/resnet152.a1_in1k)|288 |82.74|95.71|60.2 |19.1 |37.3 |905 |
|[resnext101_32x8d.fb_wsl_ig1b_ft_in1k](https://huggingface.co/timm/resnext101_32x8d.fb_wsl_ig1b_ft_in1k)|224 |82.69|96.63|88.8 |16.5 |31.2 |1100 |
|[resnet152.a2_in1k](https://huggingface.co/timm/resnet152.a2_in1k)|288 |82.62|95.75|60.2 |19.1 |37.3 |904 |
|[resnetaa50d.sw_in12k_ft_in1k](https://huggingface.co/timm/resnetaa50d.sw_in12k_ft_in1k)|288 |82.61|96.49|25.6 |8.9 |20.6 |1729 |
|[resnet61q.ra2_in1k](https://huggingface.co/timm/resnet61q.ra2_in1k)|288 |82.53|96.13|36.8 |9.9 |21.5 |1773 |
|[wide_resnet101_2.tv2_in1k](https://huggingface.co/timm/wide_resnet101_2.tv2_in1k)|224 |82.5 |96.02|126.9 |22.8 |21.2 |1078 |
|[resnext101_64x4d.c1_in1k](https://huggingface.co/timm/resnext101_64x4d.c1_in1k)|224 |82.46|95.92|83.5 |15.5 |31.2 |987 |
|[resnet51q.ra2_in1k](https://huggingface.co/timm/resnet51q.ra2_in1k)|288 |82.36|96.18|35.7 |8.1 |20.9 |1964 |
|[ecaresnet50t.ra2_in1k](https://huggingface.co/timm/ecaresnet50t.ra2_in1k)|320 |82.35|96.14|25.6 |8.8 |24.1 |1386 |
|[resnet101.a1_in1k](https://huggingface.co/timm/resnet101.a1_in1k)|288 |82.31|95.63|44.6 |13.0 |26.8 |1291 |
|[resnetrs101.tf_in1k](https://huggingface.co/timm/resnetrs101.tf_in1k)|288 |82.29|96.01|63.6 |13.6 |28.5 |1078 |
|[resnet152.tv2_in1k](https://huggingface.co/timm/resnet152.tv2_in1k)|224 |82.29|96.0 |60.2 |11.6 |22.6 |1484 |
|[wide_resnet50_2.racm_in1k](https://huggingface.co/timm/wide_resnet50_2.racm_in1k)|288 |82.27|96.06|68.9 |18.9 |23.8 |1176 |
|[resnet101d.ra2_in1k](https://huggingface.co/timm/resnet101d.ra2_in1k)|256 |82.26|96.07|44.6 |10.6 |22.2 |1542 |
|[resnet101.a2_in1k](https://huggingface.co/timm/resnet101.a2_in1k)|288 |82.24|95.73|44.6 |13.0 |26.8 |1290 |
|[seresnext50_32x4d.racm_in1k](https://huggingface.co/timm/seresnext50_32x4d.racm_in1k)|288 |82.2 |96.14|27.6 |7.0 |23.8 |1547 |
|[ecaresnet101d.miil_in1k](https://huggingface.co/timm/ecaresnet101d.miil_in1k)|224 |82.18|96.05|44.6 |8.1 |17.1 |1771 |
|[resnext50_32x4d.fb_swsl_ig1b_ft_in1k](https://huggingface.co/timm/resnext50_32x4d.fb_swsl_ig1b_ft_in1k)|224 |82.17|96.22|25.0 |4.3 |14.4 |2943 |
|[ecaresnet50t.a1_in1k](https://huggingface.co/timm/ecaresnet50t.a1_in1k)|288 |82.12|95.65|25.6 |7.1 |19.6 |1704 |
|[resnext50_32x4d.a1h_in1k](https://huggingface.co/timm/resnext50_32x4d.a1h_in1k)|288 |82.03|95.94|25.0 |7.0 |23.8 |1745 |
|[ecaresnet101d_pruned.miil_in1k](https://huggingface.co/timm/ecaresnet101d_pruned.miil_in1k)|288 |82.0 |96.15|24.9 |5.8 |12.7 |1787 |
|[resnet61q.ra2_in1k](https://huggingface.co/timm/resnet61q.ra2_in1k)|256 |81.99|95.85|36.8 |7.8 |17.0 |2230 |
|[resnext101_32x8d.tv2_in1k](https://huggingface.co/timm/resnext101_32x8d.tv2_in1k)|176 |81.98|95.72|88.8 |10.3 |19.4 |1768 |
|[resnet152.a1_in1k](https://huggingface.co/timm/resnet152.a1_in1k)|224 |81.97|95.24|60.2 |11.6 |22.6 |1486 |
|[resnet101.a1h_in1k](https://huggingface.co/timm/resnet101.a1h_in1k)|224 |81.93|95.75|44.6 |7.8 |16.2 |2122 |
|[resnet101.tv2_in1k](https://huggingface.co/timm/resnet101.tv2_in1k)|224 |81.9 |95.77|44.6 |7.8 |16.2 |2118 |
|[resnext101_32x16d.fb_ssl_yfcc100m_ft_in1k](https://huggingface.co/timm/resnext101_32x16d.fb_ssl_yfcc100m_ft_in1k)|224 |81.84|96.1 |194.0 |36.3 |51.2 |583 |
|[resnet51q.ra2_in1k](https://huggingface.co/timm/resnet51q.ra2_in1k)|256 |81.78|95.94|35.7 |6.4 |16.6 |2471 |
|[resnet152.a2_in1k](https://huggingface.co/timm/resnet152.a2_in1k)|224 |81.77|95.22|60.2 |11.6 |22.6 |1485 |
|[resnetaa50d.sw_in12k_ft_in1k](https://huggingface.co/timm/resnetaa50d.sw_in12k_ft_in1k)|224 |81.74|96.06|25.6 |5.4 |12.4 |2813 |
|[ecaresnet50t.a2_in1k](https://huggingface.co/timm/ecaresnet50t.a2_in1k)|288 |81.65|95.54|25.6 |7.1 |19.6 |1703 |
|[ecaresnet50d.miil_in1k](https://huggingface.co/timm/ecaresnet50d.miil_in1k)|288 |81.64|95.88|25.6 |7.2 |19.7 |1694 |
|[resnext101_32x8d.fb_ssl_yfcc100m_ft_in1k](https://huggingface.co/timm/resnext101_32x8d.fb_ssl_yfcc100m_ft_in1k)|224 |81.62|96.04|88.8 |16.5 |31.2 |1101 |
|[wide_resnet50_2.tv2_in1k](https://huggingface.co/timm/wide_resnet50_2.tv2_in1k)|224 |81.61|95.76|68.9 |11.4 |14.4 |1930 |
|[resnetaa50.a1h_in1k](https://huggingface.co/timm/resnetaa50.a1h_in1k)|288 |81.61|95.83|25.6 |8.5 |19.2 |1868 |
|[resnet101.a1_in1k](https://huggingface.co/timm/resnet101.a1_in1k)|224 |81.5 |95.16|44.6 |7.8 |16.2 |2125 |
|[resnext50_32x4d.a1_in1k](https://huggingface.co/timm/resnext50_32x4d.a1_in1k)|288 |81.48|95.16|25.0 |7.0 |23.8 |1745 |
|[gcresnet50t.ra2_in1k](https://huggingface.co/timm/gcresnet50t.ra2_in1k)|288 |81.47|95.71|25.9 |6.9 |18.6 |2071 |
|[wide_resnet50_2.racm_in1k](https://huggingface.co/timm/wide_resnet50_2.racm_in1k)|224 |81.45|95.53|68.9 |11.4 |14.4 |1929 |
|[resnet50d.a1_in1k](https://huggingface.co/timm/resnet50d.a1_in1k)|288 |81.44|95.22|25.6 |7.2 |19.7 |1908 |
|[ecaresnet50t.ra2_in1k](https://huggingface.co/timm/ecaresnet50t.ra2_in1k)|256 |81.44|95.67|25.6 |5.6 |15.4 |2168 |
|[ecaresnetlight.miil_in1k](https://huggingface.co/timm/ecaresnetlight.miil_in1k)|288 |81.4 |95.82|30.2 |6.8 |13.9 |2132 |
|[resnet50d.ra2_in1k](https://huggingface.co/timm/resnet50d.ra2_in1k)|288 |81.37|95.74|25.6 |7.2 |19.7 |1910 |
|[resnet101.a2_in1k](https://huggingface.co/timm/resnet101.a2_in1k)|224 |81.32|95.19|44.6 |7.8 |16.2 |2125 |
|[seresnet50.ra2_in1k](https://huggingface.co/timm/seresnet50.ra2_in1k)|288 |81.3 |95.65|28.1 |6.8 |18.4 |1803 |
|[resnext50_32x4d.a2_in1k](https://huggingface.co/timm/resnext50_32x4d.a2_in1k)|288 |81.3 |95.11|25.0 |7.0 |23.8 |1746 |
|[seresnext50_32x4d.racm_in1k](https://huggingface.co/timm/seresnext50_32x4d.racm_in1k)|224 |81.27|95.62|27.6 |4.3 |14.4 |2591 |
|[ecaresnet50t.a1_in1k](https://huggingface.co/timm/ecaresnet50t.a1_in1k)|224 |81.26|95.16|25.6 |4.3 |11.8 |2823 |
|[gcresnext50ts.ch_in1k](https://huggingface.co/timm/gcresnext50ts.ch_in1k)|288 |81.23|95.54|15.7 |4.8 |19.6 |2117 |
|[senet154.gluon_in1k](https://huggingface.co/timm/senet154.gluon_in1k)|224 |81.23|95.35|115.1 |20.8 |38.7 |545 |
|[resnet50.a1_in1k](https://huggingface.co/timm/resnet50.a1_in1k)|288 |81.22|95.11|25.6 |6.8 |18.4 |2089 |
|[resnet50_gn.a1h_in1k](https://huggingface.co/timm/resnet50_gn.a1h_in1k)|288 |81.22|95.63|25.6 |6.8 |18.4 |676 |
|[resnet50d.a2_in1k](https://huggingface.co/timm/resnet50d.a2_in1k)|288 |81.18|95.09|25.6 |7.2 |19.7 |1908 |
|[resnet50.fb_swsl_ig1b_ft_in1k](https://huggingface.co/timm/resnet50.fb_swsl_ig1b_ft_in1k)|224 |81.18|95.98|25.6 |4.1 |11.1 |3455 |
|[resnext50_32x4d.tv2_in1k](https://huggingface.co/timm/resnext50_32x4d.tv2_in1k)|224 |81.17|95.34|25.0 |4.3 |14.4 |2933 |
|[resnext50_32x4d.a1h_in1k](https://huggingface.co/timm/resnext50_32x4d.a1h_in1k)|224 |81.1 |95.33|25.0 |4.3 |14.4 |2934 |
|[seresnet50.a2_in1k](https://huggingface.co/timm/seresnet50.a2_in1k)|288 |81.1 |95.23|28.1 |6.8 |18.4 |1801 |
|[seresnet50.a1_in1k](https://huggingface.co/timm/seresnet50.a1_in1k)|288 |81.1 |95.12|28.1 |6.8 |18.4 |1799 |
|[resnet152s.gluon_in1k](https://huggingface.co/timm/resnet152s.gluon_in1k)|224 |81.02|95.41|60.3 |12.9 |25.0 |1347 |
|[resnet50.d_in1k](https://huggingface.co/timm/resnet50.d_in1k)|288 |80.97|95.44|25.6 |6.8 |18.4 |2085 |
|[gcresnet50t.ra2_in1k](https://huggingface.co/timm/gcresnet50t.ra2_in1k)|256 |80.94|95.45|25.9 |5.4 |14.7 |2571 |
|[resnext101_32x4d.fb_ssl_yfcc100m_ft_in1k](https://huggingface.co/timm/resnext101_32x4d.fb_ssl_yfcc100m_ft_in1k)|224 |80.93|95.73|44.2 |8.0 |21.2 |1814 |
|[resnet50.c1_in1k](https://huggingface.co/timm/resnet50.c1_in1k)|288 |80.91|95.55|25.6 |6.8 |18.4 |2084 |
|[seresnext101_32x4d.gluon_in1k](https://huggingface.co/timm/seresnext101_32x4d.gluon_in1k)|224 |80.9 |95.31|49.0 |8.0 |21.3 |1585 |
|[seresnext101_64x4d.gluon_in1k](https://huggingface.co/timm/seresnext101_64x4d.gluon_in1k)|224 |80.9 |95.3 |88.2 |15.5 |31.2 |918 |
|[resnet50.c2_in1k](https://huggingface.co/timm/resnet50.c2_in1k)|288 |80.86|95.52|25.6 |6.8 |18.4 |2085 |
|[resnet50.tv2_in1k](https://huggingface.co/timm/resnet50.tv2_in1k)|224 |80.85|95.43|25.6 |4.1 |11.1 |3450 |
|[ecaresnet50t.a2_in1k](https://huggingface.co/timm/ecaresnet50t.a2_in1k)|224 |80.84|95.02|25.6 |4.3 |11.8 |2821 |
|[ecaresnet101d_pruned.miil_in1k](https://huggingface.co/timm/ecaresnet101d_pruned.miil_in1k)|224 |80.79|95.62|24.9 |3.5 |7.7 |2961 |
|[seresnet33ts.ra2_in1k](https://huggingface.co/timm/seresnet33ts.ra2_in1k)|288 |80.79|95.36|19.8 |6.0 |14.8 |2506 |
|[ecaresnet50d_pruned.miil_in1k](https://huggingface.co/timm/ecaresnet50d_pruned.miil_in1k)|288 |80.79|95.58|19.9 |4.2 |10.6 |2349 |
|[resnet50.a2_in1k](https://huggingface.co/timm/resnet50.a2_in1k)|288 |80.78|94.99|25.6 |6.8 |18.4 |2088 |
|[resnet50.b1k_in1k](https://huggingface.co/timm/resnet50.b1k_in1k)|288 |80.71|95.43|25.6 |6.8 |18.4 |2087 |
|[resnext50_32x4d.ra_in1k](https://huggingface.co/timm/resnext50_32x4d.ra_in1k)|288 |80.7 |95.39|25.0 |7.0 |23.8 |1749 |
|[resnetrs101.tf_in1k](https://huggingface.co/timm/resnetrs101.tf_in1k)|192 |80.69|95.24|63.6 |6.0 |12.7 |2270 |
|[resnet50d.a1_in1k](https://huggingface.co/timm/resnet50d.a1_in1k)|224 |80.68|94.71|25.6 |4.4 |11.9 |3162 |
|[eca_resnet33ts.ra2_in1k](https://huggingface.co/timm/eca_resnet33ts.ra2_in1k)|288 |80.68|95.36|19.7 |6.0 |14.8 |2637 |
|[resnet50.a1h_in1k](https://huggingface.co/timm/resnet50.a1h_in1k)|224 |80.67|95.3 |25.6 |4.1 |11.1 |3452 |
|[resnext50d_32x4d.bt_in1k](https://huggingface.co/timm/resnext50d_32x4d.bt_in1k)|288 |80.67|95.42|25.0 |7.4 |25.1 |1626 |
|[resnetaa50.a1h_in1k](https://huggingface.co/timm/resnetaa50.a1h_in1k)|224 |80.63|95.21|25.6 |5.2 |11.6 |3034 |
|[ecaresnet50d.miil_in1k](https://huggingface.co/timm/ecaresnet50d.miil_in1k)|224 |80.61|95.32|25.6 |4.4 |11.9 |2813 |
|[resnext101_64x4d.gluon_in1k](https://huggingface.co/timm/resnext101_64x4d.gluon_in1k)|224 |80.61|94.99|83.5 |15.5 |31.2 |989 |
|[gcresnet33ts.ra2_in1k](https://huggingface.co/timm/gcresnet33ts.ra2_in1k)|288 |80.6 |95.31|19.9 |6.0 |14.8 |2578 |
|[gcresnext50ts.ch_in1k](https://huggingface.co/timm/gcresnext50ts.ch_in1k)|256 |80.57|95.17|15.7 |3.8 |15.5 |2710 |
|[resnet152.a3_in1k](https://huggingface.co/timm/resnet152.a3_in1k)|224 |80.56|95.0 |60.2 |11.6 |22.6 |1483 |
|[resnet50d.ra2_in1k](https://huggingface.co/timm/resnet50d.ra2_in1k)|224 |80.53|95.16|25.6 |4.4 |11.9 |3164 |
|[resnext50_32x4d.a1_in1k](https://huggingface.co/timm/resnext50_32x4d.a1_in1k)|224 |80.53|94.46|25.0 |4.3 |14.4 |2930 |
|[wide_resnet101_2.tv2_in1k](https://huggingface.co/timm/wide_resnet101_2.tv2_in1k)|176 |80.48|94.98|126.9 |14.3 |13.2 |1719 |
|[resnet152d.gluon_in1k](https://huggingface.co/timm/resnet152d.gluon_in1k)|224 |80.47|95.2 |60.2 |11.8 |23.4 |1428 |
|[resnet50.b2k_in1k](https://huggingface.co/timm/resnet50.b2k_in1k)|288 |80.45|95.32|25.6 |6.8 |18.4 |2086 |
|[ecaresnetlight.miil_in1k](https://huggingface.co/timm/ecaresnetlight.miil_in1k)|224 |80.45|95.24|30.2 |4.1 |8.4 |3530 |
|[resnext50_32x4d.a2_in1k](https://huggingface.co/timm/resnext50_32x4d.a2_in1k)|224 |80.45|94.63|25.0 |4.3 |14.4 |2936 |
|[wide_resnet50_2.tv2_in1k](https://huggingface.co/timm/wide_resnet50_2.tv2_in1k)|176 |80.43|95.09|68.9 |7.3 |9.0 |3015 |
|[resnet101d.gluon_in1k](https://huggingface.co/timm/resnet101d.gluon_in1k)|224 |80.42|95.01|44.6 |8.1 |17.0 |2007 |
|[resnet50.a1_in1k](https://huggingface.co/timm/resnet50.a1_in1k)|224 |80.38|94.6 |25.6 |4.1 |11.1 |3461 |
|[seresnet33ts.ra2_in1k](https://huggingface.co/timm/seresnet33ts.ra2_in1k)|256 |80.36|95.1 |19.8 |4.8 |11.7 |3267 |
|[resnext101_32x4d.gluon_in1k](https://huggingface.co/timm/resnext101_32x4d.gluon_in1k)|224 |80.34|94.93|44.2 |8.0 |21.2 |1814 |
|[resnext50_32x4d.fb_ssl_yfcc100m_ft_in1k](https://huggingface.co/timm/resnext50_32x4d.fb_ssl_yfcc100m_ft_in1k)|224 |80.32|95.4 |25.0 |4.3 |14.4 |2941 |
|[resnet101s.gluon_in1k](https://huggingface.co/timm/resnet101s.gluon_in1k)|224 |80.28|95.16|44.7 |9.2 |18.6 |1851 |
|[seresnet50.ra2_in1k](https://huggingface.co/timm/seresnet50.ra2_in1k)|224 |80.26|95.08|28.1 |4.1 |11.1 |2972 |
|[resnetblur50.bt_in1k](https://huggingface.co/timm/resnetblur50.bt_in1k)|288 |80.24|95.24|25.6 |8.5 |19.9 |1523 |
|[resnet50d.a2_in1k](https://huggingface.co/timm/resnet50d.a2_in1k)|224 |80.22|94.63|25.6 |4.4 |11.9 |3162 |
|[resnet152.tv2_in1k](https://huggingface.co/timm/resnet152.tv2_in1k)|176 |80.2 |94.64|60.2 |7.2 |14.0 |2346 |
|[seresnet50.a2_in1k](https://huggingface.co/timm/seresnet50.a2_in1k)|224 |80.08|94.74|28.1 |4.1 |11.1 |2969 |
|[eca_resnet33ts.ra2_in1k](https://huggingface.co/timm/eca_resnet33ts.ra2_in1k)|256 |80.08|94.97|19.7 |4.8 |11.7 |3284 |
|[gcresnet33ts.ra2_in1k](https://huggingface.co/timm/gcresnet33ts.ra2_in1k)|256 |80.06|94.99|19.9 |4.8 |11.7 |3216 |
|[resnet50_gn.a1h_in1k](https://huggingface.co/timm/resnet50_gn.a1h_in1k)|224 |80.06|94.95|25.6 |4.1 |11.1 |1109 |
|[seresnet50.a1_in1k](https://huggingface.co/timm/seresnet50.a1_in1k)|224 |80.02|94.71|28.1 |4.1 |11.1 |2962 |
|[resnet50.ram_in1k](https://huggingface.co/timm/resnet50.ram_in1k)|288 |79.97|95.05|25.6 |6.8 |18.4 |2086 |
|[resnet152c.gluon_in1k](https://huggingface.co/timm/resnet152c.gluon_in1k)|224 |79.92|94.84|60.2 |11.8 |23.4 |1455 |
|[seresnext50_32x4d.gluon_in1k](https://huggingface.co/timm/seresnext50_32x4d.gluon_in1k)|224 |79.91|94.82|27.6 |4.3 |14.4 |2591 |
|[resnet50.d_in1k](https://huggingface.co/timm/resnet50.d_in1k)|224 |79.91|94.67|25.6 |4.1 |11.1 |3456 |
|[resnet101.tv2_in1k](https://huggingface.co/timm/resnet101.tv2_in1k)|176 |79.9 |94.6 |44.6 |4.9 |10.1 |3341 |
|[resnetrs50.tf_in1k](https://huggingface.co/timm/resnetrs50.tf_in1k)|224 |79.89|94.97|35.7 |4.5 |12.1 |2774 |
|[resnet50.c2_in1k](https://huggingface.co/timm/resnet50.c2_in1k)|224 |79.88|94.87|25.6 |4.1 |11.1 |3455 |
|[ecaresnet26t.ra2_in1k](https://huggingface.co/timm/ecaresnet26t.ra2_in1k)|320 |79.86|95.07|16.0 |5.2 |16.4 |2168 |
|[resnet50.a2_in1k](https://huggingface.co/timm/resnet50.a2_in1k)|224 |79.85|94.56|25.6 |4.1 |11.1 |3460 |
|[resnet50.ra_in1k](https://huggingface.co/timm/resnet50.ra_in1k)|288 |79.83|94.97|25.6 |6.8 |18.4 |2087 |
|[resnet101.a3_in1k](https://huggingface.co/timm/resnet101.a3_in1k)|224 |79.82|94.62|44.6 |7.8 |16.2 |2114 |
|[resnext50_32x4d.ra_in1k](https://huggingface.co/timm/resnext50_32x4d.ra_in1k)|224 |79.76|94.6 |25.0 |4.3 |14.4 |2943 |
|[resnet50.c1_in1k](https://huggingface.co/timm/resnet50.c1_in1k)|224 |79.74|94.95|25.6 |4.1 |11.1 |3455 |
|[ecaresnet50d_pruned.miil_in1k](https://huggingface.co/timm/ecaresnet50d_pruned.miil_in1k)|224 |79.74|94.87|19.9 |2.5 |6.4 |3929 |
|[resnet33ts.ra2_in1k](https://huggingface.co/timm/resnet33ts.ra2_in1k)|288 |79.71|94.83|19.7 |6.0 |14.8 |2710 |
|[resnet152.gluon_in1k](https://huggingface.co/timm/resnet152.gluon_in1k)|224 |79.68|94.74|60.2 |11.6 |22.6 |1486 |
|[resnext50d_32x4d.bt_in1k](https://huggingface.co/timm/resnext50d_32x4d.bt_in1k)|224 |79.67|94.87|25.0 |4.5 |15.2 |2729 |
|[resnet50.bt_in1k](https://huggingface.co/timm/resnet50.bt_in1k)|288 |79.63|94.91|25.6 |6.8 |18.4 |2086 |
|[ecaresnet50t.a3_in1k](https://huggingface.co/timm/ecaresnet50t.a3_in1k)|224 |79.56|94.72|25.6 |4.3 |11.8 |2805 |
|[resnet101c.gluon_in1k](https://huggingface.co/timm/resnet101c.gluon_in1k)|224 |79.53|94.58|44.6 |8.1 |17.0 |2062 |
|[resnet50.b1k_in1k](https://huggingface.co/timm/resnet50.b1k_in1k)|224 |79.52|94.61|25.6 |4.1 |11.1 |3459 |
|[resnet50.tv2_in1k](https://huggingface.co/timm/resnet50.tv2_in1k)|176 |79.42|94.64|25.6 |2.6 |6.9 |5397 |
|[resnet32ts.ra2_in1k](https://huggingface.co/timm/resnet32ts.ra2_in1k)|288 |79.4 |94.66|18.0 |5.9 |14.6 |2752 |
|[resnet50.b2k_in1k](https://huggingface.co/timm/resnet50.b2k_in1k)|224 |79.38|94.57|25.6 |4.1 |11.1 |3459 |
|[resnext50_32x4d.tv2_in1k](https://huggingface.co/timm/resnext50_32x4d.tv2_in1k)|176 |79.37|94.3 |25.0 |2.7 |9.0 |4577 |
|[resnext50_32x4d.gluon_in1k](https://huggingface.co/timm/resnext50_32x4d.gluon_in1k)|224 |79.36|94.43|25.0 |4.3 |14.4 |2942 |
|[resnext101_32x8d.tv_in1k](https://huggingface.co/timm/resnext101_32x8d.tv_in1k)|224 |79.31|94.52|88.8 |16.5 |31.2 |1100 |
|[resnet101.gluon_in1k](https://huggingface.co/timm/resnet101.gluon_in1k)|224 |79.31|94.53|44.6 |7.8 |16.2 |2125 |
|[resnetblur50.bt_in1k](https://huggingface.co/timm/resnetblur50.bt_in1k)|224 |79.31|94.63|25.6 |5.2 |12.0 |2524 |
|[resnet50.a1h_in1k](https://huggingface.co/timm/resnet50.a1h_in1k)|176 |79.27|94.49|25.6 |2.6 |6.9 |5404 |
|[resnext50_32x4d.a3_in1k](https://huggingface.co/timm/resnext50_32x4d.a3_in1k)|224 |79.25|94.31|25.0 |4.3 |14.4 |2931 |
|[resnet50.fb_ssl_yfcc100m_ft_in1k](https://huggingface.co/timm/resnet50.fb_ssl_yfcc100m_ft_in1k)|224 |79.22|94.84|25.6 |4.1 |11.1 |3451 |
|[resnet33ts.ra2_in1k](https://huggingface.co/timm/resnet33ts.ra2_in1k)|256 |79.21|94.56|19.7 |4.8 |11.7 |3392 |
|[resnet50d.gluon_in1k](https://huggingface.co/timm/resnet50d.gluon_in1k)|224 |79.07|94.48|25.6 |4.4 |11.9 |3162 |
|[resnet50.ram_in1k](https://huggingface.co/timm/resnet50.ram_in1k)|224 |79.03|94.38|25.6 |4.1 |11.1 |3453 |
|[resnet50.am_in1k](https://huggingface.co/timm/resnet50.am_in1k)|224 |79.01|94.39|25.6 |4.1 |11.1 |3461 |
|[resnet32ts.ra2_in1k](https://huggingface.co/timm/resnet32ts.ra2_in1k)|256 |79.01|94.37|18.0 |4.6 |11.6 |3440 |
|[ecaresnet26t.ra2_in1k](https://huggingface.co/timm/ecaresnet26t.ra2_in1k)|256 |78.9 |94.54|16.0 |3.4 |10.5 |3421 |
|[resnet152.a3_in1k](https://huggingface.co/timm/resnet152.a3_in1k)|160 |78.89|94.11|60.2 |5.9 |11.5 |2745 |
|[wide_resnet101_2.tv_in1k](https://huggingface.co/timm/wide_resnet101_2.tv_in1k)|224 |78.84|94.28|126.9 |22.8 |21.2 |1079 |
|[seresnext26d_32x4d.bt_in1k](https://huggingface.co/timm/seresnext26d_32x4d.bt_in1k)|288 |78.83|94.24|16.8 |4.5 |16.8 |2251 |
|[resnet50.ra_in1k](https://huggingface.co/timm/resnet50.ra_in1k)|224 |78.81|94.32|25.6 |4.1 |11.1 |3454 |
|[seresnext26t_32x4d.bt_in1k](https://huggingface.co/timm/seresnext26t_32x4d.bt_in1k)|288 |78.74|94.33|16.8 |4.5 |16.7 |2264 |
|[resnet50s.gluon_in1k](https://huggingface.co/timm/resnet50s.gluon_in1k)|224 |78.72|94.23|25.7 |5.5 |13.5 |2796 |
|[resnet50d.a3_in1k](https://huggingface.co/timm/resnet50d.a3_in1k)|224 |78.71|94.24|25.6 |4.4 |11.9 |3154 |
|[wide_resnet50_2.tv_in1k](https://huggingface.co/timm/wide_resnet50_2.tv_in1k)|224 |78.47|94.09|68.9 |11.4 |14.4 |1934 |
|[resnet50.bt_in1k](https://huggingface.co/timm/resnet50.bt_in1k)|224 |78.46|94.27|25.6 |4.1 |11.1 |3454 |
|[resnet34d.ra2_in1k](https://huggingface.co/timm/resnet34d.ra2_in1k)|288 |78.43|94.35|21.8 |6.5 |7.5 |3291 |
|[gcresnext26ts.ch_in1k](https://huggingface.co/timm/gcresnext26ts.ch_in1k)|288 |78.42|94.04|10.5 |3.1 |13.3 |3226 |
|[resnet26t.ra2_in1k](https://huggingface.co/timm/resnet26t.ra2_in1k)|320 |78.33|94.13|16.0 |5.2 |16.4 |2391 |
|[resnet152.tv_in1k](https://huggingface.co/timm/resnet152.tv_in1k)|224 |78.32|94.04|60.2 |11.6 |22.6 |1487 |
|[seresnext26ts.ch_in1k](https://huggingface.co/timm/seresnext26ts.ch_in1k)|288 |78.28|94.1 |10.4 |3.1 |13.3 |3062 |
|[bat_resnext26ts.ch_in1k](https://huggingface.co/timm/bat_resnext26ts.ch_in1k)|256 |78.25|94.1 |10.7 |2.5 |12.5 |3393 |
|[resnet50.a3_in1k](https://huggingface.co/timm/resnet50.a3_in1k)|224 |78.06|93.78|25.6 |4.1 |11.1 |3450 |
|[resnet50c.gluon_in1k](https://huggingface.co/timm/resnet50c.gluon_in1k)|224 |78.0 |93.99|25.6 |4.4 |11.9 |3286 |
|[eca_resnext26ts.ch_in1k](https://huggingface.co/timm/eca_resnext26ts.ch_in1k)|288 |78.0 |93.91|10.3 |3.1 |13.3 |3297 |
|[seresnext26t_32x4d.bt_in1k](https://huggingface.co/timm/seresnext26t_32x4d.bt_in1k)|224 |77.98|93.75|16.8 |2.7 |10.1 |3841 |
|[resnet34.a1_in1k](https://huggingface.co/timm/resnet34.a1_in1k)|288 |77.92|93.77|21.8 |6.1 |6.2 |3609 |
|[resnet101.a3_in1k](https://huggingface.co/timm/resnet101.a3_in1k)|160 |77.88|93.71|44.6 |4.0 |8.3 |3926 |
|[resnet26t.ra2_in1k](https://huggingface.co/timm/resnet26t.ra2_in1k)|256 |77.87|93.84|16.0 |3.4 |10.5 |3772 |
|[seresnext26ts.ch_in1k](https://huggingface.co/timm/seresnext26ts.ch_in1k)|256 |77.86|93.79|10.4 |2.4 |10.5 |4263 |
|[resnetrs50.tf_in1k](https://huggingface.co/timm/resnetrs50.tf_in1k)|160 |77.82|93.81|35.7 |2.3 |6.2 |5238 |
|[gcresnext26ts.ch_in1k](https://huggingface.co/timm/gcresnext26ts.ch_in1k)|256 |77.81|93.82|10.5 |2.4 |10.5 |4183 |
|[ecaresnet50t.a3_in1k](https://huggingface.co/timm/ecaresnet50t.a3_in1k)|160 |77.79|93.6 |25.6 |2.2 |6.0 |5329 |
|[resnext50_32x4d.a3_in1k](https://huggingface.co/timm/resnext50_32x4d.a3_in1k)|160 |77.73|93.32|25.0 |2.2 |7.4 |5576 |
|[resnext50_32x4d.tv_in1k](https://huggingface.co/timm/resnext50_32x4d.tv_in1k)|224 |77.61|93.7 |25.0 |4.3 |14.4 |2944 |
|[seresnext26d_32x4d.bt_in1k](https://huggingface.co/timm/seresnext26d_32x4d.bt_in1k)|224 |77.59|93.61|16.8 |2.7 |10.2 |3807 |
|[resnet50.gluon_in1k](https://huggingface.co/timm/resnet50.gluon_in1k)|224 |77.58|93.72|25.6 |4.1 |11.1 |3455 |
|[eca_resnext26ts.ch_in1k](https://huggingface.co/timm/eca_resnext26ts.ch_in1k)|256 |77.44|93.56|10.3 |2.4 |10.5 |4284 |
|[resnet26d.bt_in1k](https://huggingface.co/timm/resnet26d.bt_in1k)|288 |77.41|93.63|16.0 |4.3 |13.5 |2907 |
|[resnet101.tv_in1k](https://huggingface.co/timm/resnet101.tv_in1k)|224 |77.38|93.54|44.6 |7.8 |16.2 |2125 |
|[resnet50d.a3_in1k](https://huggingface.co/timm/resnet50d.a3_in1k)|160 |77.22|93.27|25.6 |2.2 |6.1 |5982 |
|[resnext26ts.ra2_in1k](https://huggingface.co/timm/resnext26ts.ra2_in1k)|288 |77.17|93.47|10.3 |3.1 |13.3 |3392 |
|[resnet34.a2_in1k](https://huggingface.co/timm/resnet34.a2_in1k)|288 |77.15|93.27|21.8 |6.1 |6.2 |3615 |
|[resnet34d.ra2_in1k](https://huggingface.co/timm/resnet34d.ra2_in1k)|224 |77.1 |93.37|21.8 |3.9 |4.5 |5436 |
|[seresnet50.a3_in1k](https://huggingface.co/timm/seresnet50.a3_in1k)|224 |77.02|93.07|28.1 |4.1 |11.1 |2952 |
|[resnext26ts.ra2_in1k](https://huggingface.co/timm/resnext26ts.ra2_in1k)|256 |76.78|93.13|10.3 |2.4 |10.5 |4410 |
|[resnet26d.bt_in1k](https://huggingface.co/timm/resnet26d.bt_in1k)|224 |76.7 |93.17|16.0 |2.6 |8.2 |4859 |
|[resnet34.bt_in1k](https://huggingface.co/timm/resnet34.bt_in1k)|288 |76.5 |93.35|21.8 |6.1 |6.2 |3617 |
|[resnet34.a1_in1k](https://huggingface.co/timm/resnet34.a1_in1k)|224 |76.42|92.87|21.8 |3.7 |3.7 |5984 |
|[resnet26.bt_in1k](https://huggingface.co/timm/resnet26.bt_in1k)|288 |76.35|93.18|16.0 |3.9 |12.2 |3331 |
|[resnet50.tv_in1k](https://huggingface.co/timm/resnet50.tv_in1k)|224 |76.13|92.86|25.6 |4.1 |11.1 |3457 |
|[resnet50.a3_in1k](https://huggingface.co/timm/resnet50.a3_in1k)|160 |75.96|92.5 |25.6 |2.1 |5.7 |6490 |
|[resnet34.a2_in1k](https://huggingface.co/timm/resnet34.a2_in1k)|224 |75.52|92.44|21.8 |3.7 |3.7 |5991 |
|[resnet26.bt_in1k](https://huggingface.co/timm/resnet26.bt_in1k)|224 |75.3 |92.58|16.0 |2.4 |7.4 |5583 |
|[resnet34.bt_in1k](https://huggingface.co/timm/resnet34.bt_in1k)|224 |75.16|92.18|21.8 |3.7 |3.7 |5994 |
|[seresnet50.a3_in1k](https://huggingface.co/timm/seresnet50.a3_in1k)|160 |75.1 |92.08|28.1 |2.1 |5.7 |5513 |
|[resnet34.gluon_in1k](https://huggingface.co/timm/resnet34.gluon_in1k)|224 |74.57|91.98|21.8 |3.7 |3.7 |5984 |
|[resnet18d.ra2_in1k](https://huggingface.co/timm/resnet18d.ra2_in1k)|288 |73.81|91.83|11.7 |3.4 |5.4 |5196 |
|[resnet34.tv_in1k](https://huggingface.co/timm/resnet34.tv_in1k)|224 |73.32|91.42|21.8 |3.7 |3.7 |5979 |
|[resnet18.fb_swsl_ig1b_ft_in1k](https://huggingface.co/timm/resnet18.fb_swsl_ig1b_ft_in1k)|224 |73.28|91.73|11.7 |1.8 |2.5 |10213 |
|[resnet18.a1_in1k](https://huggingface.co/timm/resnet18.a1_in1k)|288 |73.16|91.03|11.7 |3.0 |4.1 |6050 |
|[resnet34.a3_in1k](https://huggingface.co/timm/resnet34.a3_in1k)|224 |72.98|91.11|21.8 |3.7 |3.7 |5967 |
|[resnet18.fb_ssl_yfcc100m_ft_in1k](https://huggingface.co/timm/resnet18.fb_ssl_yfcc100m_ft_in1k)|224 |72.6 |91.42|11.7 |1.8 |2.5 |10213 |
|[resnet18.a2_in1k](https://huggingface.co/timm/resnet18.a2_in1k)|288 |72.37|90.59|11.7 |3.0 |4.1 |6051 |
|[resnet14t.c3_in1k](https://huggingface.co/timm/resnet14t.c3_in1k)|224 |72.26|90.31|10.1 |1.7 |5.8 |7026 |
|[resnet18d.ra2_in1k](https://huggingface.co/timm/resnet18d.ra2_in1k)|224 |72.26|90.68|11.7 |2.1 |3.3 |8707 |
|[resnet18.a1_in1k](https://huggingface.co/timm/resnet18.a1_in1k)|224 |71.49|90.07|11.7 |1.8 |2.5 |10187 |
|[resnet14t.c3_in1k](https://huggingface.co/timm/resnet14t.c3_in1k)|176 |71.31|89.69|10.1 |1.1 |3.6 |10970 |
|[resnet18.gluon_in1k](https://huggingface.co/timm/resnet18.gluon_in1k)|224 |70.84|89.76|11.7 |1.8 |2.5 |10210 |
|[resnet18.a2_in1k](https://huggingface.co/timm/resnet18.a2_in1k)|224 |70.64|89.47|11.7 |1.8 |2.5 |10194 |
|[resnet34.a3_in1k](https://huggingface.co/timm/resnet34.a3_in1k)|160 |70.56|89.52|21.8 |1.9 |1.9 |10737 |
|[resnet18.tv_in1k](https://huggingface.co/timm/resnet18.tv_in1k)|224 |69.76|89.07|11.7 |1.8 |2.5 |10205 |
|[resnet10t.c3_in1k](https://huggingface.co/timm/resnet10t.c3_in1k)|224 |68.34|88.03|5.4 |1.1 |2.4 |13079 |
|[resnet18.a3_in1k](https://huggingface.co/timm/resnet18.a3_in1k)|224 |68.25|88.17|11.7 |1.8 |2.5 |10167 |
|[resnet10t.c3_in1k](https://huggingface.co/timm/resnet10t.c3_in1k)|176 |66.71|86.96|5.4 |0.7 |1.5 |20327 |
|[resnet18.a3_in1k](https://huggingface.co/timm/resnet18.a3_in1k)|160 |65.66|86.26|11.7 |0.9 |1.3 |18229 |
## Citation
```bibtex
@inproceedings{wightman2021resnet,
title={ResNet strikes back: An improved training procedure in timm},
author={Wightman, Ross and Touvron, Hugo and Jegou, Herve},
booktitle={NeurIPS 2021 Workshop on ImageNet: Past, Present, and Future}
}
```
```bibtex
@misc{rw2019timm,
author = {Ross Wightman},
title = {PyTorch Image Models},
year = {2019},
publisher = {GitHub},
journal = {GitHub repository},
doi = {10.5281/zenodo.4414861},
howpublished = {\url{https://github.com/huggingface/pytorch-image-models}}
}
```
```bibtex
@article{He2015,
author = {Kaiming He and Xiangyu Zhang and Shaoqing Ren and Jian Sun},
title = {Deep Residual Learning for Image Recognition},
journal = {arXiv preprint arXiv:1512.03385},
year = {2015}
}
``` |
google/owlv2-base-patch16-ensemble | google | "2024-10-31T14:55:13Z" | 2,155,105 | 73 | transformers | [
"transformers",
"pytorch",
"safetensors",
"owlv2",
"zero-shot-object-detection",
"vision",
"arxiv:2306.09683",
"license:apache-2.0",
"region:us"
] | zero-shot-object-detection | "2023-10-13T09:27:09Z" | ---
license: apache-2.0
tags:
- vision
- zero-shot-object-detection
inference: false
---
# Model Card: OWLv2
## Model Details
The OWLv2 model (short for Open-World Localization) was proposed in [Scaling Open-Vocabulary Object Detection](https://arxiv.org/abs/2306.09683) by Matthias Minderer, Alexey Gritsenko, Neil Houlsby. OWLv2, like OWL-ViT, is a zero-shot text-conditioned object detection model that can be used to query an image with one or multiple text queries.
The model uses CLIP as its multi-modal backbone, with a ViT-like Transformer to get visual features and a causal language model to get the text features. To use CLIP for detection, OWL-ViT removes the final token pooling layer of the vision model and attaches a lightweight classification and box head to each transformer output token. Open-vocabulary classification is enabled by replacing the fixed classification layer weights with the class-name embeddings obtained from the text model. The authors first train CLIP from scratch and fine-tune it end-to-end with the classification and box heads on standard detection datasets using a bipartite matching loss. One or multiple text queries per image can be used to perform zero-shot text-conditioned object detection.
### Model Date
June 2023
### Model Type
The model uses a CLIP backbone with a ViT-B/16 Transformer architecture as an image encoder and uses a masked self-attention Transformer as a text encoder. These encoders are trained to maximize the similarity of (image, text) pairs via a contrastive loss. The CLIP backbone is trained from scratch and fine-tuned together with the box and class prediction heads with an object detection objective.
### Documents
- [OWLv2 Paper](https://arxiv.org/abs/2306.09683)
### Use with Transformers
```python
import requests
from PIL import Image
import torch
from transformers import Owlv2Processor, Owlv2ForObjectDetection
processor = Owlv2Processor.from_pretrained("google/owlv2-base-patch16-ensemble")
model = Owlv2ForObjectDetection.from_pretrained("google/owlv2-base-patch16-ensemble")
url = "http://images.cocodataset.org/val2017/000000039769.jpg"
image = Image.open(requests.get(url, stream=True).raw)
texts = [["a photo of a cat", "a photo of a dog"]]
inputs = processor(text=texts, images=image, return_tensors="pt")
with torch.no_grad():
outputs = model(**inputs)
# Target image sizes (height, width) to rescale box predictions [batch_size, 2]
target_sizes = torch.Tensor([image.size[::-1]])
# Convert outputs (bounding boxes and class logits) to Pascal VOC Format (xmin, ymin, xmax, ymax)
results = processor.post_process_object_detection(outputs=outputs, target_sizes=target_sizes, threshold=0.1)
i = 0 # Retrieve predictions for the first image for the corresponding text queries
text = texts[i]
boxes, scores, labels = results[i]["boxes"], results[i]["scores"], results[i]["labels"]
for box, score, label in zip(boxes, scores, labels):
box = [round(i, 2) for i in box.tolist()]
print(f"Detected {text[label]} with confidence {round(score.item(), 3)} at location {box}")
```
## Model Use
### Intended Use
The model is intended as a research output for research communities. We hope that this model will enable researchers to better understand and explore zero-shot, text-conditioned object detection. We also hope it can be used for interdisciplinary studies of the potential impact of such models, especially in areas that commonly require identifying objects whose label is unavailable during training.
#### Primary intended uses
The primary intended users of these models are AI researchers.
We primarily imagine the model will be used by researchers to better understand robustness, generalization, and other capabilities, biases, and constraints of computer vision models.
## Data
The CLIP backbone of the model was trained on publicly available image-caption data. This was done through a combination of crawling a handful of websites and using commonly-used pre-existing image datasets such as [YFCC100M](http://projects.dfki.uni-kl.de/yfcc100m/). A large portion of the data comes from our crawling of the internet. This means that the data is more representative of people and societies most connected to the internet. The prediction heads of OWL-ViT, along with the CLIP backbone, are fine-tuned on publicly available object detection datasets such as [COCO](https://cocodataset.org/#home) and [OpenImages](https://storage.googleapis.com/openimages/web/index.html).
(to be updated for v2)
### BibTeX entry and citation info
```bibtex
@misc{minderer2023scaling,
title={Scaling Open-Vocabulary Object Detection},
author={Matthias Minderer and Alexey Gritsenko and Neil Houlsby},
year={2023},
eprint={2306.09683},
archivePrefix={arXiv},
primaryClass={cs.CV}
}
``` |
patrickjohncyh/fashion-clip | patrickjohncyh | "2024-09-17T15:19:43Z" | 2,136,346 | 183 | transformers | [
"transformers",
"pytorch",
"onnx",
"safetensors",
"clip",
"zero-shot-image-classification",
"vision",
"language",
"fashion",
"ecommerce",
"en",
"license:mit",
"endpoints_compatible",
"region:us"
] | zero-shot-image-classification | "2023-02-21T19:51:47Z" | ---
license: mit
tags:
- vision
- language
- fashion
- ecommerce
library_name: transformers
language:
- en
widget:
- src: https://cdn-images.farfetch-contents.com/19/76/05/56/19760556_44221665_1000.jpg
candidate_labels: black shoe, red shoe, a cat
example_title: Black Shoe
---
[](https://www.youtube.com/watch?v=uqRSc-KSA1Y) [](https://huggingface.co/patrickjohncyh/fashion-clip) [](https://colab.research.google.com/drive/1Z1hAxBnWjF76bEi9KQ6CMBBEmI_FVDrW?usp=sharing) [](https://towardsdatascience.com/teaching-clip-some-fashion-3005ac3fdcc3) [](https://huggingface.co/spaces/vinid/fashion-clip-app)
# Model Card: Fashion CLIP
Disclaimer: The model card adapts the model card from [here](https://huggingface.co/openai/clip-vit-base-patch32).
## Model Details
UPDATE (10/03/23): We have updated the model! We found that [laion/CLIP-ViT-B-32-laion2B-s34B-b79K](https://huggingface.co/laion/CLIP-ViT-B-32-laion2B-s34B-b79K) checkpoint (thanks [Bin](https://www.linkedin.com/in/bin-duan-56205310/)!) worked better than original OpenAI CLIP on Fashion. We thus fine-tune a newer (and better!) version of FashionCLIP (henceforth FashionCLIP 2.0), while keeping the architecture the same. We postulate that the perofrmance gains afforded by `laion/CLIP-ViT-B-32-laion2B-s34B-b79K` are due to the increased training data (5x OpenAI CLIP data). Our [thesis](https://www.nature.com/articles/s41598-022-23052-9), however, remains the same -- fine-tuning `laion/CLIP` on our fashion dataset improved zero-shot perofrmance across our benchmarks. See the below table comparing weighted macro F1 score across models.
| Model | FMNIST | KAGL | DEEP |
| ------------- | ------------- | ------------- | ------------- |
| OpenAI CLIP | 0.66 | 0.63 | 0.45 |
| FashionCLIP | 0.74 | 0.67 | 0.48 |
| Laion CLIP | 0.78 | 0.71 | 0.58 |
| FashionCLIP 2.0 | __0.83__ | __0.73__ | __0.62__ |
---
FashionCLIP is a CLIP-based model developed to produce general product representations for fashion concepts. Leveraging the pre-trained checkpoint (ViT-B/32) released by [OpenAI](https://github.com/openai/CLIP), we train FashionCLIP on a large, high-quality novel fashion dataset to study whether domain specific fine-tuning of CLIP-like models is sufficient to produce product representations that are zero-shot transferable to entirely new datasets and tasks. FashionCLIP was not developed for model deplyoment - to do so, researchers will first need to carefully study their capabilities in relation to the specific context they’re being deployed within.
### Model Date
March 2023
### Model Type
The model uses a ViT-B/32 Transformer architecture as an image encoder and uses a masked self-attention Transformer as a text encoder. These encoders are trained, starting from a pre-trained checkpoint, to maximize the similarity of (image, text) pairs via a contrastive loss on a fashion dataset containing 800K products.
### Documents
- [FashionCLIP Github Repo](https://github.com/patrickjohncyh/fashion-clip)
- [FashionCLIP Paper](https://www.nature.com/articles/s41598-022-23052-9)
## Data
The model was trained on (image, text) pairs obtained from the Farfecth dataset[^1 Awaiting official release.], an English dataset comprising over 800K fashion products, with more than 3K brands across dozens of object types. The image used for encoding is the standard product image, which is a picture of the item over a white background, with no humans. The text used is a concatenation of the _highlight_ (e.g., “stripes”, “long sleeves”, “Armani”) and _short description_ (“80s styled t-shirt”)) available in the Farfetch dataset.
## Limitations, Bias and Fiarness
We acknowledge certain limitations of FashionCLIP and expect that it inherits certain limitations and biases present in the original CLIP model. We do not expect our fine-tuning to significantly augment these limitations: we acknowledge that the fashion data we use makes explicit assumptions about the notion of gender as in "blue shoes for a woman" that inevitably associate aspects of clothing with specific people.
Our investigations also suggest that the data used introduces certain limitations in FashionCLIP. From the textual modality, given that most captions derived from the Farfetch dataset are long, we observe that FashionCLIP may be more performant in longer queries than shorter ones. From the image modality, FashionCLIP is also biased towards standard product images (centered, white background).
Model selection, i.e. selecting an appropariate stopping critera during fine-tuning, remains an open challenge. We observed that using loss on an in-domain (i.e. same distribution as test) validation dataset is a poor selection critera when out-of-domain generalization (i.e. across different datasets) is desired, even when the dataset used is relatively diverse and large.
## Citation
```
@Article{Chia2022,
title="Contrastive language and vision learning of general fashion concepts",
author="Chia, Patrick John
and Attanasio, Giuseppe
and Bianchi, Federico
and Terragni, Silvia
and Magalh{\~a}es, Ana Rita
and Goncalves, Diogo
and Greco, Ciro
and Tagliabue, Jacopo",
journal="Scientific Reports",
year="2022",
month="Nov",
day="08",
volume="12",
number="1",
abstract="The steady rise of online shopping goes hand in hand with the development of increasingly complex ML and NLP models. While most use cases are cast as specialized supervised learning problems, we argue that practitioners would greatly benefit from general and transferable representations of products. In this work, we build on recent developments in contrastive learning to train FashionCLIP, a CLIP-like model adapted for the fashion industry. We demonstrate the effectiveness of the representations learned by FashionCLIP with extensive tests across a variety of tasks, datasets and generalization probes. We argue that adaptations of large pre-trained models such as CLIP offer new perspectives in terms of scalability and sustainability for certain types of players in the industry. Finally, we detail the costs and environmental impact of training, and release the model weights and code as open source contribution to the community.",
issn="2045-2322",
doi="10.1038/s41598-022-23052-9",
url="https://doi.org/10.1038/s41598-022-23052-9"
}
``` |
ProsusAI/finbert | ProsusAI | "2023-05-23T12:43:35Z" | 2,108,587 | 673 | transformers | [
"transformers",
"pytorch",
"tf",
"jax",
"bert",
"text-classification",
"financial-sentiment-analysis",
"sentiment-analysis",
"en",
"arxiv:1908.10063",
"autotrain_compatible",
"endpoints_compatible",
"region:us"
] | text-classification | "2022-03-02T23:29:04Z" | ---
language: "en"
tags:
- financial-sentiment-analysis
- sentiment-analysis
widget:
- text: "Stocks rallied and the British pound gained."
---
FinBERT is a pre-trained NLP model to analyze sentiment of financial text. It is built by further training the BERT language model in the finance domain, using a large financial corpus and thereby fine-tuning it for financial sentiment classification. [Financial PhraseBank](https://www.researchgate.net/publication/251231107_Good_Debt_or_Bad_Debt_Detecting_Semantic_Orientations_in_Economic_Texts) by Malo et al. (2014) is used for fine-tuning. For more details, please see the paper [FinBERT: Financial Sentiment Analysis with Pre-trained Language Models](https://arxiv.org/abs/1908.10063) and our related [blog post](https://medium.com/prosus-ai-tech-blog/finbert-financial-sentiment-analysis-with-bert-b277a3607101) on Medium.
The model will give softmax outputs for three labels: positive, negative or neutral.
---
About Prosus
Prosus is a global consumer internet group and one of the largest technology investors in the world. Operating and investing globally in markets with long-term growth potential, Prosus builds leading consumer internet companies that empower people and enrich communities. For more information, please visit www.prosus.com.
Contact information
Please contact Dogu Araci dogu.araci[at]prosus[dot]com and Zulkuf Genc zulkuf.genc[at]prosus[dot]com about any FinBERT related issues and questions.
|
SamLowe/roberta-base-go_emotions | SamLowe | "2023-10-04T10:00:58Z" | 2,046,261 | 458 | transformers | [
"transformers",
"pytorch",
"safetensors",
"roberta",
"text-classification",
"emotions",
"multi-class-classification",
"multi-label-classification",
"en",
"dataset:go_emotions",
"license:mit",
"autotrain_compatible",
"endpoints_compatible",
"region:us"
] | text-classification | "2022-09-15T13:04:21Z" | ---
language: en
tags:
- text-classification
- pytorch
- roberta
- emotions
- multi-class-classification
- multi-label-classification
datasets:
- go_emotions
license: mit
widget:
- text: I am not having a great day.
---
#### Overview
Model trained from [roberta-base](https://huggingface.co/roberta-base) on the [go_emotions](https://huggingface.co/datasets/go_emotions) dataset for multi-label classification.
##### ONNX version also available
A version of this model in ONNX format (including an INT8 quantized ONNX version) is now available at [https://huggingface.co/SamLowe/roberta-base-go_emotions-onnx](https://huggingface.co/SamLowe/roberta-base-go_emotions-onnx). These are faster for inference, esp for smaller batch sizes, massively reduce the size of the dependencies required for inference, make inference of the model more multi-platform, and in the case of the quantized version reduce the model file/download size by 75% whilst retaining almost all the accuracy if you only need inference.
#### Dataset used for the model
[go_emotions](https://huggingface.co/datasets/go_emotions) is based on Reddit data and has 28 labels. It is a multi-label dataset where one or multiple labels may apply for any given input text, hence this model is a multi-label classification model with 28 'probability' float outputs for any given input text. Typically a threshold of 0.5 is applied to the probabilities for the prediction for each label.
#### How the model was created
The model was trained using `AutoModelForSequenceClassification.from_pretrained` with `problem_type="multi_label_classification"` for 3 epochs with a learning rate of 2e-5 and weight decay of 0.01.
#### Inference
There are multiple ways to use this model in Huggingface Transformers. Possibly the simplest is using a pipeline:
```python
from transformers import pipeline
classifier = pipeline(task="text-classification", model="SamLowe/roberta-base-go_emotions", top_k=None)
sentences = ["I am not having a great day"]
model_outputs = classifier(sentences)
print(model_outputs[0])
# produces a list of dicts for each of the labels
```
#### Evaluation / metrics
Evaluation of the model is available at
- https://github.com/samlowe/go_emotions-dataset/blob/main/eval-roberta-base-go_emotions.ipynb
[](https://colab.research.google.com/github/samlowe/go_emotions-dataset/blob/main/eval-roberta-base-go_emotions.ipynb)
##### Summary
As provided in the above notebook, evaluation of the multi-label output (of the 28 dim output via a threshold of 0.5 to binarize each) using the dataset test split gives:
- Accuracy: 0.474
- Precision: 0.575
- Recall: 0.396
- F1: 0.450
But the metrics are more meaningful when measured per label given the multi-label nature (each label is effectively an independent binary classification) and the fact that there is drastically different representations of the labels in the dataset.
With a threshold of 0.5 applied to binarize the model outputs, as per the above notebook, the metrics per label are:
| | accuracy | precision | recall | f1 | mcc | support | threshold |
| -------------- | -------- | --------- | ------ | ----- | ----- | ------- | --------- |
| admiration | 0.946 | 0.725 | 0.675 | 0.699 | 0.670 | 504 | 0.5 |
| amusement | 0.982 | 0.790 | 0.871 | 0.829 | 0.821 | 264 | 0.5 |
| anger | 0.970 | 0.652 | 0.379 | 0.479 | 0.483 | 198 | 0.5 |
| annoyance | 0.940 | 0.472 | 0.159 | 0.238 | 0.250 | 320 | 0.5 |
| approval | 0.942 | 0.609 | 0.302 | 0.404 | 0.403 | 351 | 0.5 |
| caring | 0.973 | 0.448 | 0.319 | 0.372 | 0.364 | 135 | 0.5 |
| confusion | 0.972 | 0.500 | 0.431 | 0.463 | 0.450 | 153 | 0.5 |
| curiosity | 0.950 | 0.537 | 0.356 | 0.428 | 0.412 | 284 | 0.5 |
| desire | 0.987 | 0.630 | 0.410 | 0.496 | 0.502 | 83 | 0.5 |
| disappointment | 0.974 | 0.625 | 0.199 | 0.302 | 0.343 | 151 | 0.5 |
| disapproval | 0.950 | 0.494 | 0.307 | 0.379 | 0.365 | 267 | 0.5 |
| disgust | 0.982 | 0.707 | 0.333 | 0.453 | 0.478 | 123 | 0.5 |
| embarrassment | 0.994 | 0.750 | 0.243 | 0.367 | 0.425 | 37 | 0.5 |
| excitement | 0.983 | 0.603 | 0.340 | 0.435 | 0.445 | 103 | 0.5 |
| fear | 0.992 | 0.758 | 0.603 | 0.671 | 0.672 | 78 | 0.5 |
| gratitude | 0.990 | 0.960 | 0.881 | 0.919 | 0.914 | 352 | 0.5 |
| grief | 0.999 | 0.000 | 0.000 | 0.000 | 0.000 | 6 | 0.5 |
| joy | 0.978 | 0.647 | 0.559 | 0.600 | 0.590 | 161 | 0.5 |
| love | 0.982 | 0.773 | 0.832 | 0.802 | 0.793 | 238 | 0.5 |
| nervousness | 0.996 | 0.600 | 0.130 | 0.214 | 0.278 | 23 | 0.5 |
| optimism | 0.972 | 0.667 | 0.376 | 0.481 | 0.488 | 186 | 0.5 |
| pride | 0.997 | 0.000 | 0.000 | 0.000 | 0.000 | 16 | 0.5 |
| realization | 0.974 | 0.541 | 0.138 | 0.220 | 0.264 | 145 | 0.5 |
| relief | 0.998 | 0.000 | 0.000 | 0.000 | 0.000 | 11 | 0.5 |
| remorse | 0.991 | 0.553 | 0.750 | 0.636 | 0.640 | 56 | 0.5 |
| sadness | 0.977 | 0.621 | 0.494 | 0.550 | 0.542 | 156 | 0.5 |
| surprise | 0.981 | 0.750 | 0.404 | 0.525 | 0.542 | 141 | 0.5 |
| neutral | 0.782 | 0.694 | 0.604 | 0.646 | 0.492 | 1787 | 0.5 |
Optimizing the threshold per label for the one that gives the optimum F1 metrics gives slightly better metrics - sacrificing some precision for a greater gain in recall, hence to the benefit of F1 (how this was done is shown in the above notebook):
| | accuracy | precision | recall | f1 | mcc | support | threshold |
| -------------- | -------- | --------- | ------ | ----- | ----- | ------- | --------- |
| admiration | 0.940 | 0.651 | 0.776 | 0.708 | 0.678 | 504 | 0.25 |
| amusement | 0.982 | 0.781 | 0.890 | 0.832 | 0.825 | 264 | 0.45 |
| anger | 0.959 | 0.454 | 0.601 | 0.517 | 0.502 | 198 | 0.15 |
| annoyance | 0.864 | 0.243 | 0.619 | 0.349 | 0.328 | 320 | 0.10 |
| approval | 0.926 | 0.432 | 0.442 | 0.437 | 0.397 | 351 | 0.30 |
| caring | 0.972 | 0.426 | 0.385 | 0.405 | 0.391 | 135 | 0.40 |
| confusion | 0.974 | 0.548 | 0.412 | 0.470 | 0.462 | 153 | 0.55 |
| curiosity | 0.943 | 0.473 | 0.711 | 0.568 | 0.552 | 284 | 0.25 |
| desire | 0.985 | 0.518 | 0.530 | 0.524 | 0.516 | 83 | 0.25 |
| disappointment | 0.974 | 0.562 | 0.298 | 0.390 | 0.398 | 151 | 0.40 |
| disapproval | 0.941 | 0.414 | 0.468 | 0.439 | 0.409 | 267 | 0.30 |
| disgust | 0.978 | 0.523 | 0.463 | 0.491 | 0.481 | 123 | 0.20 |
| embarrassment | 0.994 | 0.567 | 0.459 | 0.507 | 0.507 | 37 | 0.10 |
| excitement | 0.981 | 0.500 | 0.417 | 0.455 | 0.447 | 103 | 0.35 |
| fear | 0.991 | 0.712 | 0.667 | 0.689 | 0.685 | 78 | 0.40 |
| gratitude | 0.990 | 0.957 | 0.889 | 0.922 | 0.917 | 352 | 0.45 |
| grief | 0.999 | 0.333 | 0.333 | 0.333 | 0.333 | 6 | 0.05 |
| joy | 0.978 | 0.623 | 0.646 | 0.634 | 0.623 | 161 | 0.40 |
| love | 0.982 | 0.740 | 0.899 | 0.812 | 0.807 | 238 | 0.25 |
| nervousness | 0.996 | 0.571 | 0.348 | 0.432 | 0.444 | 23 | 0.25 |
| optimism | 0.971 | 0.580 | 0.565 | 0.572 | 0.557 | 186 | 0.20 |
| pride | 0.998 | 0.875 | 0.438 | 0.583 | 0.618 | 16 | 0.10 |
| realization | 0.961 | 0.270 | 0.262 | 0.266 | 0.246 | 145 | 0.15 |
| relief | 0.992 | 0.152 | 0.636 | 0.246 | 0.309 | 11 | 0.05 |
| remorse | 0.991 | 0.541 | 0.946 | 0.688 | 0.712 | 56 | 0.10 |
| sadness | 0.977 | 0.599 | 0.583 | 0.591 | 0.579 | 156 | 0.40 |
| surprise | 0.977 | 0.543 | 0.674 | 0.601 | 0.593 | 141 | 0.15 |
| neutral | 0.758 | 0.598 | 0.810 | 0.688 | 0.513 | 1787 | 0.25 |
This improves the overall metrics:
- Precision: 0.542
- Recall: 0.577
- F1: 0.541
Or if calculated weighted by the relative size of the support of each label:
- Precision: 0.572
- Recall: 0.677
- F1: 0.611
#### Commentary on the dataset
Some labels (E.g. gratitude) when considered independently perform very strongly with F1 exceeding 0.9, whilst others (E.g. relief) perform very poorly.
This is a challenging dataset. Labels such as relief do have much fewer examples in the training data (less than 100 out of the 40k+, and only 11 in the test split).
But there is also some ambiguity and/or labelling errors visible in the training data of go_emotions that is suspected to constrain the performance. Data cleaning on the dataset to reduce some of the mistakes, ambiguity, conflicts and duplication in the labelling would produce a higher performing model. |
google/canine-c | google | "2024-04-29T10:16:15Z" | 2,016,805 | 32 | transformers | [
"transformers",
"pytorch",
"safetensors",
"canine",
"feature-extraction",
"multilingual",
"af",
"sq",
"ar",
"an",
"hy",
"ast",
"az",
"ba",
"eu",
"bar",
"be",
"bn",
"inc",
"bs",
"br",
"bg",
"my",
"ca",
"ceb",
"ce",
"zh",
"cv",
"hr",
"cs",
"da",
"nl",
"en",
"et",
"fi",
"fr",
"gl",
"ka",
"de",
"el",
"gu",
"ht",
"he",
"hi",
"hu",
"is",
"io",
"id",
"ga",
"it",
"ja",
"jv",
"kn",
"kk",
"ky",
"ko",
"la",
"lv",
"lt",
"roa",
"nds",
"lm",
"mk",
"mg",
"ms",
"ml",
"mr",
"mn",
"min",
"ne",
"new",
"nb",
"nn",
"oc",
"fa",
"pms",
"pl",
"pt",
"pa",
"ro",
"ru",
"sco",
"sr",
"scn",
"sk",
"sl",
"aze",
"es",
"su",
"sw",
"sv",
"tl",
"tg",
"th",
"ta",
"tt",
"te",
"tr",
"uk",
"ud",
"uz",
"vi",
"vo",
"war",
"cy",
"fry",
"pnb",
"yo",
"dataset:bookcorpus",
"dataset:wikipedia",
"arxiv:2103.06874",
"license:apache-2.0",
"endpoints_compatible",
"region:us"
] | feature-extraction | "2022-03-02T23:29:05Z" | ---
language:
- multilingual
- af
- sq
- ar
- an
- hy
- ast
- az
- ba
- eu
- bar
- be
- bn
- inc
- bs
- br
- bg
- my
- ca
- ceb
- ce
- zh
- cv
- hr
- cs
- da
- nl
- en
- et
- fi
- fr
- gl
- ka
- de
- el
- gu
- ht
- he
- hi
- hu
- is
- io
- id
- ga
- it
- ja
- jv
- kn
- kk
- ky
- ko
- la
- lv
- lt
- roa
- nds
- lm
- mk
- mg
- ms
- ml
- mr
- mn
- min
- ne
- new
- nb
- nn
- oc
- fa
- pms
- pl
- pt
- pa
- ro
- ru
- sco
- sr
- hr
- scn
- sk
- sl
- aze
- es
- su
- sw
- sv
- tl
- tg
- th
- ta
- tt
- te
- tr
- uk
- ud
- uz
- vi
- vo
- war
- cy
- fry
- pnb
- yo
license: apache-2.0
datasets:
- bookcorpus
- wikipedia
---
# CANINE-c (CANINE pre-trained with autoregressive character loss)
Pretrained CANINE model on 104 languages using a masked language modeling (MLM) objective. It was introduced in the paper [CANINE: Pre-training an Efficient Tokenization-Free Encoder for Language Representation](https://arxiv.org/abs/2103.06874) and first released in [this repository](https://github.com/google-research/language/tree/master/language/canine).
What's special about CANINE is that it doesn't require an explicit tokenizer (such as WordPiece or SentencePiece) as other models like BERT and RoBERTa. Instead, it directly operates at a character level: each character is turned into its [Unicode code point](https://en.wikipedia.org/wiki/Code_point#:~:text=For%20Unicode%2C%20the%20particular%20sequence,forming%20a%20self%2Dsynchronizing%20code.).
This means that input processing is trivial and can typically be accomplished as:
```
input_ids = [ord(char) for char in text]
```
The ord() function is part of Python, and turns each character into its Unicode code point.
Disclaimer: The team releasing CANINE did not write a model card for this model so this model card has been written by the Hugging Face team.
## Model description
CANINE is a transformers model pretrained on a large corpus of multilingual data in a self-supervised fashion, similar to BERT. This means it was pretrained on the raw texts only, with no humans labelling them in any way (which is why it can use lots of publicly available data) with an automatic process to generate inputs and labels from those texts. More precisely, it was pretrained with two objectives:
* Masked language modeling (MLM): one randomly masks part of the inputs, which the model needs to predict. This model (CANINE-c) is trained with an autoregressive character loss. One masks several character spans within each sequence, which the model then autoregressively predicts.
* Next sentence prediction (NSP): the model concatenates two sentences as inputs during pretraining. Sometimes they correspond to sentences that were next to each other in the original text, sometimes not. The model then has to predict if the two sentences were following each other or not.
This way, the model learns an inner representation of multiple languages that can then be used to extract features useful for downstream tasks: if you have a dataset of labeled sentences for instance, you can train a standard classifier using the features produced by the CANINE model as inputs.
## Intended uses & limitations
You can use the raw model for either masked language modeling or next sentence prediction, but it's mostly intended to be fine-tuned on a downstream task. See the [model hub](https://huggingface.co/models?filter=canine) to look for fine-tuned versions on a task that interests you.
Note that this model is primarily aimed at being fine-tuned on tasks that use the whole sentence (potentially masked) to make decisions, such as sequence classification, token classification or question answering. For tasks such as text generation you should look at models like GPT2.
### How to use
Here is how to use this model:
```python
from transformers import CanineTokenizer, CanineModel
model = CanineModel.from_pretrained('google/canine-c')
tokenizer = CanineTokenizer.from_pretrained('google/canine-c')
inputs = ["Life is like a box of chocolates.", "You never know what you gonna get."]
encoding = tokenizer(inputs, padding="longest", truncation=True, return_tensors="pt")
outputs = model(**encoding) # forward pass
pooled_output = outputs.pooler_output
sequence_output = outputs.last_hidden_state
```
## Training data
The CANINE model was pretrained on on the multilingual Wikipedia data of [mBERT](https://github.com/google-research/bert/blob/master/multilingual.md), which includes 104 languages.
### BibTeX entry and citation info
```bibtex
@article{DBLP:journals/corr/abs-2103-06874,
author = {Jonathan H. Clark and
Dan Garrette and
Iulia Turc and
John Wieting},
title = {{CANINE:} Pre-training an Efficient Tokenization-Free Encoder for
Language Representation},
journal = {CoRR},
volume = {abs/2103.06874},
year = {2021},
url = {https://arxiv.org/abs/2103.06874},
archivePrefix = {arXiv},
eprint = {2103.06874},
timestamp = {Tue, 16 Mar 2021 11:26:59 +0100},
biburl = {https://dblp.org/rec/journals/corr/abs-2103-06874.bib},
bibsource = {dblp computer science bibliography, https://dblp.org}
}
``` |
microsoft/layoutlm-base-uncased | microsoft | "2024-04-16T12:16:49Z" | 1,998,513 | 45 | transformers | [
"transformers",
"pytorch",
"tf",
"safetensors",
"layoutlm",
"en",
"arxiv:1912.13318",
"license:mit",
"endpoints_compatible",
"region:us"
] | null | "2022-03-02T23:29:05Z" | ---
language: en
license: mit
---
# LayoutLM
**Multimodal (text + layout/format + image) pre-training for document AI**
[Microsoft Document AI](https://www.microsoft.com/en-us/research/project/document-ai/) | [GitHub](https://aka.ms/layoutlm)
## Model description
LayoutLM is a simple but effective pre-training method of text and layout for document image understanding and information extraction tasks, such as form understanding and receipt understanding. LayoutLM archives the SOTA results on multiple datasets. For more details, please refer to our paper:
[LayoutLM: Pre-training of Text and Layout for Document Image Understanding](https://arxiv.org/abs/1912.13318)
Yiheng Xu, Minghao Li, Lei Cui, Shaohan Huang, Furu Wei, Ming Zhou, [KDD 2020](https://www.kdd.org/kdd2020/accepted-papers)
## Training data
We pre-train LayoutLM on IIT-CDIP Test Collection 1.0\* dataset with two settings.
* LayoutLM-Base, Uncased (11M documents, 2 epochs): 12-layer, 768-hidden, 12-heads, 113M parameters **(This Model)**
* LayoutLM-Large, Uncased (11M documents, 2 epochs): 24-layer, 1024-hidden, 16-heads, 343M parameters
## Citation
If you find LayoutLM useful in your research, please cite the following paper:
``` latex
@misc{xu2019layoutlm,
title={LayoutLM: Pre-training of Text and Layout for Document Image Understanding},
author={Yiheng Xu and Minghao Li and Lei Cui and Shaohan Huang and Furu Wei and Ming Zhou},
year={2019},
eprint={1912.13318},
archivePrefix={arXiv},
primaryClass={cs.CL}
}
```
|
google-bert/bert-base-chinese | google-bert | "2024-02-19T11:03:31Z" | 1,996,722 | 982 | transformers | [
"transformers",
"pytorch",
"tf",
"jax",
"safetensors",
"bert",
"fill-mask",
"zh",
"arxiv:1810.04805",
"autotrain_compatible",
"endpoints_compatible",
"region:us"
] | fill-mask | "2022-03-02T23:29:04Z" | ---
language: zh
---
# Bert-base-chinese
## Table of Contents
- [Model Details](#model-details)
- [Uses](#uses)
- [Risks, Limitations and Biases](#risks-limitations-and-biases)
- [Training](#training)
- [Evaluation](#evaluation)
- [How to Get Started With the Model](#how-to-get-started-with-the-model)
## Model Details
### Model Description
This model has been pre-trained for Chinese, training and random input masking has been applied independently to word pieces (as in the original BERT paper).
- **Developed by:** HuggingFace team
- **Model Type:** Fill-Mask
- **Language(s):** Chinese
- **License:** [More Information needed]
- **Parent Model:** See the [BERT base uncased model](https://huggingface.co/bert-base-uncased) for more information about the BERT base model.
### Model Sources
- **Paper:** [BERT](https://arxiv.org/abs/1810.04805)
## Uses
#### Direct Use
This model can be used for masked language modeling
## Risks, Limitations and Biases
**CONTENT WARNING: Readers should be aware this section contains content that is disturbing, offensive, and can propagate historical and current stereotypes.**
Significant research has explored bias and fairness issues with language models (see, e.g., [Sheng et al. (2021)](https://aclanthology.org/2021.acl-long.330.pdf) and [Bender et al. (2021)](https://dl.acm.org/doi/pdf/10.1145/3442188.3445922)).
## Training
#### Training Procedure
* **type_vocab_size:** 2
* **vocab_size:** 21128
* **num_hidden_layers:** 12
#### Training Data
[More Information Needed]
## Evaluation
#### Results
[More Information Needed]
## How to Get Started With the Model
```python
from transformers import AutoTokenizer, AutoModelForMaskedLM
tokenizer = AutoTokenizer.from_pretrained("bert-base-chinese")
model = AutoModelForMaskedLM.from_pretrained("bert-base-chinese")
```
|
google/byt5-small | google | "2023-01-24T16:36:59Z" | 1,991,969 | 60 | transformers | [
"transformers",
"pytorch",
"tf",
"jax",
"t5",
"text2text-generation",
"multilingual",
"af",
"am",
"ar",
"az",
"be",
"bg",
"bn",
"ca",
"ceb",
"co",
"cs",
"cy",
"da",
"de",
"el",
"en",
"eo",
"es",
"et",
"eu",
"fa",
"fi",
"fil",
"fr",
"fy",
"ga",
"gd",
"gl",
"gu",
"ha",
"haw",
"hi",
"hmn",
"ht",
"hu",
"hy",
"ig",
"is",
"it",
"iw",
"ja",
"jv",
"ka",
"kk",
"km",
"kn",
"ko",
"ku",
"ky",
"la",
"lb",
"lo",
"lt",
"lv",
"mg",
"mi",
"mk",
"ml",
"mn",
"mr",
"ms",
"mt",
"my",
"ne",
"nl",
"no",
"ny",
"pa",
"pl",
"ps",
"pt",
"ro",
"ru",
"sd",
"si",
"sk",
"sl",
"sm",
"sn",
"so",
"sq",
"sr",
"st",
"su",
"sv",
"sw",
"ta",
"te",
"tg",
"th",
"tr",
"uk",
"und",
"ur",
"uz",
"vi",
"xh",
"yi",
"yo",
"zh",
"zu",
"dataset:mc4",
"arxiv:1907.06292",
"arxiv:2105.13626",
"license:apache-2.0",
"autotrain_compatible",
"text-generation-inference",
"endpoints_compatible",
"region:us"
] | text2text-generation | "2022-03-02T23:29:05Z" | ---
language:
- multilingual
- af
- am
- ar
- az
- be
- bg
- bn
- ca
- ceb
- co
- cs
- cy
- da
- de
- el
- en
- eo
- es
- et
- eu
- fa
- fi
- fil
- fr
- fy
- ga
- gd
- gl
- gu
- ha
- haw
- hi
- hmn
- ht
- hu
- hy
- ig
- is
- it
- iw
- ja
- jv
- ka
- kk
- km
- kn
- ko
- ku
- ky
- la
- lb
- lo
- lt
- lv
- mg
- mi
- mk
- ml
- mn
- mr
- ms
- mt
- my
- ne
- nl
- no
- ny
- pa
- pl
- ps
- pt
- ro
- ru
- sd
- si
- sk
- sl
- sm
- sn
- so
- sq
- sr
- st
- su
- sv
- sw
- ta
- te
- tg
- th
- tr
- uk
- und
- ur
- uz
- vi
- xh
- yi
- yo
- zh
- zu
datasets:
- mc4
license: apache-2.0
---
# ByT5 - Small
ByT5 is a tokenizer-free version of [Google's T5](https://ai.googleblog.com/2020/02/exploring-transfer-learning-with-t5.html) and generally follows the architecture of [MT5](https://huggingface.co/google/mt5-small).
ByT5 was only pre-trained on [mC4](https://www.tensorflow.org/datasets/catalog/c4#c4multilingual) excluding any supervised training with an average span-mask of 20 UTF-8 characters. Therefore, this model has to be fine-tuned before it is useable on a downstream task.
ByT5 works especially well on noisy text data,*e.g.*, `google/byt5-small` significantly outperforms [mt5-small](https://huggingface.co/google/mt5-small) on [TweetQA](https://arxiv.org/abs/1907.06292).
Paper: [ByT5: Towards a token-free future with pre-trained byte-to-byte models](https://arxiv.org/abs/2105.13626)
Authors: *Linting Xue, Aditya Barua, Noah Constant, Rami Al-Rfou, Sharan Narang, Mihir Kale, Adam Roberts, Colin Raffel*
## Example Inference
ByT5 works on raw UTF-8 bytes and can be used without a tokenizer:
```python
from transformers import T5ForConditionalGeneration
import torch
model = T5ForConditionalGeneration.from_pretrained('google/byt5-small')
input_ids = torch.tensor([list("Life is like a box of chocolates.".encode("utf-8"))]) + 3 # add 3 for special tokens
labels = torch.tensor([list("La vie est comme une boîte de chocolat.".encode("utf-8"))]) + 3 # add 3 for special tokens
loss = model(input_ids, labels=labels).loss # forward pass
```
For batched inference & training it is however recommended using a tokenizer class for padding:
```python
from transformers import T5ForConditionalGeneration, AutoTokenizer
model = T5ForConditionalGeneration.from_pretrained('google/byt5-small')
tokenizer = AutoTokenizer.from_pretrained('google/byt5-small')
model_inputs = tokenizer(["Life is like a box of chocolates.", "Today is Monday."], padding="longest", return_tensors="pt")
labels = tokenizer(["La vie est comme une boîte de chocolat.", "Aujourd'hui c'est lundi."], padding="longest", return_tensors="pt").input_ids
loss = model(**model_inputs, labels=labels).loss # forward pass
```
## Abstract
Most widely-used pre-trained language models operate on sequences of tokens corresponding to word or subword units. Encoding text as a sequence of tokens requires a tokenizer, which is typically created as an independent artifact from the model. Token-free models that instead operate directly on raw text (bytes or characters) have many benefits: they can process text in any language out of the box, they are more robust to noise, and they minimize technical debt by removing complex and error-prone text preprocessing pipelines. Since byte or character sequences are longer than token sequences, past work on token-free models has often introduced new model architectures designed to amortize the cost of operating directly on raw text. In this paper, we show that a standard Transformer architecture can be used with minimal modifications to process byte sequences. We carefully characterize the trade-offs in terms of parameter count, training FLOPs, and inference speed, and show that byte-level models are competitive with their token-level counterparts. We also demonstrate that byte-level models are significantly more robust to noise and perform better on tasks that are sensitive to spelling and pronunciation. As part of our contribution, we release a new set of pre-trained byte-level Transformer models based on the T5 architecture, as well as all code and data used in our experiments.
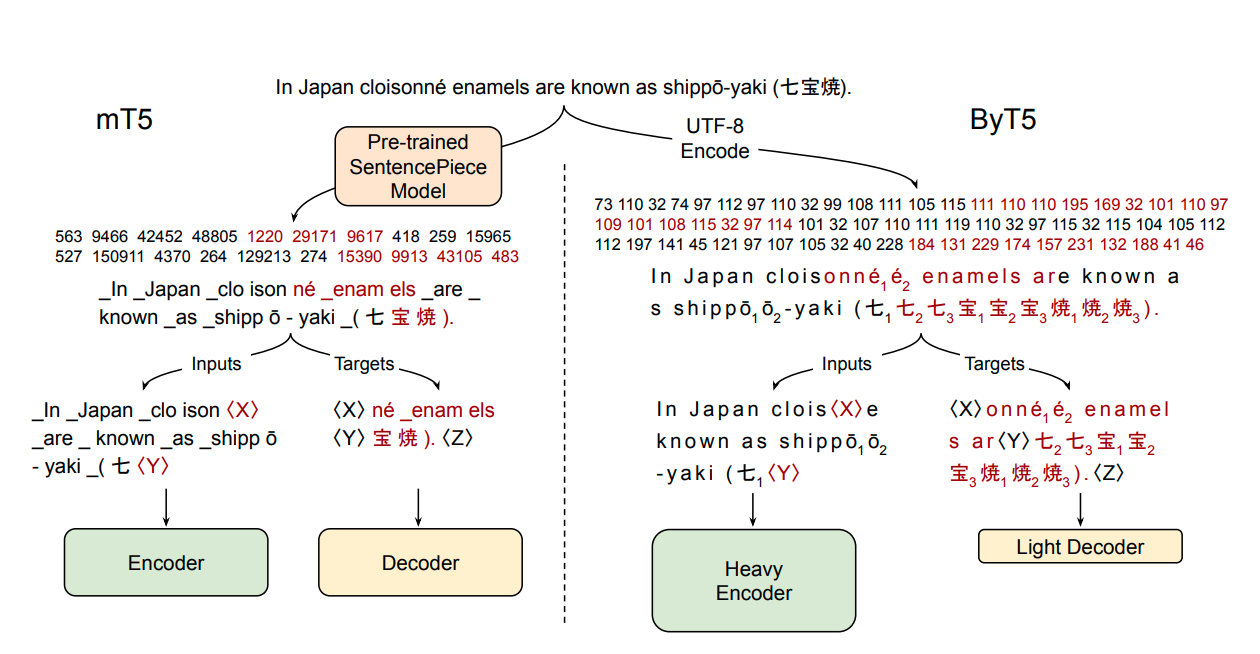
|
MahmoudAshraf/mms-300m-1130-forced-aligner | MahmoudAshraf | "2024-09-28T17:05:59Z" | 1,981,694 | 29 | transformers | [
"transformers",
"pytorch",
"safetensors",
"wav2vec2",
"automatic-speech-recognition",
"mms",
"audio",
"voice",
"speech",
"forced-alignment",
"ab",
"af",
"ak",
"am",
"ar",
"as",
"av",
"ay",
"az",
"ba",
"bm",
"be",
"bn",
"bi",
"bo",
"sh",
"br",
"bg",
"ca",
"cs",
"ce",
"cv",
"ku",
"cy",
"da",
"de",
"dv",
"dz",
"el",
"en",
"eo",
"et",
"eu",
"ee",
"fo",
"fa",
"fj",
"fi",
"fr",
"fy",
"ff",
"ga",
"gl",
"gn",
"gu",
"zh",
"ht",
"ha",
"he",
"hi",
"hu",
"hy",
"ig",
"ia",
"ms",
"is",
"it",
"jv",
"ja",
"kn",
"ka",
"kk",
"kr",
"km",
"ki",
"rw",
"ky",
"ko",
"kv",
"lo",
"la",
"lv",
"ln",
"lt",
"lb",
"lg",
"mh",
"ml",
"mr",
"mk",
"mg",
"mt",
"mn",
"mi",
"my",
"nl",
"no",
"ne",
"ny",
"oc",
"om",
"or",
"os",
"pa",
"pl",
"pt",
"ps",
"qu",
"ro",
"rn",
"ru",
"sg",
"sk",
"sl",
"sm",
"sn",
"sd",
"so",
"es",
"sq",
"su",
"sv",
"sw",
"ta",
"tt",
"te",
"tg",
"tl",
"th",
"ti",
"ts",
"tr",
"uk",
"vi",
"wo",
"xh",
"yo",
"zu",
"za",
"license:cc-by-nc-4.0",
"endpoints_compatible",
"region:us"
] | automatic-speech-recognition | "2024-05-02T21:02:39Z" | ---
language:
- ab
- af
- ak
- am
- ar
- as
- av
- ay
- az
- ba
- bm
- be
- bn
- bi
- bo
- sh
- br
- bg
- ca
- cs
- ce
- cv
- ku
- cy
- da
- de
- dv
- dz
- el
- en
- eo
- et
- eu
- ee
- fo
- fa
- fj
- fi
- fr
- fy
- ff
- ga
- gl
- gn
- gu
- zh
- ht
- ha
- he
- hi
- sh
- hu
- hy
- ig
- ia
- ms
- is
- it
- jv
- ja
- kn
- ka
- kk
- kr
- km
- ki
- rw
- ky
- ko
- kv
- lo
- la
- lv
- ln
- lt
- lb
- lg
- mh
- ml
- mr
- ms
- mk
- mg
- mt
- mn
- mi
- my
- zh
- nl
- 'no'
- 'no'
- ne
- ny
- oc
- om
- or
- os
- pa
- pl
- pt
- ms
- ps
- qu
- qu
- qu
- qu
- qu
- qu
- qu
- qu
- qu
- qu
- qu
- qu
- qu
- qu
- qu
- qu
- qu
- qu
- qu
- qu
- qu
- qu
- ro
- rn
- ru
- sg
- sk
- sl
- sm
- sn
- sd
- so
- es
- sq
- su
- sv
- sw
- ta
- tt
- te
- tg
- tl
- th
- ti
- ts
- tr
- uk
- ms
- vi
- wo
- xh
- ms
- yo
- ms
- zu
- za
license: cc-by-nc-4.0
tags:
- mms
- wav2vec2
- audio
- voice
- speech
- forced-alignment
pipeline_tag: automatic-speech-recognition
---
# Forced Alignment with Hugging Face CTC Models
This Python package provides an efficient way to perform forced alignment between text and audio using Hugging Face's pretrained models. it also features an improved implementation to use much less memory than TorchAudio forced alignment API.
The model checkpoint uploaded here is a conversion from torchaudio to HF Transformers for the MMS-300M checkpoint trained on forced alignment dataset
## Installation
```bash
pip install git+https://github.com/MahmoudAshraf97/ctc-forced-aligner.git
```
## Usage
```python
import torch
from ctc_forced_aligner import (
load_audio,
load_alignment_model,
generate_emissions,
preprocess_text,
get_alignments,
get_spans,
postprocess_results,
)
audio_path = "your/audio/path"
text_path = "your/text/path"
language = "iso" # ISO-639-3 Language code
device = "cuda" if torch.cuda.is_available() else "cpu"
batch_size = 16
alignment_model, alignment_tokenizer = load_alignment_model(
device,
dtype=torch.float16 if device == "cuda" else torch.float32,
)
audio_waveform = load_audio(audio_path, alignment_model.dtype, alignment_model.device)
with open(text_path, "r") as f:
lines = f.readlines()
text = "".join(line for line in lines).replace("\n", " ").strip()
emissions, stride = generate_emissions(
alignment_model, audio_waveform, batch_size=batch_size
)
tokens_starred, text_starred = preprocess_text(
text,
romanize=True,
language=language,
)
segments, scores, blank_token = get_alignments(
emissions,
tokens_starred,
alignment_tokenizer,
)
spans = get_spans(tokens_starred, segments, blank_token)
word_timestamps = postprocess_results(text_starred, spans, stride, scores)
``` |
sentence-transformers/paraphrase-multilingual-mpnet-base-v2 | sentence-transformers | "2024-11-05T18:28:42Z" | 1,980,505 | 317 | sentence-transformers | [
"sentence-transformers",
"pytorch",
"tf",
"onnx",
"safetensors",
"openvino",
"xlm-roberta",
"feature-extraction",
"sentence-similarity",
"transformers",
"multilingual",
"ar",
"bg",
"ca",
"cs",
"da",
"de",
"el",
"en",
"es",
"et",
"fa",
"fi",
"fr",
"gl",
"gu",
"he",
"hi",
"hr",
"hu",
"hy",
"id",
"it",
"ja",
"ka",
"ko",
"ku",
"lt",
"lv",
"mk",
"mn",
"mr",
"ms",
"my",
"nb",
"nl",
"pl",
"pt",
"ro",
"ru",
"sk",
"sl",
"sq",
"sr",
"sv",
"th",
"tr",
"uk",
"ur",
"vi",
"arxiv:1908.10084",
"license:apache-2.0",
"autotrain_compatible",
"text-embeddings-inference",
"endpoints_compatible",
"region:us"
] | sentence-similarity | "2022-03-02T23:29:05Z" | ---
language:
- multilingual
- ar
- bg
- ca
- cs
- da
- de
- el
- en
- es
- et
- fa
- fi
- fr
- gl
- gu
- he
- hi
- hr
- hu
- hy
- id
- it
- ja
- ka
- ko
- ku
- lt
- lv
- mk
- mn
- mr
- ms
- my
- nb
- nl
- pl
- pt
- ro
- ru
- sk
- sl
- sq
- sr
- sv
- th
- tr
- uk
- ur
- vi
license: apache-2.0
library_name: sentence-transformers
tags:
- sentence-transformers
- feature-extraction
- sentence-similarity
- transformers
language_bcp47:
- fr-ca
- pt-br
- zh-cn
- zh-tw
pipeline_tag: sentence-similarity
---
# sentence-transformers/paraphrase-multilingual-mpnet-base-v2
This is a [sentence-transformers](https://www.SBERT.net) model: It maps sentences & paragraphs to a 768 dimensional dense vector space and can be used for tasks like clustering or semantic search.
## Usage (Sentence-Transformers)
Using this model becomes easy when you have [sentence-transformers](https://www.SBERT.net) installed:
```
pip install -U sentence-transformers
```
Then you can use the model like this:
```python
from sentence_transformers import SentenceTransformer
sentences = ["This is an example sentence", "Each sentence is converted"]
model = SentenceTransformer('sentence-transformers/paraphrase-multilingual-mpnet-base-v2')
embeddings = model.encode(sentences)
print(embeddings)
```
## Usage (HuggingFace Transformers)
Without [sentence-transformers](https://www.SBERT.net), you can use the model like this: First, you pass your input through the transformer model, then you have to apply the right pooling-operation on-top of the contextualized word embeddings.
```python
from transformers import AutoTokenizer, AutoModel
import torch
#Mean Pooling - Take attention mask into account for correct averaging
def mean_pooling(model_output, attention_mask):
token_embeddings = model_output[0] #First element of model_output contains all token embeddings
input_mask_expanded = attention_mask.unsqueeze(-1).expand(token_embeddings.size()).float()
return torch.sum(token_embeddings * input_mask_expanded, 1) / torch.clamp(input_mask_expanded.sum(1), min=1e-9)
# Sentences we want sentence embeddings for
sentences = ['This is an example sentence', 'Each sentence is converted']
# Load model from HuggingFace Hub
tokenizer = AutoTokenizer.from_pretrained('sentence-transformers/paraphrase-multilingual-mpnet-base-v2')
model = AutoModel.from_pretrained('sentence-transformers/paraphrase-multilingual-mpnet-base-v2')
# Tokenize sentences
encoded_input = tokenizer(sentences, padding=True, truncation=True, return_tensors='pt')
# Compute token embeddings
with torch.no_grad():
model_output = model(**encoded_input)
# Perform pooling. In this case, average pooling
sentence_embeddings = mean_pooling(model_output, encoded_input['attention_mask'])
print("Sentence embeddings:")
print(sentence_embeddings)
```
## Evaluation Results
For an automated evaluation of this model, see the *Sentence Embeddings Benchmark*: [https://seb.sbert.net](https://seb.sbert.net?model_name=sentence-transformers/paraphrase-multilingual-mpnet-base-v2)
## Full Model Architecture
```
SentenceTransformer(
(0): Transformer({'max_seq_length': 128, 'do_lower_case': False}) with Transformer model: XLMRobertaModel
(1): Pooling({'word_embedding_dimension': 768, 'pooling_mode_cls_token': False, 'pooling_mode_mean_tokens': True, 'pooling_mode_max_tokens': False, 'pooling_mode_mean_sqrt_len_tokens': False})
)
```
## Citing & Authors
This model was trained by [sentence-transformers](https://www.sbert.net/).
If you find this model helpful, feel free to cite our publication [Sentence-BERT: Sentence Embeddings using Siamese BERT-Networks](https://arxiv.org/abs/1908.10084):
```bibtex
@inproceedings{reimers-2019-sentence-bert,
title = "Sentence-BERT: Sentence Embeddings using Siamese BERT-Networks",
author = "Reimers, Nils and Gurevych, Iryna",
booktitle = "Proceedings of the 2019 Conference on Empirical Methods in Natural Language Processing",
month = "11",
year = "2019",
publisher = "Association for Computational Linguistics",
url = "http://arxiv.org/abs/1908.10084",
}
``` |
black-forest-labs/FLUX.1-schnell | black-forest-labs | "2024-08-16T14:37:56Z" | 1,966,620 | 2,821 | diffusers | [
"diffusers",
"safetensors",
"text-to-image",
"image-generation",
"flux",
"en",
"license:apache-2.0",
"endpoints_compatible",
"diffusers:FluxPipeline",
"region:us"
] | text-to-image | "2024-07-31T19:58:05Z" | ---
language:
- en
license: apache-2.0
tags:
- text-to-image
- image-generation
- flux
---
![FLUX.1 [schnell] Grid](./schnell_grid.jpeg)
`FLUX.1 [schnell]` is a 12 billion parameter rectified flow transformer capable of generating images from text descriptions.
For more information, please read our [blog post](https://blackforestlabs.ai/announcing-black-forest-labs/).
# Key Features
1. Cutting-edge output quality and competitive prompt following, matching the performance of closed source alternatives.
2. Trained using latent adversarial diffusion distillation, `FLUX.1 [schnell]` can generate high-quality images in only 1 to 4 steps.
3. Released under the `apache-2.0` licence, the model can be used for personal, scientific, and commercial purposes.
# Usage
We provide a reference implementation of `FLUX.1 [schnell]`, as well as sampling code, in a dedicated [github repository](https://github.com/black-forest-labs/flux).
Developers and creatives looking to build on top of `FLUX.1 [schnell]` are encouraged to use this as a starting point.
## API Endpoints
The FLUX.1 models are also available via API from the following sources
- [bfl.ml](https://docs.bfl.ml/) (currently `FLUX.1 [pro]`)
- [replicate.com](https://replicate.com/collections/flux)
- [fal.ai](https://fal.ai/models/fal-ai/flux/schnell)
- [mystic.ai](https://www.mystic.ai/black-forest-labs/flux1-schnell)
## ComfyUI
`FLUX.1 [schnell]` is also available in [Comfy UI](https://github.com/comfyanonymous/ComfyUI) for local inference with a node-based workflow.
## Diffusers
To use `FLUX.1 [schnell]` with the 🧨 diffusers python library, first install or upgrade diffusers
```shell
pip install -U diffusers
```
Then you can use `FluxPipeline` to run the model
```python
import torch
from diffusers import FluxPipeline
pipe = FluxPipeline.from_pretrained("black-forest-labs/FLUX.1-schnell", torch_dtype=torch.bfloat16)
pipe.enable_model_cpu_offload() #save some VRAM by offloading the model to CPU. Remove this if you have enough GPU power
prompt = "A cat holding a sign that says hello world"
image = pipe(
prompt,
guidance_scale=0.0,
num_inference_steps=4,
max_sequence_length=256,
generator=torch.Generator("cpu").manual_seed(0)
).images[0]
image.save("flux-schnell.png")
```
To learn more check out the [diffusers](https://huggingface.co/docs/diffusers/main/en/api/pipelines/flux) documentation
---
# Limitations
- This model is not intended or able to provide factual information.
- As a statistical model this checkpoint might amplify existing societal biases.
- The model may fail to generate output that matches the prompts.
- Prompt following is heavily influenced by the prompting-style.
# Out-of-Scope Use
The model and its derivatives may not be used
- In any way that violates any applicable national, federal, state, local or international law or regulation.
- For the purpose of exploiting, harming or attempting to exploit or harm minors in any way; including but not limited to the solicitation, creation, acquisition, or dissemination of child exploitative content.
- To generate or disseminate verifiably false information and/or content with the purpose of harming others.
- To generate or disseminate personal identifiable information that can be used to harm an individual.
- To harass, abuse, threaten, stalk, or bully individuals or groups of individuals.
- To create non-consensual nudity or illegal pornographic content.
- For fully automated decision making that adversely impacts an individual's legal rights or otherwise creates or modifies a binding, enforceable obligation.
- Generating or facilitating large-scale disinformation campaigns. |
microsoft/git-base | microsoft | "2023-04-24T09:52:15Z" | 1,946,908 | 73 | transformers | [
"transformers",
"pytorch",
"safetensors",
"git",
"image-text-to-text",
"vision",
"image-to-text",
"image-captioning",
"en",
"arxiv:2205.14100",
"license:mit",
"endpoints_compatible",
"region:us"
] | image-to-text | "2022-12-06T09:22:35Z" | ---
language: en
license: mit
tags:
- vision
- image-to-text
- image-captioning
model_name: microsoft/git-base
pipeline_tag: image-to-text
---
# GIT (GenerativeImage2Text), base-sized
GIT (short for GenerativeImage2Text) model, base-sized version. It was introduced in the paper [GIT: A Generative Image-to-text Transformer for Vision and Language](https://arxiv.org/abs/2205.14100) by Wang et al. and first released in [this repository](https://github.com/microsoft/GenerativeImage2Text).
Disclaimer: The team releasing GIT did not write a model card for this model so this model card has been written by the Hugging Face team.
## Model description
GIT is a Transformer decoder conditioned on both CLIP image tokens and text tokens. The model is trained using "teacher forcing" on a lot of (image, text) pairs.
The goal for the model is simply to predict the next text token, giving the image tokens and previous text tokens.
The model has full access to (i.e. a bidirectional attention mask is used for) the image patch tokens, but only has access to the previous text tokens (i.e. a causal attention mask is used for the text tokens) when predicting the next text token.

This allows the model to be used for tasks like:
- image and video captioning
- visual question answering (VQA) on images and videos
- even image classification (by simply conditioning the model on the image and asking it to generate a class for it in text).
## Intended uses & limitations
You can use the raw model for image captioning. See the [model hub](https://huggingface.co/models?search=microsoft/git) to look for
fine-tuned versions on a task that interests you.
### How to use
For code examples, we refer to the [documentation](https://huggingface.co/docs/transformers/main/model_doc/git#transformers.GitForCausalLM.forward.example).
## Training data
From the paper:
> We collect 0.8B image-text pairs for pre-training, which include COCO (Lin et al., 2014), Conceptual Captions
(CC3M) (Sharma et al., 2018), SBU (Ordonez et al., 2011), Visual Genome (VG) (Krishna et al., 2016),
Conceptual Captions (CC12M) (Changpinyo et al., 2021), ALT200M (Hu et al., 2021a), and an extra 0.6B
data following a similar collection procedure in Hu et al. (2021a).
=> however this is for the model referred to as "GIT" in the paper, which is not open-sourced.
This checkpoint is "GIT-base", which is a smaller variant of GIT trained on 10 million image-text pairs.
See table 11 in the [paper](https://arxiv.org/abs/2205.14100) for more details.
### Preprocessing
We refer to the original repo regarding details for preprocessing during training.
During validation, one resizes the shorter edge of each image, after which center cropping is performed to a fixed-size resolution. Next, frames are normalized across the RGB channels with the ImageNet mean and standard deviation.
## Evaluation results
For evaluation results, we refer readers to the [paper](https://arxiv.org/abs/2205.14100). |
Salesforce/blip-image-captioning-base | Salesforce | "2023-08-01T14:46:56Z" | 1,946,120 | 508 | transformers | [
"transformers",
"pytorch",
"tf",
"blip",
"image-text-to-text",
"image-captioning",
"image-to-text",
"arxiv:2201.12086",
"license:bsd-3-clause",
"endpoints_compatible",
"region:us"
] | image-to-text | "2022-12-12T15:19:02Z" | ---
pipeline_tag: image-to-text
tags:
- image-captioning
languages:
- en
license: bsd-3-clause
---
# BLIP: Bootstrapping Language-Image Pre-training for Unified Vision-Language Understanding and Generation
Model card for image captioning pretrained on COCO dataset - base architecture (with ViT base backbone).
|  |
|:--:|
| <b> Pull figure from BLIP official repo | Image source: https://github.com/salesforce/BLIP </b>|
## TL;DR
Authors from the [paper](https://arxiv.org/abs/2201.12086) write in the abstract:
*Vision-Language Pre-training (VLP) has advanced the performance for many vision-language tasks. However, most existing pre-trained models only excel in either understanding-based tasks or generation-based tasks. Furthermore, performance improvement has been largely achieved by scaling up the dataset with noisy image-text pairs collected from the web, which is a suboptimal source of supervision. In this paper, we propose BLIP, a new VLP framework which transfers flexibly to both vision-language understanding and generation tasks. BLIP effectively utilizes the noisy web data by bootstrapping the captions, where a captioner generates synthetic captions and a filter removes the noisy ones. We achieve state-of-the-art results on a wide range of vision-language tasks, such as image-text retrieval (+2.7% in average recall@1), image captioning (+2.8% in CIDEr), and VQA (+1.6% in VQA score). BLIP also demonstrates strong generalization ability when directly transferred to videolanguage tasks in a zero-shot manner. Code, models, and datasets are released.*
## Usage
You can use this model for conditional and un-conditional image captioning
### Using the Pytorch model
#### Running the model on CPU
<details>
<summary> Click to expand </summary>
```python
import requests
from PIL import Image
from transformers import BlipProcessor, BlipForConditionalGeneration
processor = BlipProcessor.from_pretrained("Salesforce/blip-image-captioning-base")
model = BlipForConditionalGeneration.from_pretrained("Salesforce/blip-image-captioning-base")
img_url = 'https://storage.googleapis.com/sfr-vision-language-research/BLIP/demo.jpg'
raw_image = Image.open(requests.get(img_url, stream=True).raw).convert('RGB')
# conditional image captioning
text = "a photography of"
inputs = processor(raw_image, text, return_tensors="pt")
out = model.generate(**inputs)
print(processor.decode(out[0], skip_special_tokens=True))
# >>> a photography of a woman and her dog
# unconditional image captioning
inputs = processor(raw_image, return_tensors="pt")
out = model.generate(**inputs)
print(processor.decode(out[0], skip_special_tokens=True))
>>> a woman sitting on the beach with her dog
```
</details>
#### Running the model on GPU
##### In full precision
<details>
<summary> Click to expand </summary>
```python
import requests
from PIL import Image
from transformers import BlipProcessor, BlipForConditionalGeneration
processor = BlipProcessor.from_pretrained("Salesforce/blip-image-captioning-base")
model = BlipForConditionalGeneration.from_pretrained("Salesforce/blip-image-captioning-base").to("cuda")
img_url = 'https://storage.googleapis.com/sfr-vision-language-research/BLIP/demo.jpg'
raw_image = Image.open(requests.get(img_url, stream=True).raw).convert('RGB')
# conditional image captioning
text = "a photography of"
inputs = processor(raw_image, text, return_tensors="pt").to("cuda")
out = model.generate(**inputs)
print(processor.decode(out[0], skip_special_tokens=True))
# >>> a photography of a woman and her dog
# unconditional image captioning
inputs = processor(raw_image, return_tensors="pt").to("cuda")
out = model.generate(**inputs)
print(processor.decode(out[0], skip_special_tokens=True))
>>> a woman sitting on the beach with her dog
```
</details>
##### In half precision (`float16`)
<details>
<summary> Click to expand </summary>
```python
import torch
import requests
from PIL import Image
from transformers import BlipProcessor, BlipForConditionalGeneration
processor = BlipProcessor.from_pretrained("Salesforce/blip-image-captioning-base")
model = BlipForConditionalGeneration.from_pretrained("Salesforce/blip-image-captioning-base", torch_dtype=torch.float16).to("cuda")
img_url = 'https://storage.googleapis.com/sfr-vision-language-research/BLIP/demo.jpg'
raw_image = Image.open(requests.get(img_url, stream=True).raw).convert('RGB')
# conditional image captioning
text = "a photography of"
inputs = processor(raw_image, text, return_tensors="pt").to("cuda", torch.float16)
out = model.generate(**inputs)
print(processor.decode(out[0], skip_special_tokens=True))
# >>> a photography of a woman and her dog
# unconditional image captioning
inputs = processor(raw_image, return_tensors="pt").to("cuda", torch.float16)
out = model.generate(**inputs)
print(processor.decode(out[0], skip_special_tokens=True))
>>> a woman sitting on the beach with her dog
```
</details>
## BibTex and citation info
```
@misc{https://doi.org/10.48550/arxiv.2201.12086,
doi = {10.48550/ARXIV.2201.12086},
url = {https://arxiv.org/abs/2201.12086},
author = {Li, Junnan and Li, Dongxu and Xiong, Caiming and Hoi, Steven},
keywords = {Computer Vision and Pattern Recognition (cs.CV), FOS: Computer and information sciences, FOS: Computer and information sciences},
title = {BLIP: Bootstrapping Language-Image Pre-training for Unified Vision-Language Understanding and Generation},
publisher = {arXiv},
year = {2022},
copyright = {Creative Commons Attribution 4.0 International}
}
```
|
microsoft/deberta-v3-base | microsoft | "2022-09-22T12:34:19Z" | 1,929,469 | 250 | transformers | [
"transformers",
"pytorch",
"tf",
"rust",
"deberta-v2",
"deberta",
"deberta-v3",
"fill-mask",
"en",
"arxiv:2006.03654",
"arxiv:2111.09543",
"license:mit",
"endpoints_compatible",
"region:us"
] | fill-mask | "2022-03-02T23:29:05Z" | ---
language: en
tags:
- deberta
- deberta-v3
- fill-mask
thumbnail: https://huggingface.co/front/thumbnails/microsoft.png
license: mit
---
## DeBERTaV3: Improving DeBERTa using ELECTRA-Style Pre-Training with Gradient-Disentangled Embedding Sharing
[DeBERTa](https://arxiv.org/abs/2006.03654) improves the BERT and RoBERTa models using disentangled attention and enhanced mask decoder. With those two improvements, DeBERTa out perform RoBERTa on a majority of NLU tasks with 80GB training data.
In [DeBERTa V3](https://arxiv.org/abs/2111.09543), we further improved the efficiency of DeBERTa using ELECTRA-Style pre-training with Gradient Disentangled Embedding Sharing. Compared to DeBERTa, our V3 version significantly improves the model performance on downstream tasks. You can find more technique details about the new model from our [paper](https://arxiv.org/abs/2111.09543).
Please check the [official repository](https://github.com/microsoft/DeBERTa) for more implementation details and updates.
The DeBERTa V3 base model comes with 12 layers and a hidden size of 768. It has only 86M backbone parameters with a vocabulary containing 128K tokens which introduces 98M parameters in the Embedding layer. This model was trained using the 160GB data as DeBERTa V2.
#### Fine-tuning on NLU tasks
We present the dev results on SQuAD 2.0 and MNLI tasks.
| Model |Vocabulary(K)|Backbone #Params(M)| SQuAD 2.0(F1/EM) | MNLI-m/mm(ACC)|
|-------------------|----------|-------------------|-----------|----------|
| RoBERTa-base |50 |86 | 83.7/80.5 | 87.6/- |
| XLNet-base |32 |92 | -/80.2 | 86.8/- |
| ELECTRA-base |30 |86 | -/80.5 | 88.8/ |
| DeBERTa-base |50 |100 | 86.2/83.1| 88.8/88.5|
| DeBERTa-v3-base |128|86 | **88.4/85.4** | **90.6/90.7**|
| DeBERTa-v3-base + SiFT |128|86 | -/- | 91.0/-|
We present the dev results on SQuAD 1.1/2.0 and MNLI tasks.
#### Fine-tuning with HF transformers
```bash
#!/bin/bash
cd transformers/examples/pytorch/text-classification/
pip install datasets
export TASK_NAME=mnli
output_dir="ds_results"
num_gpus=8
batch_size=8
python -m torch.distributed.launch --nproc_per_node=${num_gpus} \
run_glue.py \
--model_name_or_path microsoft/deberta-v3-base \
--task_name $TASK_NAME \
--do_train \
--do_eval \
--evaluation_strategy steps \
--max_seq_length 256 \
--warmup_steps 500 \
--per_device_train_batch_size ${batch_size} \
--learning_rate 2e-5 \
--num_train_epochs 3 \
--output_dir $output_dir \
--overwrite_output_dir \
--logging_steps 1000 \
--logging_dir $output_dir
```
### Citation
If you find DeBERTa useful for your work, please cite the following papers:
``` latex
@misc{he2021debertav3,
title={DeBERTaV3: Improving DeBERTa using ELECTRA-Style Pre-Training with Gradient-Disentangled Embedding Sharing},
author={Pengcheng He and Jianfeng Gao and Weizhu Chen},
year={2021},
eprint={2111.09543},
archivePrefix={arXiv},
primaryClass={cs.CL}
}
```
``` latex
@inproceedings{
he2021deberta,
title={DEBERTA: DECODING-ENHANCED BERT WITH DISENTANGLED ATTENTION},
author={Pengcheng He and Xiaodong Liu and Jianfeng Gao and Weizhu Chen},
booktitle={International Conference on Learning Representations},
year={2021},
url={https://openreview.net/forum?id=XPZIaotutsD}
}
```
|
facebook/esmfold_v1 | facebook | "2023-03-22T17:39:28Z" | 1,919,491 | 22 | transformers | [
"transformers",
"pytorch",
"esm",
"license:mit",
"endpoints_compatible",
"region:us"
] | null | "2022-11-01T18:24:14Z" | ---
license: mit
---
# ESMFold
ESMFold is a state-of-the-art end-to-end protein folding model based on an ESM-2 backbone. It does not require any lookup or MSA step, and therefore does not require any external databases to be present in order to make predictions. As a result, inference time is very significantly faster than AlphaFold2. For details on the model architecture and training, please refer to the [accompanying paper](https://www.science.org/doi/10.1126/science.ade2574).
If you're interested in using ESMFold in practice, please check out the associated [tutorial notebook](https://colab.research.google.com/github/huggingface/notebooks/blob/main/examples/protein_folding.ipynb). |
dslim/bert-base-NER | dslim | "2024-10-08T07:51:39Z" | 1,917,280 | 518 | transformers | [
"transformers",
"pytorch",
"tf",
"jax",
"onnx",
"safetensors",
"bert",
"token-classification",
"en",
"dataset:conll2003",
"arxiv:1810.04805",
"license:mit",
"model-index",
"autotrain_compatible",
"endpoints_compatible",
"region:us"
] | token-classification | "2022-03-02T23:29:05Z" | ---
language: en
datasets:
- conll2003
license: mit
model-index:
- name: dslim/bert-base-NER
results:
- task:
type: token-classification
name: Token Classification
dataset:
name: conll2003
type: conll2003
config: conll2003
split: test
metrics:
- name: Accuracy
type: accuracy
value: 0.9118041001560013
verified: true
- name: Precision
type: precision
value: 0.9211550382257732
verified: true
- name: Recall
type: recall
value: 0.9306415698281261
verified: true
- name: F1
type: f1
value: 0.9258740048459675
verified: true
- name: loss
type: loss
value: 0.48325642943382263
verified: true
---
# bert-base-NER
If my open source models have been useful to you, please consider supporting me in building small, useful AI models for everyone (and help me afford med school / help out my parents financially). Thanks!
<a href="https://www.buymeacoffee.com/dslim" target="_blank"><img src="https://cdn.buymeacoffee.com/buttons/v2/arial-yellow.png" alt="Buy Me A Coffee" style="height: 60px !important;width: 217px !important;" ></a>
## Model description
**bert-base-NER** is a fine-tuned BERT model that is ready to use for **Named Entity Recognition** and achieves **state-of-the-art performance** for the NER task. It has been trained to recognize four types of entities: location (LOC), organizations (ORG), person (PER) and Miscellaneous (MISC).
Specifically, this model is a *bert-base-cased* model that was fine-tuned on the English version of the standard [CoNLL-2003 Named Entity Recognition](https://www.aclweb.org/anthology/W03-0419.pdf) dataset.
If you'd like to use a larger BERT-large model fine-tuned on the same dataset, a [**bert-large-NER**](https://huggingface.co/dslim/bert-large-NER/) version is also available.
### Available NER models
| Model Name | Description | Parameters |
|-------------------|-------------|------------------|
| [distilbert-NER](https://huggingface.co/dslim/distilbert-NER) **(NEW!)** | Fine-tuned DistilBERT - a smaller, faster, lighter version of BERT | 66M |
| [bert-large-NER](https://huggingface.co/dslim/bert-large-NER/) | Fine-tuned bert-large-cased - larger model with slightly better performance | 340M |
| [bert-base-NER](https://huggingface.co/dslim/bert-base-NER)-([uncased](https://huggingface.co/dslim/bert-base-NER-uncased)) | Fine-tuned bert-base, available in both cased and uncased versions | 110M |
## Intended uses & limitations
#### How to use
You can use this model with Transformers *pipeline* for NER.
```python
from transformers import AutoTokenizer, AutoModelForTokenClassification
from transformers import pipeline
tokenizer = AutoTokenizer.from_pretrained("dslim/bert-base-NER")
model = AutoModelForTokenClassification.from_pretrained("dslim/bert-base-NER")
nlp = pipeline("ner", model=model, tokenizer=tokenizer)
example = "My name is Wolfgang and I live in Berlin"
ner_results = nlp(example)
print(ner_results)
```
#### Limitations and bias
This model is limited by its training dataset of entity-annotated news articles from a specific span of time. This may not generalize well for all use cases in different domains. Furthermore, the model occassionally tags subword tokens as entities and post-processing of results may be necessary to handle those cases.
## Training data
This model was fine-tuned on English version of the standard [CoNLL-2003 Named Entity Recognition](https://www.aclweb.org/anthology/W03-0419.pdf) dataset.
The training dataset distinguishes between the beginning and continuation of an entity so that if there are back-to-back entities of the same type, the model can output where the second entity begins. As in the dataset, each token will be classified as one of the following classes:
Abbreviation|Description
-|-
O|Outside of a named entity
B-MISC |Beginning of a miscellaneous entity right after another miscellaneous entity
I-MISC | Miscellaneous entity
B-PER |Beginning of a person’s name right after another person’s name
I-PER |Person’s name
B-ORG |Beginning of an organization right after another organization
I-ORG |organization
B-LOC |Beginning of a location right after another location
I-LOC |Location
### CoNLL-2003 English Dataset Statistics
This dataset was derived from the Reuters corpus which consists of Reuters news stories. You can read more about how this dataset was created in the CoNLL-2003 paper.
#### # of training examples per entity type
Dataset|LOC|MISC|ORG|PER
-|-|-|-|-
Train|7140|3438|6321|6600
Dev|1837|922|1341|1842
Test|1668|702|1661|1617
#### # of articles/sentences/tokens per dataset
Dataset |Articles |Sentences |Tokens
-|-|-|-
Train |946 |14,987 |203,621
Dev |216 |3,466 |51,362
Test |231 |3,684 |46,435
## Training procedure
This model was trained on a single NVIDIA V100 GPU with recommended hyperparameters from the [original BERT paper](https://arxiv.org/pdf/1810.04805) which trained & evaluated the model on CoNLL-2003 NER task.
## Eval results
metric|dev|test
-|-|-
f1 |95.1 |91.3
precision |95.0 |90.7
recall |95.3 |91.9
The test metrics are a little lower than the official Google BERT results which encoded document context & experimented with CRF. More on replicating the original results [here](https://github.com/google-research/bert/issues/223).
### BibTeX entry and citation info
```
@article{DBLP:journals/corr/abs-1810-04805,
author = {Jacob Devlin and
Ming{-}Wei Chang and
Kenton Lee and
Kristina Toutanova},
title = {{BERT:} Pre-training of Deep Bidirectional Transformers for Language
Understanding},
journal = {CoRR},
volume = {abs/1810.04805},
year = {2018},
url = {http://arxiv.org/abs/1810.04805},
archivePrefix = {arXiv},
eprint = {1810.04805},
timestamp = {Tue, 30 Oct 2018 20:39:56 +0100},
biburl = {https://dblp.org/rec/journals/corr/abs-1810-04805.bib},
bibsource = {dblp computer science bibliography, https://dblp.org}
}
```
```
@inproceedings{tjong-kim-sang-de-meulder-2003-introduction,
title = "Introduction to the {C}o{NLL}-2003 Shared Task: Language-Independent Named Entity Recognition",
author = "Tjong Kim Sang, Erik F. and
De Meulder, Fien",
booktitle = "Proceedings of the Seventh Conference on Natural Language Learning at {HLT}-{NAACL} 2003",
year = "2003",
url = "https://www.aclweb.org/anthology/W03-0419",
pages = "142--147",
}
```
|
cross-encoder/ms-marco-MiniLM-L-6-v2 | cross-encoder | "2021-08-05T08:39:38Z" | 1,884,175 | 60 | transformers | [
"transformers",
"pytorch",
"jax",
"bert",
"text-classification",
"license:apache-2.0",
"autotrain_compatible",
"endpoints_compatible",
"region:us"
] | text-classification | "2022-03-02T23:29:05Z" | ---
license: apache-2.0
---
# Cross-Encoder for MS Marco
This model was trained on the [MS Marco Passage Ranking](https://github.com/microsoft/MSMARCO-Passage-Ranking) task.
The model can be used for Information Retrieval: Given a query, encode the query will all possible passages (e.g. retrieved with ElasticSearch). Then sort the passages in a decreasing order. See [SBERT.net Retrieve & Re-rank](https://www.sbert.net/examples/applications/retrieve_rerank/README.html) for more details. The training code is available here: [SBERT.net Training MS Marco](https://github.com/UKPLab/sentence-transformers/tree/master/examples/training/ms_marco)
## Usage with Transformers
```python
from transformers import AutoTokenizer, AutoModelForSequenceClassification
import torch
model = AutoModelForSequenceClassification.from_pretrained('model_name')
tokenizer = AutoTokenizer.from_pretrained('model_name')
features = tokenizer(['How many people live in Berlin?', 'How many people live in Berlin?'], ['Berlin has a population of 3,520,031 registered inhabitants in an area of 891.82 square kilometers.', 'New York City is famous for the Metropolitan Museum of Art.'], padding=True, truncation=True, return_tensors="pt")
model.eval()
with torch.no_grad():
scores = model(**features).logits
print(scores)
```
## Usage with SentenceTransformers
The usage becomes easier when you have [SentenceTransformers](https://www.sbert.net/) installed. Then, you can use the pre-trained models like this:
```python
from sentence_transformers import CrossEncoder
model = CrossEncoder('model_name', max_length=512)
scores = model.predict([('Query', 'Paragraph1'), ('Query', 'Paragraph2') , ('Query', 'Paragraph3')])
```
## Performance
In the following table, we provide various pre-trained Cross-Encoders together with their performance on the [TREC Deep Learning 2019](https://microsoft.github.io/TREC-2019-Deep-Learning/) and the [MS Marco Passage Reranking](https://github.com/microsoft/MSMARCO-Passage-Ranking/) dataset.
| Model-Name | NDCG@10 (TREC DL 19) | MRR@10 (MS Marco Dev) | Docs / Sec |
| ------------- |:-------------| -----| --- |
| **Version 2 models** | | |
| cross-encoder/ms-marco-TinyBERT-L-2-v2 | 69.84 | 32.56 | 9000
| cross-encoder/ms-marco-MiniLM-L-2-v2 | 71.01 | 34.85 | 4100
| cross-encoder/ms-marco-MiniLM-L-4-v2 | 73.04 | 37.70 | 2500
| cross-encoder/ms-marco-MiniLM-L-6-v2 | 74.30 | 39.01 | 1800
| cross-encoder/ms-marco-MiniLM-L-12-v2 | 74.31 | 39.02 | 960
| **Version 1 models** | | |
| cross-encoder/ms-marco-TinyBERT-L-2 | 67.43 | 30.15 | 9000
| cross-encoder/ms-marco-TinyBERT-L-4 | 68.09 | 34.50 | 2900
| cross-encoder/ms-marco-TinyBERT-L-6 | 69.57 | 36.13 | 680
| cross-encoder/ms-marco-electra-base | 71.99 | 36.41 | 340
| **Other models** | | |
| nboost/pt-tinybert-msmarco | 63.63 | 28.80 | 2900
| nboost/pt-bert-base-uncased-msmarco | 70.94 | 34.75 | 340
| nboost/pt-bert-large-msmarco | 73.36 | 36.48 | 100
| Capreolus/electra-base-msmarco | 71.23 | 36.89 | 340
| amberoad/bert-multilingual-passage-reranking-msmarco | 68.40 | 35.54 | 330
| sebastian-hofstaetter/distilbert-cat-margin_mse-T2-msmarco | 72.82 | 37.88 | 720
Note: Runtime was computed on a V100 GPU.
|
BAAI/bge-reranker-large | BAAI | "2024-05-11T13:39:02Z" | 1,852,683 | 340 | transformers | [
"transformers",
"pytorch",
"onnx",
"safetensors",
"xlm-roberta",
"text-classification",
"mteb",
"feature-extraction",
"en",
"zh",
"arxiv:2401.03462",
"arxiv:2312.15503",
"arxiv:2311.13534",
"arxiv:2310.07554",
"arxiv:2309.07597",
"license:mit",
"model-index",
"autotrain_compatible",
"text-embeddings-inference",
"endpoints_compatible",
"region:us"
] | feature-extraction | "2023-09-12T07:39:18Z" | ---
license: mit
language:
- en
- zh
tags:
- mteb
model-index:
- name: bge-reranker-base
results:
- task:
type: Reranking
dataset:
type: C-MTEB/CMedQAv1-reranking
name: MTEB CMedQAv1
config: default
split: test
revision: None
metrics:
- type: map
value: 81.27206722525007
- type: mrr
value: 84.14238095238095
- task:
type: Reranking
dataset:
type: C-MTEB/CMedQAv2-reranking
name: MTEB CMedQAv2
config: default
split: test
revision: None
metrics:
- type: map
value: 84.10369934291236
- type: mrr
value: 86.79376984126984
- task:
type: Reranking
dataset:
type: C-MTEB/Mmarco-reranking
name: MTEB MMarcoReranking
config: default
split: dev
revision: None
metrics:
- type: map
value: 35.4600511272538
- type: mrr
value: 34.60238095238095
- task:
type: Reranking
dataset:
type: C-MTEB/T2Reranking
name: MTEB T2Reranking
config: default
split: dev
revision: None
metrics:
- type: map
value: 67.27728847727172
- type: mrr
value: 77.1315192743764
pipeline_tag: feature-extraction
---
**We have updated the [new reranker](https://github.com/FlagOpen/FlagEmbedding/tree/master/FlagEmbedding/llm_reranker), supporting larger lengths, more languages, and achieving better performance.**
<h1 align="center">FlagEmbedding</h1>
<h4 align="center">
<p>
<a href=#model-list>Model List</a> |
<a href=#frequently-asked-questions>FAQ</a> |
<a href=#usage>Usage</a> |
<a href="#evaluation">Evaluation</a> |
<a href="#train">Train</a> |
<a href="#citation">Citation</a> |
<a href="#license">License</a>
<p>
</h4>
**More details please refer to our Github: [FlagEmbedding](https://github.com/FlagOpen/FlagEmbedding).**
[English](README.md) | [中文](https://github.com/FlagOpen/FlagEmbedding/blob/master/README_zh.md)
FlagEmbedding focuses on retrieval-augmented LLMs, consisting of the following projects currently:
- **Long-Context LLM**: [Activation Beacon](https://github.com/FlagOpen/FlagEmbedding/tree/master/Long_LLM/activation_beacon)
- **Fine-tuning of LM** : [LM-Cocktail](https://github.com/FlagOpen/FlagEmbedding/tree/master/LM_Cocktail)
- **Embedding Model**: [Visualized-BGE](https://github.com/FlagOpen/FlagEmbedding/tree/master/FlagEmbedding/visual), [BGE-M3](https://github.com/FlagOpen/FlagEmbedding/tree/master/FlagEmbedding/BGE_M3), [LLM Embedder](https://github.com/FlagOpen/FlagEmbedding/tree/master/FlagEmbedding/llm_embedder), [BGE Embedding](https://github.com/FlagOpen/FlagEmbedding/tree/master/FlagEmbedding/baai_general_embedding)
- **Reranker Model**: [llm rerankers](https://github.com/FlagOpen/FlagEmbedding/tree/master/FlagEmbedding/llm_reranker), [BGE Reranker](https://github.com/FlagOpen/FlagEmbedding/tree/master/FlagEmbedding/reranker)
- **Benchmark**: [C-MTEB](https://github.com/FlagOpen/FlagEmbedding/tree/master/C_MTEB)
## News
- 3/18/2024: Release new [rerankers](https://github.com/FlagOpen/FlagEmbedding/tree/master/FlagEmbedding/llm_reranker), built upon powerful M3 and LLM (GEMMA and MiniCPM, not so large actually) backbones, supporitng multi-lingual processing and larger inputs, massive improvements of ranking performances on BEIR, C-MTEB/Retrieval, MIRACL, LlamaIndex Evaluation.
- 3/18/2024: Release [Visualized-BGE](https://github.com/FlagOpen/FlagEmbedding/tree/master/FlagEmbedding/visual), equipping BGE with visual capabilities. Visualized-BGE can be utilized to generate embeddings for hybrid image-text data.
- 1/30/2024: Release **BGE-M3**, a new member to BGE model series! M3 stands for **M**ulti-linguality (100+ languages), **M**ulti-granularities (input length up to 8192), **M**ulti-Functionality (unification of dense, lexical, multi-vec/colbert retrieval).
It is the first embedding model which supports all three retrieval methods, achieving new SOTA on multi-lingual (MIRACL) and cross-lingual (MKQA) benchmarks.
[Technical Report](https://github.com/FlagOpen/FlagEmbedding/blob/master/FlagEmbedding/BGE_M3/BGE_M3.pdf) and [Code](https://github.com/FlagOpen/FlagEmbedding/tree/master/FlagEmbedding/BGE_M3). :fire:
- 1/9/2024: Release [Activation-Beacon](https://github.com/FlagOpen/FlagEmbedding/tree/master/Long_LLM/activation_beacon), an effective, efficient, compatible, and low-cost (training) method to extend the context length of LLM. [Technical Report](https://arxiv.org/abs/2401.03462) :fire:
- 12/24/2023: Release **LLaRA**, a LLaMA-7B based dense retriever, leading to state-of-the-art performances on MS MARCO and BEIR. Model and code will be open-sourced. Please stay tuned. [Technical Report](https://arxiv.org/abs/2312.15503)
- 11/23/2023: Release [LM-Cocktail](https://github.com/FlagOpen/FlagEmbedding/tree/master/LM_Cocktail), a method to maintain general capabilities during fine-tuning by merging multiple language models. [Technical Report](https://arxiv.org/abs/2311.13534) :fire:
- 10/12/2023: Release [LLM-Embedder](https://github.com/FlagOpen/FlagEmbedding/tree/master/FlagEmbedding/llm_embedder), a unified embedding model to support diverse retrieval augmentation needs for LLMs. [Technical Report](https://arxiv.org/pdf/2310.07554.pdf)
- 09/15/2023: The [technical report](https://arxiv.org/pdf/2309.07597.pdf) of BGE has been released
- 09/15/2023: The [massive training data](https://data.baai.ac.cn/details/BAAI-MTP) of BGE has been released
- 09/12/2023: New models:
- **New reranker model**: release cross-encoder models `BAAI/bge-reranker-base` and `BAAI/bge-reranker-large`, which are more powerful than embedding model. We recommend to use/fine-tune them to re-rank top-k documents returned by embedding models.
- **update embedding model**: release `bge-*-v1.5` embedding model to alleviate the issue of the similarity distribution, and enhance its retrieval ability without instruction.
<details>
<summary>More</summary>
<!-- ### More -->
- 09/07/2023: Update [fine-tune code](https://github.com/FlagOpen/FlagEmbedding/blob/master/FlagEmbedding/baai_general_embedding/README.md): Add script to mine hard negatives and support adding instruction during fine-tuning.
- 08/09/2023: BGE Models are integrated into **Langchain**, you can use it like [this](#using-langchain); C-MTEB **leaderboard** is [available](https://huggingface.co/spaces/mteb/leaderboard).
- 08/05/2023: Release base-scale and small-scale models, **best performance among the models of the same size 🤗**
- 08/02/2023: Release `bge-large-*`(short for BAAI General Embedding) Models, **rank 1st on MTEB and C-MTEB benchmark!** :tada: :tada:
- 08/01/2023: We release the [Chinese Massive Text Embedding Benchmark](https://github.com/FlagOpen/FlagEmbedding/blob/master/C_MTEB) (**C-MTEB**), consisting of 31 test dataset.
</details>
## Model List
`bge` is short for `BAAI general embedding`.
| Model | Language | | Description | query instruction for retrieval [1] |
|:-------------------------------|:--------:| :--------:| :--------:|:--------:|
| [BAAI/bge-m3](https://huggingface.co/BAAI/bge-m3) | Multilingual | [Inference](https://github.com/FlagOpen/FlagEmbedding/tree/master/FlagEmbedding/BGE_M3#usage) [Fine-tune](https://github.com/FlagOpen/FlagEmbedding/tree/master/FlagEmbedding/BGE_M3) | Multi-Functionality(dense retrieval, sparse retrieval, multi-vector(colbert)), Multi-Linguality, and Multi-Granularity(8192 tokens) | |
| [BAAI/llm-embedder](https://huggingface.co/BAAI/llm-embedder) | English | [Inference](./FlagEmbedding/llm_embedder/README.md) [Fine-tune](./FlagEmbedding/llm_embedder/README.md) | a unified embedding model to support diverse retrieval augmentation needs for LLMs | See [README](./FlagEmbedding/llm_embedder/README.md) |
| [BAAI/bge-reranker-large](https://huggingface.co/BAAI/bge-reranker-large) | Chinese and English | [Inference](#usage-for-reranker) [Fine-tune](https://github.com/FlagOpen/FlagEmbedding/tree/master/examples/reranker) | a cross-encoder model which is more accurate but less efficient [2] | |
| [BAAI/bge-reranker-base](https://huggingface.co/BAAI/bge-reranker-base) | Chinese and English | [Inference](#usage-for-reranker) [Fine-tune](https://github.com/FlagOpen/FlagEmbedding/tree/master/examples/reranker) | a cross-encoder model which is more accurate but less efficient [2] | |
| [BAAI/bge-large-en-v1.5](https://huggingface.co/BAAI/bge-large-en-v1.5) | English | [Inference](#usage-for-embedding-model) [Fine-tune](https://github.com/FlagOpen/FlagEmbedding/tree/master/examples/finetune) | version 1.5 with more reasonable similarity distribution | `Represent this sentence for searching relevant passages: ` |
| [BAAI/bge-base-en-v1.5](https://huggingface.co/BAAI/bge-base-en-v1.5) | English | [Inference](#usage-for-embedding-model) [Fine-tune](https://github.com/FlagOpen/FlagEmbedding/tree/master/examples/finetune) | version 1.5 with more reasonable similarity distribution | `Represent this sentence for searching relevant passages: ` |
| [BAAI/bge-small-en-v1.5](https://huggingface.co/BAAI/bge-small-en-v1.5) | English | [Inference](#usage-for-embedding-model) [Fine-tune](https://github.com/FlagOpen/FlagEmbedding/tree/master/examples/finetune) | version 1.5 with more reasonable similarity distribution | `Represent this sentence for searching relevant passages: ` |
| [BAAI/bge-large-zh-v1.5](https://huggingface.co/BAAI/bge-large-zh-v1.5) | Chinese | [Inference](#usage-for-embedding-model) [Fine-tune](https://github.com/FlagOpen/FlagEmbedding/tree/master/examples/finetune) | version 1.5 with more reasonable similarity distribution | `为这个句子生成表示以用于检索相关文章:` |
| [BAAI/bge-base-zh-v1.5](https://huggingface.co/BAAI/bge-base-zh-v1.5) | Chinese | [Inference](#usage-for-embedding-model) [Fine-tune](https://github.com/FlagOpen/FlagEmbedding/tree/master/examples/finetune) | version 1.5 with more reasonable similarity distribution | `为这个句子生成表示以用于检索相关文章:` |
| [BAAI/bge-small-zh-v1.5](https://huggingface.co/BAAI/bge-small-zh-v1.5) | Chinese | [Inference](#usage-for-embedding-model) [Fine-tune](https://github.com/FlagOpen/FlagEmbedding/tree/master/examples/finetune) | version 1.5 with more reasonable similarity distribution | `为这个句子生成表示以用于检索相关文章:` |
| [BAAI/bge-large-en](https://huggingface.co/BAAI/bge-large-en) | English | [Inference](#usage-for-embedding-model) [Fine-tune](https://github.com/FlagOpen/FlagEmbedding/tree/master/examples/finetune) | :trophy: rank **1st** in [MTEB](https://huggingface.co/spaces/mteb/leaderboard) leaderboard | `Represent this sentence for searching relevant passages: ` |
| [BAAI/bge-base-en](https://huggingface.co/BAAI/bge-base-en) | English | [Inference](#usage-for-embedding-model) [Fine-tune](https://github.com/FlagOpen/FlagEmbedding/tree/master/examples/finetune) | a base-scale model but with similar ability to `bge-large-en` | `Represent this sentence for searching relevant passages: ` |
| [BAAI/bge-small-en](https://huggingface.co/BAAI/bge-small-en) | English | [Inference](#usage-for-embedding-model) [Fine-tune](https://github.com/FlagOpen/FlagEmbedding/tree/master/examples/finetune) |a small-scale model but with competitive performance | `Represent this sentence for searching relevant passages: ` |
| [BAAI/bge-large-zh](https://huggingface.co/BAAI/bge-large-zh) | Chinese | [Inference](#usage-for-embedding-model) [Fine-tune](https://github.com/FlagOpen/FlagEmbedding/tree/master/examples/finetune) | :trophy: rank **1st** in [C-MTEB](https://github.com/FlagOpen/FlagEmbedding/tree/master/C_MTEB) benchmark | `为这个句子生成表示以用于检索相关文章:` |
| [BAAI/bge-base-zh](https://huggingface.co/BAAI/bge-base-zh) | Chinese | [Inference](#usage-for-embedding-model) [Fine-tune](https://github.com/FlagOpen/FlagEmbedding/tree/master/examples/finetune) | a base-scale model but with similar ability to `bge-large-zh` | `为这个句子生成表示以用于检索相关文章:` |
| [BAAI/bge-small-zh](https://huggingface.co/BAAI/bge-small-zh) | Chinese | [Inference](#usage-for-embedding-model) [Fine-tune](https://github.com/FlagOpen/FlagEmbedding/tree/master/examples/finetune) | a small-scale model but with competitive performance | `为这个句子生成表示以用于检索相关文章:` |
[1\]: If you need to search the relevant passages to a query, we suggest to add the instruction to the query; in other cases, no instruction is needed, just use the original query directly. In all cases, **no instruction** needs to be added to passages.
[2\]: Different from embedding model, reranker uses question and document as input and directly output similarity instead of embedding. To balance the accuracy and time cost, cross-encoder is widely used to re-rank top-k documents retrieved by other simple models.
For examples, use bge embedding model to retrieve top 100 relevant documents, and then use bge reranker to re-rank the top 100 document to get the final top-3 results.
All models have been uploaded to Huggingface Hub, and you can see them at https://huggingface.co/BAAI.
If you cannot open the Huggingface Hub, you also can download the models at https://model.baai.ac.cn/models .
## Frequently asked questions
<details>
<summary>1. How to fine-tune bge embedding model?</summary>
<!-- ### How to fine-tune bge embedding model? -->
Following this [example](https://github.com/FlagOpen/FlagEmbedding/tree/master/examples/finetune) to prepare data and fine-tune your model.
Some suggestions:
- Mine hard negatives following this [example](https://github.com/FlagOpen/FlagEmbedding/tree/master/examples/finetune#hard-negatives), which can improve the retrieval performance.
- If you pre-train bge on your data, the pre-trained model cannot be directly used to calculate similarity, and it must be fine-tuned with contrastive learning before computing similarity.
- If the accuracy of the fine-tuned model is still not high, it is recommended to use/fine-tune the cross-encoder model (bge-reranker) to re-rank top-k results.
Hard negatives also are needed to fine-tune reranker. Refer to this [example](https://github.com/FlagOpen/FlagEmbedding/tree/master/examples/reranker) for the fine-tuning for reranker
</details>
<details>
<summary>2. The similarity score between two dissimilar sentences is higher than 0.5</summary>
<!-- ### The similarity score between two dissimilar sentences is higher than 0.5 -->
**Suggest to use bge v1.5, which alleviates the issue of the similarity distribution.**
Since we finetune the models by contrastive learning with a temperature of 0.01,
the similarity distribution of the current BGE model is about in the interval \[0.6, 1\].
So a similarity score greater than 0.5 does not indicate that the two sentences are similar.
For downstream tasks, such as passage retrieval or semantic similarity,
**what matters is the relative order of the scores, not the absolute value.**
If you need to filter similar sentences based on a similarity threshold,
please select an appropriate similarity threshold based on the similarity distribution on your data (such as 0.8, 0.85, or even 0.9).
</details>
<details>
<summary>3. When does the query instruction need to be used</summary>
<!-- ### When does the query instruction need to be used -->
For the `bge-*-v1.5`, we improve its retrieval ability when not using instruction.
No instruction only has a slight degradation in retrieval performance compared with using instruction.
So you can generate embedding without instruction in all cases for convenience.
For a retrieval task that uses short queries to find long related documents,
it is recommended to add instructions for these short queries.
**The best method to decide whether to add instructions for queries is choosing the setting that achieves better performance on your task.**
In all cases, the documents/passages do not need to add the instruction.
</details>
## Usage
### Usage for Embedding Model
Here are some examples for using `bge` models with
[FlagEmbedding](#using-flagembedding), [Sentence-Transformers](#using-sentence-transformers), [Langchain](#using-langchain), or [Huggingface Transformers](#using-huggingface-transformers).
#### Using FlagEmbedding
```
pip install -U FlagEmbedding
```
If it doesn't work for you, you can see [FlagEmbedding](https://github.com/FlagOpen/FlagEmbedding/blob/master/FlagEmbedding/baai_general_embedding/README.md) for more methods to install FlagEmbedding.
```python
from FlagEmbedding import FlagModel
sentences_1 = ["样例数据-1", "样例数据-2"]
sentences_2 = ["样例数据-3", "样例数据-4"]
model = FlagModel('BAAI/bge-large-zh-v1.5',
query_instruction_for_retrieval="为这个句子生成表示以用于检索相关文章:",
use_fp16=True) # Setting use_fp16 to True speeds up computation with a slight performance degradation
embeddings_1 = model.encode(sentences_1)
embeddings_2 = model.encode(sentences_2)
similarity = embeddings_1 @ embeddings_2.T
print(similarity)
# for s2p(short query to long passage) retrieval task, suggest to use encode_queries() which will automatically add the instruction to each query
# corpus in retrieval task can still use encode() or encode_corpus(), since they don't need instruction
queries = ['query_1', 'query_2']
passages = ["样例文档-1", "样例文档-2"]
q_embeddings = model.encode_queries(queries)
p_embeddings = model.encode(passages)
scores = q_embeddings @ p_embeddings.T
```
For the value of the argument `query_instruction_for_retrieval`, see [Model List](https://github.com/FlagOpen/FlagEmbedding/tree/master#model-list).
By default, FlagModel will use all available GPUs when encoding. Please set `os.environ["CUDA_VISIBLE_DEVICES"]` to select specific GPUs.
You also can set `os.environ["CUDA_VISIBLE_DEVICES"]=""` to make all GPUs unavailable.
#### Using Sentence-Transformers
You can also use the `bge` models with [sentence-transformers](https://www.SBERT.net):
```
pip install -U sentence-transformers
```
```python
from sentence_transformers import SentenceTransformer
sentences_1 = ["样例数据-1", "样例数据-2"]
sentences_2 = ["样例数据-3", "样例数据-4"]
model = SentenceTransformer('BAAI/bge-large-zh-v1.5')
embeddings_1 = model.encode(sentences_1, normalize_embeddings=True)
embeddings_2 = model.encode(sentences_2, normalize_embeddings=True)
similarity = embeddings_1 @ embeddings_2.T
print(similarity)
```
For s2p(short query to long passage) retrieval task,
each short query should start with an instruction (instructions see [Model List](https://github.com/FlagOpen/FlagEmbedding/tree/master#model-list)).
But the instruction is not needed for passages.
```python
from sentence_transformers import SentenceTransformer
queries = ['query_1', 'query_2']
passages = ["样例文档-1", "样例文档-2"]
instruction = "为这个句子生成表示以用于检索相关文章:"
model = SentenceTransformer('BAAI/bge-large-zh-v1.5')
q_embeddings = model.encode([instruction+q for q in queries], normalize_embeddings=True)
p_embeddings = model.encode(passages, normalize_embeddings=True)
scores = q_embeddings @ p_embeddings.T
```
#### Using Langchain
You can use `bge` in langchain like this:
```python
from langchain.embeddings import HuggingFaceBgeEmbeddings
model_name = "BAAI/bge-large-en-v1.5"
model_kwargs = {'device': 'cuda'}
encode_kwargs = {'normalize_embeddings': True} # set True to compute cosine similarity
model = HuggingFaceBgeEmbeddings(
model_name=model_name,
model_kwargs=model_kwargs,
encode_kwargs=encode_kwargs,
query_instruction="为这个句子生成表示以用于检索相关文章:"
)
model.query_instruction = "为这个句子生成表示以用于检索相关文章:"
```
#### Using HuggingFace Transformers
With the transformers package, you can use the model like this: First, you pass your input through the transformer model, then you select the last hidden state of the first token (i.e., [CLS]) as the sentence embedding.
```python
from transformers import AutoTokenizer, AutoModel
import torch
# Sentences we want sentence embeddings for
sentences = ["样例数据-1", "样例数据-2"]
# Load model from HuggingFace Hub
tokenizer = AutoTokenizer.from_pretrained('BAAI/bge-large-zh-v1.5')
model = AutoModel.from_pretrained('BAAI/bge-large-zh-v1.5')
model.eval()
# Tokenize sentences
encoded_input = tokenizer(sentences, padding=True, truncation=True, return_tensors='pt')
# for s2p(short query to long passage) retrieval task, add an instruction to query (not add instruction for passages)
# encoded_input = tokenizer([instruction + q for q in queries], padding=True, truncation=True, return_tensors='pt')
# Compute token embeddings
with torch.no_grad():
model_output = model(**encoded_input)
# Perform pooling. In this case, cls pooling.
sentence_embeddings = model_output[0][:, 0]
# normalize embeddings
sentence_embeddings = torch.nn.functional.normalize(sentence_embeddings, p=2, dim=1)
print("Sentence embeddings:", sentence_embeddings)
```
### Usage for Reranker
Different from embedding model, reranker uses question and document as input and directly output similarity instead of embedding.
You can get a relevance score by inputting query and passage to the reranker.
The reranker is optimized based cross-entropy loss, so the relevance score is not bounded to a specific range.
#### Using FlagEmbedding
```
pip install -U FlagEmbedding
```
Get relevance scores (higher scores indicate more relevance):
```python
from FlagEmbedding import FlagReranker
reranker = FlagReranker('BAAI/bge-reranker-large', use_fp16=True) # Setting use_fp16 to True speeds up computation with a slight performance degradation
score = reranker.compute_score(['query', 'passage'])
print(score)
scores = reranker.compute_score([['what is panda?', 'hi'], ['what is panda?', 'The giant panda (Ailuropoda melanoleuca), sometimes called a panda bear or simply panda, is a bear species endemic to China.']])
print(scores)
```
#### Using Huggingface transformers
```python
import torch
from transformers import AutoModelForSequenceClassification, AutoTokenizer
tokenizer = AutoTokenizer.from_pretrained('BAAI/bge-reranker-large')
model = AutoModelForSequenceClassification.from_pretrained('BAAI/bge-reranker-large')
model.eval()
pairs = [['what is panda?', 'hi'], ['what is panda?', 'The giant panda (Ailuropoda melanoleuca), sometimes called a panda bear or simply panda, is a bear species endemic to China.']]
with torch.no_grad():
inputs = tokenizer(pairs, padding=True, truncation=True, return_tensors='pt', max_length=512)
scores = model(**inputs, return_dict=True).logits.view(-1, ).float()
print(scores)
```
#### Usage reranker with the ONNX files
```python
from optimum.onnxruntime import ORTModelForSequenceClassification # type: ignore
import torch
from transformers import AutoModelForSequenceClassification, AutoTokenizer
tokenizer = AutoTokenizer.from_pretrained('BAAI/bge-reranker-large')
model = AutoModelForSequenceClassification.from_pretrained('BAAI/bge-reranker-base')
model_ort = ORTModelForSequenceClassification.from_pretrained('BAAI/bge-reranker-base', file_name="onnx/model.onnx")
# Sentences we want sentence embeddings for
pairs = [['what is panda?', 'hi'], ['what is panda?', 'The giant panda (Ailuropoda melanoleuca), sometimes called a panda bear or simply panda, is a bear species endemic to China.']]
# Tokenize sentences
encoded_input = tokenizer(pairs, padding=True, truncation=True, return_tensors='pt')
scores_ort = model_ort(**encoded_input, return_dict=True).logits.view(-1, ).float()
# Compute token embeddings
with torch.inference_mode():
scores = model_ort(**encoded_input, return_dict=True).logits.view(-1, ).float()
# scores and scores_ort are identical
```
#### Usage reranker with infinity
Its also possible to deploy the onnx/torch files with the [infinity_emb](https://github.com/michaelfeil/infinity) pip package.
```python
import asyncio
from infinity_emb import AsyncEmbeddingEngine, EngineArgs
query='what is a panda?'
docs = ['The giant panda (Ailuropoda melanoleuca), sometimes called a panda bear', "Paris is in France."]
engine = AsyncEmbeddingEngine.from_args(
EngineArgs(model_name_or_path = "BAAI/bge-reranker-base", device="cpu", engine="torch" # or engine="optimum" for onnx
))
async def main():
async with engine:
ranking, usage = await engine.rerank(query=query, docs=docs)
print(list(zip(ranking, docs)))
asyncio.run(main())
```
## Evaluation
`baai-general-embedding` models achieve **state-of-the-art performance on both MTEB and C-MTEB leaderboard!**
For more details and evaluation tools see our [scripts](https://github.com/FlagOpen/FlagEmbedding/blob/master/C_MTEB/README.md).
- **MTEB**:
| Model Name | Dimension | Sequence Length | Average (56) | Retrieval (15) |Clustering (11) | Pair Classification (3) | Reranking (4) | STS (10) | Summarization (1) | Classification (12) |
|:----:|:---:|:---:|:---:|:---:|:---:|:---:|:---:|:---:|:---:|:---:|
| [BAAI/bge-large-en-v1.5](https://huggingface.co/BAAI/bge-large-en-v1.5) | 1024 | 512 | **64.23** | **54.29** | 46.08 | 87.12 | 60.03 | 83.11 | 31.61 | 75.97 |
| [BAAI/bge-base-en-v1.5](https://huggingface.co/BAAI/bge-base-en-v1.5) | 768 | 512 | 63.55 | 53.25 | 45.77 | 86.55 | 58.86 | 82.4 | 31.07 | 75.53 |
| [BAAI/bge-small-en-v1.5](https://huggingface.co/BAAI/bge-small-en-v1.5) | 384 | 512 | 62.17 |51.68 | 43.82 | 84.92 | 58.36 | 81.59 | 30.12 | 74.14 |
| [bge-large-en](https://huggingface.co/BAAI/bge-large-en) | 1024 | 512 | 63.98 | 53.9 | 46.98 | 85.8 | 59.48 | 81.56 | 32.06 | 76.21 |
| [bge-base-en](https://huggingface.co/BAAI/bge-base-en) | 768 | 512 | 63.36 | 53.0 | 46.32 | 85.86 | 58.7 | 81.84 | 29.27 | 75.27 |
| [gte-large](https://huggingface.co/thenlper/gte-large) | 1024 | 512 | 63.13 | 52.22 | 46.84 | 85.00 | 59.13 | 83.35 | 31.66 | 73.33 |
| [gte-base](https://huggingface.co/thenlper/gte-base) | 768 | 512 | 62.39 | 51.14 | 46.2 | 84.57 | 58.61 | 82.3 | 31.17 | 73.01 |
| [e5-large-v2](https://huggingface.co/intfloat/e5-large-v2) | 1024| 512 | 62.25 | 50.56 | 44.49 | 86.03 | 56.61 | 82.05 | 30.19 | 75.24 |
| [bge-small-en](https://huggingface.co/BAAI/bge-small-en) | 384 | 512 | 62.11 | 51.82 | 44.31 | 83.78 | 57.97 | 80.72 | 30.53 | 74.37 |
| [instructor-xl](https://huggingface.co/hkunlp/instructor-xl) | 768 | 512 | 61.79 | 49.26 | 44.74 | 86.62 | 57.29 | 83.06 | 32.32 | 61.79 |
| [e5-base-v2](https://huggingface.co/intfloat/e5-base-v2) | 768 | 512 | 61.5 | 50.29 | 43.80 | 85.73 | 55.91 | 81.05 | 30.28 | 73.84 |
| [gte-small](https://huggingface.co/thenlper/gte-small) | 384 | 512 | 61.36 | 49.46 | 44.89 | 83.54 | 57.7 | 82.07 | 30.42 | 72.31 |
| [text-embedding-ada-002](https://platform.openai.com/docs/guides/embeddings) | 1536 | 8192 | 60.99 | 49.25 | 45.9 | 84.89 | 56.32 | 80.97 | 30.8 | 70.93 |
| [e5-small-v2](https://huggingface.co/intfloat/e5-base-v2) | 384 | 512 | 59.93 | 49.04 | 39.92 | 84.67 | 54.32 | 80.39 | 31.16 | 72.94 |
| [sentence-t5-xxl](https://huggingface.co/sentence-transformers/sentence-t5-xxl) | 768 | 512 | 59.51 | 42.24 | 43.72 | 85.06 | 56.42 | 82.63 | 30.08 | 73.42 |
| [all-mpnet-base-v2](https://huggingface.co/sentence-transformers/all-mpnet-base-v2) | 768 | 514 | 57.78 | 43.81 | 43.69 | 83.04 | 59.36 | 80.28 | 27.49 | 65.07 |
| [sgpt-bloom-7b1-msmarco](https://huggingface.co/bigscience/sgpt-bloom-7b1-msmarco) | 4096 | 2048 | 57.59 | 48.22 | 38.93 | 81.9 | 55.65 | 77.74 | 33.6 | 66.19 |
- **C-MTEB**:
We create the benchmark C-MTEB for Chinese text embedding which consists of 31 datasets from 6 tasks.
Please refer to [C_MTEB](https://github.com/FlagOpen/FlagEmbedding/blob/master/C_MTEB/README.md) for a detailed introduction.
| Model | Embedding dimension | Avg | Retrieval | STS | PairClassification | Classification | Reranking | Clustering |
|:-------------------------------|:--------:|:--------:|:--------:|:--------:|:--------:|:--------:|:--------:|:--------:|
| [**BAAI/bge-large-zh-v1.5**](https://huggingface.co/BAAI/bge-large-zh-v1.5) | 1024 | **64.53** | 70.46 | 56.25 | 81.6 | 69.13 | 65.84 | 48.99 |
| [BAAI/bge-base-zh-v1.5](https://huggingface.co/BAAI/bge-base-zh-v1.5) | 768 | 63.13 | 69.49 | 53.72 | 79.75 | 68.07 | 65.39 | 47.53 |
| [BAAI/bge-small-zh-v1.5](https://huggingface.co/BAAI/bge-small-zh-v1.5) | 512 | 57.82 | 61.77 | 49.11 | 70.41 | 63.96 | 60.92 | 44.18 |
| [BAAI/bge-large-zh](https://huggingface.co/BAAI/bge-large-zh) | 1024 | 64.20 | 71.53 | 54.98 | 78.94 | 68.32 | 65.11 | 48.39 |
| [bge-large-zh-noinstruct](https://huggingface.co/BAAI/bge-large-zh-noinstruct) | 1024 | 63.53 | 70.55 | 53 | 76.77 | 68.58 | 64.91 | 50.01 |
| [BAAI/bge-base-zh](https://huggingface.co/BAAI/bge-base-zh) | 768 | 62.96 | 69.53 | 54.12 | 77.5 | 67.07 | 64.91 | 47.63 |
| [multilingual-e5-large](https://huggingface.co/intfloat/multilingual-e5-large) | 1024 | 58.79 | 63.66 | 48.44 | 69.89 | 67.34 | 56.00 | 48.23 |
| [BAAI/bge-small-zh](https://huggingface.co/BAAI/bge-small-zh) | 512 | 58.27 | 63.07 | 49.45 | 70.35 | 63.64 | 61.48 | 45.09 |
| [m3e-base](https://huggingface.co/moka-ai/m3e-base) | 768 | 57.10 | 56.91 | 50.47 | 63.99 | 67.52 | 59.34 | 47.68 |
| [m3e-large](https://huggingface.co/moka-ai/m3e-large) | 1024 | 57.05 | 54.75 | 50.42 | 64.3 | 68.2 | 59.66 | 48.88 |
| [multilingual-e5-base](https://huggingface.co/intfloat/multilingual-e5-base) | 768 | 55.48 | 61.63 | 46.49 | 67.07 | 65.35 | 54.35 | 40.68 |
| [multilingual-e5-small](https://huggingface.co/intfloat/multilingual-e5-small) | 384 | 55.38 | 59.95 | 45.27 | 66.45 | 65.85 | 53.86 | 45.26 |
| [text-embedding-ada-002(OpenAI)](https://platform.openai.com/docs/guides/embeddings/what-are-embeddings) | 1536 | 53.02 | 52.0 | 43.35 | 69.56 | 64.31 | 54.28 | 45.68 |
| [luotuo](https://huggingface.co/silk-road/luotuo-bert-medium) | 1024 | 49.37 | 44.4 | 42.78 | 66.62 | 61 | 49.25 | 44.39 |
| [text2vec-base](https://huggingface.co/shibing624/text2vec-base-chinese) | 768 | 47.63 | 38.79 | 43.41 | 67.41 | 62.19 | 49.45 | 37.66 |
| [text2vec-large](https://huggingface.co/GanymedeNil/text2vec-large-chinese) | 1024 | 47.36 | 41.94 | 44.97 | 70.86 | 60.66 | 49.16 | 30.02 |
- **Reranking**:
See [C_MTEB](https://github.com/FlagOpen/FlagEmbedding/blob/master/C_MTEB/) for evaluation script.
| Model | T2Reranking | T2RerankingZh2En\* | T2RerankingEn2Zh\* | MMarcoReranking | CMedQAv1 | CMedQAv2 | Avg |
|:-------------------------------|:--------:|:--------:|:--------:|:--------:|:--------:|:--------:|:--------:|
| text2vec-base-multilingual | 64.66 | 62.94 | 62.51 | 14.37 | 48.46 | 48.6 | 50.26 |
| multilingual-e5-small | 65.62 | 60.94 | 56.41 | 29.91 | 67.26 | 66.54 | 57.78 |
| multilingual-e5-large | 64.55 | 61.61 | 54.28 | 28.6 | 67.42 | 67.92 | 57.4 |
| multilingual-e5-base | 64.21 | 62.13 | 54.68 | 29.5 | 66.23 | 66.98 | 57.29 |
| m3e-base | 66.03 | 62.74 | 56.07 | 17.51 | 77.05 | 76.76 | 59.36 |
| m3e-large | 66.13 | 62.72 | 56.1 | 16.46 | 77.76 | 78.27 | 59.57 |
| bge-base-zh-v1.5 | 66.49 | 63.25 | 57.02 | 29.74 | 80.47 | 84.88 | 63.64 |
| bge-large-zh-v1.5 | 65.74 | 63.39 | 57.03 | 28.74 | 83.45 | 85.44 | 63.97 |
| [BAAI/bge-reranker-base](https://huggingface.co/BAAI/bge-reranker-base) | 67.28 | 63.95 | 60.45 | 35.46 | 81.26 | 84.1 | 65.42 |
| [BAAI/bge-reranker-large](https://huggingface.co/BAAI/bge-reranker-large) | 67.6 | 64.03 | 61.44 | 37.16 | 82.15 | 84.18 | 66.09 |
\* : T2RerankingZh2En and T2RerankingEn2Zh are cross-language retrieval tasks
## Train
### BAAI Embedding
We pre-train the models using [retromae](https://github.com/staoxiao/RetroMAE) and train them on large-scale pairs data using contrastive learning.
**You can fine-tune the embedding model on your data following our [examples](https://github.com/FlagOpen/FlagEmbedding/tree/master/examples/finetune).**
We also provide a [pre-train example](https://github.com/FlagOpen/FlagEmbedding/tree/master/examples/pretrain).
Note that the goal of pre-training is to reconstruct the text, and the pre-trained model cannot be used for similarity calculation directly, it needs to be fine-tuned.
More training details for bge see [baai_general_embedding](https://github.com/FlagOpen/FlagEmbedding/blob/master/FlagEmbedding/baai_general_embedding/README.md).
### BGE Reranker
Cross-encoder will perform full-attention over the input pair,
which is more accurate than embedding model (i.e., bi-encoder) but more time-consuming than embedding model.
Therefore, it can be used to re-rank the top-k documents returned by embedding model.
We train the cross-encoder on a multilingual pair data,
The data format is the same as embedding model, so you can fine-tune it easily following our [example](https://github.com/FlagOpen/FlagEmbedding/tree/master/examples/reranker).
More details please refer to [./FlagEmbedding/reranker/README.md](https://github.com/FlagOpen/FlagEmbedding/tree/master/FlagEmbedding/reranker)
## Citation
If you find this repository useful, please consider giving a star :star: and citation
```
@misc{bge_embedding,
title={C-Pack: Packaged Resources To Advance General Chinese Embedding},
author={Shitao Xiao and Zheng Liu and Peitian Zhang and Niklas Muennighoff},
year={2023},
eprint={2309.07597},
archivePrefix={arXiv},
primaryClass={cs.CL}
}
```
## License
FlagEmbedding is licensed under the [MIT License](https://github.com/FlagOpen/FlagEmbedding/blob/master/LICENSE). The released models can be used for commercial purposes free of charge. |
microsoft/infoxlm-large | microsoft | "2021-08-04T11:43:05Z" | 1,790,177 | 11 | transformers | [
"transformers",
"pytorch",
"xlm-roberta",
"fill-mask",
"arxiv:2007.07834",
"autotrain_compatible",
"endpoints_compatible",
"region:us"
] | fill-mask | "2022-03-02T23:29:05Z" | # InfoXLM
**InfoXLM** (NAACL 2021, [paper](https://arxiv.org/pdf/2007.07834.pdf), [repo](https://github.com/microsoft/unilm/tree/master/infoxlm), [model](https://huggingface.co/microsoft/infoxlm-base)) InfoXLM: An Information-Theoretic Framework for Cross-Lingual Language Model Pre-Training.
**MD5**
```
05b95b7d977450b364f8ea3269391953 config.json
c19438359fed6d36b0c1bbb107929579 pytorch_model.bin
bf25eb5120ad92ef5c7d8596b5dc4046 sentencepiece.bpe.model
eedbd60a7268b9fc45981b849664f747 tokenizer.json
```
**BibTeX**
```
@inproceedings{chi-etal-2021-infoxlm,
title = "{I}nfo{XLM}: An Information-Theoretic Framework for Cross-Lingual Language Model Pre-Training",
author={Chi, Zewen and Dong, Li and Wei, Furu and Yang, Nan and Singhal, Saksham and Wang, Wenhui and Song, Xia and Mao, Xian-Ling and Huang, Heyan and Zhou, Ming},
booktitle = "Proceedings of the 2021 Conference of the North American Chapter of the Association for Computational Linguistics: Human Language Technologies",
month = jun,
year = "2021",
address = "Online",
publisher = "Association for Computational Linguistics",
url = "https://aclanthology.org/2021.naacl-main.280",
doi = "10.18653/v1/2021.naacl-main.280",
pages = "3576--3588",}
``` |
jonatasgrosman/wav2vec2-large-xlsr-53-dutch | jonatasgrosman | "2022-12-14T01:58:20Z" | 1,737,726 | 9 | transformers | [
"transformers",
"pytorch",
"jax",
"wav2vec2",
"automatic-speech-recognition",
"audio",
"hf-asr-leaderboard",
"mozilla-foundation/common_voice_6_0",
"nl",
"robust-speech-event",
"speech",
"xlsr-fine-tuning-week",
"dataset:common_voice",
"dataset:mozilla-foundation/common_voice_6_0",
"doi:10.57967/hf/0203",
"license:apache-2.0",
"model-index",
"endpoints_compatible",
"region:us"
] | automatic-speech-recognition | "2022-03-02T23:29:05Z" | ---
language: nl
license: apache-2.0
datasets:
- common_voice
- mozilla-foundation/common_voice_6_0
metrics:
- wer
- cer
tags:
- audio
- automatic-speech-recognition
- hf-asr-leaderboard
- mozilla-foundation/common_voice_6_0
- nl
- robust-speech-event
- speech
- xlsr-fine-tuning-week
model-index:
- name: XLSR Wav2Vec2 Dutch by Jonatas Grosman
results:
- task:
name: Automatic Speech Recognition
type: automatic-speech-recognition
dataset:
name: Common Voice nl
type: common_voice
args: nl
metrics:
- name: Test WER
type: wer
value: 15.72
- name: Test CER
type: cer
value: 5.35
- name: Test WER (+LM)
type: wer
value: 12.84
- name: Test CER (+LM)
type: cer
value: 4.64
- task:
name: Automatic Speech Recognition
type: automatic-speech-recognition
dataset:
name: Robust Speech Event - Dev Data
type: speech-recognition-community-v2/dev_data
args: nl
metrics:
- name: Dev WER
type: wer
value: 35.79
- name: Dev CER
type: cer
value: 17.67
- name: Dev WER (+LM)
type: wer
value: 31.54
- name: Dev CER (+LM)
type: cer
value: 16.37
---
# Fine-tuned XLSR-53 large model for speech recognition in Dutch
Fine-tuned [facebook/wav2vec2-large-xlsr-53](https://huggingface.co/facebook/wav2vec2-large-xlsr-53) on Dutch using the train and validation splits of [Common Voice 6.1](https://huggingface.co/datasets/common_voice) and [CSS10](https://github.com/Kyubyong/css10).
When using this model, make sure that your speech input is sampled at 16kHz.
This model has been fine-tuned thanks to the GPU credits generously given by the [OVHcloud](https://www.ovhcloud.com/en/public-cloud/ai-training/) :)
The script used for training can be found here: https://github.com/jonatasgrosman/wav2vec2-sprint
## Usage
The model can be used directly (without a language model) as follows...
Using the [HuggingSound](https://github.com/jonatasgrosman/huggingsound) library:
```python
from huggingsound import SpeechRecognitionModel
model = SpeechRecognitionModel("jonatasgrosman/wav2vec2-large-xlsr-53-dutch")
audio_paths = ["/path/to/file.mp3", "/path/to/another_file.wav"]
transcriptions = model.transcribe(audio_paths)
```
Writing your own inference script:
```python
import torch
import librosa
from datasets import load_dataset
from transformers import Wav2Vec2ForCTC, Wav2Vec2Processor
LANG_ID = "nl"
MODEL_ID = "jonatasgrosman/wav2vec2-large-xlsr-53-dutch"
SAMPLES = 10
test_dataset = load_dataset("common_voice", LANG_ID, split=f"test[:{SAMPLES}]")
processor = Wav2Vec2Processor.from_pretrained(MODEL_ID)
model = Wav2Vec2ForCTC.from_pretrained(MODEL_ID)
# Preprocessing the datasets.
# We need to read the audio files as arrays
def speech_file_to_array_fn(batch):
speech_array, sampling_rate = librosa.load(batch["path"], sr=16_000)
batch["speech"] = speech_array
batch["sentence"] = batch["sentence"].upper()
return batch
test_dataset = test_dataset.map(speech_file_to_array_fn)
inputs = processor(test_dataset["speech"], sampling_rate=16_000, return_tensors="pt", padding=True)
with torch.no_grad():
logits = model(inputs.input_values, attention_mask=inputs.attention_mask).logits
predicted_ids = torch.argmax(logits, dim=-1)
predicted_sentences = processor.batch_decode(predicted_ids)
for i, predicted_sentence in enumerate(predicted_sentences):
print("-" * 100)
print("Reference:", test_dataset[i]["sentence"])
print("Prediction:", predicted_sentence)
```
| Reference | Prediction |
| ------------- | ------------- |
| DE ABORIGINALS ZIJN DE OORSPRONKELIJKE BEWONERS VAN AUSTRALIË. | DE ABBORIGENALS ZIJN DE OORSPRONKELIJKE BEWONERS VAN AUSTRALIË |
| MIJN TOETSENBORD ZIT VOL STOF. | MIJN TOETSENBORD ZIT VOL STOF |
| ZE HAD DE BANK BESCHADIGD MET HAAR SKATEBOARD. | ZE HAD DE BANK BESCHADIGD MET HAAR SCHEETBOORD |
| WAAR LAAT JIJ JE ONDERHOUD DOEN? | WAAR LAAT JIJ HET ONDERHOUD DOEN |
| NA HET LEZEN VAN VELE BEOORDELINGEN HAD ZE EINDELIJK HAAR OOG LATEN VALLEN OP EEN LAPTOP MET EEN QWERTY TOETSENBORD. | NA HET LEZEN VAN VELE BEOORDELINGEN HAD ZE EINDELIJK HAAR OOG LATEN VALLEN OP EEN LAPTOP MET EEN QUERTITOETSEMBORD |
| DE TAMPONS ZIJN OP. | DE TAPONT ZIJN OP |
| MARIJKE KENT OLIVIER NU AL MEER DAN TWEE JAAR. | MAARRIJKEN KENT OLIEVIER NU AL MEER DAN TWEE JAAR |
| HET VOEREN VAN BROOD AAN EENDEN IS EIGENLIJK ONGEZOND VOOR DE BEESTEN. | HET VOEREN VAN BEUROT AAN EINDEN IS EIGENLIJK ONGEZOND VOOR DE BEESTEN |
| PARKET MOET JE STOFZUIGEN, TEGELS MOET JE DWEILEN. | PARKET MOET JE STOF ZUIGEN MAAR TEGELS MOET JE DWEILEN |
| IN ONZE BUURT KENT IEDEREEN ELKAAR. | IN ONZE BUURT KENT IEDEREEN ELKAAR |
## Evaluation
1. To evaluate on `mozilla-foundation/common_voice_6_0` with split `test`
```bash
python eval.py --model_id jonatasgrosman/wav2vec2-large-xlsr-53-dutch --dataset mozilla-foundation/common_voice_6_0 --config nl --split test
```
2. To evaluate on `speech-recognition-community-v2/dev_data`
```bash
python eval.py --model_id jonatasgrosman/wav2vec2-large-xlsr-53-dutch --dataset speech-recognition-community-v2/dev_data --config nl --split validation --chunk_length_s 5.0 --stride_length_s 1.0
```
## Citation
If you want to cite this model you can use this:
```bibtex
@misc{grosman2021xlsr53-large-dutch,
title={Fine-tuned {XLSR}-53 large model for speech recognition in {D}utch},
author={Grosman, Jonatas},
howpublished={\url{https://huggingface.co/jonatasgrosman/wav2vec2-large-xlsr-53-dutch}},
year={2021}
}
``` |
microsoft/beit-base-patch16-224-pt22k-ft22k | microsoft | "2023-02-27T15:08:16Z" | 1,731,523 | 73 | transformers | [
"transformers",
"pytorch",
"jax",
"beit",
"image-classification",
"vision",
"dataset:imagenet",
"dataset:imagenet-21k",
"arxiv:2106.08254",
"license:apache-2.0",
"autotrain_compatible",
"endpoints_compatible",
"region:us"
] | image-classification | "2022-03-02T23:29:05Z" | ---
license: apache-2.0
tags:
- image-classification
- vision
datasets:
- imagenet
- imagenet-21k
---
# BEiT (base-sized model, fine-tuned on ImageNet-22k)
BEiT model pre-trained in a self-supervised fashion on ImageNet-22k - also called ImageNet-21k (14 million images, 21,841 classes) at resolution 224x224, and fine-tuned on the same dataset at resolution 224x224. It was introduced in the paper [BEIT: BERT Pre-Training of Image Transformers](https://arxiv.org/abs/2106.08254) by Hangbo Bao, Li Dong and Furu Wei and first released in [this repository](https://github.com/microsoft/unilm/tree/master/beit).
Disclaimer: The team releasing BEiT did not write a model card for this model so this model card has been written by the Hugging Face team.
## Model description
The BEiT model is a Vision Transformer (ViT), which is a transformer encoder model (BERT-like). In contrast to the original ViT model, BEiT is pretrained on a large collection of images in a self-supervised fashion, namely ImageNet-21k, at a resolution of 224x224 pixels. The pre-training objective for the model is to predict visual tokens from the encoder of OpenAI's DALL-E's VQ-VAE, based on masked patches.
Next, the model was fine-tuned in a supervised fashion on ImageNet (also referred to as ILSVRC2012), a dataset comprising 1 million images and 1,000 classes, also at resolution 224x224.
Images are presented to the model as a sequence of fixed-size patches (resolution 16x16), which are linearly embedded. Contrary to the original ViT models, BEiT models do use relative position embeddings (similar to T5) instead of absolute position embeddings, and perform classification of images by mean-pooling the final hidden states of the patches, instead of placing a linear layer on top of the final hidden state of the [CLS] token.
By pre-training the model, it learns an inner representation of images that can then be used to extract features useful for downstream tasks: if you have a dataset of labeled images for instance, you can train a standard classifier by placing a linear layer on top of the pre-trained encoder. One typically places a linear layer on top of the [CLS] token, as the last hidden state of this token can be seen as a representation of an entire image. Alternatively, one can mean-pool the final hidden states of the patch embeddings, and place a linear layer on top of that.
## Intended uses & limitations
You can use the raw model for image classification. See the [model hub](https://huggingface.co/models?search=microsoft/beit) to look for
fine-tuned versions on a task that interests you.
### How to use
Here is how to use this model to classify an image of the COCO 2017 dataset into one of the 1,000 ImageNet classes:
```python
from transformers import BeitImageProcessor, BeitForImageClassification
from PIL import Image
import requests
url = 'http://images.cocodataset.org/val2017/000000039769.jpg'
image = Image.open(requests.get(url, stream=True).raw)
processor = BeitImageProcessor.from_pretrained('microsoft/beit-base-patch16-224-pt22k-ft22k')
model = BeitForImageClassification.from_pretrained('microsoft/beit-base-patch16-224-pt22k-ft22k')
inputs = processor(images=image, return_tensors="pt")
outputs = model(**inputs)
logits = outputs.logits
# model predicts one of the 21,841 ImageNet-22k classes
predicted_class_idx = logits.argmax(-1).item()
print("Predicted class:", model.config.id2label[predicted_class_idx])
```
Currently, both the feature extractor and model support PyTorch.
## Training data
The BEiT model was pretrained on [ImageNet-21k](http://www.image-net.org/), a dataset consisting of 14 million images and 21k classes, and fine-tuned on the same dataset.
## Training procedure
### Preprocessing
The exact details of preprocessing of images during training/validation can be found [here](https://github.com/microsoft/unilm/blob/master/beit/datasets.py).
Images are resized/rescaled to the same resolution (224x224) and normalized across the RGB channels with mean (0.5, 0.5, 0.5) and standard deviation (0.5, 0.5, 0.5).
### Pretraining
For all pre-training related hyperparameters, we refer to page 15 of the [original paper](https://arxiv.org/abs/2106.08254).
## Evaluation results
For evaluation results on several image classification benchmarks, we refer to tables 1 and 2 of the original paper. Note that for fine-tuning, the best results are obtained with a higher resolution. Of course, increasing the model size will result in better performance.
### BibTeX entry and citation info
```@article{DBLP:journals/corr/abs-2106-08254,
author = {Hangbo Bao and
Li Dong and
Furu Wei},
title = {BEiT: {BERT} Pre-Training of Image Transformers},
journal = {CoRR},
volume = {abs/2106.08254},
year = {2021},
url = {https://arxiv.org/abs/2106.08254},
archivePrefix = {arXiv},
eprint = {2106.08254},
timestamp = {Tue, 29 Jun 2021 16:55:04 +0200},
biburl = {https://dblp.org/rec/journals/corr/abs-2106-08254.bib},
bibsource = {dblp computer science bibliography, https://dblp.org}
}
```
```bibtex
@inproceedings{deng2009imagenet,
title={Imagenet: A large-scale hierarchical image database},
author={Deng, Jia and Dong, Wei and Socher, Richard and Li, Li-Jia and Li, Kai and Fei-Fei, Li},
booktitle={2009 IEEE conference on computer vision and pattern recognition},
pages={248--255},
year={2009},
organization={Ieee}
}
``` |
distributed/optimized-gpt2-1b | distributed | "2024-11-13T01:18:16Z" | 1,714,226 | 0 | transformers | [
"transformers",
"safetensors",
"gpt_optimized",
"text-generation",
"custom_code",
"arxiv:1910.09700",
"autotrain_compatible",
"region:us"
] | text-generation | "2024-10-22T15:44:28Z" | ---
library_name: transformers
tags: []
---
# Model Card for Model ID
<!-- Provide a quick summary of what the model is/does. -->
## Model Details
### Model Description
<!-- Provide a longer summary of what this model is. -->
This is the model card of a 🤗 transformers model that has been pushed on the Hub. This model card has been automatically generated.
- **Developed by:** [More Information Needed]
- **Funded by [optional]:** [More Information Needed]
- **Shared by [optional]:** [More Information Needed]
- **Model type:** [More Information Needed]
- **Language(s) (NLP):** [More Information Needed]
- **License:** [More Information Needed]
- **Finetuned from model [optional]:** [More Information Needed]
### Model Sources [optional]
<!-- Provide the basic links for the model. -->
- **Repository:** [More Information Needed]
- **Paper [optional]:** [More Information Needed]
- **Demo [optional]:** [More Information Needed]
## Uses
<!-- Address questions around how the model is intended to be used, including the foreseeable users of the model and those affected by the model. -->
### Direct Use
<!-- This section is for the model use without fine-tuning or plugging into a larger ecosystem/app. -->
[More Information Needed]
### Downstream Use [optional]
<!-- This section is for the model use when fine-tuned for a task, or when plugged into a larger ecosystem/app -->
[More Information Needed]
### Out-of-Scope Use
<!-- This section addresses misuse, malicious use, and uses that the model will not work well for. -->
[More Information Needed]
## Bias, Risks, and Limitations
<!-- This section is meant to convey both technical and sociotechnical limitations. -->
[More Information Needed]
### Recommendations
<!-- This section is meant to convey recommendations with respect to the bias, risk, and technical limitations. -->
Users (both direct and downstream) should be made aware of the risks, biases and limitations of the model. More information needed for further recommendations.
## How to Get Started with the Model
Use the code below to get started with the model.
[More Information Needed]
## Training Details
### Training Data
<!-- This should link to a Dataset Card, perhaps with a short stub of information on what the training data is all about as well as documentation related to data pre-processing or additional filtering. -->
[More Information Needed]
### Training Procedure
<!-- This relates heavily to the Technical Specifications. Content here should link to that section when it is relevant to the training procedure. -->
#### Preprocessing [optional]
[More Information Needed]
#### Training Hyperparameters
- **Training regime:** [More Information Needed] <!--fp32, fp16 mixed precision, bf16 mixed precision, bf16 non-mixed precision, fp16 non-mixed precision, fp8 mixed precision -->
#### Speeds, Sizes, Times [optional]
<!-- This section provides information about throughput, start/end time, checkpoint size if relevant, etc. -->
[More Information Needed]
## Evaluation
<!-- This section describes the evaluation protocols and provides the results. -->
### Testing Data, Factors & Metrics
#### Testing Data
<!-- This should link to a Dataset Card if possible. -->
[More Information Needed]
#### Factors
<!-- These are the things the evaluation is disaggregating by, e.g., subpopulations or domains. -->
[More Information Needed]
#### Metrics
<!-- These are the evaluation metrics being used, ideally with a description of why. -->
[More Information Needed]
### Results
[More Information Needed]
#### Summary
## Model Examination [optional]
<!-- Relevant interpretability work for the model goes here -->
[More Information Needed]
## Environmental Impact
<!-- Total emissions (in grams of CO2eq) and additional considerations, such as electricity usage, go here. Edit the suggested text below accordingly -->
Carbon emissions can be estimated using the [Machine Learning Impact calculator](https://mlco2.github.io/impact#compute) presented in [Lacoste et al. (2019)](https://arxiv.org/abs/1910.09700).
- **Hardware Type:** [More Information Needed]
- **Hours used:** [More Information Needed]
- **Cloud Provider:** [More Information Needed]
- **Compute Region:** [More Information Needed]
- **Carbon Emitted:** [More Information Needed]
## Technical Specifications [optional]
### Model Architecture and Objective
[More Information Needed]
### Compute Infrastructure
[More Information Needed]
#### Hardware
[More Information Needed]
#### Software
[More Information Needed]
## Citation [optional]
<!-- If there is a paper or blog post introducing the model, the APA and Bibtex information for that should go in this section. -->
**BibTeX:**
[More Information Needed]
**APA:**
[More Information Needed]
## Glossary [optional]
<!-- If relevant, include terms and calculations in this section that can help readers understand the model or model card. -->
[More Information Needed]
## More Information [optional]
[More Information Needed]
## Model Card Authors [optional]
[More Information Needed]
## Model Card Contact
[More Information Needed] |
microsoft/layoutlmv3-base | microsoft | "2024-04-10T14:20:22Z" | 1,702,127 | 328 | transformers | [
"transformers",
"pytorch",
"tf",
"onnx",
"safetensors",
"layoutlmv3",
"en",
"arxiv:2204.08387",
"license:cc-by-nc-sa-4.0",
"endpoints_compatible",
"region:us"
] | null | "2022-04-18T06:53:05Z" | ---
language: en
license: cc-by-nc-sa-4.0
---
# LayoutLMv3
[Microsoft Document AI](https://www.microsoft.com/en-us/research/project/document-ai/) | [GitHub](https://aka.ms/layoutlmv3)
## Model description
LayoutLMv3 is a pre-trained multimodal Transformer for Document AI with unified text and image masking. The simple unified architecture and training objectives make LayoutLMv3 a general-purpose pre-trained model. For example, LayoutLMv3 can be fine-tuned for both text-centric tasks, including form understanding, receipt understanding, and document visual question answering, and image-centric tasks such as document image classification and document layout analysis.
[LayoutLMv3: Pre-training for Document AI with Unified Text and Image Masking](https://arxiv.org/abs/2204.08387)
Yupan Huang, Tengchao Lv, Lei Cui, Yutong Lu, Furu Wei, ACM Multimedia 2022.
## Citation
If you find LayoutLM useful in your research, please cite the following paper:
```
@inproceedings{huang2022layoutlmv3,
author={Yupan Huang and Tengchao Lv and Lei Cui and Yutong Lu and Furu Wei},
title={LayoutLMv3: Pre-training for Document AI with Unified Text and Image Masking},
booktitle={Proceedings of the 30th ACM International Conference on Multimedia},
year={2022}
}
```
## License
The content of this project itself is licensed under the [Attribution-NonCommercial-ShareAlike 4.0 International (CC BY-NC-SA 4.0)](https://creativecommons.org/licenses/by-nc-sa/4.0/).
Portions of the source code are based on the [transformers](https://github.com/huggingface/transformers) project.
[Microsoft Open Source Code of Conduct](https://opensource.microsoft.com/codeofconduct)
|
intfloat/e5-large-v2 | intfloat | "2023-08-07T05:01:43Z" | 1,701,557 | 217 | sentence-transformers | [
"sentence-transformers",
"pytorch",
"safetensors",
"bert",
"mteb",
"Sentence Transformers",
"sentence-similarity",
"en",
"arxiv:2212.03533",
"arxiv:2104.08663",
"arxiv:2210.07316",
"license:mit",
"model-index",
"autotrain_compatible",
"text-embeddings-inference",
"endpoints_compatible",
"region:us"
] | sentence-similarity | "2023-05-19T07:23:33Z" | ---
tags:
- mteb
- Sentence Transformers
- sentence-similarity
- sentence-transformers
model-index:
- name: e5-large-v2
results:
- task:
type: Classification
dataset:
type: mteb/amazon_counterfactual
name: MTEB AmazonCounterfactualClassification (en)
config: en
split: test
revision: e8379541af4e31359cca9fbcf4b00f2671dba205
metrics:
- type: accuracy
value: 79.22388059701493
- type: ap
value: 43.20816505595132
- type: f1
value: 73.27811303522058
- task:
type: Classification
dataset:
type: mteb/amazon_polarity
name: MTEB AmazonPolarityClassification
config: default
split: test
revision: e2d317d38cd51312af73b3d32a06d1a08b442046
metrics:
- type: accuracy
value: 93.748325
- type: ap
value: 90.72534979701297
- type: f1
value: 93.73895874282185
- task:
type: Classification
dataset:
type: mteb/amazon_reviews_multi
name: MTEB AmazonReviewsClassification (en)
config: en
split: test
revision: 1399c76144fd37290681b995c656ef9b2e06e26d
metrics:
- type: accuracy
value: 48.612
- type: f1
value: 47.61157345898393
- task:
type: Retrieval
dataset:
type: arguana
name: MTEB ArguAna
config: default
split: test
revision: None
metrics:
- type: map_at_1
value: 23.541999999999998
- type: map_at_10
value: 38.208
- type: map_at_100
value: 39.417
- type: map_at_1000
value: 39.428999999999995
- type: map_at_3
value: 33.95
- type: map_at_5
value: 36.329
- type: mrr_at_1
value: 23.755000000000003
- type: mrr_at_10
value: 38.288
- type: mrr_at_100
value: 39.511
- type: mrr_at_1000
value: 39.523
- type: mrr_at_3
value: 34.009
- type: mrr_at_5
value: 36.434
- type: ndcg_at_1
value: 23.541999999999998
- type: ndcg_at_10
value: 46.417
- type: ndcg_at_100
value: 51.812000000000005
- type: ndcg_at_1000
value: 52.137
- type: ndcg_at_3
value: 37.528
- type: ndcg_at_5
value: 41.81
- type: precision_at_1
value: 23.541999999999998
- type: precision_at_10
value: 7.269
- type: precision_at_100
value: 0.9690000000000001
- type: precision_at_1000
value: 0.099
- type: precision_at_3
value: 15.979
- type: precision_at_5
value: 11.664
- type: recall_at_1
value: 23.541999999999998
- type: recall_at_10
value: 72.688
- type: recall_at_100
value: 96.871
- type: recall_at_1000
value: 99.431
- type: recall_at_3
value: 47.937000000000005
- type: recall_at_5
value: 58.321
- task:
type: Clustering
dataset:
type: mteb/arxiv-clustering-p2p
name: MTEB ArxivClusteringP2P
config: default
split: test
revision: a122ad7f3f0291bf49cc6f4d32aa80929df69d5d
metrics:
- type: v_measure
value: 45.546499570522094
- task:
type: Clustering
dataset:
type: mteb/arxiv-clustering-s2s
name: MTEB ArxivClusteringS2S
config: default
split: test
revision: f910caf1a6075f7329cdf8c1a6135696f37dbd53
metrics:
- type: v_measure
value: 41.01607489943561
- task:
type: Reranking
dataset:
type: mteb/askubuntudupquestions-reranking
name: MTEB AskUbuntuDupQuestions
config: default
split: test
revision: 2000358ca161889fa9c082cb41daa8dcfb161a54
metrics:
- type: map
value: 59.616107510107774
- type: mrr
value: 72.75106626214661
- task:
type: STS
dataset:
type: mteb/biosses-sts
name: MTEB BIOSSES
config: default
split: test
revision: d3fb88f8f02e40887cd149695127462bbcf29b4a
metrics:
- type: cos_sim_pearson
value: 84.33018094733868
- type: cos_sim_spearman
value: 83.60190492611737
- type: euclidean_pearson
value: 82.1492450218961
- type: euclidean_spearman
value: 82.70308926526991
- type: manhattan_pearson
value: 81.93959600076842
- type: manhattan_spearman
value: 82.73260801016369
- task:
type: Classification
dataset:
type: mteb/banking77
name: MTEB Banking77Classification
config: default
split: test
revision: 0fd18e25b25c072e09e0d92ab615fda904d66300
metrics:
- type: accuracy
value: 84.54545454545455
- type: f1
value: 84.49582530928923
- task:
type: Clustering
dataset:
type: mteb/biorxiv-clustering-p2p
name: MTEB BiorxivClusteringP2P
config: default
split: test
revision: 65b79d1d13f80053f67aca9498d9402c2d9f1f40
metrics:
- type: v_measure
value: 37.362725540120096
- task:
type: Clustering
dataset:
type: mteb/biorxiv-clustering-s2s
name: MTEB BiorxivClusteringS2S
config: default
split: test
revision: 258694dd0231531bc1fd9de6ceb52a0853c6d908
metrics:
- type: v_measure
value: 34.849509608178145
- task:
type: Retrieval
dataset:
type: BeIR/cqadupstack
name: MTEB CQADupstackAndroidRetrieval
config: default
split: test
revision: None
metrics:
- type: map_at_1
value: 31.502999999999997
- type: map_at_10
value: 43.323
- type: map_at_100
value: 44.708999999999996
- type: map_at_1000
value: 44.838
- type: map_at_3
value: 38.987
- type: map_at_5
value: 41.516999999999996
- type: mrr_at_1
value: 38.769999999999996
- type: mrr_at_10
value: 49.13
- type: mrr_at_100
value: 49.697
- type: mrr_at_1000
value: 49.741
- type: mrr_at_3
value: 45.804
- type: mrr_at_5
value: 47.842
- type: ndcg_at_1
value: 38.769999999999996
- type: ndcg_at_10
value: 50.266999999999996
- type: ndcg_at_100
value: 54.967
- type: ndcg_at_1000
value: 56.976000000000006
- type: ndcg_at_3
value: 43.823
- type: ndcg_at_5
value: 47.12
- type: precision_at_1
value: 38.769999999999996
- type: precision_at_10
value: 10.057
- type: precision_at_100
value: 1.554
- type: precision_at_1000
value: 0.202
- type: precision_at_3
value: 21.125
- type: precision_at_5
value: 15.851
- type: recall_at_1
value: 31.502999999999997
- type: recall_at_10
value: 63.715999999999994
- type: recall_at_100
value: 83.61800000000001
- type: recall_at_1000
value: 96.63199999999999
- type: recall_at_3
value: 45.403
- type: recall_at_5
value: 54.481
- task:
type: Retrieval
dataset:
type: BeIR/cqadupstack
name: MTEB CQADupstackEnglishRetrieval
config: default
split: test
revision: None
metrics:
- type: map_at_1
value: 27.833000000000002
- type: map_at_10
value: 37.330999999999996
- type: map_at_100
value: 38.580999999999996
- type: map_at_1000
value: 38.708
- type: map_at_3
value: 34.713
- type: map_at_5
value: 36.104
- type: mrr_at_1
value: 35.223
- type: mrr_at_10
value: 43.419000000000004
- type: mrr_at_100
value: 44.198
- type: mrr_at_1000
value: 44.249
- type: mrr_at_3
value: 41.614000000000004
- type: mrr_at_5
value: 42.553000000000004
- type: ndcg_at_1
value: 35.223
- type: ndcg_at_10
value: 42.687999999999995
- type: ndcg_at_100
value: 47.447
- type: ndcg_at_1000
value: 49.701
- type: ndcg_at_3
value: 39.162
- type: ndcg_at_5
value: 40.557
- type: precision_at_1
value: 35.223
- type: precision_at_10
value: 7.962
- type: precision_at_100
value: 1.304
- type: precision_at_1000
value: 0.18
- type: precision_at_3
value: 19.023
- type: precision_at_5
value: 13.184999999999999
- type: recall_at_1
value: 27.833000000000002
- type: recall_at_10
value: 51.881
- type: recall_at_100
value: 72.04
- type: recall_at_1000
value: 86.644
- type: recall_at_3
value: 40.778
- type: recall_at_5
value: 45.176
- task:
type: Retrieval
dataset:
type: BeIR/cqadupstack
name: MTEB CQADupstackGamingRetrieval
config: default
split: test
revision: None
metrics:
- type: map_at_1
value: 38.175
- type: map_at_10
value: 51.174
- type: map_at_100
value: 52.26499999999999
- type: map_at_1000
value: 52.315999999999995
- type: map_at_3
value: 47.897
- type: map_at_5
value: 49.703
- type: mrr_at_1
value: 43.448
- type: mrr_at_10
value: 54.505
- type: mrr_at_100
value: 55.216
- type: mrr_at_1000
value: 55.242000000000004
- type: mrr_at_3
value: 51.98500000000001
- type: mrr_at_5
value: 53.434000000000005
- type: ndcg_at_1
value: 43.448
- type: ndcg_at_10
value: 57.282
- type: ndcg_at_100
value: 61.537
- type: ndcg_at_1000
value: 62.546
- type: ndcg_at_3
value: 51.73799999999999
- type: ndcg_at_5
value: 54.324
- type: precision_at_1
value: 43.448
- type: precision_at_10
value: 9.292
- type: precision_at_100
value: 1.233
- type: precision_at_1000
value: 0.136
- type: precision_at_3
value: 23.218
- type: precision_at_5
value: 15.887
- type: recall_at_1
value: 38.175
- type: recall_at_10
value: 72.00999999999999
- type: recall_at_100
value: 90.155
- type: recall_at_1000
value: 97.257
- type: recall_at_3
value: 57.133
- type: recall_at_5
value: 63.424
- task:
type: Retrieval
dataset:
type: BeIR/cqadupstack
name: MTEB CQADupstackGisRetrieval
config: default
split: test
revision: None
metrics:
- type: map_at_1
value: 22.405
- type: map_at_10
value: 30.043
- type: map_at_100
value: 31.191000000000003
- type: map_at_1000
value: 31.275
- type: map_at_3
value: 27.034000000000002
- type: map_at_5
value: 28.688000000000002
- type: mrr_at_1
value: 24.068
- type: mrr_at_10
value: 31.993
- type: mrr_at_100
value: 32.992
- type: mrr_at_1000
value: 33.050000000000004
- type: mrr_at_3
value: 28.964000000000002
- type: mrr_at_5
value: 30.653000000000002
- type: ndcg_at_1
value: 24.068
- type: ndcg_at_10
value: 35.198
- type: ndcg_at_100
value: 40.709
- type: ndcg_at_1000
value: 42.855
- type: ndcg_at_3
value: 29.139
- type: ndcg_at_5
value: 32.045
- type: precision_at_1
value: 24.068
- type: precision_at_10
value: 5.65
- type: precision_at_100
value: 0.885
- type: precision_at_1000
value: 0.11199999999999999
- type: precision_at_3
value: 12.279
- type: precision_at_5
value: 8.994
- type: recall_at_1
value: 22.405
- type: recall_at_10
value: 49.391
- type: recall_at_100
value: 74.53699999999999
- type: recall_at_1000
value: 90.605
- type: recall_at_3
value: 33.126
- type: recall_at_5
value: 40.073
- task:
type: Retrieval
dataset:
type: BeIR/cqadupstack
name: MTEB CQADupstackMathematicaRetrieval
config: default
split: test
revision: None
metrics:
- type: map_at_1
value: 13.309999999999999
- type: map_at_10
value: 20.688000000000002
- type: map_at_100
value: 22.022
- type: map_at_1000
value: 22.152
- type: map_at_3
value: 17.954
- type: map_at_5
value: 19.439
- type: mrr_at_1
value: 16.294
- type: mrr_at_10
value: 24.479
- type: mrr_at_100
value: 25.515
- type: mrr_at_1000
value: 25.593
- type: mrr_at_3
value: 21.642
- type: mrr_at_5
value: 23.189999999999998
- type: ndcg_at_1
value: 16.294
- type: ndcg_at_10
value: 25.833000000000002
- type: ndcg_at_100
value: 32.074999999999996
- type: ndcg_at_1000
value: 35.083
- type: ndcg_at_3
value: 20.493
- type: ndcg_at_5
value: 22.949
- type: precision_at_1
value: 16.294
- type: precision_at_10
value: 5.112
- type: precision_at_100
value: 0.96
- type: precision_at_1000
value: 0.134
- type: precision_at_3
value: 9.908999999999999
- type: precision_at_5
value: 7.587000000000001
- type: recall_at_1
value: 13.309999999999999
- type: recall_at_10
value: 37.851
- type: recall_at_100
value: 64.835
- type: recall_at_1000
value: 86.334
- type: recall_at_3
value: 23.493
- type: recall_at_5
value: 29.528
- task:
type: Retrieval
dataset:
type: BeIR/cqadupstack
name: MTEB CQADupstackPhysicsRetrieval
config: default
split: test
revision: None
metrics:
- type: map_at_1
value: 25.857999999999997
- type: map_at_10
value: 35.503
- type: map_at_100
value: 36.957
- type: map_at_1000
value: 37.065
- type: map_at_3
value: 32.275999999999996
- type: map_at_5
value: 34.119
- type: mrr_at_1
value: 31.954
- type: mrr_at_10
value: 40.851
- type: mrr_at_100
value: 41.863
- type: mrr_at_1000
value: 41.900999999999996
- type: mrr_at_3
value: 38.129999999999995
- type: mrr_at_5
value: 39.737
- type: ndcg_at_1
value: 31.954
- type: ndcg_at_10
value: 41.343999999999994
- type: ndcg_at_100
value: 47.397
- type: ndcg_at_1000
value: 49.501
- type: ndcg_at_3
value: 36.047000000000004
- type: ndcg_at_5
value: 38.639
- type: precision_at_1
value: 31.954
- type: precision_at_10
value: 7.68
- type: precision_at_100
value: 1.247
- type: precision_at_1000
value: 0.16199999999999998
- type: precision_at_3
value: 17.132
- type: precision_at_5
value: 12.589
- type: recall_at_1
value: 25.857999999999997
- type: recall_at_10
value: 53.43599999999999
- type: recall_at_100
value: 78.82400000000001
- type: recall_at_1000
value: 92.78999999999999
- type: recall_at_3
value: 38.655
- type: recall_at_5
value: 45.216
- task:
type: Retrieval
dataset:
type: BeIR/cqadupstack
name: MTEB CQADupstackProgrammersRetrieval
config: default
split: test
revision: None
metrics:
- type: map_at_1
value: 24.709
- type: map_at_10
value: 34.318
- type: map_at_100
value: 35.657
- type: map_at_1000
value: 35.783
- type: map_at_3
value: 31.326999999999998
- type: map_at_5
value: 33.021
- type: mrr_at_1
value: 30.137000000000004
- type: mrr_at_10
value: 39.093
- type: mrr_at_100
value: 39.992
- type: mrr_at_1000
value: 40.056999999999995
- type: mrr_at_3
value: 36.606
- type: mrr_at_5
value: 37.861
- type: ndcg_at_1
value: 30.137000000000004
- type: ndcg_at_10
value: 39.974
- type: ndcg_at_100
value: 45.647999999999996
- type: ndcg_at_1000
value: 48.259
- type: ndcg_at_3
value: 35.028
- type: ndcg_at_5
value: 37.175999999999995
- type: precision_at_1
value: 30.137000000000004
- type: precision_at_10
value: 7.363
- type: precision_at_100
value: 1.184
- type: precision_at_1000
value: 0.161
- type: precision_at_3
value: 16.857
- type: precision_at_5
value: 11.963
- type: recall_at_1
value: 24.709
- type: recall_at_10
value: 52.087
- type: recall_at_100
value: 76.125
- type: recall_at_1000
value: 93.82300000000001
- type: recall_at_3
value: 38.149
- type: recall_at_5
value: 43.984
- task:
type: Retrieval
dataset:
type: BeIR/cqadupstack
name: MTEB CQADupstackRetrieval
config: default
split: test
revision: None
metrics:
- type: map_at_1
value: 23.40791666666667
- type: map_at_10
value: 32.458083333333335
- type: map_at_100
value: 33.691916666666664
- type: map_at_1000
value: 33.81191666666666
- type: map_at_3
value: 29.51625
- type: map_at_5
value: 31.168083333333335
- type: mrr_at_1
value: 27.96591666666666
- type: mrr_at_10
value: 36.528583333333344
- type: mrr_at_100
value: 37.404
- type: mrr_at_1000
value: 37.464333333333336
- type: mrr_at_3
value: 33.92883333333333
- type: mrr_at_5
value: 35.41933333333333
- type: ndcg_at_1
value: 27.96591666666666
- type: ndcg_at_10
value: 37.89141666666666
- type: ndcg_at_100
value: 43.23066666666666
- type: ndcg_at_1000
value: 45.63258333333333
- type: ndcg_at_3
value: 32.811249999999994
- type: ndcg_at_5
value: 35.22566666666667
- type: precision_at_1
value: 27.96591666666666
- type: precision_at_10
value: 6.834083333333332
- type: precision_at_100
value: 1.12225
- type: precision_at_1000
value: 0.15241666666666667
- type: precision_at_3
value: 15.264333333333335
- type: precision_at_5
value: 11.039416666666666
- type: recall_at_1
value: 23.40791666666667
- type: recall_at_10
value: 49.927083333333336
- type: recall_at_100
value: 73.44641666666668
- type: recall_at_1000
value: 90.19950000000001
- type: recall_at_3
value: 35.88341666666667
- type: recall_at_5
value: 42.061249999999994
- task:
type: Retrieval
dataset:
type: BeIR/cqadupstack
name: MTEB CQADupstackStatsRetrieval
config: default
split: test
revision: None
metrics:
- type: map_at_1
value: 19.592000000000002
- type: map_at_10
value: 26.895999999999997
- type: map_at_100
value: 27.921000000000003
- type: map_at_1000
value: 28.02
- type: map_at_3
value: 24.883
- type: map_at_5
value: 25.812
- type: mrr_at_1
value: 22.698999999999998
- type: mrr_at_10
value: 29.520999999999997
- type: mrr_at_100
value: 30.458000000000002
- type: mrr_at_1000
value: 30.526999999999997
- type: mrr_at_3
value: 27.633000000000003
- type: mrr_at_5
value: 28.483999999999998
- type: ndcg_at_1
value: 22.698999999999998
- type: ndcg_at_10
value: 31.061
- type: ndcg_at_100
value: 36.398
- type: ndcg_at_1000
value: 38.89
- type: ndcg_at_3
value: 27.149
- type: ndcg_at_5
value: 28.627000000000002
- type: precision_at_1
value: 22.698999999999998
- type: precision_at_10
value: 5.106999999999999
- type: precision_at_100
value: 0.857
- type: precision_at_1000
value: 0.11499999999999999
- type: precision_at_3
value: 11.963
- type: precision_at_5
value: 8.221
- type: recall_at_1
value: 19.592000000000002
- type: recall_at_10
value: 41.329
- type: recall_at_100
value: 66.094
- type: recall_at_1000
value: 84.511
- type: recall_at_3
value: 30.61
- type: recall_at_5
value: 34.213
- task:
type: Retrieval
dataset:
type: BeIR/cqadupstack
name: MTEB CQADupstackTexRetrieval
config: default
split: test
revision: None
metrics:
- type: map_at_1
value: 14.71
- type: map_at_10
value: 20.965
- type: map_at_100
value: 21.994
- type: map_at_1000
value: 22.133
- type: map_at_3
value: 18.741
- type: map_at_5
value: 19.951
- type: mrr_at_1
value: 18.307000000000002
- type: mrr_at_10
value: 24.66
- type: mrr_at_100
value: 25.540000000000003
- type: mrr_at_1000
value: 25.629
- type: mrr_at_3
value: 22.511
- type: mrr_at_5
value: 23.72
- type: ndcg_at_1
value: 18.307000000000002
- type: ndcg_at_10
value: 25.153
- type: ndcg_at_100
value: 30.229
- type: ndcg_at_1000
value: 33.623
- type: ndcg_at_3
value: 21.203
- type: ndcg_at_5
value: 23.006999999999998
- type: precision_at_1
value: 18.307000000000002
- type: precision_at_10
value: 4.725
- type: precision_at_100
value: 0.8659999999999999
- type: precision_at_1000
value: 0.133
- type: precision_at_3
value: 10.14
- type: precision_at_5
value: 7.481
- type: recall_at_1
value: 14.71
- type: recall_at_10
value: 34.087
- type: recall_at_100
value: 57.147999999999996
- type: recall_at_1000
value: 81.777
- type: recall_at_3
value: 22.996
- type: recall_at_5
value: 27.73
- task:
type: Retrieval
dataset:
type: BeIR/cqadupstack
name: MTEB CQADupstackUnixRetrieval
config: default
split: test
revision: None
metrics:
- type: map_at_1
value: 23.472
- type: map_at_10
value: 32.699
- type: map_at_100
value: 33.867000000000004
- type: map_at_1000
value: 33.967000000000006
- type: map_at_3
value: 29.718
- type: map_at_5
value: 31.345
- type: mrr_at_1
value: 28.265
- type: mrr_at_10
value: 36.945
- type: mrr_at_100
value: 37.794
- type: mrr_at_1000
value: 37.857
- type: mrr_at_3
value: 34.266000000000005
- type: mrr_at_5
value: 35.768
- type: ndcg_at_1
value: 28.265
- type: ndcg_at_10
value: 38.35
- type: ndcg_at_100
value: 43.739
- type: ndcg_at_1000
value: 46.087
- type: ndcg_at_3
value: 33.004
- type: ndcg_at_5
value: 35.411
- type: precision_at_1
value: 28.265
- type: precision_at_10
value: 6.715999999999999
- type: precision_at_100
value: 1.059
- type: precision_at_1000
value: 0.13799999999999998
- type: precision_at_3
value: 15.299
- type: precision_at_5
value: 10.951
- type: recall_at_1
value: 23.472
- type: recall_at_10
value: 51.413
- type: recall_at_100
value: 75.17
- type: recall_at_1000
value: 91.577
- type: recall_at_3
value: 36.651
- type: recall_at_5
value: 42.814
- task:
type: Retrieval
dataset:
type: BeIR/cqadupstack
name: MTEB CQADupstackWebmastersRetrieval
config: default
split: test
revision: None
metrics:
- type: map_at_1
value: 23.666
- type: map_at_10
value: 32.963
- type: map_at_100
value: 34.544999999999995
- type: map_at_1000
value: 34.792
- type: map_at_3
value: 29.74
- type: map_at_5
value: 31.5
- type: mrr_at_1
value: 29.051
- type: mrr_at_10
value: 38.013000000000005
- type: mrr_at_100
value: 38.997
- type: mrr_at_1000
value: 39.055
- type: mrr_at_3
value: 34.947
- type: mrr_at_5
value: 36.815
- type: ndcg_at_1
value: 29.051
- type: ndcg_at_10
value: 39.361000000000004
- type: ndcg_at_100
value: 45.186
- type: ndcg_at_1000
value: 47.867
- type: ndcg_at_3
value: 33.797
- type: ndcg_at_5
value: 36.456
- type: precision_at_1
value: 29.051
- type: precision_at_10
value: 7.668
- type: precision_at_100
value: 1.532
- type: precision_at_1000
value: 0.247
- type: precision_at_3
value: 15.876000000000001
- type: precision_at_5
value: 11.779
- type: recall_at_1
value: 23.666
- type: recall_at_10
value: 51.858000000000004
- type: recall_at_100
value: 77.805
- type: recall_at_1000
value: 94.504
- type: recall_at_3
value: 36.207
- type: recall_at_5
value: 43.094
- task:
type: Retrieval
dataset:
type: BeIR/cqadupstack
name: MTEB CQADupstackWordpressRetrieval
config: default
split: test
revision: None
metrics:
- type: map_at_1
value: 15.662
- type: map_at_10
value: 23.594
- type: map_at_100
value: 24.593999999999998
- type: map_at_1000
value: 24.694
- type: map_at_3
value: 20.925
- type: map_at_5
value: 22.817999999999998
- type: mrr_at_1
value: 17.375
- type: mrr_at_10
value: 25.734
- type: mrr_at_100
value: 26.586
- type: mrr_at_1000
value: 26.671
- type: mrr_at_3
value: 23.044
- type: mrr_at_5
value: 24.975
- type: ndcg_at_1
value: 17.375
- type: ndcg_at_10
value: 28.186
- type: ndcg_at_100
value: 33.436
- type: ndcg_at_1000
value: 36.203
- type: ndcg_at_3
value: 23.152
- type: ndcg_at_5
value: 26.397
- type: precision_at_1
value: 17.375
- type: precision_at_10
value: 4.677
- type: precision_at_100
value: 0.786
- type: precision_at_1000
value: 0.109
- type: precision_at_3
value: 10.351
- type: precision_at_5
value: 7.985
- type: recall_at_1
value: 15.662
- type: recall_at_10
value: 40.066
- type: recall_at_100
value: 65.006
- type: recall_at_1000
value: 85.94000000000001
- type: recall_at_3
value: 27.400000000000002
- type: recall_at_5
value: 35.002
- task:
type: Retrieval
dataset:
type: climate-fever
name: MTEB ClimateFEVER
config: default
split: test
revision: None
metrics:
- type: map_at_1
value: 8.853
- type: map_at_10
value: 15.568000000000001
- type: map_at_100
value: 17.383000000000003
- type: map_at_1000
value: 17.584
- type: map_at_3
value: 12.561
- type: map_at_5
value: 14.056
- type: mrr_at_1
value: 18.958
- type: mrr_at_10
value: 28.288000000000004
- type: mrr_at_100
value: 29.432000000000002
- type: mrr_at_1000
value: 29.498
- type: mrr_at_3
value: 25.049
- type: mrr_at_5
value: 26.857
- type: ndcg_at_1
value: 18.958
- type: ndcg_at_10
value: 22.21
- type: ndcg_at_100
value: 29.596
- type: ndcg_at_1000
value: 33.583
- type: ndcg_at_3
value: 16.994999999999997
- type: ndcg_at_5
value: 18.95
- type: precision_at_1
value: 18.958
- type: precision_at_10
value: 7.192
- type: precision_at_100
value: 1.5
- type: precision_at_1000
value: 0.22399999999999998
- type: precision_at_3
value: 12.573
- type: precision_at_5
value: 10.202
- type: recall_at_1
value: 8.853
- type: recall_at_10
value: 28.087
- type: recall_at_100
value: 53.701
- type: recall_at_1000
value: 76.29899999999999
- type: recall_at_3
value: 15.913
- type: recall_at_5
value: 20.658
- task:
type: Retrieval
dataset:
type: dbpedia-entity
name: MTEB DBPedia
config: default
split: test
revision: None
metrics:
- type: map_at_1
value: 9.077
- type: map_at_10
value: 20.788999999999998
- type: map_at_100
value: 30.429000000000002
- type: map_at_1000
value: 32.143
- type: map_at_3
value: 14.692
- type: map_at_5
value: 17.139
- type: mrr_at_1
value: 70.75
- type: mrr_at_10
value: 78.036
- type: mrr_at_100
value: 78.401
- type: mrr_at_1000
value: 78.404
- type: mrr_at_3
value: 76.75
- type: mrr_at_5
value: 77.47500000000001
- type: ndcg_at_1
value: 58.12500000000001
- type: ndcg_at_10
value: 44.015
- type: ndcg_at_100
value: 49.247
- type: ndcg_at_1000
value: 56.211999999999996
- type: ndcg_at_3
value: 49.151
- type: ndcg_at_5
value: 46.195
- type: precision_at_1
value: 70.75
- type: precision_at_10
value: 35.5
- type: precision_at_100
value: 11.355
- type: precision_at_1000
value: 2.1950000000000003
- type: precision_at_3
value: 53.083000000000006
- type: precision_at_5
value: 44.800000000000004
- type: recall_at_1
value: 9.077
- type: recall_at_10
value: 26.259
- type: recall_at_100
value: 56.547000000000004
- type: recall_at_1000
value: 78.551
- type: recall_at_3
value: 16.162000000000003
- type: recall_at_5
value: 19.753999999999998
- task:
type: Classification
dataset:
type: mteb/emotion
name: MTEB EmotionClassification
config: default
split: test
revision: 4f58c6b202a23cf9a4da393831edf4f9183cad37
metrics:
- type: accuracy
value: 49.44500000000001
- type: f1
value: 44.67067691783401
- task:
type: Retrieval
dataset:
type: fever
name: MTEB FEVER
config: default
split: test
revision: None
metrics:
- type: map_at_1
value: 68.182
- type: map_at_10
value: 78.223
- type: map_at_100
value: 78.498
- type: map_at_1000
value: 78.512
- type: map_at_3
value: 76.71
- type: map_at_5
value: 77.725
- type: mrr_at_1
value: 73.177
- type: mrr_at_10
value: 82.513
- type: mrr_at_100
value: 82.633
- type: mrr_at_1000
value: 82.635
- type: mrr_at_3
value: 81.376
- type: mrr_at_5
value: 82.182
- type: ndcg_at_1
value: 73.177
- type: ndcg_at_10
value: 82.829
- type: ndcg_at_100
value: 83.84
- type: ndcg_at_1000
value: 84.07900000000001
- type: ndcg_at_3
value: 80.303
- type: ndcg_at_5
value: 81.846
- type: precision_at_1
value: 73.177
- type: precision_at_10
value: 10.241999999999999
- type: precision_at_100
value: 1.099
- type: precision_at_1000
value: 0.11399999999999999
- type: precision_at_3
value: 31.247999999999998
- type: precision_at_5
value: 19.697
- type: recall_at_1
value: 68.182
- type: recall_at_10
value: 92.657
- type: recall_at_100
value: 96.709
- type: recall_at_1000
value: 98.184
- type: recall_at_3
value: 85.9
- type: recall_at_5
value: 89.755
- task:
type: Retrieval
dataset:
type: fiqa
name: MTEB FiQA2018
config: default
split: test
revision: None
metrics:
- type: map_at_1
value: 21.108
- type: map_at_10
value: 33.342
- type: map_at_100
value: 35.281
- type: map_at_1000
value: 35.478
- type: map_at_3
value: 29.067
- type: map_at_5
value: 31.563000000000002
- type: mrr_at_1
value: 41.667
- type: mrr_at_10
value: 49.913000000000004
- type: mrr_at_100
value: 50.724000000000004
- type: mrr_at_1000
value: 50.766
- type: mrr_at_3
value: 47.504999999999995
- type: mrr_at_5
value: 49.033
- type: ndcg_at_1
value: 41.667
- type: ndcg_at_10
value: 41.144
- type: ndcg_at_100
value: 48.326
- type: ndcg_at_1000
value: 51.486
- type: ndcg_at_3
value: 37.486999999999995
- type: ndcg_at_5
value: 38.78
- type: precision_at_1
value: 41.667
- type: precision_at_10
value: 11.358
- type: precision_at_100
value: 1.873
- type: precision_at_1000
value: 0.244
- type: precision_at_3
value: 25
- type: precision_at_5
value: 18.519
- type: recall_at_1
value: 21.108
- type: recall_at_10
value: 47.249
- type: recall_at_100
value: 74.52
- type: recall_at_1000
value: 93.31
- type: recall_at_3
value: 33.271
- type: recall_at_5
value: 39.723000000000006
- task:
type: Retrieval
dataset:
type: hotpotqa
name: MTEB HotpotQA
config: default
split: test
revision: None
metrics:
- type: map_at_1
value: 40.317
- type: map_at_10
value: 64.861
- type: map_at_100
value: 65.697
- type: map_at_1000
value: 65.755
- type: map_at_3
value: 61.258
- type: map_at_5
value: 63.590999999999994
- type: mrr_at_1
value: 80.635
- type: mrr_at_10
value: 86.528
- type: mrr_at_100
value: 86.66199999999999
- type: mrr_at_1000
value: 86.666
- type: mrr_at_3
value: 85.744
- type: mrr_at_5
value: 86.24300000000001
- type: ndcg_at_1
value: 80.635
- type: ndcg_at_10
value: 73.13199999999999
- type: ndcg_at_100
value: 75.927
- type: ndcg_at_1000
value: 76.976
- type: ndcg_at_3
value: 68.241
- type: ndcg_at_5
value: 71.071
- type: precision_at_1
value: 80.635
- type: precision_at_10
value: 15.326
- type: precision_at_100
value: 1.7500000000000002
- type: precision_at_1000
value: 0.189
- type: precision_at_3
value: 43.961
- type: precision_at_5
value: 28.599999999999998
- type: recall_at_1
value: 40.317
- type: recall_at_10
value: 76.631
- type: recall_at_100
value: 87.495
- type: recall_at_1000
value: 94.362
- type: recall_at_3
value: 65.94200000000001
- type: recall_at_5
value: 71.499
- task:
type: Classification
dataset:
type: mteb/imdb
name: MTEB ImdbClassification
config: default
split: test
revision: 3d86128a09e091d6018b6d26cad27f2739fc2db7
metrics:
- type: accuracy
value: 91.686
- type: ap
value: 87.5577120393173
- type: f1
value: 91.6629447355139
- task:
type: Retrieval
dataset:
type: msmarco
name: MTEB MSMARCO
config: default
split: dev
revision: None
metrics:
- type: map_at_1
value: 23.702
- type: map_at_10
value: 36.414
- type: map_at_100
value: 37.561
- type: map_at_1000
value: 37.605
- type: map_at_3
value: 32.456
- type: map_at_5
value: 34.827000000000005
- type: mrr_at_1
value: 24.355
- type: mrr_at_10
value: 37.01
- type: mrr_at_100
value: 38.085
- type: mrr_at_1000
value: 38.123000000000005
- type: mrr_at_3
value: 33.117999999999995
- type: mrr_at_5
value: 35.452
- type: ndcg_at_1
value: 24.384
- type: ndcg_at_10
value: 43.456
- type: ndcg_at_100
value: 48.892
- type: ndcg_at_1000
value: 49.964
- type: ndcg_at_3
value: 35.475
- type: ndcg_at_5
value: 39.711
- type: precision_at_1
value: 24.384
- type: precision_at_10
value: 6.7940000000000005
- type: precision_at_100
value: 0.951
- type: precision_at_1000
value: 0.104
- type: precision_at_3
value: 15.052999999999999
- type: precision_at_5
value: 11.189
- type: recall_at_1
value: 23.702
- type: recall_at_10
value: 65.057
- type: recall_at_100
value: 90.021
- type: recall_at_1000
value: 98.142
- type: recall_at_3
value: 43.551
- type: recall_at_5
value: 53.738
- task:
type: Classification
dataset:
type: mteb/mtop_domain
name: MTEB MTOPDomainClassification (en)
config: en
split: test
revision: d80d48c1eb48d3562165c59d59d0034df9fff0bf
metrics:
- type: accuracy
value: 94.62380300957591
- type: f1
value: 94.49871222100734
- task:
type: Classification
dataset:
type: mteb/mtop_intent
name: MTEB MTOPIntentClassification (en)
config: en
split: test
revision: ae001d0e6b1228650b7bd1c2c65fb50ad11a8aba
metrics:
- type: accuracy
value: 77.14090287277702
- type: f1
value: 60.32101258220515
- task:
type: Classification
dataset:
type: mteb/amazon_massive_intent
name: MTEB MassiveIntentClassification (en)
config: en
split: test
revision: 31efe3c427b0bae9c22cbb560b8f15491cc6bed7
metrics:
- type: accuracy
value: 73.84330867518494
- type: f1
value: 71.92248688515255
- task:
type: Classification
dataset:
type: mteb/amazon_massive_scenario
name: MTEB MassiveScenarioClassification (en)
config: en
split: test
revision: 7d571f92784cd94a019292a1f45445077d0ef634
metrics:
- type: accuracy
value: 78.10692669804976
- type: f1
value: 77.9904839122866
- task:
type: Clustering
dataset:
type: mteb/medrxiv-clustering-p2p
name: MTEB MedrxivClusteringP2P
config: default
split: test
revision: e7a26af6f3ae46b30dde8737f02c07b1505bcc73
metrics:
- type: v_measure
value: 31.822988923078444
- task:
type: Clustering
dataset:
type: mteb/medrxiv-clustering-s2s
name: MTEB MedrxivClusteringS2S
config: default
split: test
revision: 35191c8c0dca72d8ff3efcd72aa802307d469663
metrics:
- type: v_measure
value: 30.38394880253403
- task:
type: Reranking
dataset:
type: mteb/mind_small
name: MTEB MindSmallReranking
config: default
split: test
revision: 3bdac13927fdc888b903db93b2ffdbd90b295a69
metrics:
- type: map
value: 31.82504612539082
- type: mrr
value: 32.84462298174977
- task:
type: Retrieval
dataset:
type: nfcorpus
name: MTEB NFCorpus
config: default
split: test
revision: None
metrics:
- type: map_at_1
value: 6.029
- type: map_at_10
value: 14.088999999999999
- type: map_at_100
value: 17.601
- type: map_at_1000
value: 19.144
- type: map_at_3
value: 10.156
- type: map_at_5
value: 11.892
- type: mrr_at_1
value: 46.44
- type: mrr_at_10
value: 56.596999999999994
- type: mrr_at_100
value: 57.11000000000001
- type: mrr_at_1000
value: 57.14
- type: mrr_at_3
value: 54.334
- type: mrr_at_5
value: 55.774
- type: ndcg_at_1
value: 44.891999999999996
- type: ndcg_at_10
value: 37.134
- type: ndcg_at_100
value: 33.652
- type: ndcg_at_1000
value: 42.548
- type: ndcg_at_3
value: 41.851
- type: ndcg_at_5
value: 39.842
- type: precision_at_1
value: 46.44
- type: precision_at_10
value: 27.647
- type: precision_at_100
value: 8.309999999999999
- type: precision_at_1000
value: 2.146
- type: precision_at_3
value: 39.422000000000004
- type: precision_at_5
value: 34.675
- type: recall_at_1
value: 6.029
- type: recall_at_10
value: 18.907
- type: recall_at_100
value: 33.76
- type: recall_at_1000
value: 65.14999999999999
- type: recall_at_3
value: 11.584999999999999
- type: recall_at_5
value: 14.626
- task:
type: Retrieval
dataset:
type: nq
name: MTEB NQ
config: default
split: test
revision: None
metrics:
- type: map_at_1
value: 39.373000000000005
- type: map_at_10
value: 55.836
- type: map_at_100
value: 56.611999999999995
- type: map_at_1000
value: 56.63
- type: map_at_3
value: 51.747
- type: map_at_5
value: 54.337999999999994
- type: mrr_at_1
value: 44.147999999999996
- type: mrr_at_10
value: 58.42699999999999
- type: mrr_at_100
value: 58.902
- type: mrr_at_1000
value: 58.914
- type: mrr_at_3
value: 55.156000000000006
- type: mrr_at_5
value: 57.291000000000004
- type: ndcg_at_1
value: 44.119
- type: ndcg_at_10
value: 63.444
- type: ndcg_at_100
value: 66.40599999999999
- type: ndcg_at_1000
value: 66.822
- type: ndcg_at_3
value: 55.962
- type: ndcg_at_5
value: 60.228
- type: precision_at_1
value: 44.119
- type: precision_at_10
value: 10.006
- type: precision_at_100
value: 1.17
- type: precision_at_1000
value: 0.121
- type: precision_at_3
value: 25.135
- type: precision_at_5
value: 17.59
- type: recall_at_1
value: 39.373000000000005
- type: recall_at_10
value: 83.78999999999999
- type: recall_at_100
value: 96.246
- type: recall_at_1000
value: 99.324
- type: recall_at_3
value: 64.71900000000001
- type: recall_at_5
value: 74.508
- task:
type: Retrieval
dataset:
type: quora
name: MTEB QuoraRetrieval
config: default
split: test
revision: None
metrics:
- type: map_at_1
value: 69.199
- type: map_at_10
value: 82.892
- type: map_at_100
value: 83.578
- type: map_at_1000
value: 83.598
- type: map_at_3
value: 79.948
- type: map_at_5
value: 81.779
- type: mrr_at_1
value: 79.67
- type: mrr_at_10
value: 86.115
- type: mrr_at_100
value: 86.249
- type: mrr_at_1000
value: 86.251
- type: mrr_at_3
value: 85.08200000000001
- type: mrr_at_5
value: 85.783
- type: ndcg_at_1
value: 79.67
- type: ndcg_at_10
value: 86.839
- type: ndcg_at_100
value: 88.252
- type: ndcg_at_1000
value: 88.401
- type: ndcg_at_3
value: 83.86200000000001
- type: ndcg_at_5
value: 85.473
- type: precision_at_1
value: 79.67
- type: precision_at_10
value: 13.19
- type: precision_at_100
value: 1.521
- type: precision_at_1000
value: 0.157
- type: precision_at_3
value: 36.677
- type: precision_at_5
value: 24.118000000000002
- type: recall_at_1
value: 69.199
- type: recall_at_10
value: 94.321
- type: recall_at_100
value: 99.20400000000001
- type: recall_at_1000
value: 99.947
- type: recall_at_3
value: 85.787
- type: recall_at_5
value: 90.365
- task:
type: Clustering
dataset:
type: mteb/reddit-clustering
name: MTEB RedditClustering
config: default
split: test
revision: 24640382cdbf8abc73003fb0fa6d111a705499eb
metrics:
- type: v_measure
value: 55.82810046856353
- task:
type: Clustering
dataset:
type: mteb/reddit-clustering-p2p
name: MTEB RedditClusteringP2P
config: default
split: test
revision: 282350215ef01743dc01b456c7f5241fa8937f16
metrics:
- type: v_measure
value: 63.38132611783628
- task:
type: Retrieval
dataset:
type: scidocs
name: MTEB SCIDOCS
config: default
split: test
revision: None
metrics:
- type: map_at_1
value: 5.127000000000001
- type: map_at_10
value: 12.235
- type: map_at_100
value: 14.417
- type: map_at_1000
value: 14.75
- type: map_at_3
value: 8.906
- type: map_at_5
value: 10.591000000000001
- type: mrr_at_1
value: 25.2
- type: mrr_at_10
value: 35.879
- type: mrr_at_100
value: 36.935
- type: mrr_at_1000
value: 36.997
- type: mrr_at_3
value: 32.783
- type: mrr_at_5
value: 34.367999999999995
- type: ndcg_at_1
value: 25.2
- type: ndcg_at_10
value: 20.509
- type: ndcg_at_100
value: 28.67
- type: ndcg_at_1000
value: 34.42
- type: ndcg_at_3
value: 19.948
- type: ndcg_at_5
value: 17.166
- type: precision_at_1
value: 25.2
- type: precision_at_10
value: 10.440000000000001
- type: precision_at_100
value: 2.214
- type: precision_at_1000
value: 0.359
- type: precision_at_3
value: 18.533
- type: precision_at_5
value: 14.860000000000001
- type: recall_at_1
value: 5.127000000000001
- type: recall_at_10
value: 21.147
- type: recall_at_100
value: 44.946999999999996
- type: recall_at_1000
value: 72.89
- type: recall_at_3
value: 11.277
- type: recall_at_5
value: 15.042
- task:
type: STS
dataset:
type: mteb/sickr-sts
name: MTEB SICK-R
config: default
split: test
revision: a6ea5a8cab320b040a23452cc28066d9beae2cee
metrics:
- type: cos_sim_pearson
value: 83.0373011786213
- type: cos_sim_spearman
value: 79.27889560856613
- type: euclidean_pearson
value: 80.31186315495655
- type: euclidean_spearman
value: 79.41630415280811
- type: manhattan_pearson
value: 80.31755140442013
- type: manhattan_spearman
value: 79.43069870027611
- task:
type: STS
dataset:
type: mteb/sts12-sts
name: MTEB STS12
config: default
split: test
revision: a0d554a64d88156834ff5ae9920b964011b16384
metrics:
- type: cos_sim_pearson
value: 84.8659751342045
- type: cos_sim_spearman
value: 76.95377612997667
- type: euclidean_pearson
value: 81.24552945497848
- type: euclidean_spearman
value: 77.18236963555253
- type: manhattan_pearson
value: 81.26477607759037
- type: manhattan_spearman
value: 77.13821753062756
- task:
type: STS
dataset:
type: mteb/sts13-sts
name: MTEB STS13
config: default
split: test
revision: 7e90230a92c190f1bf69ae9002b8cea547a64cca
metrics:
- type: cos_sim_pearson
value: 83.34597139044875
- type: cos_sim_spearman
value: 84.124169425592
- type: euclidean_pearson
value: 83.68590721511401
- type: euclidean_spearman
value: 84.18846190846398
- type: manhattan_pearson
value: 83.57630235061498
- type: manhattan_spearman
value: 84.10244043726902
- task:
type: STS
dataset:
type: mteb/sts14-sts
name: MTEB STS14
config: default
split: test
revision: 6031580fec1f6af667f0bd2da0a551cf4f0b2375
metrics:
- type: cos_sim_pearson
value: 82.67641885599572
- type: cos_sim_spearman
value: 80.46450725650428
- type: euclidean_pearson
value: 81.61645042715865
- type: euclidean_spearman
value: 80.61418394236874
- type: manhattan_pearson
value: 81.55712034928871
- type: manhattan_spearman
value: 80.57905670523951
- task:
type: STS
dataset:
type: mteb/sts15-sts
name: MTEB STS15
config: default
split: test
revision: ae752c7c21bf194d8b67fd573edf7ae58183cbe3
metrics:
- type: cos_sim_pearson
value: 88.86650310886782
- type: cos_sim_spearman
value: 89.76081629222328
- type: euclidean_pearson
value: 89.1530747029954
- type: euclidean_spearman
value: 89.80990657280248
- type: manhattan_pearson
value: 89.10640563278132
- type: manhattan_spearman
value: 89.76282108434047
- task:
type: STS
dataset:
type: mteb/sts16-sts
name: MTEB STS16
config: default
split: test
revision: 4d8694f8f0e0100860b497b999b3dbed754a0513
metrics:
- type: cos_sim_pearson
value: 83.93864027911118
- type: cos_sim_spearman
value: 85.47096193999023
- type: euclidean_pearson
value: 85.03141840870533
- type: euclidean_spearman
value: 85.43124029598181
- type: manhattan_pearson
value: 84.99002664393512
- type: manhattan_spearman
value: 85.39169195120834
- task:
type: STS
dataset:
type: mteb/sts17-crosslingual-sts
name: MTEB STS17 (en-en)
config: en-en
split: test
revision: af5e6fb845001ecf41f4c1e033ce921939a2a68d
metrics:
- type: cos_sim_pearson
value: 88.7045343749832
- type: cos_sim_spearman
value: 89.03262221146677
- type: euclidean_pearson
value: 89.56078218264365
- type: euclidean_spearman
value: 89.17827006466868
- type: manhattan_pearson
value: 89.52717595468582
- type: manhattan_spearman
value: 89.15878115952923
- task:
type: STS
dataset:
type: mteb/sts22-crosslingual-sts
name: MTEB STS22 (en)
config: en
split: test
revision: 6d1ba47164174a496b7fa5d3569dae26a6813b80
metrics:
- type: cos_sim_pearson
value: 64.20191302875551
- type: cos_sim_spearman
value: 64.11446552557646
- type: euclidean_pearson
value: 64.6918197393619
- type: euclidean_spearman
value: 63.440182631197764
- type: manhattan_pearson
value: 64.55692904121835
- type: manhattan_spearman
value: 63.424877742756266
- task:
type: STS
dataset:
type: mteb/stsbenchmark-sts
name: MTEB STSBenchmark
config: default
split: test
revision: b0fddb56ed78048fa8b90373c8a3cfc37b684831
metrics:
- type: cos_sim_pearson
value: 86.37793104662344
- type: cos_sim_spearman
value: 87.7357802629067
- type: euclidean_pearson
value: 87.4286301545109
- type: euclidean_spearman
value: 87.78452920777421
- type: manhattan_pearson
value: 87.42445169331255
- type: manhattan_spearman
value: 87.78537677249598
- task:
type: Reranking
dataset:
type: mteb/scidocs-reranking
name: MTEB SciDocsRR
config: default
split: test
revision: d3c5e1fc0b855ab6097bf1cda04dd73947d7caab
metrics:
- type: map
value: 84.31465405081792
- type: mrr
value: 95.7173781193389
- task:
type: Retrieval
dataset:
type: scifact
name: MTEB SciFact
config: default
split: test
revision: None
metrics:
- type: map_at_1
value: 57.760999999999996
- type: map_at_10
value: 67.904
- type: map_at_100
value: 68.539
- type: map_at_1000
value: 68.562
- type: map_at_3
value: 65.415
- type: map_at_5
value: 66.788
- type: mrr_at_1
value: 60.333000000000006
- type: mrr_at_10
value: 68.797
- type: mrr_at_100
value: 69.236
- type: mrr_at_1000
value: 69.257
- type: mrr_at_3
value: 66.667
- type: mrr_at_5
value: 67.967
- type: ndcg_at_1
value: 60.333000000000006
- type: ndcg_at_10
value: 72.24199999999999
- type: ndcg_at_100
value: 74.86
- type: ndcg_at_1000
value: 75.354
- type: ndcg_at_3
value: 67.93400000000001
- type: ndcg_at_5
value: 70.02199999999999
- type: precision_at_1
value: 60.333000000000006
- type: precision_at_10
value: 9.533
- type: precision_at_100
value: 1.09
- type: precision_at_1000
value: 0.11299999999999999
- type: precision_at_3
value: 26.778000000000002
- type: precision_at_5
value: 17.467
- type: recall_at_1
value: 57.760999999999996
- type: recall_at_10
value: 84.383
- type: recall_at_100
value: 96.267
- type: recall_at_1000
value: 100
- type: recall_at_3
value: 72.628
- type: recall_at_5
value: 78.094
- task:
type: PairClassification
dataset:
type: mteb/sprintduplicatequestions-pairclassification
name: MTEB SprintDuplicateQuestions
config: default
split: test
revision: d66bd1f72af766a5cc4b0ca5e00c162f89e8cc46
metrics:
- type: cos_sim_accuracy
value: 99.8029702970297
- type: cos_sim_ap
value: 94.9210324173411
- type: cos_sim_f1
value: 89.8521162672106
- type: cos_sim_precision
value: 91.67533818938605
- type: cos_sim_recall
value: 88.1
- type: dot_accuracy
value: 99.69504950495049
- type: dot_ap
value: 90.4919719146181
- type: dot_f1
value: 84.72289156626506
- type: dot_precision
value: 81.76744186046511
- type: dot_recall
value: 87.9
- type: euclidean_accuracy
value: 99.79702970297029
- type: euclidean_ap
value: 94.87827463795753
- type: euclidean_f1
value: 89.55680081507896
- type: euclidean_precision
value: 91.27725856697819
- type: euclidean_recall
value: 87.9
- type: manhattan_accuracy
value: 99.7990099009901
- type: manhattan_ap
value: 94.87587025149682
- type: manhattan_f1
value: 89.76298537569339
- type: manhattan_precision
value: 90.53916581892166
- type: manhattan_recall
value: 89
- type: max_accuracy
value: 99.8029702970297
- type: max_ap
value: 94.9210324173411
- type: max_f1
value: 89.8521162672106
- task:
type: Clustering
dataset:
type: mteb/stackexchange-clustering
name: MTEB StackExchangeClustering
config: default
split: test
revision: 6cbc1f7b2bc0622f2e39d2c77fa502909748c259
metrics:
- type: v_measure
value: 65.92385753948724
- task:
type: Clustering
dataset:
type: mteb/stackexchange-clustering-p2p
name: MTEB StackExchangeClusteringP2P
config: default
split: test
revision: 815ca46b2622cec33ccafc3735d572c266efdb44
metrics:
- type: v_measure
value: 33.671756975431144
- task:
type: Reranking
dataset:
type: mteb/stackoverflowdupquestions-reranking
name: MTEB StackOverflowDupQuestions
config: default
split: test
revision: e185fbe320c72810689fc5848eb6114e1ef5ec69
metrics:
- type: map
value: 50.677928036739004
- type: mrr
value: 51.56413133435193
- task:
type: Summarization
dataset:
type: mteb/summeval
name: MTEB SummEval
config: default
split: test
revision: cda12ad7615edc362dbf25a00fdd61d3b1eaf93c
metrics:
- type: cos_sim_pearson
value: 30.523589340819683
- type: cos_sim_spearman
value: 30.187407518823235
- type: dot_pearson
value: 29.039713969699015
- type: dot_spearman
value: 29.114740651155508
- task:
type: Retrieval
dataset:
type: trec-covid
name: MTEB TRECCOVID
config: default
split: test
revision: None
metrics:
- type: map_at_1
value: 0.211
- type: map_at_10
value: 1.6199999999999999
- type: map_at_100
value: 8.658000000000001
- type: map_at_1000
value: 21.538
- type: map_at_3
value: 0.575
- type: map_at_5
value: 0.919
- type: mrr_at_1
value: 78
- type: mrr_at_10
value: 86.18599999999999
- type: mrr_at_100
value: 86.18599999999999
- type: mrr_at_1000
value: 86.18599999999999
- type: mrr_at_3
value: 85
- type: mrr_at_5
value: 85.9
- type: ndcg_at_1
value: 74
- type: ndcg_at_10
value: 66.542
- type: ndcg_at_100
value: 50.163999999999994
- type: ndcg_at_1000
value: 45.696999999999996
- type: ndcg_at_3
value: 71.531
- type: ndcg_at_5
value: 70.45
- type: precision_at_1
value: 78
- type: precision_at_10
value: 69.39999999999999
- type: precision_at_100
value: 51.06
- type: precision_at_1000
value: 20.022000000000002
- type: precision_at_3
value: 76
- type: precision_at_5
value: 74.8
- type: recall_at_1
value: 0.211
- type: recall_at_10
value: 1.813
- type: recall_at_100
value: 12.098
- type: recall_at_1000
value: 42.618
- type: recall_at_3
value: 0.603
- type: recall_at_5
value: 0.987
- task:
type: Retrieval
dataset:
type: webis-touche2020
name: MTEB Touche2020
config: default
split: test
revision: None
metrics:
- type: map_at_1
value: 2.2079999999999997
- type: map_at_10
value: 7.777000000000001
- type: map_at_100
value: 12.825000000000001
- type: map_at_1000
value: 14.196
- type: map_at_3
value: 4.285
- type: map_at_5
value: 6.177
- type: mrr_at_1
value: 30.612000000000002
- type: mrr_at_10
value: 42.635
- type: mrr_at_100
value: 43.955
- type: mrr_at_1000
value: 43.955
- type: mrr_at_3
value: 38.435
- type: mrr_at_5
value: 41.088
- type: ndcg_at_1
value: 28.571
- type: ndcg_at_10
value: 20.666999999999998
- type: ndcg_at_100
value: 31.840000000000003
- type: ndcg_at_1000
value: 43.191
- type: ndcg_at_3
value: 23.45
- type: ndcg_at_5
value: 22.994
- type: precision_at_1
value: 30.612000000000002
- type: precision_at_10
value: 17.959
- type: precision_at_100
value: 6.755
- type: precision_at_1000
value: 1.4200000000000002
- type: precision_at_3
value: 23.810000000000002
- type: precision_at_5
value: 23.673
- type: recall_at_1
value: 2.2079999999999997
- type: recall_at_10
value: 13.144
- type: recall_at_100
value: 42.491
- type: recall_at_1000
value: 77.04299999999999
- type: recall_at_3
value: 5.3469999999999995
- type: recall_at_5
value: 9.139
- task:
type: Classification
dataset:
type: mteb/toxic_conversations_50k
name: MTEB ToxicConversationsClassification
config: default
split: test
revision: d7c0de2777da35d6aae2200a62c6e0e5af397c4c
metrics:
- type: accuracy
value: 70.9044
- type: ap
value: 14.625783489340755
- type: f1
value: 54.814936562590546
- task:
type: Classification
dataset:
type: mteb/tweet_sentiment_extraction
name: MTEB TweetSentimentExtractionClassification
config: default
split: test
revision: d604517c81ca91fe16a244d1248fc021f9ecee7a
metrics:
- type: accuracy
value: 60.94227504244483
- type: f1
value: 61.22516038508854
- task:
type: Clustering
dataset:
type: mteb/twentynewsgroups-clustering
name: MTEB TwentyNewsgroupsClustering
config: default
split: test
revision: 6125ec4e24fa026cec8a478383ee943acfbd5449
metrics:
- type: v_measure
value: 49.602409155145864
- task:
type: PairClassification
dataset:
type: mteb/twittersemeval2015-pairclassification
name: MTEB TwitterSemEval2015
config: default
split: test
revision: 70970daeab8776df92f5ea462b6173c0b46fd2d1
metrics:
- type: cos_sim_accuracy
value: 86.94641473445789
- type: cos_sim_ap
value: 76.91572747061197
- type: cos_sim_f1
value: 70.14348097317529
- type: cos_sim_precision
value: 66.53254437869822
- type: cos_sim_recall
value: 74.1688654353562
- type: dot_accuracy
value: 84.80061989628658
- type: dot_ap
value: 70.7952548895177
- type: dot_f1
value: 65.44780728844965
- type: dot_precision
value: 61.53310104529617
- type: dot_recall
value: 69.89445910290237
- type: euclidean_accuracy
value: 86.94641473445789
- type: euclidean_ap
value: 76.80774009393652
- type: euclidean_f1
value: 70.30522503879979
- type: euclidean_precision
value: 68.94977168949772
- type: euclidean_recall
value: 71.71503957783642
- type: manhattan_accuracy
value: 86.8629671574179
- type: manhattan_ap
value: 76.76518632600317
- type: manhattan_f1
value: 70.16056518946692
- type: manhattan_precision
value: 68.360450563204
- type: manhattan_recall
value: 72.0580474934037
- type: max_accuracy
value: 86.94641473445789
- type: max_ap
value: 76.91572747061197
- type: max_f1
value: 70.30522503879979
- task:
type: PairClassification
dataset:
type: mteb/twitterurlcorpus-pairclassification
name: MTEB TwitterURLCorpus
config: default
split: test
revision: 8b6510b0b1fa4e4c4f879467980e9be563ec1cdf
metrics:
- type: cos_sim_accuracy
value: 89.10428066907285
- type: cos_sim_ap
value: 86.25114759921435
- type: cos_sim_f1
value: 78.37857884586856
- type: cos_sim_precision
value: 75.60818546078993
- type: cos_sim_recall
value: 81.35971666153372
- type: dot_accuracy
value: 87.41995575736406
- type: dot_ap
value: 81.51838010086782
- type: dot_f1
value: 74.77398015435503
- type: dot_precision
value: 71.53002390662354
- type: dot_recall
value: 78.32614721281182
- type: euclidean_accuracy
value: 89.12368533395428
- type: euclidean_ap
value: 86.33456799874504
- type: euclidean_f1
value: 78.45496750232127
- type: euclidean_precision
value: 75.78388462366364
- type: euclidean_recall
value: 81.32121958731136
- type: manhattan_accuracy
value: 89.10622113556099
- type: manhattan_ap
value: 86.31215061745333
- type: manhattan_f1
value: 78.40684906011539
- type: manhattan_precision
value: 75.89536643366722
- type: manhattan_recall
value: 81.09023714197721
- type: max_accuracy
value: 89.12368533395428
- type: max_ap
value: 86.33456799874504
- type: max_f1
value: 78.45496750232127
language:
- en
license: mit
---
# E5-large-v2
[Text Embeddings by Weakly-Supervised Contrastive Pre-training](https://arxiv.org/pdf/2212.03533.pdf).
Liang Wang, Nan Yang, Xiaolong Huang, Binxing Jiao, Linjun Yang, Daxin Jiang, Rangan Majumder, Furu Wei, arXiv 2022
This model has 24 layers and the embedding size is 1024.
## Usage
Below is an example to encode queries and passages from the MS-MARCO passage ranking dataset.
```python
import torch.nn.functional as F
from torch import Tensor
from transformers import AutoTokenizer, AutoModel
def average_pool(last_hidden_states: Tensor,
attention_mask: Tensor) -> Tensor:
last_hidden = last_hidden_states.masked_fill(~attention_mask[..., None].bool(), 0.0)
return last_hidden.sum(dim=1) / attention_mask.sum(dim=1)[..., None]
# Each input text should start with "query: " or "passage: ".
# For tasks other than retrieval, you can simply use the "query: " prefix.
input_texts = ['query: how much protein should a female eat',
'query: summit define',
"passage: As a general guideline, the CDC's average requirement of protein for women ages 19 to 70 is 46 grams per day. But, as you can see from this chart, you'll need to increase that if you're expecting or training for a marathon. Check out the chart below to see how much protein you should be eating each day.",
"passage: Definition of summit for English Language Learners. : 1 the highest point of a mountain : the top of a mountain. : 2 the highest level. : 3 a meeting or series of meetings between the leaders of two or more governments."]
tokenizer = AutoTokenizer.from_pretrained('intfloat/e5-large-v2')
model = AutoModel.from_pretrained('intfloat/e5-large-v2')
# Tokenize the input texts
batch_dict = tokenizer(input_texts, max_length=512, padding=True, truncation=True, return_tensors='pt')
outputs = model(**batch_dict)
embeddings = average_pool(outputs.last_hidden_state, batch_dict['attention_mask'])
# normalize embeddings
embeddings = F.normalize(embeddings, p=2, dim=1)
scores = (embeddings[:2] @ embeddings[2:].T) * 100
print(scores.tolist())
```
## Training Details
Please refer to our paper at [https://arxiv.org/pdf/2212.03533.pdf](https://arxiv.org/pdf/2212.03533.pdf).
## Benchmark Evaluation
Check out [unilm/e5](https://github.com/microsoft/unilm/tree/master/e5) to reproduce evaluation results
on the [BEIR](https://arxiv.org/abs/2104.08663) and [MTEB benchmark](https://arxiv.org/abs/2210.07316).
## Support for Sentence Transformers
Below is an example for usage with sentence_transformers.
```python
from sentence_transformers import SentenceTransformer
model = SentenceTransformer('intfloat/e5-large-v2')
input_texts = [
'query: how much protein should a female eat',
'query: summit define',
"passage: As a general guideline, the CDC's average requirement of protein for women ages 19 to 70 is 46 grams per day. But, as you can see from this chart, you'll need to increase that if you're expecting or training for a marathon. Check out the chart below to see how much protein you should be eating each day.",
"passage: Definition of summit for English Language Learners. : 1 the highest point of a mountain : the top of a mountain. : 2 the highest level. : 3 a meeting or series of meetings between the leaders of two or more governments."
]
embeddings = model.encode(input_texts, normalize_embeddings=True)
```
Package requirements
`pip install sentence_transformers~=2.2.2`
Contributors: [michaelfeil](https://huggingface.co/michaelfeil)
## FAQ
**1. Do I need to add the prefix "query: " and "passage: " to input texts?**
Yes, this is how the model is trained, otherwise you will see a performance degradation.
Here are some rules of thumb:
- Use "query: " and "passage: " correspondingly for asymmetric tasks such as passage retrieval in open QA, ad-hoc information retrieval.
- Use "query: " prefix for symmetric tasks such as semantic similarity, paraphrase retrieval.
- Use "query: " prefix if you want to use embeddings as features, such as linear probing classification, clustering.
**2. Why are my reproduced results slightly different from reported in the model card?**
Different versions of `transformers` and `pytorch` could cause negligible but non-zero performance differences.
**3. Why does the cosine similarity scores distribute around 0.7 to 1.0?**
This is a known and expected behavior as we use a low temperature 0.01 for InfoNCE contrastive loss.
For text embedding tasks like text retrieval or semantic similarity,
what matters is the relative order of the scores instead of the absolute values,
so this should not be an issue.
## Citation
If you find our paper or models helpful, please consider cite as follows:
```
@article{wang2022text,
title={Text Embeddings by Weakly-Supervised Contrastive Pre-training},
author={Wang, Liang and Yang, Nan and Huang, Xiaolong and Jiao, Binxing and Yang, Linjun and Jiang, Daxin and Majumder, Rangan and Wei, Furu},
journal={arXiv preprint arXiv:2212.03533},
year={2022}
}
```
## Limitations
This model only works for English texts. Long texts will be truncated to at most 512 tokens.
|
cambridgeltl/SapBERT-from-PubMedBERT-fulltext | cambridgeltl | "2023-06-14T19:03:02Z" | 1,682,068 | 41 | transformers | [
"transformers",
"pytorch",
"tf",
"jax",
"safetensors",
"bert",
"feature-extraction",
"biomedical",
"lexical semantics",
"bionlp",
"biology",
"science",
"embedding",
"entity linking",
"en",
"arxiv:2010.11784",
"license:apache-2.0",
"endpoints_compatible",
"region:us"
] | feature-extraction | "2022-03-02T23:29:05Z" | ---
license: apache-2.0
language:
- en
tags:
- biomedical
- lexical semantics
- bionlp
- biology
- science
- embedding
- entity linking
---
---
datasets:
- UMLS
**[news]** A cross-lingual extension of SapBERT will appear in the main onference of **ACL 2021**! <br>
**[news]** SapBERT will appear in the conference proceedings of **NAACL 2021**!
### SapBERT-PubMedBERT
SapBERT by [Liu et al. (2020)](https://arxiv.org/pdf/2010.11784.pdf). Trained with [UMLS](https://www.nlm.nih.gov/research/umls/licensedcontent/umlsknowledgesources.html) 2020AA (English only), using [microsoft/BiomedNLP-PubMedBERT-base-uncased-abstract-fulltext](https://huggingface.co/microsoft/BiomedNLP-PubMedBERT-base-uncased-abstract-fulltext) as the base model.
### Expected input and output
The input should be a string of biomedical entity names, e.g., "covid infection" or "Hydroxychloroquine". The [CLS] embedding of the last layer is regarded as the output.
#### Extracting embeddings from SapBERT
The following script converts a list of strings (entity names) into embeddings.
```python
import numpy as np
import torch
from tqdm.auto import tqdm
from transformers import AutoTokenizer, AutoModel
tokenizer = AutoTokenizer.from_pretrained("cambridgeltl/SapBERT-from-PubMedBERT-fulltext")
model = AutoModel.from_pretrained("cambridgeltl/SapBERT-from-PubMedBERT-fulltext").cuda()
# replace with your own list of entity names
all_names = ["covid-19", "Coronavirus infection", "high fever", "Tumor of posterior wall of oropharynx"]
bs = 128 # batch size during inference
all_embs = []
for i in tqdm(np.arange(0, len(all_names), bs)):
toks = tokenizer.batch_encode_plus(all_names[i:i+bs],
padding="max_length",
max_length=25,
truncation=True,
return_tensors="pt")
toks_cuda = {}
for k,v in toks.items():
toks_cuda[k] = v.cuda()
cls_rep = model(**toks_cuda)[0][:,0,:] # use CLS representation as the embedding
all_embs.append(cls_rep.cpu().detach().numpy())
all_embs = np.concatenate(all_embs, axis=0)
```
For more details about training and eval, see SapBERT [github repo](https://github.com/cambridgeltl/sapbert).
### Citation
```bibtex
@inproceedings{liu-etal-2021-self,
title = "Self-Alignment Pretraining for Biomedical Entity Representations",
author = "Liu, Fangyu and
Shareghi, Ehsan and
Meng, Zaiqiao and
Basaldella, Marco and
Collier, Nigel",
booktitle = "Proceedings of the 2021 Conference of the North American Chapter of the Association for Computational Linguistics: Human Language Technologies",
month = jun,
year = "2021",
address = "Online",
publisher = "Association for Computational Linguistics",
url = "https://www.aclweb.org/anthology/2021.naacl-main.334",
pages = "4228--4238",
abstract = "Despite the widespread success of self-supervised learning via masked language models (MLM), accurately capturing fine-grained semantic relationships in the biomedical domain remains a challenge. This is of paramount importance for entity-level tasks such as entity linking where the ability to model entity relations (especially synonymy) is pivotal. To address this challenge, we propose SapBERT, a pretraining scheme that self-aligns the representation space of biomedical entities. We design a scalable metric learning framework that can leverage UMLS, a massive collection of biomedical ontologies with 4M+ concepts. In contrast with previous pipeline-based hybrid systems, SapBERT offers an elegant one-model-for-all solution to the problem of medical entity linking (MEL), achieving a new state-of-the-art (SOTA) on six MEL benchmarking datasets. In the scientific domain, we achieve SOTA even without task-specific supervision. With substantial improvement over various domain-specific pretrained MLMs such as BioBERT, SciBERTand and PubMedBERT, our pretraining scheme proves to be both effective and robust.",
}
``` |
mrm8488/distilroberta-finetuned-financial-news-sentiment-analysis | mrm8488 | "2024-01-21T15:17:58Z" | 1,604,260 | 337 | transformers | [
"transformers",
"pytorch",
"tensorboard",
"safetensors",
"roberta",
"text-classification",
"generated_from_trainer",
"financial",
"stocks",
"sentiment",
"dataset:financial_phrasebank",
"license:apache-2.0",
"model-index",
"autotrain_compatible",
"endpoints_compatible",
"region:us"
] | text-classification | "2022-03-02T23:29:05Z" | ---
license: apache-2.0
thumbnail: https://huggingface.co/mrm8488/distilroberta-finetuned-financial-news-sentiment-analysis/resolve/main/logo_no_bg.png
tags:
- generated_from_trainer
- financial
- stocks
- sentiment
widget:
- text: "Operating profit totaled EUR 9.4 mn , down from EUR 11.7 mn in 2004 ."
datasets:
- financial_phrasebank
metrics:
- accuracy
model-index:
- name: distilRoberta-financial-sentiment
results:
- task:
name: Text Classification
type: text-classification
dataset:
name: financial_phrasebank
type: financial_phrasebank
args: sentences_allagree
metrics:
- name: Accuracy
type: accuracy
value: 0.9823008849557522
---
<!-- This model card has been generated automatically according to the information the Trainer had access to. You
should probably proofread and complete it, then remove this comment. -->
<div style="text-align:center;width:250px;height:250px;">
<img src="https://huggingface.co/mrm8488/distilroberta-finetuned-financial-news-sentiment-analysis/resolve/main/logo_no_bg.png" alt="logo">
</div>
# DistilRoberta-financial-sentiment
This model is a fine-tuned version of [distilroberta-base](https://huggingface.co/distilroberta-base) on the financial_phrasebank dataset.
It achieves the following results on the evaluation set:
- Loss: 0.1116
- Accuracy: **0.98**23
## Base Model description
This model is a distilled version of the [RoBERTa-base model](https://huggingface.co/roberta-base). It follows the same training procedure as [DistilBERT](https://huggingface.co/distilbert-base-uncased).
The code for the distillation process can be found [here](https://github.com/huggingface/transformers/tree/master/examples/distillation).
This model is case-sensitive: it makes a difference between English and English.
The model has 6 layers, 768 dimension and 12 heads, totalizing 82M parameters (compared to 125M parameters for RoBERTa-base).
On average DistilRoBERTa is twice as fast as Roberta-base.
## Training Data
Polar sentiment dataset of sentences from financial news. The dataset consists of 4840 sentences from English language financial news categorised by sentiment. The dataset is divided by agreement rate of 5-8 annotators.
## Training procedure
### Training hyperparameters
The following hyperparameters were used during training:
- learning_rate: 2e-05
- train_batch_size: 8
- eval_batch_size: 8
- seed: 42
- optimizer: Adam with betas=(0.9,0.999) and epsilon=1e-08
- lr_scheduler_type: linear
- num_epochs: 5
### Training results
| Training Loss | Epoch | Step | Validation Loss | Accuracy |
|:-------------:|:-----:|:----:|:---------------:|:--------:|
| No log | 1.0 | 255 | 0.1670 | 0.9646 |
| 0.209 | 2.0 | 510 | 0.2290 | 0.9558 |
| 0.209 | 3.0 | 765 | 0.2044 | 0.9558 |
| 0.0326 | 4.0 | 1020 | 0.1116 | 0.9823 |
| 0.0326 | 5.0 | 1275 | 0.1127 | 0.9779 |
### Framework versions
- Transformers 4.10.2
- Pytorch 1.9.0+cu102
- Datasets 1.12.1
- Tokenizers 0.10.3
|
sentence-transformers/bert-base-nli-mean-tokens | sentence-transformers | "2024-11-05T15:50:46Z" | 1,597,152 | 32 | sentence-transformers | [
"sentence-transformers",
"pytorch",
"tf",
"jax",
"rust",
"onnx",
"safetensors",
"openvino",
"bert",
"feature-extraction",
"sentence-similarity",
"transformers",
"arxiv:1908.10084",
"license:apache-2.0",
"autotrain_compatible",
"text-embeddings-inference",
"endpoints_compatible",
"region:us"
] | sentence-similarity | "2022-03-02T23:29:05Z" | ---
license: apache-2.0
library_name: sentence-transformers
tags:
- sentence-transformers
- feature-extraction
- sentence-similarity
- transformers
pipeline_tag: sentence-similarity
---
**⚠️ This model is deprecated. Please don't use it as it produces sentence embeddings of low quality. You can find recommended sentence embedding models here: [SBERT.net - Pretrained Models](https://www.sbert.net/docs/pretrained_models.html)**
# sentence-transformers/bert-base-nli-mean-tokens
This is a [sentence-transformers](https://www.SBERT.net) model: It maps sentences & paragraphs to a 768 dimensional dense vector space and can be used for tasks like clustering or semantic search.
## Usage (Sentence-Transformers)
Using this model becomes easy when you have [sentence-transformers](https://www.SBERT.net) installed:
```
pip install -U sentence-transformers
```
Then you can use the model like this:
```python
from sentence_transformers import SentenceTransformer
sentences = ["This is an example sentence", "Each sentence is converted"]
model = SentenceTransformer('sentence-transformers/bert-base-nli-mean-tokens')
embeddings = model.encode(sentences)
print(embeddings)
```
## Usage (HuggingFace Transformers)
Without [sentence-transformers](https://www.SBERT.net), you can use the model like this: First, you pass your input through the transformer model, then you have to apply the right pooling-operation on-top of the contextualized word embeddings.
```python
from transformers import AutoTokenizer, AutoModel
import torch
#Mean Pooling - Take attention mask into account for correct averaging
def mean_pooling(model_output, attention_mask):
token_embeddings = model_output[0] #First element of model_output contains all token embeddings
input_mask_expanded = attention_mask.unsqueeze(-1).expand(token_embeddings.size()).float()
return torch.sum(token_embeddings * input_mask_expanded, 1) / torch.clamp(input_mask_expanded.sum(1), min=1e-9)
# Sentences we want sentence embeddings for
sentences = ['This is an example sentence', 'Each sentence is converted']
# Load model from HuggingFace Hub
tokenizer = AutoTokenizer.from_pretrained('sentence-transformers/bert-base-nli-mean-tokens')
model = AutoModel.from_pretrained('sentence-transformers/bert-base-nli-mean-tokens')
# Tokenize sentences
encoded_input = tokenizer(sentences, padding=True, truncation=True, return_tensors='pt')
# Compute token embeddings
with torch.no_grad():
model_output = model(**encoded_input)
# Perform pooling. In this case, max pooling.
sentence_embeddings = mean_pooling(model_output, encoded_input['attention_mask'])
print("Sentence embeddings:")
print(sentence_embeddings)
```
## Evaluation Results
For an automated evaluation of this model, see the *Sentence Embeddings Benchmark*: [https://seb.sbert.net](https://seb.sbert.net?model_name=sentence-transformers/bert-base-nli-mean-tokens)
## Full Model Architecture
```
SentenceTransformer(
(0): Transformer({'max_seq_length': 128, 'do_lower_case': False}) with Transformer model: BertModel
(1): Pooling({'word_embedding_dimension': 768, 'pooling_mode_cls_token': False, 'pooling_mode_mean_tokens': True, 'pooling_mode_max_tokens': False, 'pooling_mode_mean_sqrt_len_tokens': False})
)
```
## Citing & Authors
This model was trained by [sentence-transformers](https://www.sbert.net/).
If you find this model helpful, feel free to cite our publication [Sentence-BERT: Sentence Embeddings using Siamese BERT-Networks](https://arxiv.org/abs/1908.10084):
```bibtex
@inproceedings{reimers-2019-sentence-bert,
title = "Sentence-BERT: Sentence Embeddings using Siamese BERT-Networks",
author = "Reimers, Nils and Gurevych, Iryna",
booktitle = "Proceedings of the 2019 Conference on Empirical Methods in Natural Language Processing",
month = "11",
year = "2019",
publisher = "Association for Computational Linguistics",
url = "http://arxiv.org/abs/1908.10084",
}
``` |
stabilityai/stable-diffusion-2-1-base | stabilityai | "2023-07-05T16:19:20Z" | 1,589,989 | 628 | diffusers | [
"diffusers",
"safetensors",
"stable-diffusion",
"text-to-image",
"arxiv:2112.10752",
"arxiv:2202.00512",
"arxiv:1910.09700",
"license:openrail++",
"autotrain_compatible",
"endpoints_compatible",
"diffusers:StableDiffusionPipeline",
"region:us"
] | text-to-image | "2022-12-06T17:25:36Z" | ---
license: openrail++
tags:
- stable-diffusion
- text-to-image
---
# Stable Diffusion v2-1-base Model Card
This model card focuses on the model associated with the Stable Diffusion v2-1-base model.
This `stable-diffusion-2-1-base` model fine-tunes [stable-diffusion-2-base](https://huggingface.co/stabilityai/stable-diffusion-2-base) (`512-base-ema.ckpt`) with 220k extra steps taken, with `punsafe=0.98` on the same dataset.
- Use it with the [`stablediffusion`](https://github.com/Stability-AI/stablediffusion) repository: download the `v2-1_512-ema-pruned.ckpt` [here](https://huggingface.co/stabilityai/stable-diffusion-2-1-base/resolve/main/v2-1_512-ema-pruned.ckpt).
- Use it with 🧨 [`diffusers`](#examples)
## Model Details
- **Developed by:** Robin Rombach, Patrick Esser
- **Model type:** Diffusion-based text-to-image generation model
- **Language(s):** English
- **License:** [CreativeML Open RAIL++-M License](https://huggingface.co/stabilityai/stable-diffusion-2/blob/main/LICENSE-MODEL)
- **Model Description:** This is a model that can be used to generate and modify images based on text prompts. It is a [Latent Diffusion Model](https://arxiv.org/abs/2112.10752) that uses a fixed, pretrained text encoder ([OpenCLIP-ViT/H](https://github.com/mlfoundations/open_clip)).
- **Resources for more information:** [GitHub Repository](https://github.com/Stability-AI/).
- **Cite as:**
@InProceedings{Rombach_2022_CVPR,
author = {Rombach, Robin and Blattmann, Andreas and Lorenz, Dominik and Esser, Patrick and Ommer, Bj\"orn},
title = {High-Resolution Image Synthesis With Latent Diffusion Models},
booktitle = {Proceedings of the IEEE/CVF Conference on Computer Vision and Pattern Recognition (CVPR)},
month = {June},
year = {2022},
pages = {10684-10695}
}
## Examples
Using the [🤗's Diffusers library](https://github.com/huggingface/diffusers) to run Stable Diffusion 2 in a simple and efficient manner.
```bash
pip install diffusers transformers accelerate scipy safetensors
```
Running the pipeline (if you don't swap the scheduler it will run with the default PNDM/PLMS scheduler, in this example we are swapping it to EulerDiscreteScheduler):
```python
from diffusers import StableDiffusionPipeline, EulerDiscreteScheduler
import torch
model_id = "stabilityai/stable-diffusion-2-1-base"
scheduler = EulerDiscreteScheduler.from_pretrained(model_id, subfolder="scheduler")
pipe = StableDiffusionPipeline.from_pretrained(model_id, scheduler=scheduler, torch_dtype=torch.float16)
pipe = pipe.to("cuda")
prompt = "a photo of an astronaut riding a horse on mars"
image = pipe(prompt).images[0]
image.save("astronaut_rides_horse.png")
```
**Notes**:
- Despite not being a dependency, we highly recommend you to install [xformers](https://github.com/facebookresearch/xformers) for memory efficient attention (better performance)
- If you have low GPU RAM available, make sure to add a `pipe.enable_attention_slicing()` after sending it to `cuda` for less VRAM usage (to the cost of speed)
# Uses
## Direct Use
The model is intended for research purposes only. Possible research areas and tasks include
- Safe deployment of models which have the potential to generate harmful content.
- Probing and understanding the limitations and biases of generative models.
- Generation of artworks and use in design and other artistic processes.
- Applications in educational or creative tools.
- Research on generative models.
Excluded uses are described below.
### Misuse, Malicious Use, and Out-of-Scope Use
_Note: This section is originally taken from the [DALLE-MINI model card](https://huggingface.co/dalle-mini/dalle-mini), was used for Stable Diffusion v1, but applies in the same way to Stable Diffusion v2_.
The model should not be used to intentionally create or disseminate images that create hostile or alienating environments for people. This includes generating images that people would foreseeably find disturbing, distressing, or offensive; or content that propagates historical or current stereotypes.
#### Out-of-Scope Use
The model was not trained to be factual or true representations of people or events, and therefore using the model to generate such content is out-of-scope for the abilities of this model.
#### Misuse and Malicious Use
Using the model to generate content that is cruel to individuals is a misuse of this model. This includes, but is not limited to:
- Generating demeaning, dehumanizing, or otherwise harmful representations of people or their environments, cultures, religions, etc.
- Intentionally promoting or propagating discriminatory content or harmful stereotypes.
- Impersonating individuals without their consent.
- Sexual content without consent of the people who might see it.
- Mis- and disinformation
- Representations of egregious violence and gore
- Sharing of copyrighted or licensed material in violation of its terms of use.
- Sharing content that is an alteration of copyrighted or licensed material in violation of its terms of use.
## Limitations and Bias
### Limitations
- The model does not achieve perfect photorealism
- The model cannot render legible text
- The model does not perform well on more difficult tasks which involve compositionality, such as rendering an image corresponding to “A red cube on top of a blue sphere”
- Faces and people in general may not be generated properly.
- The model was trained mainly with English captions and will not work as well in other languages.
- The autoencoding part of the model is lossy
- The model was trained on a subset of the large-scale dataset
[LAION-5B](https://laion.ai/blog/laion-5b/), which contains adult, violent and sexual content. To partially mitigate this, we have filtered the dataset using LAION's NFSW detector (see Training section).
### Bias
While the capabilities of image generation models are impressive, they can also reinforce or exacerbate social biases.
Stable Diffusion vw was primarily trained on subsets of [LAION-2B(en)](https://laion.ai/blog/laion-5b/),
which consists of images that are limited to English descriptions.
Texts and images from communities and cultures that use other languages are likely to be insufficiently accounted for.
This affects the overall output of the model, as white and western cultures are often set as the default. Further, the
ability of the model to generate content with non-English prompts is significantly worse than with English-language prompts.
Stable Diffusion v2 mirrors and exacerbates biases to such a degree that viewer discretion must be advised irrespective of the input or its intent.
## Training
**Training Data**
The model developers used the following dataset for training the model:
- LAION-5B and subsets (details below). The training data is further filtered using LAION's NSFW detector, with a "p_unsafe" score of 0.1 (conservative). For more details, please refer to LAION-5B's [NeurIPS 2022](https://openreview.net/forum?id=M3Y74vmsMcY) paper and reviewer discussions on the topic.
**Training Procedure**
Stable Diffusion v2 is a latent diffusion model which combines an autoencoder with a diffusion model that is trained in the latent space of the autoencoder. During training,
- Images are encoded through an encoder, which turns images into latent representations. The autoencoder uses a relative downsampling factor of 8 and maps images of shape H x W x 3 to latents of shape H/f x W/f x 4
- Text prompts are encoded through the OpenCLIP-ViT/H text-encoder.
- The output of the text encoder is fed into the UNet backbone of the latent diffusion model via cross-attention.
- The loss is a reconstruction objective between the noise that was added to the latent and the prediction made by the UNet. We also use the so-called _v-objective_, see https://arxiv.org/abs/2202.00512.
We currently provide the following checkpoints, for various versions:
### Version 2.1
- `512-base-ema.ckpt`: Fine-tuned on `512-base-ema.ckpt` 2.0 with 220k extra steps taken, with `punsafe=0.98` on the same dataset.
- `768-v-ema.ckpt`: Resumed from `768-v-ema.ckpt` 2.0 with an additional 55k steps on the same dataset (`punsafe=0.1`), and then fine-tuned for another 155k extra steps with `punsafe=0.98`.
### Version 2.0
- `512-base-ema.ckpt`: 550k steps at resolution `256x256` on a subset of [LAION-5B](https://laion.ai/blog/laion-5b/) filtered for explicit pornographic material, using the [LAION-NSFW classifier](https://github.com/LAION-AI/CLIP-based-NSFW-Detector) with `punsafe=0.1` and an [aesthetic score](https://github.com/christophschuhmann/improved-aesthetic-predictor) >= `4.5`.
850k steps at resolution `512x512` on the same dataset with resolution `>= 512x512`.
- `768-v-ema.ckpt`: Resumed from `512-base-ema.ckpt` and trained for 150k steps using a [v-objective](https://arxiv.org/abs/2202.00512) on the same dataset. Resumed for another 140k steps on a `768x768` subset of our dataset.
- `512-depth-ema.ckpt`: Resumed from `512-base-ema.ckpt` and finetuned for 200k steps. Added an extra input channel to process the (relative) depth prediction produced by [MiDaS](https://github.com/isl-org/MiDaS) (`dpt_hybrid`) which is used as an additional conditioning.
The additional input channels of the U-Net which process this extra information were zero-initialized.
- `512-inpainting-ema.ckpt`: Resumed from `512-base-ema.ckpt` and trained for another 200k steps. Follows the mask-generation strategy presented in [LAMA](https://github.com/saic-mdal/lama) which, in combination with the latent VAE representations of the masked image, are used as an additional conditioning.
The additional input channels of the U-Net which process this extra information were zero-initialized. The same strategy was used to train the [1.5-inpainting checkpoint](https://github.com/saic-mdal/lama).
- `x4-upscaling-ema.ckpt`: Trained for 1.25M steps on a 10M subset of LAION containing images `>2048x2048`. The model was trained on crops of size `512x512` and is a text-guided [latent upscaling diffusion model](https://arxiv.org/abs/2112.10752).
In addition to the textual input, it receives a `noise_level` as an input parameter, which can be used to add noise to the low-resolution input according to a [predefined diffusion schedule](configs/stable-diffusion/x4-upscaling.yaml).
- **Hardware:** 32 x 8 x A100 GPUs
- **Optimizer:** AdamW
- **Gradient Accumulations**: 1
- **Batch:** 32 x 8 x 2 x 4 = 2048
- **Learning rate:** warmup to 0.0001 for 10,000 steps and then kept constant
## Evaluation Results
Evaluations with different classifier-free guidance scales (1.5, 2.0, 3.0, 4.0,
5.0, 6.0, 7.0, 8.0) and 50 steps DDIM sampling steps show the relative improvements of the checkpoints:
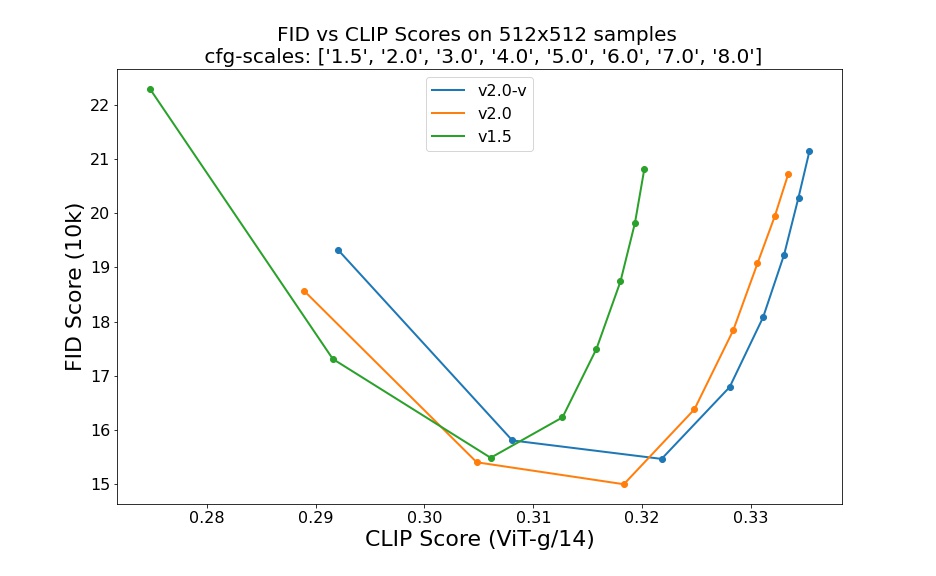
Evaluated using 50 DDIM steps and 10000 random prompts from the COCO2017 validation set, evaluated at 512x512 resolution. Not optimized for FID scores.
## Environmental Impact
**Stable Diffusion v1** **Estimated Emissions**
Based on that information, we estimate the following CO2 emissions using the [Machine Learning Impact calculator](https://mlco2.github.io/impact#compute) presented in [Lacoste et al. (2019)](https://arxiv.org/abs/1910.09700). The hardware, runtime, cloud provider, and compute region were utilized to estimate the carbon impact.
- **Hardware Type:** A100 PCIe 40GB
- **Hours used:** 200000
- **Cloud Provider:** AWS
- **Compute Region:** US-east
- **Carbon Emitted (Power consumption x Time x Carbon produced based on location of power grid):** 15000 kg CO2 eq.
## Citation
@InProceedings{Rombach_2022_CVPR,
author = {Rombach, Robin and Blattmann, Andreas and Lorenz, Dominik and Esser, Patrick and Ommer, Bj\"orn},
title = {High-Resolution Image Synthesis With Latent Diffusion Models},
booktitle = {Proceedings of the IEEE/CVF Conference on Computer Vision and Pattern Recognition (CVPR)},
month = {June},
year = {2022},
pages = {10684-10695}
}
*This model card was written by: Robin Rombach, Patrick Esser and David Ha and is based on the [Stable Diffusion v1](https://github.com/CompVis/stable-diffusion/blob/main/Stable_Diffusion_v1_Model_Card.md) and [DALL-E Mini model card](https://huggingface.co/dalle-mini/dalle-mini).* |
sentence-transformers/multi-qa-mpnet-base-dot-v1 | sentence-transformers | "2024-11-05T15:15:42Z" | 1,586,857 | 157 | sentence-transformers | [
"sentence-transformers",
"pytorch",
"onnx",
"safetensors",
"openvino",
"mpnet",
"fill-mask",
"feature-extraction",
"sentence-similarity",
"transformers",
"en",
"dataset:flax-sentence-embeddings/stackexchange_xml",
"dataset:ms_marco",
"dataset:gooaq",
"dataset:yahoo_answers_topics",
"dataset:search_qa",
"dataset:eli5",
"dataset:natural_questions",
"dataset:trivia_qa",
"dataset:embedding-data/QQP",
"dataset:embedding-data/PAQ_pairs",
"dataset:embedding-data/Amazon-QA",
"dataset:embedding-data/WikiAnswers",
"autotrain_compatible",
"endpoints_compatible",
"region:us"
] | sentence-similarity | "2022-03-02T23:29:05Z" | ---
language:
- en
library_name: sentence-transformers
tags:
- sentence-transformers
- feature-extraction
- sentence-similarity
- transformers
datasets:
- flax-sentence-embeddings/stackexchange_xml
- ms_marco
- gooaq
- yahoo_answers_topics
- search_qa
- eli5
- natural_questions
- trivia_qa
- embedding-data/QQP
- embedding-data/PAQ_pairs
- embedding-data/Amazon-QA
- embedding-data/WikiAnswers
pipeline_tag: sentence-similarity
---
# multi-qa-mpnet-base-dot-v1
This is a [sentence-transformers](https://www.SBERT.net) model: It maps sentences & paragraphs to a 768 dimensional dense vector space and was designed for **semantic search**. It has been trained on 215M (question, answer) pairs from diverse sources. For an introduction to semantic search, have a look at: [SBERT.net - Semantic Search](https://www.sbert.net/examples/applications/semantic-search/README.html)
## Usage (Sentence-Transformers)
Using this model becomes easy when you have [sentence-transformers](https://www.SBERT.net) installed:
```
pip install -U sentence-transformers
```
Then you can use the model like this:
```python
from sentence_transformers import SentenceTransformer, util
query = "How many people live in London?"
docs = ["Around 9 Million people live in London", "London is known for its financial district"]
#Load the model
model = SentenceTransformer('sentence-transformers/multi-qa-mpnet-base-dot-v1')
#Encode query and documents
query_emb = model.encode(query)
doc_emb = model.encode(docs)
#Compute dot score between query and all document embeddings
scores = util.dot_score(query_emb, doc_emb)[0].cpu().tolist()
#Combine docs & scores
doc_score_pairs = list(zip(docs, scores))
#Sort by decreasing score
doc_score_pairs = sorted(doc_score_pairs, key=lambda x: x[1], reverse=True)
#Output passages & scores
for doc, score in doc_score_pairs:
print(score, doc)
```
## Usage (HuggingFace Transformers)
Without [sentence-transformers](https://www.SBERT.net), you can use the model like this: First, you pass your input through the transformer model, then you have to apply the correct pooling-operation on-top of the contextualized word embeddings.
```python
from transformers import AutoTokenizer, AutoModel
import torch
#CLS Pooling - Take output from first token
def cls_pooling(model_output):
return model_output.last_hidden_state[:,0]
#Encode text
def encode(texts):
# Tokenize sentences
encoded_input = tokenizer(texts, padding=True, truncation=True, return_tensors='pt')
# Compute token embeddings
with torch.no_grad():
model_output = model(**encoded_input, return_dict=True)
# Perform pooling
embeddings = cls_pooling(model_output)
return embeddings
# Sentences we want sentence embeddings for
query = "How many people live in London?"
docs = ["Around 9 Million people live in London", "London is known for its financial district"]
# Load model from HuggingFace Hub
tokenizer = AutoTokenizer.from_pretrained("sentence-transformers/multi-qa-mpnet-base-dot-v1")
model = AutoModel.from_pretrained("sentence-transformers/multi-qa-mpnet-base-dot-v1")
#Encode query and docs
query_emb = encode(query)
doc_emb = encode(docs)
#Compute dot score between query and all document embeddings
scores = torch.mm(query_emb, doc_emb.transpose(0, 1))[0].cpu().tolist()
#Combine docs & scores
doc_score_pairs = list(zip(docs, scores))
#Sort by decreasing score
doc_score_pairs = sorted(doc_score_pairs, key=lambda x: x[1], reverse=True)
#Output passages & scores
for doc, score in doc_score_pairs:
print(score, doc)
```
## Technical Details
In the following some technical details how this model must be used:
| Setting | Value |
| --- | :---: |
| Dimensions | 768 |
| Produces normalized embeddings | No |
| Pooling-Method | CLS pooling |
| Suitable score functions | dot-product (e.g. `util.dot_score`) |
----
## Background
The project aims to train sentence embedding models on very large sentence level datasets using a self-supervised
contrastive learning objective. We use a contrastive learning objective: given a sentence from the pair, the model should predict which out of a set of randomly sampled other sentences, was actually paired with it in our dataset.
We developped this model during the
[Community week using JAX/Flax for NLP & CV](https://discuss.huggingface.co/t/open-to-the-community-community-week-using-jax-flax-for-nlp-cv/7104),
organized by Hugging Face. We developped this model as part of the project:
[Train the Best Sentence Embedding Model Ever with 1B Training Pairs](https://discuss.huggingface.co/t/train-the-best-sentence-embedding-model-ever-with-1b-training-pairs/7354). We benefited from efficient hardware infrastructure to run the project: 7 TPUs v3-8, as well as intervention from Googles Flax, JAX, and Cloud team member about efficient deep learning frameworks.
## Intended uses
Our model is intented to be used for semantic search: It encodes queries / questions and text paragraphs in a dense vector space. It finds relevant documents for the given passages.
Note that there is a limit of 512 word pieces: Text longer than that will be truncated. Further note that the model was just trained on input text up to 250 word pieces. It might not work well for longer text.
## Training procedure
The full training script is accessible in this current repository: `train_script.py`.
### Pre-training
We use the pretrained [`mpnet-base`](https://huggingface.co/microsoft/mpnet-base) model. Please refer to the model card for more detailed information about the pre-training procedure.
#### Training
We use the concatenation from multiple datasets to fine-tune our model. In total we have about 215M (question, answer) pairs.
We sampled each dataset given a weighted probability which configuration is detailed in the `data_config.json` file.
The model was trained with [MultipleNegativesRankingLoss](https://www.sbert.net/docs/package_reference/losses.html#multiplenegativesrankingloss) using CLS-pooling, dot-product as similarity function, and a scale of 1.
| Dataset | Number of training tuples |
|--------------------------------------------------------|:--------------------------:|
| [WikiAnswers](https://github.com/afader/oqa#wikianswers-corpus) Duplicate question pairs from WikiAnswers | 77,427,422 |
| [PAQ](https://github.com/facebookresearch/PAQ) Automatically generated (Question, Paragraph) pairs for each paragraph in Wikipedia | 64,371,441 |
| [Stack Exchange](https://huggingface.co/datasets/flax-sentence-embeddings/stackexchange_xml) (Title, Body) pairs from all StackExchanges | 25,316,456 |
| [Stack Exchange](https://huggingface.co/datasets/flax-sentence-embeddings/stackexchange_xml) (Title, Answer) pairs from all StackExchanges | 21,396,559 |
| [MS MARCO](https://microsoft.github.io/msmarco/) Triplets (query, answer, hard_negative) for 500k queries from Bing search engine | 17,579,773 |
| [GOOAQ: Open Question Answering with Diverse Answer Types](https://github.com/allenai/gooaq) (query, answer) pairs for 3M Google queries and Google featured snippet | 3,012,496 |
| [Amazon-QA](http://jmcauley.ucsd.edu/data/amazon/qa/) (Question, Answer) pairs from Amazon product pages | 2,448,839
| [Yahoo Answers](https://www.kaggle.com/soumikrakshit/yahoo-answers-dataset) (Title, Answer) pairs from Yahoo Answers | 1,198,260 |
| [Yahoo Answers](https://www.kaggle.com/soumikrakshit/yahoo-answers-dataset) (Question, Answer) pairs from Yahoo Answers | 681,164 |
| [Yahoo Answers](https://www.kaggle.com/soumikrakshit/yahoo-answers-dataset) (Title, Question) pairs from Yahoo Answers | 659,896 |
| [SearchQA](https://huggingface.co/datasets/search_qa) (Question, Answer) pairs for 140k questions, each with Top5 Google snippets on that question | 582,261 |
| [ELI5](https://huggingface.co/datasets/eli5) (Question, Answer) pairs from Reddit ELI5 (explainlikeimfive) | 325,475 |
| [Stack Exchange](https://huggingface.co/datasets/flax-sentence-embeddings/stackexchange_xml) Duplicate questions pairs (titles) | 304,525 |
| [Quora Question Triplets](https://quoradata.quora.com/First-Quora-Dataset-Release-Question-Pairs) (Question, Duplicate_Question, Hard_Negative) triplets for Quora Questions Pairs dataset | 103,663 |
| [Natural Questions (NQ)](https://ai.google.com/research/NaturalQuestions) (Question, Paragraph) pairs for 100k real Google queries with relevant Wikipedia paragraph | 100,231 |
| [SQuAD2.0](https://rajpurkar.github.io/SQuAD-explorer/) (Question, Paragraph) pairs from SQuAD2.0 dataset | 87,599 |
| [TriviaQA](https://huggingface.co/datasets/trivia_qa) (Question, Evidence) pairs | 73,346 |
| **Total** | **214,988,242** | |
ashawkey/mvdream-sd2.1-diffusers | ashawkey | "2024-01-14T05:03:05Z" | 1,573,169 | 4 | diffusers | [
"diffusers",
"safetensors",
"arxiv:2308.16512",
"license:openrail",
"diffusers:MVDreamPipeline",
"region:us"
] | null | "2024-01-13T11:27:07Z" | ---
license: openrail
---
# MVDream-diffusers Model Card
This is a port of https://huggingface.co/MVDream/MVDream into diffusers.
For usage, please check: https://github.com/ashawkey/mvdream_diffusers
## Citation
```
@article{shi2023MVDream,
author = {Shi, Yichun and Wang, Peng and Ye, Jianglong and Mai, Long and Li, Kejie and Yang, Xiao},
title = {MVDream: Multi-view Diffusion for 3D Generation},
journal = {arXiv:2308.16512},
year = {2023},
}
```
## Misuse, Malicious Use, and Out-of-Scope Use
The model should not be used to intentionally create or disseminate images that create hostile or alienating environments for people. This includes generating images that people would foreseeably find disturbing, distressing, or offensive; or content that propagates historical or current stereotypes.
|
MaziyarPanahi/Qwen2-7B-Instruct-GGUF | MaziyarPanahi | "2024-06-06T17:54:17Z" | 1,536,033 | 8 | transformers | [
"transformers",
"gguf",
"mistral",
"quantized",
"2-bit",
"3-bit",
"4-bit",
"5-bit",
"6-bit",
"8-bit",
"GGUF",
"text-generation",
"llama-3",
"llama",
"base_model:Qwen/Qwen2-7B-Instruct",
"base_model:quantized:Qwen/Qwen2-7B-Instruct",
"region:us",
"imatrix",
"conversational"
] | text-generation | "2024-06-06T17:14:16Z" | ---
tags:
- quantized
- 2-bit
- 3-bit
- 4-bit
- 5-bit
- 6-bit
- 8-bit
- GGUF
- text-generation
- llama-3
- llama
- text-generation
model_name: Qwen2-7B-Instruct-GGUF
base_model: Qwen/Qwen2-7B-Instruct
inference: false
model_creator: Qwen
pipeline_tag: text-generation
quantized_by: MaziyarPanahi
---
# [MaziyarPanahi/Qwen2-7B-Instruct-GGUF](https://huggingface.co/MaziyarPanahi/Qwen2-7B-Instruct-GGUF)
- Model creator: [Qwen](https://huggingface.co/Qwen)
- Original model: [Qwen/Qwen2-7B-Instruct](https://huggingface.co/Qwen/Qwen2-7B-Instruct)
## Description
[MaziyarPanahi/Qwen2-7B-Instruct-GGUF](https://huggingface.co/MaziyarPanahi/Qwen2-7B-Instruct-GGUF) contains GGUF format model files for [Qwen/Qwen2-7B-Instruct](https://huggingface.co/Qwen/Qwen2-7B-Instruct).
### About GGUF
GGUF is a new format introduced by the llama.cpp team on August 21st 2023. It is a replacement for GGML, which is no longer supported by llama.cpp.
Here is an incomplete list of clients and libraries that are known to support GGUF:
* [llama.cpp](https://github.com/ggerganov/llama.cpp). The source project for GGUF. Offers a CLI and a server option.
* [llama-cpp-python](https://github.com/abetlen/llama-cpp-python), a Python library with GPU accel, LangChain support, and OpenAI-compatible API server.
* [LM Studio](https://lmstudio.ai/), an easy-to-use and powerful local GUI for Windows and macOS (Silicon), with GPU acceleration. Linux available, in beta as of 27/11/2023.
* [text-generation-webui](https://github.com/oobabooga/text-generation-webui), the most widely used web UI, with many features and powerful extensions. Supports GPU acceleration.
* [KoboldCpp](https://github.com/LostRuins/koboldcpp), a fully featured web UI, with GPU accel across all platforms and GPU architectures. Especially good for story telling.
* [GPT4All](https://gpt4all.io/index.html), a free and open source local running GUI, supporting Windows, Linux and macOS with full GPU accel.
* [LoLLMS Web UI](https://github.com/ParisNeo/lollms-webui), a great web UI with many interesting and unique features, including a full model library for easy model selection.
* [Faraday.dev](https://faraday.dev/), an attractive and easy to use character-based chat GUI for Windows and macOS (both Silicon and Intel), with GPU acceleration.
* [candle](https://github.com/huggingface/candle), a Rust ML framework with a focus on performance, including GPU support, and ease of use.
* [ctransformers](https://github.com/marella/ctransformers), a Python library with GPU accel, LangChain support, and OpenAI-compatible AI server. Note, as of time of writing (November 27th 2023), ctransformers has not been updated in a long time and does not support many recent models.
## Special thanks
🙏 Special thanks to [Georgi Gerganov](https://github.com/ggerganov) and the whole team working on [llama.cpp](https://github.com/ggerganov/llama.cpp/) for making all of this possible. |
google-bert/bert-large-uncased | google-bert | "2024-02-19T11:06:54Z" | 1,532,420 | 114 | transformers | [
"transformers",
"pytorch",
"tf",
"jax",
"rust",
"safetensors",
"bert",
"fill-mask",
"en",
"dataset:bookcorpus",
"dataset:wikipedia",
"arxiv:1810.04805",
"license:apache-2.0",
"autotrain_compatible",
"endpoints_compatible",
"region:us"
] | fill-mask | "2022-03-02T23:29:04Z" | ---
language: en
license: apache-2.0
datasets:
- bookcorpus
- wikipedia
---
# BERT large model (uncased)
Pretrained model on English language using a masked language modeling (MLM) objective. It was introduced in
[this paper](https://arxiv.org/abs/1810.04805) and first released in
[this repository](https://github.com/google-research/bert). This model is uncased: it does not make a difference
between english and English.
Disclaimer: The team releasing BERT did not write a model card for this model so this model card has been written by
the Hugging Face team.
## Model description
BERT is a transformers model pretrained on a large corpus of English data in a self-supervised fashion. This means it
was pretrained on the raw texts only, with no humans labelling them in any way (which is why it can use lots of
publicly available data) with an automatic process to generate inputs and labels from those texts. More precisely, it
was pretrained with two objectives:
- Masked language modeling (MLM): taking a sentence, the model randomly masks 15% of the words in the input then run
the entire masked sentence through the model and has to predict the masked words. This is different from traditional
recurrent neural networks (RNNs) that usually see the words one after the other, or from autoregressive models like
GPT which internally mask the future tokens. It allows the model to learn a bidirectional representation of the
sentence.
- Next sentence prediction (NSP): the models concatenates two masked sentences as inputs during pretraining. Sometimes
they correspond to sentences that were next to each other in the original text, sometimes not. The model then has to
predict if the two sentences were following each other or not.
This way, the model learns an inner representation of the English language that can then be used to extract features
useful for downstream tasks: if you have a dataset of labeled sentences for instance, you can train a standard
classifier using the features produced by the BERT model as inputs.
This model has the following configuration:
- 24-layer
- 1024 hidden dimension
- 16 attention heads
- 336M parameters.
## Intended uses & limitations
You can use the raw model for either masked language modeling or next sentence prediction, but it's mostly intended to
be fine-tuned on a downstream task. See the [model hub](https://huggingface.co/models?filter=bert) to look for
fine-tuned versions on a task that interests you.
Note that this model is primarily aimed at being fine-tuned on tasks that use the whole sentence (potentially masked)
to make decisions, such as sequence classification, token classification or question answering. For tasks such as text
generation you should look at model like GPT2.
### How to use
You can use this model directly with a pipeline for masked language modeling:
```python
>>> from transformers import pipeline
>>> unmasker = pipeline('fill-mask', model='bert-large-uncased')
>>> unmasker("Hello I'm a [MASK] model.")
[{'sequence': "[CLS] hello i'm a fashion model. [SEP]",
'score': 0.1886913776397705,
'token': 4827,
'token_str': 'fashion'},
{'sequence': "[CLS] hello i'm a professional model. [SEP]",
'score': 0.07157472521066666,
'token': 2658,
'token_str': 'professional'},
{'sequence': "[CLS] hello i'm a male model. [SEP]",
'score': 0.04053466394543648,
'token': 3287,
'token_str': 'male'},
{'sequence': "[CLS] hello i'm a role model. [SEP]",
'score': 0.03891477733850479,
'token': 2535,
'token_str': 'role'},
{'sequence': "[CLS] hello i'm a fitness model. [SEP]",
'score': 0.03038121573626995,
'token': 10516,
'token_str': 'fitness'}]
```
Here is how to use this model to get the features of a given text in PyTorch:
```python
from transformers import BertTokenizer, BertModel
tokenizer = BertTokenizer.from_pretrained('bert-large-uncased')
model = BertModel.from_pretrained("bert-large-uncased")
text = "Replace me by any text you'd like."
encoded_input = tokenizer(text, return_tensors='pt')
output = model(**encoded_input)
```
and in TensorFlow:
```python
from transformers import BertTokenizer, TFBertModel
tokenizer = BertTokenizer.from_pretrained('bert-large-uncased')
model = TFBertModel.from_pretrained("bert-large-uncased")
text = "Replace me by any text you'd like."
encoded_input = tokenizer(text, return_tensors='tf')
output = model(encoded_input)
```
### Limitations and bias
Even if the training data used for this model could be characterized as fairly neutral, this model can have biased
predictions:
```python
>>> from transformers import pipeline
>>> unmasker = pipeline('fill-mask', model='bert-large-uncased')
>>> unmasker("The man worked as a [MASK].")
[{'sequence': '[CLS] the man worked as a bartender. [SEP]',
'score': 0.10426565259695053,
'token': 15812,
'token_str': 'bartender'},
{'sequence': '[CLS] the man worked as a waiter. [SEP]',
'score': 0.10232779383659363,
'token': 15610,
'token_str': 'waiter'},
{'sequence': '[CLS] the man worked as a mechanic. [SEP]',
'score': 0.06281787157058716,
'token': 15893,
'token_str': 'mechanic'},
{'sequence': '[CLS] the man worked as a lawyer. [SEP]',
'score': 0.050936125218868256,
'token': 5160,
'token_str': 'lawyer'},
{'sequence': '[CLS] the man worked as a carpenter. [SEP]',
'score': 0.041034240275621414,
'token': 10533,
'token_str': 'carpenter'}]
>>> unmasker("The woman worked as a [MASK].")
[{'sequence': '[CLS] the woman worked as a waitress. [SEP]',
'score': 0.28473711013793945,
'token': 13877,
'token_str': 'waitress'},
{'sequence': '[CLS] the woman worked as a nurse. [SEP]',
'score': 0.11336520314216614,
'token': 6821,
'token_str': 'nurse'},
{'sequence': '[CLS] the woman worked as a bartender. [SEP]',
'score': 0.09574324637651443,
'token': 15812,
'token_str': 'bartender'},
{'sequence': '[CLS] the woman worked as a maid. [SEP]',
'score': 0.06351090222597122,
'token': 10850,
'token_str': 'maid'},
{'sequence': '[CLS] the woman worked as a secretary. [SEP]',
'score': 0.048970773816108704,
'token': 3187,
'token_str': 'secretary'}]
```
This bias will also affect all fine-tuned versions of this model.
## Training data
The BERT model was pretrained on [BookCorpus](https://yknzhu.wixsite.com/mbweb), a dataset consisting of 11,038
unpublished books and [English Wikipedia](https://en.wikipedia.org/wiki/English_Wikipedia) (excluding lists, tables and
headers).
## Training procedure
### Preprocessing
The texts are lowercased and tokenized using WordPiece and a vocabulary size of 30,000. The inputs of the model are
then of the form:
```
[CLS] Sentence A [SEP] Sentence B [SEP]
```
With probability 0.5, sentence A and sentence B correspond to two consecutive sentences in the original corpus and in
the other cases, it's another random sentence in the corpus. Note that what is considered a sentence here is a
consecutive span of text usually longer than a single sentence. The only constrain is that the result with the two
"sentences" has a combined length of less than 512 tokens.
The details of the masking procedure for each sentence are the following:
- 15% of the tokens are masked.
- In 80% of the cases, the masked tokens are replaced by `[MASK]`.
- In 10% of the cases, the masked tokens are replaced by a random token (different) from the one they replace.
- In the 10% remaining cases, the masked tokens are left as is.
### Pretraining
The model was trained on 4 cloud TPUs in Pod configuration (16 TPU chips total) for one million steps with a batch size
of 256. The sequence length was limited to 128 tokens for 90% of the steps and 512 for the remaining 10%. The optimizer
used is Adam with a learning rate of 1e-4, \\(\beta_{1} = 0.9\\) and \\(\beta_{2} = 0.999\\), a weight decay of 0.01,
learning rate warmup for 10,000 steps and linear decay of the learning rate after.
## Evaluation results
When fine-tuned on downstream tasks, this model achieves the following results:
Model | SQUAD 1.1 F1/EM | Multi NLI Accuracy
---------------------------------------- | :-------------: | :----------------:
BERT-Large, Uncased (Original) | 91.0/84.3 | 86.05
### BibTeX entry and citation info
```bibtex
@article{DBLP:journals/corr/abs-1810-04805,
author = {Jacob Devlin and
Ming{-}Wei Chang and
Kenton Lee and
Kristina Toutanova},
title = {{BERT:} Pre-training of Deep Bidirectional Transformers for Language
Understanding},
journal = {CoRR},
volume = {abs/1810.04805},
year = {2018},
url = {http://arxiv.org/abs/1810.04805},
archivePrefix = {arXiv},
eprint = {1810.04805},
timestamp = {Tue, 30 Oct 2018 20:39:56 +0100},
biburl = {https://dblp.org/rec/journals/corr/abs-1810-04805.bib},
bibsource = {dblp computer science bibliography, https://dblp.org}
}
``` |
MaziyarPanahi/Qwen2-1.5B-Instruct-GGUF | MaziyarPanahi | "2024-06-06T19:06:35Z" | 1,528,034 | 8 | transformers | [
"transformers",
"gguf",
"mistral",
"quantized",
"2-bit",
"3-bit",
"4-bit",
"5-bit",
"6-bit",
"8-bit",
"GGUF",
"text-generation",
"llama-3",
"llama",
"base_model:Qwen/Qwen2-1.5B-Instruct",
"base_model:quantized:Qwen/Qwen2-1.5B-Instruct",
"region:us",
"imatrix",
"conversational"
] | text-generation | "2024-06-06T18:59:09Z" | ---
tags:
- quantized
- 2-bit
- 3-bit
- 4-bit
- 5-bit
- 6-bit
- 8-bit
- GGUF
- text-generation
- llama-3
- llama
- text-generation
model_name: Qwen2-1.5B-Instruct-GGUF
base_model: Qwen/Qwen2-1.5B-Instruct
inference: false
model_creator: Qwen
pipeline_tag: text-generation
quantized_by: MaziyarPanahi
---
# [MaziyarPanahi/Qwen2-1.5B-Instruct-GGUF](https://huggingface.co/MaziyarPanahi/Qwen2-1.5B-Instruct-GGUF)
- Model creator: [Qwen](https://huggingface.co/Qwen)
- Original model: [Qwen/Qwen2-1.5B-Instruct](https://huggingface.co/Qwen/Qwen2-1.5B-Instruct)
## Description
[MaziyarPanahi/Qwen2-1.5B-Instruct-GGUF](https://huggingface.co/MaziyarPanahi/Qwen2-1.5B-Instruct-GGUF) contains GGUF format model files for [Qwen/Qwen2-1.5B-Instruct](https://huggingface.co/Qwen/Qwen2-1.5B-Instruct).
### About GGUF
GGUF is a new format introduced by the llama.cpp team on August 21st 2023. It is a replacement for GGML, which is no longer supported by llama.cpp.
Here is an incomplete list of clients and libraries that are known to support GGUF:
* [llama.cpp](https://github.com/ggerganov/llama.cpp). The source project for GGUF. Offers a CLI and a server option.
* [llama-cpp-python](https://github.com/abetlen/llama-cpp-python), a Python library with GPU accel, LangChain support, and OpenAI-compatible API server.
* [LM Studio](https://lmstudio.ai/), an easy-to-use and powerful local GUI for Windows and macOS (Silicon), with GPU acceleration. Linux available, in beta as of 27/11/2023.
* [text-generation-webui](https://github.com/oobabooga/text-generation-webui), the most widely used web UI, with many features and powerful extensions. Supports GPU acceleration.
* [KoboldCpp](https://github.com/LostRuins/koboldcpp), a fully featured web UI, with GPU accel across all platforms and GPU architectures. Especially good for story telling.
* [GPT4All](https://gpt4all.io/index.html), a free and open source local running GUI, supporting Windows, Linux and macOS with full GPU accel.
* [LoLLMS Web UI](https://github.com/ParisNeo/lollms-webui), a great web UI with many interesting and unique features, including a full model library for easy model selection.
* [Faraday.dev](https://faraday.dev/), an attractive and easy to use character-based chat GUI for Windows and macOS (both Silicon and Intel), with GPU acceleration.
* [candle](https://github.com/huggingface/candle), a Rust ML framework with a focus on performance, including GPU support, and ease of use.
* [ctransformers](https://github.com/marella/ctransformers), a Python library with GPU accel, LangChain support, and OpenAI-compatible AI server. Note, as of time of writing (November 27th 2023), ctransformers has not been updated in a long time and does not support many recent models.
## Special thanks
🙏 Special thanks to [Georgi Gerganov](https://github.com/ggerganov) and the whole team working on [llama.cpp](https://github.com/ggerganov/llama.cpp/) for making all of this possible. |
kyutai/mimi | kyutai | "2024-09-18T14:09:30Z" | 1,478,715 | 83 | transformers | [
"transformers",
"safetensors",
"mimi",
"feature-extraction",
"audio",
"license:cc-by-4.0",
"endpoints_compatible",
"region:us"
] | feature-extraction | "2024-09-10T12:38:15Z" | ---
license: cc-by-4.0
library_name: transformers
tags:
- mimi
- audio
---

# Model Card for Mimi
Mimi codec is a state-of-the-art audio neural codec, developped by [Kyutai](https://kyutai.org/), that combines semantic and acoustic information into audio tokens running at 12Hz and a bitrate of 1.1kbps.
## Model Details
### Model Description
Mimi is a high-fidelity audio codec leveraging neural networks. It introduces a streaming encoder-decoder architecture with quantized latent space, trained in an end-to-end fashion.
It was trained on speech data, which makes it particularly adapted to train speech language models or text-to-speech systems.
- **Developed by:** Kyutai
- **Model type:** Audio codec
- **Audio types:** Speech
- **License:** CC-BY
### Model Sources
- **Repository:** [repo](https://github.com/kyutai-labs/moshi)
- **Paper:** [paper](http://kyutai.org/Moshi.pdf)
- **Demo:** [demo](https://moshi.chat/)
## Uses
## How to Get Started with the Model
### Usage with `transformers`
Use the following code to get started with the Mimi model using a dummy example from the LibriSpeech dataset (~9MB). First, install the required Python packages:
```
pip install --upgrade pip
pip install --upgrade datasets[audio]
pip install git+https://github.com/huggingface/transformers.git@main
```
Then load an audio sample, and run a forward pass of the model:
```python
from datasets import load_dataset, Audio
from transformers import MimiModel, AutoFeatureExtractor
# load a demonstration datasets
librispeech_dummy = load_dataset("hf-internal-testing/librispeech_asr_dummy", "clean", split="validation")
# load the model + feature extractor (for pre-processing the audio)
model = MimiModel.from_pretrained("kyutai/mimi")
feature_extractor = AutoFeatureExtractor.from_pretrained("kyutai/mimi")
# cast the audio data to the correct sampling rate for the model
librispeech_dummy = librispeech_dummy.cast_column("audio", Audio(sampling_rate=feature_extractor.sampling_rate))
audio_sample = librispeech_dummy[0]["audio"]["array"]
# pre-process the inputs
inputs = feature_extractor(raw_audio=audio_sample, sampling_rate=feature_extractor.sampling_rate, return_tensors="pt")
# explicitly encode then decode the audio inputs
encoder_outputs = model.encode(inputs["input_values"])
audio_values = model.decode(encoder_outputs.audio_codes)[0]
# or the equivalent with a forward pass
audio_values = model(inputs["input_values"]).audio_values
```
### Usage with Moshi
See the main [README](https://github.com/kyutai-labs/moshi) file.
### Direct Use
Mimi can be used directly as an audio codec for real-time compression and decompression of speech signals.
It provides high-quality audio compression and efficient decoding.
### Out-of-Scope Use
The model is not intended to be used to impersonate other people or any malicious use of any kind.
## Bias, Risks, and Limitations
The model has been trained with a few safeguards to try to limit potential toxic usages, however our toxicity analysis shows that it behaves in the middle of existing models with respect to textual generation. It has some bias towards certain domains and topics that are over-represented in the training data. Its capabilities are relatively limited so far and it is trained to produce only one voice to avoid impersonation. Yet, we need the perspective in time to establish the sociotechnical limitations.
## Training Details
### Training Data
The training data is detailled in the paper.
### Training procedure and hyper-parameters
The different stages of the training procedure are detailled in the paper along with the hyper-parameters.
## Citation
```
@techreport{kyutai2024moshi,
author = {Alexandre D\'efossez and Laurent Mazar\'e and Manu Orsini and Am\'elie Royer and Patrick P\'erez and Herv\'e J\'egou and Edouard Grave and Neil Zeghidour},
title = {Moshi: a speech-text foundation model for real-time dialogue},
institution = {Kyutai},
year={2024},
month={September},
url={http://kyutai.org/Moshi.pdf},
}
```
## Model Card Authors
Alexandre Défossez, Laurent Mazaré, Manu Orsini, Amélie Royer, Patrick Pérez, Hervé Jégou, Edouard Grave, Neil Zeghidour, Yoach Lacombe |
facebook/esm2_t36_3B_UR50D | facebook | "2022-12-01T20:22:22Z" | 1,477,407 | 14 | transformers | [
"transformers",
"pytorch",
"tf",
"esm",
"fill-mask",
"license:mit",
"autotrain_compatible",
"endpoints_compatible",
"region:us"
] | fill-mask | "2022-10-13T12:38:30Z" | ---
license: mit
widget:
- text: "MQIFVKTLTGKTITLEVEPS<mask>TIENVKAKIQDKEGIPPDQQRLIFAGKQLEDGRTLSDYNIQKESTLHLVLRLRGG"
---
## ESM-2
ESM-2 is a state-of-the-art protein model trained on a masked language modelling objective. It is suitable for fine-tuning on a wide range of tasks that take protein sequences as input. For detailed information on the model architecture and training data, please refer to the [accompanying paper](https://www.biorxiv.org/content/10.1101/2022.07.20.500902v2). You may also be interested in some demo notebooks ([PyTorch](https://colab.research.google.com/github/huggingface/notebooks/blob/main/examples/protein_language_modeling.ipynb), [TensorFlow](https://colab.research.google.com/github/huggingface/notebooks/blob/main/examples/protein_language_modeling-tf.ipynb)) which demonstrate how to fine-tune ESM-2 models on your tasks of interest.
Several ESM-2 checkpoints are available in the Hub with varying sizes. Larger sizes generally have somewhat better accuracy, but require much more memory and time to train:
| Checkpoint name | Num layers | Num parameters |
|------------------------------|----|----------|
| [esm2_t48_15B_UR50D](https://huggingface.co/facebook/esm2_t48_15B_UR50D) | 48 | 15B |
| [esm2_t36_3B_UR50D](https://huggingface.co/facebook/esm2_t36_3B_UR50D) | 36 | 3B |
| [esm2_t33_650M_UR50D](https://huggingface.co/facebook/esm2_t33_650M_UR50D) | 33 | 650M |
| [esm2_t30_150M_UR50D](https://huggingface.co/facebook/esm2_t30_150M_UR50D) | 30 | 150M |
| [esm2_t12_35M_UR50D](https://huggingface.co/facebook/esm2_t12_35M_UR50D) | 12 | 35M |
| [esm2_t6_8M_UR50D](https://huggingface.co/facebook/esm2_t6_8M_UR50D) | 6 | 8M | |
allenai/scibert_scivocab_uncased | allenai | "2022-10-03T22:06:12Z" | 1,472,821 | 127 | transformers | [
"transformers",
"pytorch",
"jax",
"bert",
"en",
"endpoints_compatible",
"region:us"
] | null | "2022-03-02T23:29:05Z" | ---
language: en
---
# SciBERT
This is the pretrained model presented in [SciBERT: A Pretrained Language Model for Scientific Text](https://www.aclweb.org/anthology/D19-1371/), which is a BERT model trained on scientific text.
The training corpus was papers taken from [Semantic Scholar](https://www.semanticscholar.org). Corpus size is 1.14M papers, 3.1B tokens. We use the full text of the papers in training, not just abstracts.
SciBERT has its own wordpiece vocabulary (scivocab) that's built to best match the training corpus. We trained cased and uncased versions.
Available models include:
* `scibert_scivocab_cased`
* `scibert_scivocab_uncased`
The original repo can be found [here](https://github.com/allenai/scibert).
If using these models, please cite the following paper:
```
@inproceedings{beltagy-etal-2019-scibert,
title = "SciBERT: A Pretrained Language Model for Scientific Text",
author = "Beltagy, Iz and Lo, Kyle and Cohan, Arman",
booktitle = "EMNLP",
year = "2019",
publisher = "Association for Computational Linguistics",
url = "https://www.aclweb.org/anthology/D19-1371"
}
```
|
prajjwal1/bert-tiny | prajjwal1 | "2021-10-27T18:29:01Z" | 1,450,930 | 102 | transformers | [
"transformers",
"pytorch",
"BERT",
"MNLI",
"NLI",
"transformer",
"pre-training",
"en",
"arxiv:1908.08962",
"arxiv:2110.01518",
"license:mit",
"endpoints_compatible",
"region:us"
] | null | "2022-03-02T23:29:05Z" | ---
language:
- en
license:
- mit
tags:
- BERT
- MNLI
- NLI
- transformer
- pre-training
---
The following model is a Pytorch pre-trained model obtained from converting Tensorflow checkpoint found in the [official Google BERT repository](https://github.com/google-research/bert).
This is one of the smaller pre-trained BERT variants, together with [bert-mini](https://huggingface.co/prajjwal1/bert-mini) [bert-small](https://huggingface.co/prajjwal1/bert-small) and [bert-medium](https://huggingface.co/prajjwal1/bert-medium). They were introduced in the study `Well-Read Students Learn Better: On the Importance of Pre-training Compact Models` ([arxiv](https://arxiv.org/abs/1908.08962)), and ported to HF for the study `Generalization in NLI: Ways (Not) To Go Beyond Simple Heuristics` ([arXiv](https://arxiv.org/abs/2110.01518)). These models are supposed to be trained on a downstream task.
If you use the model, please consider citing both the papers:
```
@misc{bhargava2021generalization,
title={Generalization in NLI: Ways (Not) To Go Beyond Simple Heuristics},
author={Prajjwal Bhargava and Aleksandr Drozd and Anna Rogers},
year={2021},
eprint={2110.01518},
archivePrefix={arXiv},
primaryClass={cs.CL}
}
@article{DBLP:journals/corr/abs-1908-08962,
author = {Iulia Turc and
Ming{-}Wei Chang and
Kenton Lee and
Kristina Toutanova},
title = {Well-Read Students Learn Better: The Impact of Student Initialization
on Knowledge Distillation},
journal = {CoRR},
volume = {abs/1908.08962},
year = {2019},
url = {http://arxiv.org/abs/1908.08962},
eprinttype = {arXiv},
eprint = {1908.08962},
timestamp = {Thu, 29 Aug 2019 16:32:34 +0200},
biburl = {https://dblp.org/rec/journals/corr/abs-1908-08962.bib},
bibsource = {dblp computer science bibliography, https://dblp.org}
}
```
Config of this model:
- `prajjwal1/bert-tiny` (L=2, H=128) [Model Link](https://huggingface.co/prajjwal1/bert-tiny)
Other models to check out:
- `prajjwal1/bert-mini` (L=4, H=256) [Model Link](https://huggingface.co/prajjwal1/bert-mini)
- `prajjwal1/bert-small` (L=4, H=512) [Model Link](https://huggingface.co/prajjwal1/bert-small)
- `prajjwal1/bert-medium` (L=8, H=512) [Model Link](https://huggingface.co/prajjwal1/bert-medium)
Original Implementation and more info can be found in [this Github repository](https://github.com/prajjwal1/generalize_lm_nli).
Twitter: [@prajjwal_1](https://twitter.com/prajjwal_1)
|
IDEA-Research/grounding-dino-base | IDEA-Research | "2024-05-12T09:03:22Z" | 1,446,863 | 63 | transformers | [
"transformers",
"pytorch",
"safetensors",
"grounding-dino",
"zero-shot-object-detection",
"vision",
"arxiv:2303.05499",
"license:apache-2.0",
"region:us"
] | zero-shot-object-detection | "2023-09-25T01:27:30Z" | ---
license: apache-2.0
tags:
- vision
inference: false
pipeline_tag: zero-shot-object-detection
---
# Grounding DINO model (base variant)
The Grounding DINO model was proposed in [Grounding DINO: Marrying DINO with Grounded Pre-Training for Open-Set Object Detection](https://arxiv.org/abs/2303.05499) by Shilong Liu, Zhaoyang Zeng, Tianhe Ren, Feng Li, Hao Zhang, Jie Yang, Chunyuan Li, Jianwei Yang, Hang Su, Jun Zhu, Lei Zhang. Grounding DINO extends a closed-set object detection model with a text encoder, enabling open-set object detection. The model achieves remarkable results, such as 52.5 AP on COCO zero-shot.
<img src="https://huggingface.co/datasets/huggingface/documentation-images/resolve/main/transformers/model_doc/grouding_dino_architecture.png"
alt="drawing" width="600"/>
<small> Grounding DINO overview. Taken from the <a href="https://arxiv.org/abs/2303.05499">original paper</a>. </small>
## Intended uses & limitations
You can use the raw model for zero-shot object detection (the task of detecting things in an image out-of-the-box without labeled data).
### How to use
Here's how to use the model for zero-shot object detection:
```python
import requests
import torch
from PIL import Image
from transformers import AutoProcessor, AutoModelForZeroShotObjectDetection
model_id = "IDEA-Research/grounding-dino-base"
device = "cuda" if torch.cuda.is_available() else "cpu"
processor = AutoProcessor.from_pretrained(model_id)
model = AutoModelForZeroShotObjectDetection.from_pretrained(model_id).to(device)
image_url = "http://images.cocodataset.org/val2017/000000039769.jpg"
image = Image.open(requests.get(image_url, stream=True).raw)
# Check for cats and remote controls
# VERY important: text queries need to be lowercased + end with a dot
text = "a cat. a remote control."
inputs = processor(images=image, text=text, return_tensors="pt").to(device)
with torch.no_grad():
outputs = model(**inputs)
results = processor.post_process_grounded_object_detection(
outputs,
inputs.input_ids,
box_threshold=0.4,
text_threshold=0.3,
target_sizes=[image.size[::-1]]
)
```
### BibTeX entry and citation info
```bibtex
@misc{liu2023grounding,
title={Grounding DINO: Marrying DINO with Grounded Pre-Training for Open-Set Object Detection},
author={Shilong Liu and Zhaoyang Zeng and Tianhe Ren and Feng Li and Hao Zhang and Jie Yang and Chunyuan Li and Jianwei Yang and Hang Su and Jun Zhu and Lei Zhang},
year={2023},
eprint={2303.05499},
archivePrefix={arXiv},
primaryClass={cs.CV}
}
``` |
yiyanghkust/finbert-tone | yiyanghkust | "2022-10-17T00:35:39Z" | 1,439,864 | 153 | transformers | [
"transformers",
"pytorch",
"tf",
"text-classification",
"financial-sentiment-analysis",
"sentiment-analysis",
"en",
"autotrain_compatible",
"endpoints_compatible",
"region:us"
] | text-classification | "2022-03-02T23:29:05Z" | ---
language: "en"
tags:
- financial-sentiment-analysis
- sentiment-analysis
widget:
- text: "growth is strong and we have plenty of liquidity"
---
`FinBERT` is a BERT model pre-trained on financial communication text. The purpose is to enhance financial NLP research and practice. It is trained on the following three financial communication corpus. The total corpora size is 4.9B tokens.
- Corporate Reports 10-K & 10-Q: 2.5B tokens
- Earnings Call Transcripts: 1.3B tokens
- Analyst Reports: 1.1B tokens
More technical details on `FinBERT`: [Click Link](https://github.com/yya518/FinBERT)
This released `finbert-tone` model is the `FinBERT` model fine-tuned on 10,000 manually annotated (positive, negative, neutral) sentences from analyst reports. This model achieves superior performance on financial tone analysis task. If you are simply interested in using `FinBERT` for financial tone analysis, give it a try.
If you use the model in your academic work, please cite the following paper:
Huang, Allen H., Hui Wang, and Yi Yang. "FinBERT: A Large Language Model for Extracting Information from Financial Text." *Contemporary Accounting Research* (2022).
# How to use
You can use this model with Transformers pipeline for sentiment analysis.
```python
from transformers import BertTokenizer, BertForSequenceClassification
from transformers import pipeline
finbert = BertForSequenceClassification.from_pretrained('yiyanghkust/finbert-tone',num_labels=3)
tokenizer = BertTokenizer.from_pretrained('yiyanghkust/finbert-tone')
nlp = pipeline("sentiment-analysis", model=finbert, tokenizer=tokenizer)
sentences = ["there is a shortage of capital, and we need extra financing",
"growth is strong and we have plenty of liquidity",
"there are doubts about our finances",
"profits are flat"]
results = nlp(sentences)
print(results) #LABEL_0: neutral; LABEL_1: positive; LABEL_2: negative
``` |
dunzhang/stella_en_400M_v5 | dunzhang | "2024-09-28T13:10:16Z" | 1,433,205 | 112 | sentence-transformers | [
"sentence-transformers",
"pytorch",
"safetensors",
"new",
"feature-extraction",
"mteb",
"transformers",
"sentence-similarity",
"custom_code",
"arxiv:2205.13147",
"license:mit",
"model-index",
"autotrain_compatible",
"text-embeddings-inference",
"endpoints_compatible",
"region:us"
] | sentence-similarity | "2024-07-12T15:52:33Z" | ---
model-index:
- name: stella_en_400M_v5
results:
- dataset:
config: en
name: MTEB AmazonCounterfactualClassification (en)
revision: e8379541af4e31359cca9fbcf4b00f2671dba205
split: test
type: mteb/amazon_counterfactual
metrics:
- type: accuracy
value: 92.35820895522387
- type: ap
value: 70.81322736988783
- type: ap_weighted
value: 70.81322736988783
- type: f1
value: 88.9505466159595
- type: f1_weighted
value: 92.68630932872613
- type: main_score
value: 92.35820895522387
task:
type: Classification
- dataset:
config: default
name: MTEB AmazonPolarityClassification
revision: e2d317d38cd51312af73b3d32a06d1a08b442046
split: test
type: mteb/amazon_polarity
metrics:
- type: accuracy
value: 97.1945
- type: ap
value: 96.08192192244094
- type: ap_weighted
value: 96.08192192244094
- type: f1
value: 97.1936887167346
- type: f1_weighted
value: 97.1936887167346
- type: main_score
value: 97.1945
task:
type: Classification
- dataset:
config: en
name: MTEB AmazonReviewsClassification (en)
revision: 1399c76144fd37290681b995c656ef9b2e06e26d
split: test
type: mteb/amazon_reviews_multi
metrics:
- type: accuracy
value: 59.528000000000006
- type: f1
value: 59.21016819840188
- type: f1_weighted
value: 59.21016819840188
- type: main_score
value: 59.528000000000006
task:
type: Classification
- dataset:
config: default
name: MTEB ArguAna
revision: c22ab2a51041ffd869aaddef7af8d8215647e41a
split: test
type: mteb/arguana
metrics:
- type: main_score
value: 64.24
- type: map_at_1
value: 40.398
- type: map_at_10
value: 56.215
- type: map_at_100
value: 56.833999999999996
- type: map_at_1000
value: 56.835
- type: map_at_20
value: 56.747
- type: map_at_3
value: 52.181
- type: map_at_5
value: 54.628
- type: mrr_at_1
value: 41.25177809388336
- type: mrr_at_10
value: 56.570762491815216
- type: mrr_at_100
value: 57.17548614361504
- type: mrr_at_1000
value: 57.176650626377466
- type: mrr_at_20
value: 57.08916253512566
- type: mrr_at_3
value: 52.47747747747754
- type: mrr_at_5
value: 54.94547178757718
- type: nauc_map_at_1000_diff1
value: 22.408086887100158
- type: nauc_map_at_1000_max
value: -8.730419096847543
- type: nauc_map_at_1000_std
value: -17.789262741255737
- type: nauc_map_at_100_diff1
value: 22.407371684274025
- type: nauc_map_at_100_max
value: -8.732263549026266
- type: nauc_map_at_100_std
value: -17.79550515579994
- type: nauc_map_at_10_diff1
value: 21.925005073301246
- type: nauc_map_at_10_max
value: -8.990323944492134
- type: nauc_map_at_10_std
value: -18.199246301671458
- type: nauc_map_at_1_diff1
value: 26.23276644969203
- type: nauc_map_at_1_max
value: -12.376511389571245
- type: nauc_map_at_1_std
value: -18.11411715207284
- type: nauc_map_at_20_diff1
value: 22.32455790850922
- type: nauc_map_at_20_max
value: -8.664671547236034
- type: nauc_map_at_20_std
value: -17.8290016125137
- type: nauc_map_at_3_diff1
value: 22.395462147465064
- type: nauc_map_at_3_max
value: -8.206580750918844
- type: nauc_map_at_3_std
value: -17.604490446911484
- type: nauc_map_at_5_diff1
value: 21.95307379904799
- type: nauc_map_at_5_max
value: -8.03958102978443
- type: nauc_map_at_5_std
value: -17.36578866595004
- type: nauc_mrr_at_1000_diff1
value: 20.124236798365587
- type: nauc_mrr_at_1000_max
value: -9.587376069575898
- type: nauc_mrr_at_1000_std
value: -17.79191612151833
- type: nauc_mrr_at_100_diff1
value: 20.123612603474033
- type: nauc_mrr_at_100_max
value: -9.589187218607831
- type: nauc_mrr_at_100_std
value: -17.7981617777748
- type: nauc_mrr_at_10_diff1
value: 19.723683875738075
- type: nauc_mrr_at_10_max
value: -9.774151729178815
- type: nauc_mrr_at_10_std
value: -18.168668675495162
- type: nauc_mrr_at_1_diff1
value: 23.945332059908132
- type: nauc_mrr_at_1_max
value: -12.260461466152819
- type: nauc_mrr_at_1_std
value: -18.007194922921148
- type: nauc_mrr_at_20_diff1
value: 20.04819461810257
- type: nauc_mrr_at_20_max
value: -9.518368283588936
- type: nauc_mrr_at_20_std
value: -17.831608149836136
- type: nauc_mrr_at_3_diff1
value: 19.8571785245832
- type: nauc_mrr_at_3_max
value: -9.464375021240478
- type: nauc_mrr_at_3_std
value: -17.728533927330453
- type: nauc_mrr_at_5_diff1
value: 19.670313652167827
- type: nauc_mrr_at_5_max
value: -8.966372585728434
- type: nauc_mrr_at_5_std
value: -17.468955834324817
- type: nauc_ndcg_at_1000_diff1
value: 21.863049281767417
- type: nauc_ndcg_at_1000_max
value: -8.18698520924057
- type: nauc_ndcg_at_1000_std
value: -17.634483364794804
- type: nauc_ndcg_at_100_diff1
value: 21.849924385738586
- type: nauc_ndcg_at_100_max
value: -8.226437560889345
- type: nauc_ndcg_at_100_std
value: -17.774648478087002
- type: nauc_ndcg_at_10_diff1
value: 19.888395590413573
- type: nauc_ndcg_at_10_max
value: -8.968706085632382
- type: nauc_ndcg_at_10_std
value: -19.31386964628115
- type: nauc_ndcg_at_1_diff1
value: 26.23276644969203
- type: nauc_ndcg_at_1_max
value: -12.376511389571245
- type: nauc_ndcg_at_1_std
value: -18.11411715207284
- type: nauc_ndcg_at_20_diff1
value: 21.38413342416933
- type: nauc_ndcg_at_20_max
value: -7.636238194084164
- type: nauc_ndcg_at_20_std
value: -17.946390844693028
- type: nauc_ndcg_at_3_diff1
value: 21.29169165029195
- type: nauc_ndcg_at_3_max
value: -6.793840499730093
- type: nauc_ndcg_at_3_std
value: -17.52359001586737
- type: nauc_ndcg_at_5_diff1
value: 20.238297656671364
- type: nauc_ndcg_at_5_max
value: -6.424992706950072
- type: nauc_ndcg_at_5_std
value: -17.082391132291356
- type: nauc_precision_at_1000_diff1
value: -7.05195108528572
- type: nauc_precision_at_1000_max
value: 34.439879624882145
- type: nauc_precision_at_1000_std
value: 68.72436351659353
- type: nauc_precision_at_100_diff1
value: -2.769464113932605
- type: nauc_precision_at_100_max
value: 9.89562961226698
- type: nauc_precision_at_100_std
value: -0.5880967482224028
- type: nauc_precision_at_10_diff1
value: 2.1371544726832323
- type: nauc_precision_at_10_max
value: -11.93051325147756
- type: nauc_precision_at_10_std
value: -30.83144187392059
- type: nauc_precision_at_1_diff1
value: 26.23276644969203
- type: nauc_precision_at_1_max
value: -12.376511389571245
- type: nauc_precision_at_1_std
value: -18.11411715207284
- type: nauc_precision_at_20_diff1
value: 3.780146814257504
- type: nauc_precision_at_20_max
value: 17.06527540214615
- type: nauc_precision_at_20_std
value: -20.36832563035565
- type: nauc_precision_at_3_diff1
value: 17.63894384012077
- type: nauc_precision_at_3_max
value: -2.0220490624638887
- type: nauc_precision_at_3_std
value: -17.285601413493918
- type: nauc_precision_at_5_diff1
value: 12.557855071944601
- type: nauc_precision_at_5_max
value: 0.5840236463956658
- type: nauc_precision_at_5_std
value: -15.827224420217846
- type: nauc_recall_at_1000_diff1
value: -7.051951085286463
- type: nauc_recall_at_1000_max
value: 34.43987962487738
- type: nauc_recall_at_1000_std
value: 68.724363516591
- type: nauc_recall_at_100_diff1
value: -2.769464113930314
- type: nauc_recall_at_100_max
value: 9.895629612270017
- type: nauc_recall_at_100_std
value: -0.58809674821745
- type: nauc_recall_at_10_diff1
value: 2.1371544726834495
- type: nauc_recall_at_10_max
value: -11.930513251477253
- type: nauc_recall_at_10_std
value: -30.83144187392047
- type: nauc_recall_at_1_diff1
value: 26.23276644969203
- type: nauc_recall_at_1_max
value: -12.376511389571245
- type: nauc_recall_at_1_std
value: -18.11411715207284
- type: nauc_recall_at_20_diff1
value: 3.7801468142575922
- type: nauc_recall_at_20_max
value: 17.0652754021456
- type: nauc_recall_at_20_std
value: -20.36832563035559
- type: nauc_recall_at_3_diff1
value: 17.63894384012074
- type: nauc_recall_at_3_max
value: -2.02204906246383
- type: nauc_recall_at_3_std
value: -17.28560141349386
- type: nauc_recall_at_5_diff1
value: 12.55785507194463
- type: nauc_recall_at_5_max
value: 0.5840236463957296
- type: nauc_recall_at_5_std
value: -15.827224420217856
- type: ndcg_at_1
value: 40.398
- type: ndcg_at_10
value: 64.24
- type: ndcg_at_100
value: 66.631
- type: ndcg_at_1000
value: 66.65100000000001
- type: ndcg_at_20
value: 66.086
- type: ndcg_at_3
value: 55.938
- type: ndcg_at_5
value: 60.370000000000005
- type: precision_at_1
value: 40.398
- type: precision_at_10
value: 8.962
- type: precision_at_100
value: 0.9950000000000001
- type: precision_at_1000
value: 0.1
- type: precision_at_20
value: 4.836
- type: precision_at_3
value: 22.262
- type: precision_at_5
value: 15.519
- type: recall_at_1
value: 40.398
- type: recall_at_10
value: 89.616
- type: recall_at_100
value: 99.502
- type: recall_at_1000
value: 99.644
- type: recall_at_20
value: 96.72800000000001
- type: recall_at_3
value: 66.78500000000001
- type: recall_at_5
value: 77.596
task:
type: Retrieval
- dataset:
config: default
name: MTEB ArxivClusteringP2P
revision: a122ad7f3f0291bf49cc6f4d32aa80929df69d5d
split: test
type: mteb/arxiv-clustering-p2p
metrics:
- type: main_score
value: 55.1564333205451
- type: v_measure
value: 55.1564333205451
- type: v_measure_std
value: 14.696883012214512
task:
type: Clustering
- dataset:
config: default
name: MTEB ArxivClusteringS2S
revision: f910caf1a6075f7329cdf8c1a6135696f37dbd53
split: test
type: mteb/arxiv-clustering-s2s
metrics:
- type: main_score
value: 49.823698316694795
- type: v_measure
value: 49.823698316694795
- type: v_measure_std
value: 14.951660654298186
task:
type: Clustering
- dataset:
config: default
name: MTEB AskUbuntuDupQuestions
revision: 2000358ca161889fa9c082cb41daa8dcfb161a54
split: test
type: mteb/askubuntudupquestions-reranking
metrics:
- type: main_score
value: 66.15294503553424
- type: map
value: 66.15294503553424
- type: mrr
value: 78.53438420612935
- type: nAUC_map_diff1
value: 12.569697092717997
- type: nAUC_map_max
value: 21.50670312412572
- type: nAUC_map_std
value: 16.943786429229064
- type: nAUC_mrr_diff1
value: 15.590272897361238
- type: nAUC_mrr_max
value: 34.96072022474653
- type: nAUC_mrr_std
value: 21.649217605241045
task:
type: Reranking
- dataset:
config: default
name: MTEB BIOSSES
revision: d3fb88f8f02e40887cd149695127462bbcf29b4a
split: test
type: mteb/biosses-sts
metrics:
- type: cosine_pearson
value: 85.7824546319275
- type: cosine_spearman
value: 83.29587385660628
- type: euclidean_pearson
value: 84.58764190565167
- type: euclidean_spearman
value: 83.30069324352772
- type: main_score
value: 83.29587385660628
- type: manhattan_pearson
value: 84.95996839947179
- type: manhattan_spearman
value: 83.87480271054358
- type: pearson
value: 85.7824546319275
- type: spearman
value: 83.29587385660628
task:
type: STS
- dataset:
config: default
name: MTEB Banking77Classification
revision: 0fd18e25b25c072e09e0d92ab615fda904d66300
split: test
type: mteb/banking77
metrics:
- type: accuracy
value: 89.30194805194806
- type: f1
value: 89.26182507266391
- type: f1_weighted
value: 89.26182507266391
- type: main_score
value: 89.30194805194806
task:
type: Classification
- dataset:
config: default
name: MTEB BiorxivClusteringP2P
revision: 65b79d1d13f80053f67aca9498d9402c2d9f1f40
split: test
type: mteb/biorxiv-clustering-p2p
metrics:
- type: main_score
value: 50.67972171889736
- type: v_measure
value: 50.67972171889736
- type: v_measure_std
value: 0.7687409980036303
task:
type: Clustering
- dataset:
config: default
name: MTEB BiorxivClusteringS2S
revision: 258694dd0231531bc1fd9de6ceb52a0853c6d908
split: test
type: mteb/biorxiv-clustering-s2s
metrics:
- type: main_score
value: 45.80539715556144
- type: v_measure
value: 45.80539715556144
- type: v_measure_std
value: 0.9601346216579142
task:
type: Clustering
- dataset:
config: default
name: MTEB CQADupstackRetrieval
revision: 4ffe81d471b1924886b33c7567bfb200e9eec5c4
split: test
type: mteb/cqadupstack
metrics:
- type: main_score
value: 44.361250000000005
- type: map_at_1
value: 28.304499999999997
- type: map_at_10
value: 38.54841666666666
- type: map_at_100
value: 39.83141666666667
- type: map_at_1000
value: 39.944750000000006
- type: map_at_20
value: 39.25341666666667
- type: map_at_3
value: 35.406749999999995
- type: map_at_5
value: 37.15558333333333
- type: mrr_at_1
value: 34.09077232860122
- type: mrr_at_10
value: 43.15445393211421
- type: mrr_at_100
value: 43.98645286848257
- type: mrr_at_1000
value: 44.037631313469404
- type: mrr_at_20
value: 43.64045813249614
- type: mrr_at_3
value: 40.674138648480486
- type: mrr_at_5
value: 42.106251182620255
- type: nauc_map_at_1000_diff1
value: 46.250011739434996
- type: nauc_map_at_1000_max
value: 30.13664446260598
- type: nauc_map_at_1000_std
value: 5.422301791618935
- type: nauc_map_at_100_diff1
value: 46.253631351999395
- type: nauc_map_at_100_max
value: 30.12612918885181
- type: nauc_map_at_100_std
value: 5.367077019987172
- type: nauc_map_at_10_diff1
value: 46.328171341741346
- type: nauc_map_at_10_max
value: 29.80274612581464
- type: nauc_map_at_10_std
value: 4.62996685176396
- type: nauc_map_at_1_diff1
value: 51.56118117729493
- type: nauc_map_at_1_max
value: 27.94885243863768
- type: nauc_map_at_1_std
value: 1.700366508927356
- type: nauc_map_at_20_diff1
value: 46.286750260299094
- type: nauc_map_at_20_max
value: 29.979205290353278
- type: nauc_map_at_20_std
value: 5.010588412441873
- type: nauc_map_at_3_diff1
value: 47.10018183619064
- type: nauc_map_at_3_max
value: 29.062318206078753
- type: nauc_map_at_3_std
value: 3.2235696254694197
- type: nauc_map_at_5_diff1
value: 46.41971733050039
- type: nauc_map_at_5_max
value: 29.456798617695657
- type: nauc_map_at_5_std
value: 4.0921691023077145
- type: nauc_mrr_at_1000_diff1
value: 45.88888977975723
- type: nauc_mrr_at_1000_max
value: 32.162138978089544
- type: nauc_mrr_at_1000_std
value: 6.2811943424217915
- type: nauc_mrr_at_100_diff1
value: 45.87480433011124
- type: nauc_mrr_at_100_max
value: 32.16011334212834
- type: nauc_mrr_at_100_std
value: 6.2865717772421785
- type: nauc_mrr_at_10_diff1
value: 45.849652904658825
- type: nauc_mrr_at_10_max
value: 32.13847916232293
- type: nauc_mrr_at_10_std
value: 6.105718728141999
- type: nauc_mrr_at_1_diff1
value: 51.013730325062156
- type: nauc_mrr_at_1_max
value: 32.77457396492779
- type: nauc_mrr_at_1_std
value: 4.415684893471724
- type: nauc_mrr_at_20_diff1
value: 45.86663046255274
- type: nauc_mrr_at_20_max
value: 32.15219360697865
- type: nauc_mrr_at_20_std
value: 6.19603046412763
- type: nauc_mrr_at_3_diff1
value: 46.522376582423185
- type: nauc_mrr_at_3_max
value: 32.18259009733714
- type: nauc_mrr_at_3_std
value: 5.288000648220897
- type: nauc_mrr_at_5_diff1
value: 45.86611481369745
- type: nauc_mrr_at_5_max
value: 32.14261639054921
- type: nauc_mrr_at_5_std
value: 5.8811238177073735
- type: nauc_ndcg_at_1000_diff1
value: 44.5055097547565
- type: nauc_ndcg_at_1000_max
value: 31.149682057975458
- type: nauc_ndcg_at_1000_std
value: 8.157937194901333
- type: nauc_ndcg_at_100_diff1
value: 44.12398363638596
- type: nauc_ndcg_at_100_max
value: 30.878064321409994
- type: nauc_ndcg_at_100_std
value: 8.40493441452808
- type: nauc_ndcg_at_10_diff1
value: 44.200093505221474
- type: nauc_ndcg_at_10_max
value: 30.15267107733158
- type: nauc_ndcg_at_10_std
value: 6.407495361566107
- type: nauc_ndcg_at_1_diff1
value: 51.013730325062156
- type: nauc_ndcg_at_1_max
value: 32.77457396492779
- type: nauc_ndcg_at_1_std
value: 4.415684893471724
- type: nauc_ndcg_at_20_diff1
value: 44.16988321564116
- type: nauc_ndcg_at_20_max
value: 30.333532500651213
- type: nauc_ndcg_at_20_std
value: 7.10024701386895
- type: nauc_ndcg_at_3_diff1
value: 45.35982873879988
- type: nauc_ndcg_at_3_max
value: 30.288312457948702
- type: nauc_ndcg_at_3_std
value: 4.653900898293395
- type: nauc_ndcg_at_5_diff1
value: 44.324558115380185
- type: nauc_ndcg_at_5_max
value: 30.048149698941373
- type: nauc_ndcg_at_5_std
value: 5.6684459618413205
- type: nauc_precision_at_1000_diff1
value: -7.282175798304458
- type: nauc_precision_at_1000_max
value: 7.820142031765352
- type: nauc_precision_at_1000_std
value: 11.736131836431172
- type: nauc_precision_at_100_diff1
value: 1.0222940256506976
- type: nauc_precision_at_100_max
value: 16.12346497070298
- type: nauc_precision_at_100_std
value: 18.202607395247874
- type: nauc_precision_at_10_diff1
value: 18.289439185857837
- type: nauc_precision_at_10_max
value: 26.116517399154375
- type: nauc_precision_at_10_std
value: 13.921214069982302
- type: nauc_precision_at_1_diff1
value: 51.013730325062156
- type: nauc_precision_at_1_max
value: 32.77457396492779
- type: nauc_precision_at_1_std
value: 4.415684893471724
- type: nauc_precision_at_20_diff1
value: 12.365165405210886
- type: nauc_precision_at_20_max
value: 22.946297258937367
- type: nauc_precision_at_20_std
value: 16.13862870358933
- type: nauc_precision_at_3_diff1
value: 32.063423642849685
- type: nauc_precision_at_3_max
value: 30.140965811989407
- type: nauc_precision_at_3_std
value: 8.501746262550146
- type: nauc_precision_at_5_diff1
value: 24.777203357717948
- type: nauc_precision_at_5_max
value: 28.401579566848472
- type: nauc_precision_at_5_std
value: 11.643246774390914
- type: nauc_recall_at_1000_diff1
value: 30.04216463401409
- type: nauc_recall_at_1000_max
value: 34.98067760563842
- type: nauc_recall_at_1000_std
value: 48.01453905250591
- type: nauc_recall_at_100_diff1
value: 31.193415507513972
- type: nauc_recall_at_100_max
value: 28.69740149270981
- type: nauc_recall_at_100_std
value: 25.20960758920368
- type: nauc_recall_at_10_diff1
value: 36.18870823636506
- type: nauc_recall_at_10_max
value: 26.005625231341238
- type: nauc_recall_at_10_std
value: 8.891983977041376
- type: nauc_recall_at_1_diff1
value: 51.56118117729493
- type: nauc_recall_at_1_max
value: 27.94885243863768
- type: nauc_recall_at_1_std
value: 1.700366508927356
- type: nauc_recall_at_20_diff1
value: 34.93996118564803
- type: nauc_recall_at_20_max
value: 26.149961715956138
- type: nauc_recall_at_20_std
value: 12.0657502367633
- type: nauc_recall_at_3_diff1
value: 40.80743946709512
- type: nauc_recall_at_3_max
value: 26.443127773025783
- type: nauc_recall_at_3_std
value: 3.7011448604241477
- type: nauc_recall_at_5_diff1
value: 37.608535157055776
- type: nauc_recall_at_5_max
value: 26.168016189725822
- type: nauc_recall_at_5_std
value: 6.344191564595316
- type: ndcg_at_1
value: 34.09083333333333
- type: ndcg_at_10
value: 44.361250000000005
- type: ndcg_at_100
value: 49.586166666666664
- type: ndcg_at_1000
value: 51.623583333333336
- type: ndcg_at_20
value: 46.40158333333333
- type: ndcg_at_3
value: 39.27733333333333
- type: ndcg_at_5
value: 41.662333333333336
- type: precision_at_1
value: 34.09083333333333
- type: precision_at_10
value: 7.957000000000002
- type: precision_at_100
value: 1.2521666666666669
- type: precision_at_1000
value: 0.16125
- type: precision_at_20
value: 4.6755
- type: precision_at_3
value: 18.402083333333334
- type: precision_at_5
value: 13.104333333333335
- type: recall_at_1
value: 28.304499999999997
- type: recall_at_10
value: 56.80666666666667
- type: recall_at_100
value: 79.66208333333334
- type: recall_at_1000
value: 93.6455
- type: recall_at_20
value: 64.2495
- type: recall_at_3
value: 42.431333333333335
- type: recall_at_5
value: 48.665416666666665
task:
type: Retrieval
- dataset:
config: default
name: MTEB ClimateFEVER
revision: 47f2ac6acb640fc46020b02a5b59fdda04d39380
split: test
type: mteb/climate-fever
metrics:
- type: main_score
value: 43.525999999999996
- type: map_at_1
value: 19.291
- type: map_at_10
value: 33.471000000000004
- type: map_at_100
value: 35.388999999999996
- type: map_at_1000
value: 35.568
- type: map_at_20
value: 34.496
- type: map_at_3
value: 28.713
- type: map_at_5
value: 31.384
- type: mrr_at_1
value: 43.77850162866449
- type: mrr_at_10
value: 56.28576598934912
- type: mrr_at_100
value: 56.8588518168194
- type: mrr_at_1000
value: 56.878236725973544
- type: mrr_at_20
value: 56.6409328120183
- type: mrr_at_3
value: 53.56134636264935
- type: mrr_at_5
value: 55.27795874049956
- type: nauc_map_at_1000_diff1
value: 27.262513153363876
- type: nauc_map_at_1000_max
value: 40.099398684385584
- type: nauc_map_at_1000_std
value: 18.847812394005512
- type: nauc_map_at_100_diff1
value: 27.238993503030745
- type: nauc_map_at_100_max
value: 40.07730434492169
- type: nauc_map_at_100_std
value: 18.795349250833684
- type: nauc_map_at_10_diff1
value: 27.70929180366227
- type: nauc_map_at_10_max
value: 39.55987024970173
- type: nauc_map_at_10_std
value: 17.214881544648996
- type: nauc_map_at_1_diff1
value: 43.34155892182403
- type: nauc_map_at_1_max
value: 38.23324890148018
- type: nauc_map_at_1_std
value: 6.0781444393516075
- type: nauc_map_at_20_diff1
value: 27.311577477800103
- type: nauc_map_at_20_max
value: 39.624414083413456
- type: nauc_map_at_20_std
value: 18.149811054163287
- type: nauc_map_at_3_diff1
value: 30.475965062734367
- type: nauc_map_at_3_max
value: 38.49324825043695
- type: nauc_map_at_3_std
value: 13.357656038648487
- type: nauc_map_at_5_diff1
value: 28.425110095017747
- type: nauc_map_at_5_max
value: 39.017894870747796
- type: nauc_map_at_5_std
value: 15.543817194122564
- type: nauc_mrr_at_1000_diff1
value: 33.16689354701644
- type: nauc_mrr_at_1000_max
value: 41.70755363247148
- type: nauc_mrr_at_1000_std
value: 24.61667417463176
- type: nauc_mrr_at_100_diff1
value: 33.147229262917506
- type: nauc_mrr_at_100_max
value: 41.712455697170725
- type: nauc_mrr_at_100_std
value: 24.6418922043652
- type: nauc_mrr_at_10_diff1
value: 32.94185191112572
- type: nauc_mrr_at_10_max
value: 41.64272730141954
- type: nauc_mrr_at_10_std
value: 24.663391015702707
- type: nauc_mrr_at_1_diff1
value: 39.571969559016395
- type: nauc_mrr_at_1_max
value: 39.396249211263495
- type: nauc_mrr_at_1_std
value: 16.984149923258357
- type: nauc_mrr_at_20_diff1
value: 33.10040770334742
- type: nauc_mrr_at_20_max
value: 41.807565560083034
- type: nauc_mrr_at_20_std
value: 24.8064180365271
- type: nauc_mrr_at_3_diff1
value: 33.065406161485704
- type: nauc_mrr_at_3_max
value: 41.049510969934694
- type: nauc_mrr_at_3_std
value: 23.18371458928609
- type: nauc_mrr_at_5_diff1
value: 33.2389593543916
- type: nauc_mrr_at_5_max
value: 41.629486918949915
- type: nauc_mrr_at_5_std
value: 24.5777253036149
- type: nauc_ndcg_at_1000_diff1
value: 25.868840609197637
- type: nauc_ndcg_at_1000_max
value: 42.79564910784761
- type: nauc_ndcg_at_1000_std
value: 27.035091271680113
- type: nauc_ndcg_at_100_diff1
value: 25.019789319579942
- type: nauc_ndcg_at_100_max
value: 42.482345143533735
- type: nauc_ndcg_at_100_std
value: 26.76872010731345
- type: nauc_ndcg_at_10_diff1
value: 25.949464660653238
- type: nauc_ndcg_at_10_max
value: 40.79769544643906
- type: nauc_ndcg_at_10_std
value: 22.486116508973204
- type: nauc_ndcg_at_1_diff1
value: 39.571969559016395
- type: nauc_ndcg_at_1_max
value: 39.396249211263495
- type: nauc_ndcg_at_1_std
value: 16.984149923258357
- type: nauc_ndcg_at_20_diff1
value: 25.173455685962214
- type: nauc_ndcg_at_20_max
value: 40.88873540662413
- type: nauc_ndcg_at_20_std
value: 24.4451041955519
- type: nauc_ndcg_at_3_diff1
value: 28.185416070726333
- type: nauc_ndcg_at_3_max
value: 39.10600031163912
- type: nauc_ndcg_at_3_std
value: 18.42694044215541
- type: nauc_ndcg_at_5_diff1
value: 27.112647584005583
- type: nauc_ndcg_at_5_max
value: 40.154045682322526
- type: nauc_ndcg_at_5_std
value: 20.26822517176828
- type: nauc_precision_at_1000_diff1
value: -16.42087927044017
- type: nauc_precision_at_1000_max
value: 3.5326295053913
- type: nauc_precision_at_1000_std
value: 24.406810708493197
- type: nauc_precision_at_100_diff1
value: -12.17648135724982
- type: nauc_precision_at_100_max
value: 15.895489260126183
- type: nauc_precision_at_100_std
value: 32.48346122610907
- type: nauc_precision_at_10_diff1
value: -1.2493131347748072
- type: nauc_precision_at_10_max
value: 26.409459305604376
- type: nauc_precision_at_10_std
value: 31.115432019300016
- type: nauc_precision_at_1_diff1
value: 39.571969559016395
- type: nauc_precision_at_1_max
value: 39.396249211263495
- type: nauc_precision_at_1_std
value: 16.984149923258357
- type: nauc_precision_at_20_diff1
value: -6.597509397240593
- type: nauc_precision_at_20_max
value: 21.461984620659695
- type: nauc_precision_at_20_std
value: 32.9450259748889
- type: nauc_precision_at_3_diff1
value: 9.46378764865453
- type: nauc_precision_at_3_max
value: 32.03650819375425
- type: nauc_precision_at_3_std
value: 26.489382638510765
- type: nauc_precision_at_5_diff1
value: 3.5987036728169537
- type: nauc_precision_at_5_max
value: 30.633955978579703
- type: nauc_precision_at_5_std
value: 30.532430088014443
- type: nauc_recall_at_1000_diff1
value: 10.714633106872254
- type: nauc_recall_at_1000_max
value: 43.94958623961
- type: nauc_recall_at_1000_std
value: 51.78914468954123
- type: nauc_recall_at_100_diff1
value: 9.63781472255557
- type: nauc_recall_at_100_max
value: 38.50917465255336
- type: nauc_recall_at_100_std
value: 37.78623984642377
- type: nauc_recall_at_10_diff1
value: 16.480342820841688
- type: nauc_recall_at_10_max
value: 35.982566867357406
- type: nauc_recall_at_10_std
value: 23.30688188788895
- type: nauc_recall_at_1_diff1
value: 43.34155892182403
- type: nauc_recall_at_1_max
value: 38.23324890148018
- type: nauc_recall_at_1_std
value: 6.0781444393516075
- type: nauc_recall_at_20_diff1
value: 13.521048985146367
- type: nauc_recall_at_20_max
value: 34.62462209239834
- type: nauc_recall_at_20_std
value: 27.85924191501618
- type: nauc_recall_at_3_diff1
value: 23.57032748533523
- type: nauc_recall_at_3_max
value: 36.32703197635613
- type: nauc_recall_at_3_std
value: 15.730238734014337
- type: nauc_recall_at_5_diff1
value: 19.61387036368584
- type: nauc_recall_at_5_max
value: 36.22030835529556
- type: nauc_recall_at_5_std
value: 19.76310648649897
- type: ndcg_at_1
value: 43.779
- type: ndcg_at_10
value: 43.525999999999996
- type: ndcg_at_100
value: 50.138000000000005
- type: ndcg_at_1000
value: 52.991
- type: ndcg_at_20
value: 46.083
- type: ndcg_at_3
value: 38.002
- type: ndcg_at_5
value: 39.842
- type: precision_at_1
value: 43.779
- type: precision_at_10
value: 13.205
- type: precision_at_100
value: 2.051
- type: precision_at_1000
value: 0.259
- type: precision_at_20
value: 7.722999999999999
- type: precision_at_3
value: 28.903000000000002
- type: precision_at_5
value: 21.368000000000002
- type: recall_at_1
value: 19.291
- type: recall_at_10
value: 48.754
- type: recall_at_100
value: 70.97200000000001
- type: recall_at_1000
value: 86.611
- type: recall_at_20
value: 55.884
- type: recall_at_3
value: 34.101
- type: recall_at_5
value: 40.784
task:
type: Retrieval
- dataset:
config: default
name: MTEB DBPedia
revision: c0f706b76e590d620bd6618b3ca8efdd34e2d659
split: test
type: mteb/dbpedia
metrics:
- type: main_score
value: 49.884
- type: map_at_1
value: 9.913
- type: map_at_10
value: 23.186999999999998
- type: map_at_100
value: 34.207
- type: map_at_1000
value: 36.318
- type: map_at_20
value: 27.419
- type: map_at_3
value: 15.656
- type: map_at_5
value: 18.945999999999998
- type: mrr_at_1
value: 75.75
- type: mrr_at_10
value: 82.16279761904761
- type: mrr_at_100
value: 82.48445635330299
- type: mrr_at_1000
value: 82.4870246719901
- type: mrr_at_20
value: 82.36203632968338
- type: mrr_at_3
value: 81.29166666666666
- type: mrr_at_5
value: 82.02916666666667
- type: nauc_map_at_1000_diff1
value: 17.0739966990996
- type: nauc_map_at_1000_max
value: 28.440065298437133
- type: nauc_map_at_1000_std
value: 20.83498154003865
- type: nauc_map_at_100_diff1
value: 17.75982086107111
- type: nauc_map_at_100_max
value: 26.87850835673573
- type: nauc_map_at_100_std
value: 18.350282298599275
- type: nauc_map_at_10_diff1
value: 17.15984258564116
- type: nauc_map_at_10_max
value: 10.846179132675553
- type: nauc_map_at_10_std
value: -6.263534464094614
- type: nauc_map_at_1_diff1
value: 24.014897777973694
- type: nauc_map_at_1_max
value: -4.556638938723358
- type: nauc_map_at_1_std
value: -22.7844467526989
- type: nauc_map_at_20_diff1
value: 16.3179372493187
- type: nauc_map_at_20_max
value: 17.176378915498915
- type: nauc_map_at_20_std
value: 1.9378637630340372
- type: nauc_map_at_3_diff1
value: 19.12786794046792
- type: nauc_map_at_3_max
value: 0.09063919305677291
- type: nauc_map_at_3_std
value: -16.713143158330492
- type: nauc_map_at_5_diff1
value: 18.76504725420023
- type: nauc_map_at_5_max
value: 5.040867712207419
- type: nauc_map_at_5_std
value: -12.382578318931165
- type: nauc_mrr_at_1000_diff1
value: 54.61266255011247
- type: nauc_mrr_at_1000_max
value: 60.83961280977112
- type: nauc_mrr_at_1000_std
value: 32.70429260443016
- type: nauc_mrr_at_100_diff1
value: 54.61346236538542
- type: nauc_mrr_at_100_max
value: 60.8407974416647
- type: nauc_mrr_at_100_std
value: 32.69272843993462
- type: nauc_mrr_at_10_diff1
value: 54.74633685810871
- type: nauc_mrr_at_10_max
value: 61.084525933097865
- type: nauc_mrr_at_10_std
value: 33.001220210025565
- type: nauc_mrr_at_1_diff1
value: 56.12708423835806
- type: nauc_mrr_at_1_max
value: 58.9314540998289
- type: nauc_mrr_at_1_std
value: 27.39422607651012
- type: nauc_mrr_at_20_diff1
value: 54.58896150245695
- type: nauc_mrr_at_20_max
value: 60.890929983464815
- type: nauc_mrr_at_20_std
value: 32.65559641276393
- type: nauc_mrr_at_3_diff1
value: 54.38229071443791
- type: nauc_mrr_at_3_max
value: 59.987849044098596
- type: nauc_mrr_at_3_std
value: 33.439813880719974
- type: nauc_mrr_at_5_diff1
value: 54.961790262449824
- type: nauc_mrr_at_5_max
value: 61.17705173908951
- type: nauc_mrr_at_5_std
value: 33.30939850734856
- type: nauc_ndcg_at_1000_diff1
value: 29.27465932507067
- type: nauc_ndcg_at_1000_max
value: 47.952543312315214
- type: nauc_ndcg_at_1000_std
value: 36.17132236391485
- type: nauc_ndcg_at_100_diff1
value: 28.63072328980134
- type: nauc_ndcg_at_100_max
value: 41.460833419186564
- type: nauc_ndcg_at_100_std
value: 27.157100358988135
- type: nauc_ndcg_at_10_diff1
value: 23.41488013023301
- type: nauc_ndcg_at_10_max
value: 39.27798133072349
- type: nauc_ndcg_at_10_std
value: 21.979241438928312
- type: nauc_ndcg_at_1_diff1
value: 46.12120543657642
- type: nauc_ndcg_at_1_max
value: 47.28452124039853
- type: nauc_ndcg_at_1_std
value: 19.799884708952543
- type: nauc_ndcg_at_20_diff1
value: 23.627669045115574
- type: nauc_ndcg_at_20_max
value: 35.88225062457673
- type: nauc_ndcg_at_20_std
value: 18.218628030529498
- type: nauc_ndcg_at_3_diff1
value: 25.37309228946118
- type: nauc_ndcg_at_3_max
value: 40.64426332992231
- type: nauc_ndcg_at_3_std
value: 24.608330645901482
- type: nauc_ndcg_at_5_diff1
value: 24.055798594999654
- type: nauc_ndcg_at_5_max
value: 41.16180524175431
- type: nauc_ndcg_at_5_std
value: 24.048305528761315
- type: nauc_precision_at_1000_diff1
value: -18.234943251015576
- type: nauc_precision_at_1000_max
value: 0.48708502364659184
- type: nauc_precision_at_1000_std
value: 2.4473601543134027
- type: nauc_precision_at_100_diff1
value: -3.0077810947381227
- type: nauc_precision_at_100_max
value: 25.27249321108913
- type: nauc_precision_at_100_std
value: 37.36575792126928
- type: nauc_precision_at_10_diff1
value: -0.2393778190297635
- type: nauc_precision_at_10_max
value: 36.40513293547299
- type: nauc_precision_at_10_std
value: 37.4827885766009
- type: nauc_precision_at_1_diff1
value: 56.12708423835806
- type: nauc_precision_at_1_max
value: 58.9314540998289
- type: nauc_precision_at_1_std
value: 27.39422607651012
- type: nauc_precision_at_20_diff1
value: -1.2010133229402933
- type: nauc_precision_at_20_max
value: 34.117541814385966
- type: nauc_precision_at_20_std
value: 39.13273254177449
- type: nauc_precision_at_3_diff1
value: 11.757378092198486
- type: nauc_precision_at_3_max
value: 42.637962482588875
- type: nauc_precision_at_3_std
value: 37.42465077352342
- type: nauc_precision_at_5_diff1
value: 7.233177203405101
- type: nauc_precision_at_5_max
value: 43.1663582897407
- type: nauc_precision_at_5_std
value: 38.848449220750055
- type: nauc_recall_at_1000_diff1
value: 27.33938551969145
- type: nauc_recall_at_1000_max
value: 45.5614254479334
- type: nauc_recall_at_1000_std
value: 50.58528916250458
- type: nauc_recall_at_100_diff1
value: 23.610383761920097
- type: nauc_recall_at_100_max
value: 31.422168485847184
- type: nauc_recall_at_100_std
value: 25.58649926458304
- type: nauc_recall_at_10_diff1
value: 14.62495111808408
- type: nauc_recall_at_10_max
value: 7.4295041277681095
- type: nauc_recall_at_10_std
value: -9.32297089600654
- type: nauc_recall_at_1_diff1
value: 24.014897777973694
- type: nauc_recall_at_1_max
value: -4.556638938723358
- type: nauc_recall_at_1_std
value: -22.7844467526989
- type: nauc_recall_at_20_diff1
value: 14.027862330014662
- type: nauc_recall_at_20_max
value: 12.437478731690844
- type: nauc_recall_at_20_std
value: -3.0740743798103676
- type: nauc_recall_at_3_diff1
value: 16.354018356566712
- type: nauc_recall_at_3_max
value: -2.9812231240997917
- type: nauc_recall_at_3_std
value: -18.27746460743442
- type: nauc_recall_at_5_diff1
value: 16.81486583473587
- type: nauc_recall_at_5_max
value: 2.420128513974744
- type: nauc_recall_at_5_std
value: -14.441820321214108
- type: ndcg_at_1
value: 63.87500000000001
- type: ndcg_at_10
value: 49.884
- type: ndcg_at_100
value: 54.738
- type: ndcg_at_1000
value: 61.635
- type: ndcg_at_20
value: 48.894999999999996
- type: ndcg_at_3
value: 54.287
- type: ndcg_at_5
value: 52.40899999999999
- type: precision_at_1
value: 75.75
- type: precision_at_10
value: 40.9
- type: precision_at_100
value: 13.139999999999999
- type: precision_at_1000
value: 2.533
- type: precision_at_20
value: 30.8
- type: precision_at_3
value: 57.667
- type: precision_at_5
value: 51.05
- type: recall_at_1
value: 9.913
- type: recall_at_10
value: 28.591
- type: recall_at_100
value: 61.017999999999994
- type: recall_at_1000
value: 83.383
- type: recall_at_20
value: 37.834
- type: recall_at_3
value: 17.049
- type: recall_at_5
value: 21.685
task:
type: Retrieval
- dataset:
config: default
name: MTEB EmotionClassification
revision: 4f58c6b202a23cf9a4da393831edf4f9183cad37
split: test
type: mteb/emotion
metrics:
- type: accuracy
value: 78.77499999999999
- type: f1
value: 73.74058240799386
- type: f1_weighted
value: 79.78804377638227
- type: main_score
value: 78.77499999999999
task:
type: Classification
- dataset:
config: default
name: MTEB FEVER
revision: bea83ef9e8fb933d90a2f1d5515737465d613e12
split: test
type: mteb/fever
metrics:
- type: main_score
value: 90.986
- type: map_at_1
value: 81.601
- type: map_at_10
value: 88.242
- type: map_at_100
value: 88.46000000000001
- type: map_at_1000
value: 88.472
- type: map_at_20
value: 88.375
- type: map_at_3
value: 87.237
- type: map_at_5
value: 87.85300000000001
- type: mrr_at_1
value: 87.81878187818782
- type: mrr_at_10
value: 92.20301196786335
- type: mrr_at_100
value: 92.24884236673292
- type: mrr_at_1000
value: 92.2496338899362
- type: mrr_at_20
value: 92.23112073283473
- type: mrr_at_3
value: 91.77417741774165
- type: mrr_at_5
value: 92.03970397039689
- type: nauc_map_at_1000_diff1
value: 56.54670664910505
- type: nauc_map_at_1000_max
value: 33.08375749975477
- type: nauc_map_at_1000_std
value: 2.7491595418252865
- type: nauc_map_at_100_diff1
value: 56.50887688686924
- type: nauc_map_at_100_max
value: 33.075487189958494
- type: nauc_map_at_100_std
value: 2.7675869969253375
- type: nauc_map_at_10_diff1
value: 56.08080806610569
- type: nauc_map_at_10_max
value: 32.776972098819066
- type: nauc_map_at_10_std
value: 2.5904846711290097
- type: nauc_map_at_1_diff1
value: 60.645344065853145
- type: nauc_map_at_1_max
value: 31.232776777514797
- type: nauc_map_at_1_std
value: -1.1946138176109171
- type: nauc_map_at_20_diff1
value: 56.28378454162355
- type: nauc_map_at_20_max
value: 32.98207150385811
- type: nauc_map_at_20_std
value: 2.8469814040214025
- type: nauc_map_at_3_diff1
value: 55.81958007095375
- type: nauc_map_at_3_max
value: 31.602707711038313
- type: nauc_map_at_3_std
value: 0.8117019292273401
- type: nauc_map_at_5_diff1
value: 55.706025752316535
- type: nauc_map_at_5_max
value: 32.16032683604737
- type: nauc_map_at_5_std
value: 1.8853201503498669
- type: nauc_mrr_at_1000_diff1
value: 75.4997173366251
- type: nauc_mrr_at_1000_max
value: 41.49117135484116
- type: nauc_mrr_at_1000_std
value: -2.0636172883680852
- type: nauc_mrr_at_100_diff1
value: 75.50118860648519
- type: nauc_mrr_at_100_max
value: 41.49490161517194
- type: nauc_mrr_at_100_std
value: -2.057024385178682
- type: nauc_mrr_at_10_diff1
value: 75.47295153099428
- type: nauc_mrr_at_10_max
value: 41.55003304042536
- type: nauc_mrr_at_10_std
value: -2.0353663198929253
- type: nauc_mrr_at_1_diff1
value: 76.632058433229
- type: nauc_mrr_at_1_max
value: 39.754483718891656
- type: nauc_mrr_at_1_std
value: -2.962241058101701
- type: nauc_mrr_at_20_diff1
value: 75.47221882396194
- type: nauc_mrr_at_20_max
value: 41.50779280480839
- type: nauc_mrr_at_20_std
value: -1.9620212266426307
- type: nauc_mrr_at_3_diff1
value: 75.5682297897137
- type: nauc_mrr_at_3_max
value: 41.53543801506081
- type: nauc_mrr_at_3_std
value: -3.391681195945978
- type: nauc_mrr_at_5_diff1
value: 75.37562775183947
- type: nauc_mrr_at_5_max
value: 41.42028509006753
- type: nauc_mrr_at_5_std
value: -2.418698675622726
- type: nauc_ndcg_at_1000_diff1
value: 59.364557011624
- type: nauc_ndcg_at_1000_max
value: 35.4112238125149
- type: nauc_ndcg_at_1000_std
value: 3.717516193303376
- type: nauc_ndcg_at_100_diff1
value: 58.55706703023122
- type: nauc_ndcg_at_100_max
value: 35.352285999934594
- type: nauc_ndcg_at_100_std
value: 4.273437944266781
- type: nauc_ndcg_at_10_diff1
value: 56.77422701267037
- type: nauc_ndcg_at_10_max
value: 34.24909893882957
- type: nauc_ndcg_at_10_std
value: 4.178151434006727
- type: nauc_ndcg_at_1_diff1
value: 76.632058433229
- type: nauc_ndcg_at_1_max
value: 39.754483718891656
- type: nauc_ndcg_at_1_std
value: -2.962241058101701
- type: nauc_ndcg_at_20_diff1
value: 57.27343398231262
- type: nauc_ndcg_at_20_max
value: 34.7416626740278
- type: nauc_ndcg_at_20_std
value: 4.955858766014002
- type: nauc_ndcg_at_3_diff1
value: 57.69267803121093
- type: nauc_ndcg_at_3_max
value: 33.13744317023105
- type: nauc_ndcg_at_3_std
value: 0.40380284030057023
- type: nauc_ndcg_at_5_diff1
value: 56.57461019113917
- type: nauc_ndcg_at_5_max
value: 33.244657840804386
- type: nauc_ndcg_at_5_std
value: 2.5121440827702046
- type: nauc_precision_at_1000_diff1
value: -14.54492513449718
- type: nauc_precision_at_1000_max
value: -5.94552147573623
- type: nauc_precision_at_1000_std
value: 1.2446209816057374
- type: nauc_precision_at_100_diff1
value: -15.452676132568344
- type: nauc_precision_at_100_max
value: -3.760241749847617
- type: nauc_precision_at_100_std
value: 4.623534605290865
- type: nauc_precision_at_10_diff1
value: -12.712908026086176
- type: nauc_precision_at_10_max
value: 0.45241316994816805
- type: nauc_precision_at_10_std
value: 7.849478570138391
- type: nauc_precision_at_1_diff1
value: 76.632058433229
- type: nauc_precision_at_1_max
value: 39.754483718891656
- type: nauc_precision_at_1_std
value: -2.962241058101701
- type: nauc_precision_at_20_diff1
value: -14.514618673172041
- type: nauc_precision_at_20_max
value: -1.113635490621818
- type: nauc_precision_at_20_std
value: 8.599811730457576
- type: nauc_precision_at_3_diff1
value: 6.1367799850003815
- type: nauc_precision_at_3_max
value: 8.466271950897857
- type: nauc_precision_at_3_std
value: 1.7458051543195068
- type: nauc_precision_at_5_diff1
value: -5.804548945783379
- type: nauc_precision_at_5_max
value: 3.4060251839074818
- type: nauc_precision_at_5_std
value: 5.583410511782371
- type: nauc_recall_at_1000_diff1
value: 19.329432953574095
- type: nauc_recall_at_1000_max
value: 43.260442595158736
- type: nauc_recall_at_1000_std
value: 53.89644660661804
- type: nauc_recall_at_100_diff1
value: 21.265326296051235
- type: nauc_recall_at_100_max
value: 38.573000195373695
- type: nauc_recall_at_100_std
value: 42.169391082152785
- type: nauc_recall_at_10_diff1
value: 29.785129558987432
- type: nauc_recall_at_10_max
value: 28.379657867558034
- type: nauc_recall_at_10_std
value: 21.132574624091973
- type: nauc_recall_at_1_diff1
value: 60.645344065853145
- type: nauc_recall_at_1_max
value: 31.232776777514797
- type: nauc_recall_at_1_std
value: -1.1946138176109171
- type: nauc_recall_at_20_diff1
value: 25.88845612373954
- type: nauc_recall_at_20_max
value: 30.24785945821152
- type: nauc_recall_at_20_std
value: 31.73911437468067
- type: nauc_recall_at_3_diff1
value: 42.2968464797395
- type: nauc_recall_at_3_max
value: 26.494318009870018
- type: nauc_recall_at_3_std
value: 2.6045977160467544
- type: nauc_recall_at_5_diff1
value: 35.81340094401374
- type: nauc_recall_at_5_max
value: 25.91082947510634
- type: nauc_recall_at_5_std
value: 9.759404930864779
- type: ndcg_at_1
value: 87.819
- type: ndcg_at_10
value: 90.986
- type: ndcg_at_100
value: 91.69
- type: ndcg_at_1000
value: 91.863
- type: ndcg_at_20
value: 91.293
- type: ndcg_at_3
value: 89.621
- type: ndcg_at_5
value: 90.333
- type: precision_at_1
value: 87.819
- type: precision_at_10
value: 10.753
- type: precision_at_100
value: 1.138
- type: precision_at_1000
value: 0.117
- type: precision_at_20
value: 5.4879999999999995
- type: precision_at_3
value: 33.703
- type: precision_at_5
value: 20.831
- type: recall_at_1
value: 81.601
- type: recall_at_10
value: 95.44200000000001
- type: recall_at_100
value: 98.14399999999999
- type: recall_at_1000
value: 99.157
- type: recall_at_20
value: 96.43
- type: recall_at_3
value: 91.729
- type: recall_at_5
value: 93.552
task:
type: Retrieval
- dataset:
config: default
name: MTEB FiQA2018
revision: 27a168819829fe9bcd655c2df245fb19452e8e06
split: test
type: mteb/fiqa
metrics:
- type: main_score
value: 56.056
- type: map_at_1
value: 28.666000000000004
- type: map_at_10
value: 47.437000000000005
- type: map_at_100
value: 49.537
- type: map_at_1000
value: 49.665
- type: map_at_20
value: 48.618
- type: map_at_3
value: 41.355
- type: map_at_5
value: 44.525
- type: mrr_at_1
value: 55.55555555555556
- type: mrr_at_10
value: 63.705173427395614
- type: mrr_at_100
value: 64.25449940779741
- type: mrr_at_1000
value: 64.27635581092147
- type: mrr_at_20
value: 64.03796029079103
- type: mrr_at_3
value: 61.49691358024688
- type: mrr_at_5
value: 62.73148148148143
- type: nauc_map_at_1000_diff1
value: 43.24282910397747
- type: nauc_map_at_1000_max
value: 28.506093180265644
- type: nauc_map_at_1000_std
value: -13.040508386155054
- type: nauc_map_at_100_diff1
value: 43.23650442904607
- type: nauc_map_at_100_max
value: 28.470565635459156
- type: nauc_map_at_100_std
value: -12.988098780714935
- type: nauc_map_at_10_diff1
value: 43.393840733087686
- type: nauc_map_at_10_max
value: 26.637302062720153
- type: nauc_map_at_10_std
value: -14.47500292113762
- type: nauc_map_at_1_diff1
value: 47.705150227211725
- type: nauc_map_at_1_max
value: 15.354189686550129
- type: nauc_map_at_1_std
value: -14.559819859039067
- type: nauc_map_at_20_diff1
value: 43.14121075706104
- type: nauc_map_at_20_max
value: 27.811170590408395
- type: nauc_map_at_20_std
value: -13.459413585283583
- type: nauc_map_at_3_diff1
value: 44.33938667720801
- type: nauc_map_at_3_max
value: 21.785619884549398
- type: nauc_map_at_3_std
value: -15.569980103071593
- type: nauc_map_at_5_diff1
value: 43.39280905665027
- type: nauc_map_at_5_max
value: 25.021492190645017
- type: nauc_map_at_5_std
value: -14.48856622187443
- type: nauc_mrr_at_1000_diff1
value: 52.971563939946286
- type: nauc_mrr_at_1000_max
value: 38.88019486172324
- type: nauc_mrr_at_1000_std
value: -12.412991642381616
- type: nauc_mrr_at_100_diff1
value: 52.978468139876945
- type: nauc_mrr_at_100_max
value: 38.89751787948751
- type: nauc_mrr_at_100_std
value: -12.3677876252269
- type: nauc_mrr_at_10_diff1
value: 52.78507148048174
- type: nauc_mrr_at_10_max
value: 38.55079809310022
- type: nauc_mrr_at_10_std
value: -12.944127025078755
- type: nauc_mrr_at_1_diff1
value: 55.52626805861546
- type: nauc_mrr_at_1_max
value: 40.49306809164979
- type: nauc_mrr_at_1_std
value: -12.886607701317681
- type: nauc_mrr_at_20_diff1
value: 52.9592152665678
- type: nauc_mrr_at_20_max
value: 38.88514014589964
- type: nauc_mrr_at_20_std
value: -12.434464359819444
- type: nauc_mrr_at_3_diff1
value: 52.73696844091174
- type: nauc_mrr_at_3_max
value: 38.61018727252859
- type: nauc_mrr_at_3_std
value: -13.123989867364166
- type: nauc_mrr_at_5_diff1
value: 53.037110010188
- type: nauc_mrr_at_5_max
value: 38.44770729849151
- type: nauc_mrr_at_5_std
value: -13.49318771828972
- type: nauc_ndcg_at_1000_diff1
value: 44.73813840091289
- type: nauc_ndcg_at_1000_max
value: 33.70113904685389
- type: nauc_ndcg_at_1000_std
value: -10.328687058192742
- type: nauc_ndcg_at_100_diff1
value: 44.595174119928835
- type: nauc_ndcg_at_100_max
value: 33.4788285112467
- type: nauc_ndcg_at_100_std
value: -8.695355259716946
- type: nauc_ndcg_at_10_diff1
value: 44.39837225263
- type: nauc_ndcg_at_10_max
value: 29.188289725593393
- type: nauc_ndcg_at_10_std
value: -13.67608323673103
- type: nauc_ndcg_at_1_diff1
value: 55.52626805861546
- type: nauc_ndcg_at_1_max
value: 40.49306809164979
- type: nauc_ndcg_at_1_std
value: -12.886607701317681
- type: nauc_ndcg_at_20_diff1
value: 44.24661739902305
- type: nauc_ndcg_at_20_max
value: 31.667868318249965
- type: nauc_ndcg_at_20_std
value: -10.65470780066342
- type: nauc_ndcg_at_3_diff1
value: 43.39857166975522
- type: nauc_ndcg_at_3_max
value: 31.764668313577495
- type: nauc_ndcg_at_3_std
value: -14.494866954678152
- type: nauc_ndcg_at_5_diff1
value: 43.16976647347281
- type: nauc_ndcg_at_5_max
value: 29.878329062643143
- type: nauc_ndcg_at_5_std
value: -13.987689089179739
- type: nauc_precision_at_1000_diff1
value: -9.807973252625484
- type: nauc_precision_at_1000_max
value: 26.6279603849494
- type: nauc_precision_at_1000_std
value: 7.113187103520632
- type: nauc_precision_at_100_diff1
value: -4.777149603323976
- type: nauc_precision_at_100_max
value: 31.03410463692187
- type: nauc_precision_at_100_std
value: 10.463144150275435
- type: nauc_precision_at_10_diff1
value: 8.691528703215962
- type: nauc_precision_at_10_max
value: 33.329579434123374
- type: nauc_precision_at_10_std
value: -0.8002015226329403
- type: nauc_precision_at_1_diff1
value: 55.52626805861546
- type: nauc_precision_at_1_max
value: 40.49306809164979
- type: nauc_precision_at_1_std
value: -12.886607701317681
- type: nauc_precision_at_20_diff1
value: 3.4564653474184284
- type: nauc_precision_at_20_max
value: 34.401070158471136
- type: nauc_precision_at_20_std
value: 5.813431200164549
- type: nauc_precision_at_3_diff1
value: 22.463219705462187
- type: nauc_precision_at_3_max
value: 34.77413976546924
- type: nauc_precision_at_3_std
value: -7.083890789741479
- type: nauc_precision_at_5_diff1
value: 14.011006004883154
- type: nauc_precision_at_5_max
value: 35.73655466853702
- type: nauc_precision_at_5_std
value: -2.8395172077771598
- type: nauc_recall_at_1000_diff1
value: 16.478046357391555
- type: nauc_recall_at_1000_max
value: 43.231704288282344
- type: nauc_recall_at_1000_std
value: 38.430684937573645
- type: nauc_recall_at_100_diff1
value: 30.764718344602436
- type: nauc_recall_at_100_max
value: 31.769050487166655
- type: nauc_recall_at_100_std
value: 23.48468311677149
- type: nauc_recall_at_10_diff1
value: 34.47339565324045
- type: nauc_recall_at_10_max
value: 19.054212335800454
- type: nauc_recall_at_10_std
value: -11.039734015330437
- type: nauc_recall_at_1_diff1
value: 47.705150227211725
- type: nauc_recall_at_1_max
value: 15.354189686550129
- type: nauc_recall_at_1_std
value: -14.559819859039067
- type: nauc_recall_at_20_diff1
value: 32.1011474016873
- type: nauc_recall_at_20_max
value: 25.546372988304423
- type: nauc_recall_at_20_std
value: -0.007233471152482897
- type: nauc_recall_at_3_diff1
value: 37.5708138019065
- type: nauc_recall_at_3_max
value: 16.66410785756736
- type: nauc_recall_at_3_std
value: -15.404817020108966
- type: nauc_recall_at_5_diff1
value: 35.714519648479595
- type: nauc_recall_at_5_max
value: 19.02075233009296
- type: nauc_recall_at_5_std
value: -13.180963359760725
- type: ndcg_at_1
value: 55.556000000000004
- type: ndcg_at_10
value: 56.056
- type: ndcg_at_100
value: 62.44
- type: ndcg_at_1000
value: 64.263
- type: ndcg_at_20
value: 58.638999999999996
- type: ndcg_at_3
value: 51.722
- type: ndcg_at_5
value: 52.701
- type: precision_at_1
value: 55.556000000000004
- type: precision_at_10
value: 15.679000000000002
- type: precision_at_100
value: 2.252
- type: precision_at_1000
value: 0.257
- type: precision_at_20
value: 9.02
- type: precision_at_3
value: 34.619
- type: precision_at_5
value: 25.093
- type: recall_at_1
value: 28.666000000000004
- type: recall_at_10
value: 63.717999999999996
- type: recall_at_100
value: 86.938
- type: recall_at_1000
value: 97.603
- type: recall_at_20
value: 71.649
- type: recall_at_3
value: 46.663
- type: recall_at_5
value: 53.313
task:
type: Retrieval
- dataset:
config: default
name: MTEB HotpotQA
revision: ab518f4d6fcca38d87c25209f94beba119d02014
split: test
type: mteb/hotpotqa
metrics:
- type: main_score
value: 71.74199999999999
- type: map_at_1
value: 41.729
- type: map_at_10
value: 63.168
- type: map_at_100
value: 64.132
- type: map_at_1000
value: 64.199
- type: map_at_20
value: 63.736000000000004
- type: map_at_3
value: 59.826
- type: map_at_5
value: 61.882000000000005
- type: mrr_at_1
value: 83.45712356515868
- type: mrr_at_10
value: 87.850342432719
- type: mrr_at_100
value: 88.0016320691113
- type: mrr_at_1000
value: 88.00576596968136
- type: mrr_at_20
value: 87.94463253190389
- type: mrr_at_3
value: 87.13706954760278
- type: mrr_at_5
value: 87.59419311276136
- type: nauc_map_at_1000_diff1
value: 13.635446621095054
- type: nauc_map_at_1000_max
value: 18.670632529445633
- type: nauc_map_at_1000_std
value: 10.444842636150575
- type: nauc_map_at_100_diff1
value: 13.599262398010783
- type: nauc_map_at_100_max
value: 18.636389405484806
- type: nauc_map_at_100_std
value: 10.460027483576043
- type: nauc_map_at_10_diff1
value: 13.235053919323942
- type: nauc_map_at_10_max
value: 18.252140477080047
- type: nauc_map_at_10_std
value: 9.9075337042203
- type: nauc_map_at_1_diff1
value: 76.51940497836482
- type: nauc_map_at_1_max
value: 51.251419487235474
- type: nauc_map_at_1_std
value: 0.16714896857146574
- type: nauc_map_at_20_diff1
value: 13.4178245722222
- type: nauc_map_at_20_max
value: 18.40988771210718
- type: nauc_map_at_20_std
value: 10.216685163366282
- type: nauc_map_at_3_diff1
value: 13.38370761663418
- type: nauc_map_at_3_max
value: 17.760962555456537
- type: nauc_map_at_3_std
value: 7.15741965624388
- type: nauc_map_at_5_diff1
value: 13.138133309724855
- type: nauc_map_at_5_max
value: 17.871761295251044
- type: nauc_map_at_5_std
value: 8.475147426940074
- type: nauc_mrr_at_1000_diff1
value: 75.82650818891959
- type: nauc_mrr_at_1000_max
value: 53.6736100668434
- type: nauc_mrr_at_1000_std
value: 1.8025016349213916
- type: nauc_mrr_at_100_diff1
value: 75.82530574210111
- type: nauc_mrr_at_100_max
value: 53.68067545829002
- type: nauc_mrr_at_100_std
value: 1.8147470536495791
- type: nauc_mrr_at_10_diff1
value: 75.8330135686799
- type: nauc_mrr_at_10_max
value: 53.78626885349077
- type: nauc_mrr_at_10_std
value: 1.7975782717226636
- type: nauc_mrr_at_1_diff1
value: 76.51940497836482
- type: nauc_mrr_at_1_max
value: 51.251419487235474
- type: nauc_mrr_at_1_std
value: 0.16714896857146574
- type: nauc_mrr_at_20_diff1
value: 75.82783382464166
- type: nauc_mrr_at_20_max
value: 53.68364567043885
- type: nauc_mrr_at_20_std
value: 1.742037904463963
- type: nauc_mrr_at_3_diff1
value: 75.6944609768663
- type: nauc_mrr_at_3_max
value: 53.803941340341666
- type: nauc_mrr_at_3_std
value: 1.1849945458077804
- type: nauc_mrr_at_5_diff1
value: 75.73006960604903
- type: nauc_mrr_at_5_max
value: 53.62223096420106
- type: nauc_mrr_at_5_std
value: 1.6144067563410909
- type: nauc_ndcg_at_1000_diff1
value: 21.58025241642726
- type: nauc_ndcg_at_1000_max
value: 24.675747527001153
- type: nauc_ndcg_at_1000_std
value: 13.075943547492718
- type: nauc_ndcg_at_100_diff1
value: 20.30260137544846
- type: nauc_ndcg_at_100_max
value: 23.757528813872018
- type: nauc_ndcg_at_100_std
value: 13.648994687574062
- type: nauc_ndcg_at_10_diff1
value: 18.995052360997818
- type: nauc_ndcg_at_10_max
value: 22.254260808196037
- type: nauc_ndcg_at_10_std
value: 11.27212390633054
- type: nauc_ndcg_at_1_diff1
value: 76.51940497836482
- type: nauc_ndcg_at_1_max
value: 51.251419487235474
- type: nauc_ndcg_at_1_std
value: 0.16714896857146574
- type: nauc_ndcg_at_20_diff1
value: 19.333742380695757
- type: nauc_ndcg_at_20_max
value: 22.527779834633364
- type: nauc_ndcg_at_20_std
value: 12.161009000707917
- type: nauc_ndcg_at_3_diff1
value: 20.013329040965534
- type: nauc_ndcg_at_3_max
value: 21.99692460311921
- type: nauc_ndcg_at_3_std
value: 6.8076290638386165
- type: nauc_ndcg_at_5_diff1
value: 19.08226315942471
- type: nauc_ndcg_at_5_max
value: 21.71185964294168
- type: nauc_ndcg_at_5_std
value: 8.671911269518214
- type: nauc_precision_at_1000_diff1
value: 2.4462475489446764
- type: nauc_precision_at_1000_max
value: 29.145662064268578
- type: nauc_precision_at_1000_std
value: 49.20704909525856
- type: nauc_precision_at_100_diff1
value: 0.11271196725540299
- type: nauc_precision_at_100_max
value: 17.37584606388067
- type: nauc_precision_at_100_std
value: 34.66099346244071
- type: nauc_precision_at_10_diff1
value: 2.9923183951227825
- type: nauc_precision_at_10_max
value: 14.261884731124264
- type: nauc_precision_at_10_std
value: 18.084188795498378
- type: nauc_precision_at_1_diff1
value: 76.51940497836482
- type: nauc_precision_at_1_max
value: 51.251419487235474
- type: nauc_precision_at_1_std
value: 0.16714896857146574
- type: nauc_precision_at_20_diff1
value: 1.9180293008303761
- type: nauc_precision_at_20_max
value: 13.832269193468512
- type: nauc_precision_at_20_std
value: 21.65284406055607
- type: nauc_precision_at_3_diff1
value: 7.226609484731811
- type: nauc_precision_at_3_max
value: 15.162908526977272
- type: nauc_precision_at_3_std
value: 8.451859972962776
- type: nauc_precision_at_5_diff1
value: 4.705236845538159
- type: nauc_precision_at_5_max
value: 14.022910843582666
- type: nauc_precision_at_5_std
value: 11.777269322821605
- type: nauc_recall_at_1000_diff1
value: 2.446247548945172
- type: nauc_recall_at_1000_max
value: 29.14566206426889
- type: nauc_recall_at_1000_std
value: 49.20704909525879
- type: nauc_recall_at_100_diff1
value: 0.1127119672553316
- type: nauc_recall_at_100_max
value: 17.37584606388062
- type: nauc_recall_at_100_std
value: 34.660993462440686
- type: nauc_recall_at_10_diff1
value: 2.9923183951227927
- type: nauc_recall_at_10_max
value: 14.261884731124299
- type: nauc_recall_at_10_std
value: 18.08418879549837
- type: nauc_recall_at_1_diff1
value: 76.51940497836482
- type: nauc_recall_at_1_max
value: 51.251419487235474
- type: nauc_recall_at_1_std
value: 0.16714896857146574
- type: nauc_recall_at_20_diff1
value: 1.918029300830432
- type: nauc_recall_at_20_max
value: 13.832269193468566
- type: nauc_recall_at_20_std
value: 21.65284406055605
- type: nauc_recall_at_3_diff1
value: 7.226609484731802
- type: nauc_recall_at_3_max
value: 15.162908526977182
- type: nauc_recall_at_3_std
value: 8.451859972962634
- type: nauc_recall_at_5_diff1
value: 4.705236845538197
- type: nauc_recall_at_5_max
value: 14.02291084358265
- type: nauc_recall_at_5_std
value: 11.777269322821638
- type: ndcg_at_1
value: 83.45700000000001
- type: ndcg_at_10
value: 71.74199999999999
- type: ndcg_at_100
value: 75.008
- type: ndcg_at_1000
value: 76.242
- type: ndcg_at_20
value: 73.114
- type: ndcg_at_3
value: 67.128
- type: ndcg_at_5
value: 69.645
- type: precision_at_1
value: 83.45700000000001
- type: precision_at_10
value: 14.747
- type: precision_at_100
value: 1.73
- type: precision_at_1000
value: 0.189
- type: precision_at_20
value: 7.8149999999999995
- type: precision_at_3
value: 42.323
- type: precision_at_5
value: 27.381
- type: recall_at_1
value: 41.729
- type: recall_at_10
value: 73.734
- type: recall_at_100
value: 86.502
- type: recall_at_1000
value: 94.60499999999999
- type: recall_at_20
value: 78.14999999999999
- type: recall_at_3
value: 63.483999999999995
- type: recall_at_5
value: 68.45400000000001
task:
type: Retrieval
- dataset:
config: default
name: MTEB ImdbClassification
revision: 3d86128a09e091d6018b6d26cad27f2739fc2db7
split: test
type: mteb/imdb
metrics:
- type: accuracy
value: 96.4904
- type: ap
value: 94.85481918794709
- type: ap_weighted
value: 94.85481918794709
- type: f1
value: 96.4898592305707
- type: f1_weighted
value: 96.4898592305707
- type: main_score
value: 96.4904
task:
type: Classification
- dataset:
config: default
name: MTEB MSMARCO
revision: c5a29a104738b98a9e76336939199e264163d4a0
split: dev
type: mteb/msmarco
metrics:
- type: main_score
value: 43.692
- type: map_at_1
value: 23.751
- type: map_at_10
value: 36.553999999999995
- type: map_at_100
value: 37.721
- type: map_at_1000
value: 37.763999999999996
- type: map_at_20
value: 37.289
- type: map_at_3
value: 32.643
- type: map_at_5
value: 34.851
- type: mrr_at_1
value: 24.455587392550143
- type: mrr_at_10
value: 37.18388706963206
- type: mrr_at_100
value: 38.28330737932916
- type: mrr_at_1000
value: 38.32054399710817
- type: mrr_at_20
value: 37.8818001216278
- type: mrr_at_3
value: 33.35721107927405
- type: mrr_at_5
value: 35.52483285577843
- type: nauc_map_at_1000_diff1
value: 36.3576177260684
- type: nauc_map_at_1000_max
value: 7.854511605962703
- type: nauc_map_at_1000_std
value: -17.701121059746878
- type: nauc_map_at_100_diff1
value: 36.356075649230505
- type: nauc_map_at_100_max
value: 7.862168042999533
- type: nauc_map_at_100_std
value: -17.670102459097233
- type: nauc_map_at_10_diff1
value: 36.22122978875574
- type: nauc_map_at_10_max
value: 7.80848606967416
- type: nauc_map_at_10_std
value: -18.3265151386167
- type: nauc_map_at_1_diff1
value: 39.28605466408357
- type: nauc_map_at_1_max
value: 6.20202977590459
- type: nauc_map_at_1_std
value: -15.734334090045026
- type: nauc_map_at_20_diff1
value: 36.33637880909657
- type: nauc_map_at_20_max
value: 7.843437969476022
- type: nauc_map_at_20_std
value: -17.917533363025996
- type: nauc_map_at_3_diff1
value: 36.24864976076741
- type: nauc_map_at_3_max
value: 7.420345251835957
- type: nauc_map_at_3_std
value: -18.71678497722944
- type: nauc_map_at_5_diff1
value: 36.0789619291824
- type: nauc_map_at_5_max
value: 7.7314285669514495
- type: nauc_map_at_5_std
value: -18.748688764538706
- type: nauc_mrr_at_1000_diff1
value: 36.23912675623378
- type: nauc_mrr_at_1000_max
value: 7.690553436255147
- type: nauc_mrr_at_1000_std
value: -17.609526070212304
- type: nauc_mrr_at_100_diff1
value: 36.23782651189002
- type: nauc_mrr_at_100_max
value: 7.70075095171647
- type: nauc_mrr_at_100_std
value: -17.575714144960184
- type: nauc_mrr_at_10_diff1
value: 36.125229472534215
- type: nauc_mrr_at_10_max
value: 7.635472248755658
- type: nauc_mrr_at_10_std
value: -18.208166616511086
- type: nauc_mrr_at_1_diff1
value: 39.20986875554532
- type: nauc_mrr_at_1_max
value: 6.062668487561363
- type: nauc_mrr_at_1_std
value: -16.04130340817602
- type: nauc_mrr_at_20_diff1
value: 36.21207088739667
- type: nauc_mrr_at_20_max
value: 7.699610250145951
- type: nauc_mrr_at_20_std
value: -17.778245221724028
- type: nauc_mrr_at_3_diff1
value: 36.03957583885305
- type: nauc_mrr_at_3_max
value: 7.225515576504581
- type: nauc_mrr_at_3_std
value: -18.74478742943741
- type: nauc_mrr_at_5_diff1
value: 35.969152496648974
- type: nauc_mrr_at_5_max
value: 7.584059789018233
- type: nauc_mrr_at_5_std
value: -18.569374723129332
- type: nauc_ndcg_at_1000_diff1
value: 35.894655529841806
- type: nauc_ndcg_at_1000_max
value: 8.579327424366236
- type: nauc_ndcg_at_1000_std
value: -16.359677367747896
- type: nauc_ndcg_at_100_diff1
value: 35.89861902483983
- type: nauc_ndcg_at_100_max
value: 8.830873623962242
- type: nauc_ndcg_at_100_std
value: -15.173125564722978
- type: nauc_ndcg_at_10_diff1
value: 35.36499811105169
- type: nauc_ndcg_at_10_max
value: 8.449267180956992
- type: nauc_ndcg_at_10_std
value: -18.41978802362402
- type: nauc_ndcg_at_1_diff1
value: 39.15422481210622
- type: nauc_ndcg_at_1_max
value: 6.055515791928331
- type: nauc_ndcg_at_1_std
value: -16.042779610876252
- type: nauc_ndcg_at_20_diff1
value: 35.73402868264468
- type: nauc_ndcg_at_20_max
value: 8.695705518210847
- type: nauc_ndcg_at_20_std
value: -16.7735829470466
- type: nauc_ndcg_at_3_diff1
value: 35.31358242856231
- type: nauc_ndcg_at_3_max
value: 7.645692789058997
- type: nauc_ndcg_at_3_std
value: -19.460003734786874
- type: nauc_ndcg_at_5_diff1
value: 35.05216588927143
- type: nauc_ndcg_at_5_max
value: 8.216690520604715
- type: nauc_ndcg_at_5_std
value: -19.3982054492159
- type: nauc_precision_at_1000_diff1
value: -4.440002625111349
- type: nauc_precision_at_1000_max
value: 7.886988951901723
- type: nauc_precision_at_1000_std
value: 9.88111187048247
- type: nauc_precision_at_100_diff1
value: 15.728286119463325
- type: nauc_precision_at_100_max
value: 13.218650824470654
- type: nauc_precision_at_100_std
value: 16.113245895522553
- type: nauc_precision_at_10_diff1
value: 29.51218489610567
- type: nauc_precision_at_10_max
value: 10.197432401942912
- type: nauc_precision_at_10_std
value: -16.950603431359493
- type: nauc_precision_at_1_diff1
value: 39.15422481210622
- type: nauc_precision_at_1_max
value: 6.055515791928331
- type: nauc_precision_at_1_std
value: -16.042779610876252
- type: nauc_precision_at_20_diff1
value: 27.825993070397338
- type: nauc_precision_at_20_max
value: 11.437632287846007
- type: nauc_precision_at_20_std
value: -7.450353566405601
- type: nauc_precision_at_3_diff1
value: 32.14135556796588
- type: nauc_precision_at_3_max
value: 7.989252443574163
- type: nauc_precision_at_3_std
value: -21.566254595671055
- type: nauc_precision_at_5_diff1
value: 30.68778685307082
- type: nauc_precision_at_5_max
value: 9.332160758499892
- type: nauc_precision_at_5_std
value: -20.928554713448914
- type: nauc_recall_at_1000_diff1
value: 25.00810478716878
- type: nauc_recall_at_1000_max
value: 46.518165765201644
- type: nauc_recall_at_1000_std
value: 61.4734635576085
- type: nauc_recall_at_100_diff1
value: 33.895581318261726
- type: nauc_recall_at_100_max
value: 20.10706035872801
- type: nauc_recall_at_100_std
value: 24.204226584457047
- type: nauc_recall_at_10_diff1
value: 32.363127359576296
- type: nauc_recall_at_10_max
value: 10.729923804989545
- type: nauc_recall_at_10_std
value: -18.1335370184202
- type: nauc_recall_at_1_diff1
value: 39.28605466408357
- type: nauc_recall_at_1_max
value: 6.20202977590459
- type: nauc_recall_at_1_std
value: -15.734334090045026
- type: nauc_recall_at_20_diff1
value: 33.47804003169795
- type: nauc_recall_at_20_max
value: 12.781494765263382
- type: nauc_recall_at_20_std
value: -9.263970132202658
- type: nauc_recall_at_3_diff1
value: 32.71001429428999
- type: nauc_recall_at_3_max
value: 8.353439197382693
- type: nauc_recall_at_3_std
value: -21.235097744366954
- type: nauc_recall_at_5_diff1
value: 31.87451464963415
- type: nauc_recall_at_5_max
value: 9.635051450907305
- type: nauc_recall_at_5_std
value: -21.113235357132794
- type: ndcg_at_1
value: 24.47
- type: ndcg_at_10
value: 43.692
- type: ndcg_at_100
value: 49.211
- type: ndcg_at_1000
value: 50.244
- type: ndcg_at_20
value: 46.278000000000006
- type: ndcg_at_3
value: 35.719
- type: ndcg_at_5
value: 39.652
- type: precision_at_1
value: 24.47
- type: precision_at_10
value: 6.857
- type: precision_at_100
value: 0.9610000000000001
- type: precision_at_1000
value: 0.105
- type: precision_at_20
value: 3.968
- type: precision_at_3
value: 15.181000000000001
- type: precision_at_5
value: 11.117
- type: recall_at_1
value: 23.751
- type: recall_at_10
value: 65.64
- type: recall_at_100
value: 90.967
- type: recall_at_1000
value: 98.738
- type: recall_at_20
value: 75.639
- type: recall_at_3
value: 43.927
- type: recall_at_5
value: 53.366
task:
type: Retrieval
- dataset:
config: en
name: MTEB MTOPDomainClassification (en)
revision: d80d48c1eb48d3562165c59d59d0034df9fff0bf
split: test
type: mteb/mtop_domain
metrics:
- type: accuracy
value: 98.82580939352485
- type: f1
value: 98.75201754333801
- type: f1_weighted
value: 98.82795205108245
- type: main_score
value: 98.82580939352485
task:
type: Classification
- dataset:
config: en
name: MTEB MTOPIntentClassification (en)
revision: ae001d0e6b1228650b7bd1c2c65fb50ad11a8aba
split: test
type: mteb/mtop_intent
metrics:
- type: accuracy
value: 92.29822161422709
- type: f1
value: 77.75210224871594
- type: f1_weighted
value: 93.58661422540348
- type: main_score
value: 92.29822161422709
task:
type: Classification
- dataset:
config: en
name: MTEB MassiveIntentClassification (en)
revision: 4672e20407010da34463acc759c162ca9734bca6
split: test
type: mteb/amazon_massive_intent
metrics:
- type: accuracy
value: 85.17484868863484
- type: f1
value: 81.94484244487094
- type: f1_weighted
value: 85.21022593423332
- type: main_score
value: 85.17484868863484
task:
type: Classification
- dataset:
config: en
name: MTEB MassiveScenarioClassification (en)
revision: fad2c6e8459f9e1c45d9315f4953d921437d70f8
split: test
type: mteb/amazon_massive_scenario
metrics:
- type: accuracy
value: 89.61667787491594
- type: f1
value: 89.02701927621264
- type: f1_weighted
value: 89.56306982022801
- type: main_score
value: 89.61667787491594
task:
type: Classification
- dataset:
config: default
name: MTEB MedrxivClusteringP2P
revision: e7a26af6f3ae46b30dde8737f02c07b1505bcc73
split: test
type: mteb/medrxiv-clustering-p2p
metrics:
- type: main_score
value: 46.318282423948574
- type: v_measure
value: 46.318282423948574
- type: v_measure_std
value: 0.9729055662461538
task:
type: Clustering
- dataset:
config: default
name: MTEB MedrxivClusteringS2S
revision: 35191c8c0dca72d8ff3efcd72aa802307d469663
split: test
type: mteb/medrxiv-clustering-s2s
metrics:
- type: main_score
value: 44.29033625273981
- type: v_measure
value: 44.29033625273981
- type: v_measure_std
value: 1.0596383629128594
task:
type: Clustering
- dataset:
config: default
name: MTEB MindSmallReranking
revision: 59042f120c80e8afa9cdbb224f67076cec0fc9a7
split: test
type: mteb/mind_small
metrics:
- type: main_score
value: 33.0526129239962
- type: map
value: 33.0526129239962
- type: mrr
value: 34.29260046890935
- type: nAUC_map_diff1
value: 12.579738077238032
- type: nAUC_map_max
value: -20.936629344962
- type: nAUC_map_std
value: -1.6096805784945216
- type: nAUC_mrr_diff1
value: 11.597584463580807
- type: nAUC_mrr_max
value: -15.723702838537504
- type: nAUC_mrr_std
value: 0.2719172965777737
task:
type: Reranking
- dataset:
config: default
name: MTEB NFCorpus
revision: ec0fa4fe99da2ff19ca1214b7966684033a58814
split: test
type: mteb/nfcorpus
metrics:
- type: main_score
value: 41.486000000000004
- type: map_at_1
value: 6.866
- type: map_at_10
value: 15.895999999999999
- type: map_at_100
value: 21.093
- type: map_at_1000
value: 23.067
- type: map_at_20
value: 18.125
- type: map_at_3
value: 11.421000000000001
- type: map_at_5
value: 13.415
- type: mrr_at_1
value: 52.63157894736842
- type: mrr_at_10
value: 61.486805248415166
- type: mrr_at_100
value: 62.08211009182091
- type: mrr_at_1000
value: 62.10828701365016
- type: mrr_at_20
value: 61.904411187915784
- type: mrr_at_3
value: 59.90712074303407
- type: mrr_at_5
value: 60.91331269349847
- type: nauc_map_at_1000_diff1
value: 25.484625278529403
- type: nauc_map_at_1000_max
value: 31.206600396418853
- type: nauc_map_at_1000_std
value: 15.569448072357156
- type: nauc_map_at_100_diff1
value: 27.636750226316764
- type: nauc_map_at_100_max
value: 29.66992681250722
- type: nauc_map_at_100_std
value: 10.570600484002671
- type: nauc_map_at_10_diff1
value: 32.76642525548697
- type: nauc_map_at_10_max
value: 21.459225397237663
- type: nauc_map_at_10_std
value: -3.546494734209264
- type: nauc_map_at_1_diff1
value: 48.8002894871328
- type: nauc_map_at_1_max
value: 5.7236722609868815
- type: nauc_map_at_1_std
value: -13.283554044471352
- type: nauc_map_at_20_diff1
value: 30.57169701502308
- type: nauc_map_at_20_max
value: 25.79666139518404
- type: nauc_map_at_20_std
value: 1.781732492989651
- type: nauc_map_at_3_diff1
value: 40.076315947201095
- type: nauc_map_at_3_max
value: 12.862524429140054
- type: nauc_map_at_3_std
value: -9.188349777126817
- type: nauc_map_at_5_diff1
value: 36.9918718052938
- type: nauc_map_at_5_max
value: 16.74234374361876
- type: nauc_map_at_5_std
value: -7.818523349307494
- type: nauc_mrr_at_1000_diff1
value: 26.88183002609805
- type: nauc_mrr_at_1000_max
value: 47.10209348428658
- type: nauc_mrr_at_1000_std
value: 32.067825924992924
- type: nauc_mrr_at_100_diff1
value: 26.871482491566745
- type: nauc_mrr_at_100_max
value: 47.11303868498556
- type: nauc_mrr_at_100_std
value: 32.08961428818868
- type: nauc_mrr_at_10_diff1
value: 26.6356914977722
- type: nauc_mrr_at_10_max
value: 47.091624558810366
- type: nauc_mrr_at_10_std
value: 31.942424120660164
- type: nauc_mrr_at_1_diff1
value: 28.19774198483673
- type: nauc_mrr_at_1_max
value: 41.44380927834253
- type: nauc_mrr_at_1_std
value: 25.18222691885917
- type: nauc_mrr_at_20_diff1
value: 26.86487347109452
- type: nauc_mrr_at_20_max
value: 47.1987778214726
- type: nauc_mrr_at_20_std
value: 32.143517921610034
- type: nauc_mrr_at_3_diff1
value: 27.34340373236422
- type: nauc_mrr_at_3_max
value: 46.358726506276646
- type: nauc_mrr_at_3_std
value: 31.74924155572593
- type: nauc_mrr_at_5_diff1
value: 27.209667205060672
- type: nauc_mrr_at_5_max
value: 46.79883369072009
- type: nauc_mrr_at_5_std
value: 31.655605306670758
- type: nauc_ndcg_at_1000_diff1
value: 18.940195769769687
- type: nauc_ndcg_at_1000_max
value: 46.48551313937331
- type: nauc_ndcg_at_1000_std
value: 33.64819502089232
- type: nauc_ndcg_at_100_diff1
value: 19.50885253809146
- type: nauc_ndcg_at_100_max
value: 40.53174462354878
- type: nauc_ndcg_at_100_std
value: 28.516152877751118
- type: nauc_ndcg_at_10_diff1
value: 16.01699218096564
- type: nauc_ndcg_at_10_max
value: 41.17322878314514
- type: nauc_ndcg_at_10_std
value: 29.002233224832196
- type: nauc_ndcg_at_1_diff1
value: 27.443547710102205
- type: nauc_ndcg_at_1_max
value: 40.66529763309582
- type: nauc_ndcg_at_1_std
value: 24.15016766225869
- type: nauc_ndcg_at_20_diff1
value: 17.541197675685062
- type: nauc_ndcg_at_20_max
value: 40.53231266973844
- type: nauc_ndcg_at_20_std
value: 29.54096347876548
- type: nauc_ndcg_at_3_diff1
value: 18.649628357473716
- type: nauc_ndcg_at_3_max
value: 41.18603570171764
- type: nauc_ndcg_at_3_std
value: 27.125524188420396
- type: nauc_ndcg_at_5_diff1
value: 17.519593751448483
- type: nauc_ndcg_at_5_max
value: 42.715997890377345
- type: nauc_ndcg_at_5_std
value: 27.902627839899868
- type: nauc_precision_at_1000_diff1
value: -15.528797630565155
- type: nauc_precision_at_1000_max
value: 13.741640921778671
- type: nauc_precision_at_1000_std
value: 44.50896053788372
- type: nauc_precision_at_100_diff1
value: -14.491464489721887
- type: nauc_precision_at_100_max
value: 23.136434418999457
- type: nauc_precision_at_100_std
value: 49.73145147863128
- type: nauc_precision_at_10_diff1
value: -4.829188942994277
- type: nauc_precision_at_10_max
value: 40.327612559528866
- type: nauc_precision_at_10_std
value: 39.34919529635044
- type: nauc_precision_at_1_diff1
value: 28.19774198483673
- type: nauc_precision_at_1_max
value: 41.44380927834253
- type: nauc_precision_at_1_std
value: 25.18222691885917
- type: nauc_precision_at_20_diff1
value: -7.210726293112847
- type: nauc_precision_at_20_max
value: 37.195679576636984
- type: nauc_precision_at_20_std
value: 45.4597096418357
- type: nauc_precision_at_3_diff1
value: 7.578219537774854
- type: nauc_precision_at_3_max
value: 41.59775233475654
- type: nauc_precision_at_3_std
value: 30.764584790895118
- type: nauc_precision_at_5_diff1
value: 1.655451789039598
- type: nauc_precision_at_5_max
value: 43.435739407610455
- type: nauc_precision_at_5_std
value: 33.42552263325999
- type: nauc_recall_at_1000_diff1
value: 5.030705700690516
- type: nauc_recall_at_1000_max
value: 19.108072570815583
- type: nauc_recall_at_1000_std
value: 14.697734974217308
- type: nauc_recall_at_100_diff1
value: 14.746540318132407
- type: nauc_recall_at_100_max
value: 21.798705033854795
- type: nauc_recall_at_100_std
value: 11.416195108842587
- type: nauc_recall_at_10_diff1
value: 25.548642427860486
- type: nauc_recall_at_10_max
value: 18.711677681987474
- type: nauc_recall_at_10_std
value: -5.988904818971677
- type: nauc_recall_at_1_diff1
value: 48.8002894871328
- type: nauc_recall_at_1_max
value: 5.7236722609868815
- type: nauc_recall_at_1_std
value: -13.283554044471352
- type: nauc_recall_at_20_diff1
value: 23.39140739154809
- type: nauc_recall_at_20_max
value: 19.351150636155474
- type: nauc_recall_at_20_std
value: -2.757280266915132
- type: nauc_recall_at_3_diff1
value: 38.17453576012812
- type: nauc_recall_at_3_max
value: 13.47003839643972
- type: nauc_recall_at_3_std
value: -8.75780163862688
- type: nauc_recall_at_5_diff1
value: 33.02812855226899
- type: nauc_recall_at_5_max
value: 15.477626408978477
- type: nauc_recall_at_5_std
value: -9.072206441070708
- type: ndcg_at_1
value: 50.773999999999994
- type: ndcg_at_10
value: 41.486000000000004
- type: ndcg_at_100
value: 39.051
- type: ndcg_at_1000
value: 48.106
- type: ndcg_at_20
value: 39.432
- type: ndcg_at_3
value: 47.428
- type: ndcg_at_5
value: 45.227000000000004
- type: precision_at_1
value: 52.632
- type: precision_at_10
value: 31.146
- type: precision_at_100
value: 10.328
- type: precision_at_1000
value: 2.432
- type: precision_at_20
value: 23.793
- type: precision_at_3
value: 45.201
- type: precision_at_5
value: 39.876
- type: recall_at_1
value: 6.866
- type: recall_at_10
value: 20.447000000000003
- type: recall_at_100
value: 40.607
- type: recall_at_1000
value: 73.411
- type: recall_at_20
value: 26.082
- type: recall_at_3
value: 12.484
- type: recall_at_5
value: 15.847
task:
type: Retrieval
- dataset:
config: default
name: MTEB NQ
revision: b774495ed302d8c44a3a7ea25c90dbce03968f31
split: test
type: mteb/nq
metrics:
- type: main_score
value: 69.072
- type: map_at_1
value: 45.483000000000004
- type: map_at_10
value: 62.050000000000004
- type: map_at_100
value: 62.693
- type: map_at_1000
value: 62.702999999999996
- type: map_at_20
value: 62.498
- type: map_at_3
value: 58.285
- type: map_at_5
value: 60.711000000000006
- type: mrr_at_1
value: 50.840092699884124
- type: mrr_at_10
value: 64.54635224116673
- type: mrr_at_100
value: 64.9526548702289
- type: mrr_at_1000
value: 64.95908460752281
- type: mrr_at_20
value: 64.82949565799959
- type: mrr_at_3
value: 61.89165701042856
- type: mrr_at_5
value: 63.632676709154026
- type: nauc_map_at_1000_diff1
value: 43.187285304185224
- type: nauc_map_at_1000_max
value: 32.39921659632756
- type: nauc_map_at_1000_std
value: -5.780901333066553
- type: nauc_map_at_100_diff1
value: 43.184487221204456
- type: nauc_map_at_100_max
value: 32.41176116347982
- type: nauc_map_at_100_std
value: -5.76422606662383
- type: nauc_map_at_10_diff1
value: 42.967066814031746
- type: nauc_map_at_10_max
value: 32.489617364418514
- type: nauc_map_at_10_std
value: -6.029045531102664
- type: nauc_map_at_1_diff1
value: 46.16376563218624
- type: nauc_map_at_1_max
value: 26.342624776802232
- type: nauc_map_at_1_std
value: -7.142171388751972
- type: nauc_map_at_20_diff1
value: 43.15894358608328
- type: nauc_map_at_20_max
value: 32.46492198956245
- type: nauc_map_at_20_std
value: -5.788373305449195
- type: nauc_map_at_3_diff1
value: 43.231752344608545
- type: nauc_map_at_3_max
value: 31.68003009949564
- type: nauc_map_at_3_std
value: -8.015235132765458
- type: nauc_map_at_5_diff1
value: 42.86197608819917
- type: nauc_map_at_5_max
value: 32.363857571094485
- type: nauc_map_at_5_std
value: -6.780487416387977
- type: nauc_mrr_at_1000_diff1
value: 43.40542912045782
- type: nauc_mrr_at_1000_max
value: 32.8461770324533
- type: nauc_mrr_at_1000_std
value: -3.6505425530008204
- type: nauc_mrr_at_100_diff1
value: 43.40233508014468
- type: nauc_mrr_at_100_max
value: 32.85598538385942
- type: nauc_mrr_at_100_std
value: -3.637477352635459
- type: nauc_mrr_at_10_diff1
value: 43.260179162806054
- type: nauc_mrr_at_10_max
value: 32.942643527040474
- type: nauc_mrr_at_10_std
value: -3.712052825320437
- type: nauc_mrr_at_1_diff1
value: 46.354919460881206
- type: nauc_mrr_at_1_max
value: 29.1760258591106
- type: nauc_mrr_at_1_std
value: -4.107225031227406
- type: nauc_mrr_at_20_diff1
value: 43.37092385434311
- type: nauc_mrr_at_20_max
value: 32.93390254712846
- type: nauc_mrr_at_20_std
value: -3.5719056112132006
- type: nauc_mrr_at_3_diff1
value: 43.1744474040527
- type: nauc_mrr_at_3_max
value: 32.741290559777994
- type: nauc_mrr_at_3_std
value: -4.72677925120697
- type: nauc_mrr_at_5_diff1
value: 43.108396819975674
- type: nauc_mrr_at_5_max
value: 32.970519514893084
- type: nauc_mrr_at_5_std
value: -4.090906158975974
- type: nauc_ndcg_at_1000_diff1
value: 42.786664193638714
- type: nauc_ndcg_at_1000_max
value: 33.65554095609296
- type: nauc_ndcg_at_1000_std
value: -4.024030130584482
- type: nauc_ndcg_at_100_diff1
value: 42.691246775210814
- type: nauc_ndcg_at_100_max
value: 34.063232335110875
- type: nauc_ndcg_at_100_std
value: -3.477813807415248
- type: nauc_ndcg_at_10_diff1
value: 41.90988990571757
- type: nauc_ndcg_at_10_max
value: 34.58934812881633
- type: nauc_ndcg_at_10_std
value: -4.3295110195497655
- type: nauc_ndcg_at_1_diff1
value: 46.354919460881206
- type: nauc_ndcg_at_1_max
value: 29.1760258591106
- type: nauc_ndcg_at_1_std
value: -4.107225031227406
- type: nauc_ndcg_at_20_diff1
value: 42.493206675867114
- type: nauc_ndcg_at_20_max
value: 34.562441307459544
- type: nauc_ndcg_at_20_std
value: -3.4456116866749107
- type: nauc_ndcg_at_3_diff1
value: 42.24180336502808
- type: nauc_ndcg_at_3_max
value: 33.064267018100594
- type: nauc_ndcg_at_3_std
value: -7.786248093572142
- type: nauc_ndcg_at_5_diff1
value: 41.692714787779565
- type: nauc_ndcg_at_5_max
value: 34.20502498949156
- type: nauc_ndcg_at_5_std
value: -5.979557859282785
- type: nauc_precision_at_1000_diff1
value: -13.779832506640702
- type: nauc_precision_at_1000_max
value: 1.243001688631421
- type: nauc_precision_at_1000_std
value: 17.351623398622323
- type: nauc_precision_at_100_diff1
value: -11.310526816290297
- type: nauc_precision_at_100_max
value: 5.771669506192959
- type: nauc_precision_at_100_std
value: 19.917795079540113
- type: nauc_precision_at_10_diff1
value: 2.163699384635286
- type: nauc_precision_at_10_max
value: 19.66440698458386
- type: nauc_precision_at_10_std
value: 13.689876348315726
- type: nauc_precision_at_1_diff1
value: 46.354919460881206
- type: nauc_precision_at_1_max
value: 29.1760258591106
- type: nauc_precision_at_1_std
value: -4.107225031227406
- type: nauc_precision_at_20_diff1
value: -3.038735879584471
- type: nauc_precision_at_20_max
value: 14.132968299701695
- type: nauc_precision_at_20_std
value: 17.78069734664346
- type: nauc_precision_at_3_diff1
value: 21.783760758070095
- type: nauc_precision_at_3_max
value: 30.244127986404497
- type: nauc_precision_at_3_std
value: -0.12411163467738723
- type: nauc_precision_at_5_diff1
value: 10.980635723302418
- type: nauc_precision_at_5_max
value: 25.302293738975575
- type: nauc_precision_at_5_std
value: 6.4740817488722024
- type: nauc_recall_at_1000_diff1
value: 34.10343772356593
- type: nauc_recall_at_1000_max
value: 80.72497340357538
- type: nauc_recall_at_1000_std
value: 69.54564103264093
- type: nauc_recall_at_100_diff1
value: 33.427719956774126
- type: nauc_recall_at_100_max
value: 71.54086768335449
- type: nauc_recall_at_100_std
value: 49.66157377654885
- type: nauc_recall_at_10_diff1
value: 33.70139560054039
- type: nauc_recall_at_10_max
value: 45.47878072860151
- type: nauc_recall_at_10_std
value: 1.4188516615716378
- type: nauc_recall_at_1_diff1
value: 46.16376563218624
- type: nauc_recall_at_1_max
value: 26.342624776802232
- type: nauc_recall_at_1_std
value: -7.142171388751972
- type: nauc_recall_at_20_diff1
value: 35.805379874970086
- type: nauc_recall_at_20_max
value: 51.80479822253392
- type: nauc_recall_at_20_std
value: 13.531467576460143
- type: nauc_recall_at_3_diff1
value: 37.288500141631616
- type: nauc_recall_at_3_max
value: 35.07078243516728
- type: nauc_recall_at_3_std
value: -10.452926441410405
- type: nauc_recall_at_5_diff1
value: 34.83186104526897
- type: nauc_recall_at_5_max
value: 39.58488976496973
- type: nauc_recall_at_5_std
value: -6.3049292065708835
- type: ndcg_at_1
value: 50.839999999999996
- type: ndcg_at_10
value: 69.072
- type: ndcg_at_100
value: 71.538
- type: ndcg_at_1000
value: 71.77799999999999
- type: ndcg_at_20
value: 70.41
- type: ndcg_at_3
value: 62.544999999999995
- type: ndcg_at_5
value: 66.33099999999999
- type: precision_at_1
value: 50.839999999999996
- type: precision_at_10
value: 10.495000000000001
- type: precision_at_100
value: 1.1900000000000002
- type: precision_at_1000
value: 0.121
- type: precision_at_20
value: 5.5809999999999995
- type: precision_at_3
value: 27.636
- type: precision_at_5
value: 18.864
- type: recall_at_1
value: 45.483000000000004
- type: recall_at_10
value: 87.483
- type: recall_at_100
value: 97.844
- type: recall_at_1000
value: 99.66199999999999
- type: recall_at_20
value: 92.294
- type: recall_at_3
value: 71.2
- type: recall_at_5
value: 79.753
task:
type: Retrieval
- dataset:
config: default
name: MTEB QuoraRetrieval
revision: e4e08e0b7dbe3c8700f0daef558ff32256715259
split: test
type: mteb/quora
metrics:
- type: main_score
value: 89.58
- type: map_at_1
value: 71.819
- type: map_at_10
value: 86.04899999999999
- type: map_at_100
value: 86.648
- type: map_at_1000
value: 86.66199999999999
- type: map_at_20
value: 86.441
- type: map_at_3
value: 83.114
- type: map_at_5
value: 84.981
- type: mrr_at_1
value: 82.62
- type: mrr_at_10
value: 88.62899999999979
- type: mrr_at_100
value: 88.70918591324215
- type: mrr_at_1000
value: 88.70973091492397
- type: mrr_at_20
value: 88.68914765317221
- type: mrr_at_3
value: 87.74999999999979
- type: mrr_at_5
value: 88.36799999999974
- type: nauc_map_at_1000_diff1
value: 77.89207709760448
- type: nauc_map_at_1000_max
value: 29.63371361495422
- type: nauc_map_at_1000_std
value: -48.628180385874344
- type: nauc_map_at_100_diff1
value: 77.89592179104915
- type: nauc_map_at_100_max
value: 29.617171506130756
- type: nauc_map_at_100_std
value: -48.66057170774648
- type: nauc_map_at_10_diff1
value: 78.0618161228185
- type: nauc_map_at_10_max
value: 29.178490609366737
- type: nauc_map_at_10_std
value: -50.74755004592002
- type: nauc_map_at_1_diff1
value: 81.64335579973574
- type: nauc_map_at_1_max
value: 21.813832226652174
- type: nauc_map_at_1_std
value: -42.57570978190876
- type: nauc_map_at_20_diff1
value: 77.9299081005938
- type: nauc_map_at_20_max
value: 29.458718470003888
- type: nauc_map_at_20_std
value: -49.63337236763102
- type: nauc_map_at_3_diff1
value: 78.72941448509229
- type: nauc_map_at_3_max
value: 26.600997896960056
- type: nauc_map_at_3_std
value: -51.889002227479885
- type: nauc_map_at_5_diff1
value: 78.31466610917171
- type: nauc_map_at_5_max
value: 28.09863984582896
- type: nauc_map_at_5_std
value: -52.14058096096497
- type: nauc_mrr_at_1000_diff1
value: 78.42667263739992
- type: nauc_mrr_at_1000_max
value: 31.98996235127974
- type: nauc_mrr_at_1000_std
value: -44.380439148429296
- type: nauc_mrr_at_100_diff1
value: 78.42661032698115
- type: nauc_mrr_at_100_max
value: 31.991652631740102
- type: nauc_mrr_at_100_std
value: -44.37854108460535
- type: nauc_mrr_at_10_diff1
value: 78.39126022544136
- type: nauc_mrr_at_10_max
value: 32.02023484451197
- type: nauc_mrr_at_10_std
value: -44.561252349176954
- type: nauc_mrr_at_1_diff1
value: 79.21630894647448
- type: nauc_mrr_at_1_max
value: 31.526303156060177
- type: nauc_mrr_at_1_std
value: -41.887504422443136
- type: nauc_mrr_at_20_diff1
value: 78.42548039170424
- type: nauc_mrr_at_20_max
value: 31.99588275070137
- type: nauc_mrr_at_20_std
value: -44.44957722627042
- type: nauc_mrr_at_3_diff1
value: 78.26165151833735
- type: nauc_mrr_at_3_max
value: 32.18028826126801
- type: nauc_mrr_at_3_std
value: -44.6998237213182
- type: nauc_mrr_at_5_diff1
value: 78.34786430903962
- type: nauc_mrr_at_5_max
value: 32.168476272879566
- type: nauc_mrr_at_5_std
value: -44.7915919956712
- type: nauc_ndcg_at_1000_diff1
value: 77.79198355957816
- type: nauc_ndcg_at_1000_max
value: 31.14363511518406
- type: nauc_ndcg_at_1000_std
value: -46.69335151274275
- type: nauc_ndcg_at_100_diff1
value: 77.79898090286419
- type: nauc_ndcg_at_100_max
value: 31.115103811629215
- type: nauc_ndcg_at_100_std
value: -46.73078913421965
- type: nauc_ndcg_at_10_diff1
value: 77.74856635461343
- type: nauc_ndcg_at_10_max
value: 30.279584686212747
- type: nauc_ndcg_at_10_std
value: -50.23514662356807
- type: nauc_ndcg_at_1_diff1
value: 79.17833000040999
- type: nauc_ndcg_at_1_max
value: 31.703788144510746
- type: nauc_ndcg_at_1_std
value: -41.854817402870715
- type: nauc_ndcg_at_20_diff1
value: 77.7380353804671
- type: nauc_ndcg_at_20_max
value: 30.622294129001553
- type: nauc_ndcg_at_20_std
value: -49.035794761065254
- type: nauc_ndcg_at_3_diff1
value: 77.41476880573593
- type: nauc_ndcg_at_3_max
value: 29.015949978243032
- type: nauc_ndcg_at_3_std
value: -49.78627087622648
- type: nauc_ndcg_at_5_diff1
value: 77.64439137502896
- type: nauc_ndcg_at_5_max
value: 29.444684897492206
- type: nauc_ndcg_at_5_std
value: -51.21908400252501
- type: nauc_precision_at_1000_diff1
value: -44.92396459446822
- type: nauc_precision_at_1000_max
value: -3.674153720989045
- type: nauc_precision_at_1000_std
value: 39.56552468277785
- type: nauc_precision_at_100_diff1
value: -44.75143023259094
- type: nauc_precision_at_100_max
value: -3.705280025140011
- type: nauc_precision_at_100_std
value: 39.433619999113326
- type: nauc_precision_at_10_diff1
value: -41.0651074726579
- type: nauc_precision_at_10_max
value: -0.21097985601783667
- type: nauc_precision_at_10_std
value: 26.24652824589493
- type: nauc_precision_at_1_diff1
value: 79.17833000040999
- type: nauc_precision_at_1_max
value: 31.703788144510746
- type: nauc_precision_at_1_std
value: -41.854817402870715
- type: nauc_precision_at_20_diff1
value: -43.368001340920294
- type: nauc_precision_at_20_max
value: -2.036990010399129
- type: nauc_precision_at_20_std
value: 32.37747041406297
- type: nauc_precision_at_3_diff1
value: -22.089307548346877
- type: nauc_precision_at_3_max
value: 6.2280973175296
- type: nauc_precision_at_3_std
value: 5.323992514036145
- type: nauc_precision_at_5_diff1
value: -34.07115055244003
- type: nauc_precision_at_5_max
value: 2.5955315789198834
- type: nauc_precision_at_5_std
value: 16.26096689407332
- type: nauc_recall_at_1000_diff1
value: 58.27703860947467
- type: nauc_recall_at_1000_max
value: 68.59835835315768
- type: nauc_recall_at_1000_std
value: 77.96687006056064
- type: nauc_recall_at_100_diff1
value: 73.24371223081737
- type: nauc_recall_at_100_max
value: 39.55925344664591
- type: nauc_recall_at_100_std
value: -32.25605030215798
- type: nauc_recall_at_10_diff1
value: 73.41261201339202
- type: nauc_recall_at_10_max
value: 26.822979434062926
- type: nauc_recall_at_10_std
value: -74.2909332592806
- type: nauc_recall_at_1_diff1
value: 81.64335579973574
- type: nauc_recall_at_1_max
value: 21.813832226652174
- type: nauc_recall_at_1_std
value: -42.57570978190876
- type: nauc_recall_at_20_diff1
value: 72.7621297920656
- type: nauc_recall_at_20_max
value: 26.02492304096079
- type: nauc_recall_at_20_std
value: -77.8724532438279
- type: nauc_recall_at_3_diff1
value: 75.25149312810714
- type: nauc_recall_at_3_max
value: 23.20545662481487
- type: nauc_recall_at_3_std
value: -59.69689982140521
- type: nauc_recall_at_5_diff1
value: 73.69807273001406
- type: nauc_recall_at_5_max
value: 24.073666798066057
- type: nauc_recall_at_5_std
value: -67.91121268130719
- type: ndcg_at_1
value: 82.64
- type: ndcg_at_10
value: 89.58
- type: ndcg_at_100
value: 90.606
- type: ndcg_at_1000
value: 90.676
- type: ndcg_at_20
value: 90.132
- type: ndcg_at_3
value: 86.88
- type: ndcg_at_5
value: 88.40299999999999
- type: precision_at_1
value: 82.64
- type: precision_at_10
value: 13.604
- type: precision_at_100
value: 1.539
- type: precision_at_1000
value: 0.157
- type: precision_at_20
value: 7.188
- type: precision_at_3
value: 38.083
- type: precision_at_5
value: 25.018
- type: recall_at_1
value: 71.819
- type: recall_at_10
value: 96.34700000000001
- type: recall_at_100
value: 99.715
- type: recall_at_1000
value: 99.995
- type: recall_at_20
value: 98.073
- type: recall_at_3
value: 88.57300000000001
- type: recall_at_5
value: 92.908
task:
type: Retrieval
- dataset:
config: default
name: MTEB RedditClustering
revision: 24640382cdbf8abc73003fb0fa6d111a705499eb
split: test
type: mteb/reddit-clustering
metrics:
- type: main_score
value: 71.18966762070158
- type: v_measure
value: 71.18966762070158
- type: v_measure_std
value: 2.7498969054457048
task:
type: Clustering
- dataset:
config: default
name: MTEB RedditClusteringP2P
revision: 385e3cb46b4cfa89021f56c4380204149d0efe33
split: test
type: mteb/reddit-clustering-p2p
metrics:
- type: main_score
value: 74.42014716862516
- type: v_measure
value: 74.42014716862516
- type: v_measure_std
value: 9.909739891410648
task:
type: Clustering
- dataset:
config: default
name: MTEB SCIDOCS
revision: f8c2fcf00f625baaa80f62ec5bd9e1fff3b8ae88
split: test
type: mteb/scidocs
metrics:
- type: main_score
value: 25.041999999999998
- type: map_at_1
value: 5.893000000000001
- type: map_at_10
value: 15.260000000000002
- type: map_at_100
value: 18.084
- type: map_at_1000
value: 18.467
- type: map_at_20
value: 16.675
- type: map_at_3
value: 10.526
- type: map_at_5
value: 12.775
- type: mrr_at_1
value: 28.999999999999996
- type: mrr_at_10
value: 41.03575396825395
- type: mrr_at_100
value: 42.136771862785835
- type: mrr_at_1000
value: 42.16698555415099
- type: mrr_at_20
value: 41.707493696104315
- type: mrr_at_3
value: 37.34999999999998
- type: mrr_at_5
value: 39.59999999999995
- type: nauc_map_at_1000_diff1
value: 12.080002654911883
- type: nauc_map_at_1000_max
value: 29.813563682286276
- type: nauc_map_at_1000_std
value: 20.36659817908673
- type: nauc_map_at_100_diff1
value: 12.108735517749706
- type: nauc_map_at_100_max
value: 29.76830671710955
- type: nauc_map_at_100_std
value: 20.3433621032846
- type: nauc_map_at_10_diff1
value: 12.91575031185637
- type: nauc_map_at_10_max
value: 29.427600958386318
- type: nauc_map_at_10_std
value: 16.89867275177153
- type: nauc_map_at_1_diff1
value: 19.353069488987916
- type: nauc_map_at_1_max
value: 17.093914951159693
- type: nauc_map_at_1_std
value: 8.19886078055046
- type: nauc_map_at_20_diff1
value: 11.977233457943113
- type: nauc_map_at_20_max
value: 29.171812822948805
- type: nauc_map_at_20_std
value: 18.780517506173965
- type: nauc_map_at_3_diff1
value: 14.453129464176092
- type: nauc_map_at_3_max
value: 25.801958649112077
- type: nauc_map_at_3_std
value: 11.572823684429643
- type: nauc_map_at_5_diff1
value: 13.167155808104997
- type: nauc_map_at_5_max
value: 27.355626948365792
- type: nauc_map_at_5_std
value: 14.414151839192183
- type: nauc_mrr_at_1000_diff1
value: 17.262104643988636
- type: nauc_mrr_at_1000_max
value: 23.991373837217058
- type: nauc_mrr_at_1000_std
value: 12.44755488671623
- type: nauc_mrr_at_100_diff1
value: 17.267280132318703
- type: nauc_mrr_at_100_max
value: 24.022189287889294
- type: nauc_mrr_at_100_std
value: 12.480695500214788
- type: nauc_mrr_at_10_diff1
value: 17.012383998246268
- type: nauc_mrr_at_10_max
value: 24.192637911171722
- type: nauc_mrr_at_10_std
value: 12.524608847408917
- type: nauc_mrr_at_1_diff1
value: 19.43518811038007
- type: nauc_mrr_at_1_max
value: 17.747482933395602
- type: nauc_mrr_at_1_std
value: 8.410779775558684
- type: nauc_mrr_at_20_diff1
value: 17.202663281407446
- type: nauc_mrr_at_20_max
value: 24.091991130543118
- type: nauc_mrr_at_20_std
value: 12.503814263019908
- type: nauc_mrr_at_3_diff1
value: 17.52733013432995
- type: nauc_mrr_at_3_max
value: 23.569459518780214
- type: nauc_mrr_at_3_std
value: 11.770846827520726
- type: nauc_mrr_at_5_diff1
value: 17.10817561975543
- type: nauc_mrr_at_5_max
value: 23.945141435234678
- type: nauc_mrr_at_5_std
value: 12.034468615317719
- type: nauc_ndcg_at_1000_diff1
value: 12.317811393346936
- type: nauc_ndcg_at_1000_max
value: 30.809991350156103
- type: nauc_ndcg_at_1000_std
value: 24.517501065205067
- type: nauc_ndcg_at_100_diff1
value: 12.824804203182936
- type: nauc_ndcg_at_100_max
value: 30.895499817010748
- type: nauc_ndcg_at_100_std
value: 25.424376279745402
- type: nauc_ndcg_at_10_diff1
value: 13.32724552457439
- type: nauc_ndcg_at_10_max
value: 30.409088666807456
- type: nauc_ndcg_at_10_std
value: 18.216330475714113
- type: nauc_ndcg_at_1_diff1
value: 19.43518811038007
- type: nauc_ndcg_at_1_max
value: 17.747482933395602
- type: nauc_ndcg_at_1_std
value: 8.410779775558684
- type: nauc_ndcg_at_20_diff1
value: 12.224399111852902
- type: nauc_ndcg_at_20_max
value: 29.86352330445272
- type: nauc_ndcg_at_20_std
value: 21.196937851331807
- type: nauc_ndcg_at_3_diff1
value: 15.367489533734027
- type: nauc_ndcg_at_3_max
value: 26.76486390741532
- type: nauc_ndcg_at_3_std
value: 12.606077508789923
- type: nauc_ndcg_at_5_diff1
value: 13.831157482390935
- type: nauc_ndcg_at_5_max
value: 28.070226983968904
- type: nauc_ndcg_at_5_std
value: 15.236787943125435
- type: nauc_precision_at_1000_diff1
value: 0.016122957101357048
- type: nauc_precision_at_1000_max
value: 24.380929903557334
- type: nauc_precision_at_1000_std
value: 34.54045112720052
- type: nauc_precision_at_100_diff1
value: 7.255224788507301
- type: nauc_precision_at_100_max
value: 27.98453788447542
- type: nauc_precision_at_100_std
value: 35.38999555441665
- type: nauc_precision_at_10_diff1
value: 9.69185099834181
- type: nauc_precision_at_10_max
value: 32.532315522580454
- type: nauc_precision_at_10_std
value: 21.48948348473612
- type: nauc_precision_at_1_diff1
value: 19.43518811038007
- type: nauc_precision_at_1_max
value: 17.747482933395602
- type: nauc_precision_at_1_std
value: 8.410779775558684
- type: nauc_precision_at_20_diff1
value: 6.964076536695672
- type: nauc_precision_at_20_max
value: 29.30087236410044
- type: nauc_precision_at_20_std
value: 26.413625895571986
- type: nauc_precision_at_3_diff1
value: 14.145134359925155
- type: nauc_precision_at_3_max
value: 29.915650960808303
- type: nauc_precision_at_3_std
value: 14.095370019867797
- type: nauc_precision_at_5_diff1
value: 11.043933558522692
- type: nauc_precision_at_5_max
value: 30.93016505807111
- type: nauc_precision_at_5_std
value: 17.749256196062603
- type: nauc_recall_at_1000_diff1
value: -0.7776817772090345
- type: nauc_recall_at_1000_max
value: 23.094717340324518
- type: nauc_recall_at_1000_std
value: 37.189908681396425
- type: nauc_recall_at_100_diff1
value: 6.887748742013364
- type: nauc_recall_at_100_max
value: 27.00798435230277
- type: nauc_recall_at_100_std
value: 35.908147807345344
- type: nauc_recall_at_10_diff1
value: 9.605632017480751
- type: nauc_recall_at_10_max
value: 31.845202901168655
- type: nauc_recall_at_10_std
value: 21.497414586634683
- type: nauc_recall_at_1_diff1
value: 19.353069488987916
- type: nauc_recall_at_1_max
value: 17.093914951159693
- type: nauc_recall_at_1_std
value: 8.19886078055046
- type: nauc_recall_at_20_diff1
value: 6.927503731844782
- type: nauc_recall_at_20_max
value: 28.611698183338202
- type: nauc_recall_at_20_std
value: 26.69018660149911
- type: nauc_recall_at_3_diff1
value: 14.043724087062268
- type: nauc_recall_at_3_max
value: 29.269835821380465
- type: nauc_recall_at_3_std
value: 14.104419605998094
- type: nauc_recall_at_5_diff1
value: 11.017319452873336
- type: nauc_recall_at_5_max
value: 30.295720628306228
- type: nauc_recall_at_5_std
value: 17.758048545573825
- type: ndcg_at_1
value: 28.999999999999996
- type: ndcg_at_10
value: 25.041999999999998
- type: ndcg_at_100
value: 35.045
- type: ndcg_at_1000
value: 40.803
- type: ndcg_at_20
value: 28.584
- type: ndcg_at_3
value: 23.249
- type: ndcg_at_5
value: 20.533
- type: precision_at_1
value: 28.999999999999996
- type: precision_at_10
value: 13.120000000000001
- type: precision_at_100
value: 2.7470000000000003
- type: precision_at_1000
value: 0.41200000000000003
- type: precision_at_20
value: 8.584999999999999
- type: precision_at_3
value: 21.633
- type: precision_at_5
value: 18.099999999999998
- type: recall_at_1
value: 5.893000000000001
- type: recall_at_10
value: 26.567
- type: recall_at_100
value: 55.800000000000004
- type: recall_at_1000
value: 83.608
- type: recall_at_20
value: 34.86
- type: recall_at_3
value: 13.153
- type: recall_at_5
value: 18.323
task:
type: Retrieval
- dataset:
config: default
name: MTEB SICK-R
revision: 20a6d6f312dd54037fe07a32d58e5e168867909d
split: test
type: mteb/sickr-sts
metrics:
- type: cosine_pearson
value: 86.57284584320382
- type: cosine_spearman
value: 82.20531642680812
- type: euclidean_pearson
value: 83.94261758556554
- type: euclidean_spearman
value: 82.20721497738559
- type: main_score
value: 82.20531642680812
- type: manhattan_pearson
value: 84.15902154703083
- type: manhattan_spearman
value: 82.19506027155957
- type: pearson
value: 86.57284584320382
- type: spearman
value: 82.20531642680812
task:
type: STS
- dataset:
config: default
name: MTEB STS12
revision: a0d554a64d88156834ff5ae9920b964011b16384
split: test
type: mteb/sts12-sts
metrics:
- type: cosine_pearson
value: 86.28047602146931
- type: cosine_spearman
value: 79.51504881448884
- type: euclidean_pearson
value: 83.10545189967856
- type: euclidean_spearman
value: 79.50586960492797
- type: main_score
value: 79.51504881448884
- type: manhattan_pearson
value: 83.44244457500889
- type: manhattan_spearman
value: 79.730303339846
- type: pearson
value: 86.28047602146931
- type: spearman
value: 79.51504881448884
task:
type: STS
- dataset:
config: default
name: MTEB STS13
revision: 7e90230a92c190f1bf69ae9002b8cea547a64cca
split: test
type: mteb/sts13-sts
metrics:
- type: cosine_pearson
value: 88.74723553048702
- type: cosine_spearman
value: 89.18936052329725
- type: euclidean_pearson
value: 88.90400878928668
- type: euclidean_spearman
value: 89.19174821431281
- type: main_score
value: 89.18936052329725
- type: manhattan_pearson
value: 88.81504628424054
- type: manhattan_spearman
value: 89.18063294142597
- type: pearson
value: 88.74723553048702
- type: spearman
value: 89.18936052329725
task:
type: STS
- dataset:
config: default
name: MTEB STS14
revision: 6031580fec1f6af667f0bd2da0a551cf4f0b2375
split: test
type: mteb/sts14-sts
metrics:
- type: cosine_pearson
value: 86.45403437836023
- type: cosine_spearman
value: 85.14654611519086
- type: euclidean_pearson
value: 85.87509624462743
- type: euclidean_spearman
value: 85.1391108856681
- type: main_score
value: 85.14654611519086
- type: manhattan_pearson
value: 85.96635794953866
- type: manhattan_spearman
value: 85.3271371527667
- type: pearson
value: 86.45403437836023
- type: spearman
value: 85.14654611519086
task:
type: STS
- dataset:
config: default
name: MTEB STS15
revision: ae752c7c21bf194d8b67fd573edf7ae58183cbe3
split: test
type: mteb/sts15-sts
metrics:
- type: cosine_pearson
value: 87.84742260009705
- type: cosine_spearman
value: 89.10215217191254
- type: euclidean_pearson
value: 88.97393286325477
- type: euclidean_spearman
value: 89.1014105509662
- type: main_score
value: 89.10215217191254
- type: manhattan_pearson
value: 89.31698781090151
- type: manhattan_spearman
value: 89.53000001764433
- type: pearson
value: 87.84742260009705
- type: spearman
value: 89.10215217191254
task:
type: STS
- dataset:
config: default
name: MTEB STS16
revision: 4d8694f8f0e0100860b497b999b3dbed754a0513
split: test
type: mteb/sts16-sts
metrics:
- type: cosine_pearson
value: 85.22397535461835
- type: cosine_spearman
value: 87.14066355879785
- type: euclidean_pearson
value: 86.31393364087295
- type: euclidean_spearman
value: 87.14018892702765
- type: main_score
value: 87.14066355879785
- type: manhattan_pearson
value: 86.36366855248434
- type: manhattan_spearman
value: 87.20858630423012
- type: pearson
value: 85.22397535461835
- type: spearman
value: 87.14066355879785
task:
type: STS
- dataset:
config: en-en
name: MTEB STS17 (en-en)
revision: faeb762787bd10488a50c8b5be4a3b82e411949c
split: test
type: mteb/sts17-crosslingual-sts
metrics:
- type: cosine_pearson
value: 90.66131612061355
- type: cosine_spearman
value: 90.97082650129164
- type: euclidean_pearson
value: 90.98181906744969
- type: euclidean_spearman
value: 90.99008476850047
- type: main_score
value: 90.97082650129164
- type: manhattan_pearson
value: 90.75245040709021
- type: manhattan_spearman
value: 90.6199877691265
- type: pearson
value: 90.66131612061355
- type: spearman
value: 90.97082650129164
task:
type: STS
- dataset:
config: en
name: MTEB STS22 (en)
revision: de9d86b3b84231dc21f76c7b7af1f28e2f57f6e3
split: test
type: mteb/sts22-crosslingual-sts
metrics:
- type: cosine_pearson
value: 67.270656447085
- type: cosine_spearman
value: 67.82870469746828
- type: euclidean_pearson
value: 69.03857775285664
- type: euclidean_spearman
value: 67.74455108773341
- type: main_score
value: 67.82870469746828
- type: manhattan_pearson
value: 69.25304172245812
- type: manhattan_spearman
value: 68.00987097916055
- type: pearson
value: 67.270656447085
- type: spearman
value: 67.82870469746828
task:
type: STS
- dataset:
config: default
name: MTEB STSBenchmark
revision: b0fddb56ed78048fa8b90373c8a3cfc37b684831
split: test
type: mteb/stsbenchmark-sts
metrics:
- type: cosine_pearson
value: 87.17245205384889
- type: cosine_spearman
value: 87.7360146030987
- type: euclidean_pearson
value: 87.48919412794656
- type: euclidean_spearman
value: 87.7312047878383
- type: main_score
value: 87.7360146030987
- type: manhattan_pearson
value: 87.61476224354806
- type: manhattan_spearman
value: 87.95220889254693
- type: pearson
value: 87.17245205384889
- type: spearman
value: 87.7360146030987
task:
type: STS
- dataset:
config: default
name: MTEB SciDocsRR
revision: d3c5e1fc0b855ab6097bf1cda04dd73947d7caab
split: test
type: mteb/scidocs-reranking
metrics:
- type: main_score
value: 88.43547871921146
- type: map
value: 88.43547871921146
- type: mrr
value: 96.5564473652709
- type: nAUC_map_diff1
value: -13.66029392579231
- type: nAUC_map_max
value: 50.325613574053506
- type: nAUC_map_std
value: 60.02986231275796
- type: nAUC_mrr_diff1
value: 23.83821476411125
- type: nAUC_mrr_max
value: 86.72643311769906
- type: nAUC_mrr_std
value: 72.12741063469213
task:
type: Reranking
- dataset:
config: default
name: MTEB SciFact
revision: 0228b52cf27578f30900b9e5271d331663a030d7
split: test
type: mteb/scifact
metrics:
- type: main_score
value: 78.233
- type: map_at_1
value: 61.49400000000001
- type: map_at_10
value: 73.30600000000001
- type: map_at_100
value: 73.719
- type: map_at_1000
value: 73.724
- type: map_at_20
value: 73.611
- type: map_at_3
value: 70.626
- type: map_at_5
value: 72.417
- type: mrr_at_1
value: 64.66666666666666
- type: mrr_at_10
value: 74.30357142857143
- type: mrr_at_100
value: 74.56950898079988
- type: mrr_at_1000
value: 74.57295833098681
- type: mrr_at_20
value: 74.46165223665226
- type: mrr_at_3
value: 72.3888888888889
- type: mrr_at_5
value: 73.60555555555557
- type: nauc_map_at_1000_diff1
value: 76.51524604780636
- type: nauc_map_at_1000_max
value: 53.48521938401881
- type: nauc_map_at_1000_std
value: -7.347799382158861
- type: nauc_map_at_100_diff1
value: 76.5122888096236
- type: nauc_map_at_100_max
value: 53.49221847471618
- type: nauc_map_at_100_std
value: -7.329683735681086
- type: nauc_map_at_10_diff1
value: 76.30928630674504
- type: nauc_map_at_10_max
value: 53.00102977185941
- type: nauc_map_at_10_std
value: -7.7467740085108705
- type: nauc_map_at_1_diff1
value: 79.54189281784247
- type: nauc_map_at_1_max
value: 46.630071622109526
- type: nauc_map_at_1_std
value: -14.395943134644112
- type: nauc_map_at_20_diff1
value: 76.41604361947962
- type: nauc_map_at_20_max
value: 53.578883876146875
- type: nauc_map_at_20_std
value: -7.403103451288041
- type: nauc_map_at_3_diff1
value: 76.25911617571941
- type: nauc_map_at_3_max
value: 49.140287380513605
- type: nauc_map_at_3_std
value: -11.35992449218983
- type: nauc_map_at_5_diff1
value: 76.35122077770336
- type: nauc_map_at_5_max
value: 52.1744367901208
- type: nauc_map_at_5_std
value: -7.85753955055384
- type: nauc_mrr_at_1000_diff1
value: 76.97223309515867
- type: nauc_mrr_at_1000_max
value: 57.263787498613326
- type: nauc_mrr_at_1000_std
value: -4.884090708840035
- type: nauc_mrr_at_100_diff1
value: 76.97312970894603
- type: nauc_mrr_at_100_max
value: 57.26850730446478
- type: nauc_mrr_at_100_std
value: -4.875200894216617
- type: nauc_mrr_at_10_diff1
value: 76.65927674223613
- type: nauc_mrr_at_10_max
value: 57.30979763941454
- type: nauc_mrr_at_10_std
value: -4.863331094022142
- type: nauc_mrr_at_1_diff1
value: 80.0454932568644
- type: nauc_mrr_at_1_max
value: 56.76038421319305
- type: nauc_mrr_at_1_std
value: -4.101939392632653
- type: nauc_mrr_at_20_diff1
value: 76.87237970440503
- type: nauc_mrr_at_20_max
value: 57.33843605225869
- type: nauc_mrr_at_20_std
value: -4.96248984417978
- type: nauc_mrr_at_3_diff1
value: 76.74130186666727
- type: nauc_mrr_at_3_max
value: 56.19313244846155
- type: nauc_mrr_at_3_std
value: -5.684365934009136
- type: nauc_mrr_at_5_diff1
value: 76.66406918799962
- type: nauc_mrr_at_5_max
value: 57.56110093228628
- type: nauc_mrr_at_5_std
value: -3.7464413085588073
- type: nauc_ndcg_at_1000_diff1
value: 76.19194173971773
- type: nauc_ndcg_at_1000_max
value: 55.57464600170693
- type: nauc_ndcg_at_1000_std
value: -6.0761689532372625
- type: nauc_ndcg_at_100_diff1
value: 76.14631273843654
- type: nauc_ndcg_at_100_max
value: 55.72246565373382
- type: nauc_ndcg_at_100_std
value: -5.595160698860595
- type: nauc_ndcg_at_10_diff1
value: 75.0108223611192
- type: nauc_ndcg_at_10_max
value: 55.27894212877493
- type: nauc_ndcg_at_10_std
value: -6.968331740214591
- type: nauc_ndcg_at_1_diff1
value: 80.0454932568644
- type: nauc_ndcg_at_1_max
value: 56.76038421319305
- type: nauc_ndcg_at_1_std
value: -4.101939392632653
- type: nauc_ndcg_at_20_diff1
value: 75.54887755702472
- type: nauc_ndcg_at_20_max
value: 56.406879417251496
- type: nauc_ndcg_at_20_std
value: -6.495231061329629
- type: nauc_ndcg_at_3_diff1
value: 75.03620356688509
- type: nauc_ndcg_at_3_max
value: 52.147381077773424
- type: nauc_ndcg_at_3_std
value: -8.448005688956199
- type: nauc_ndcg_at_5_diff1
value: 75.1195898074229
- type: nauc_ndcg_at_5_max
value: 54.2321033861173
- type: nauc_ndcg_at_5_std
value: -5.882690780895338
- type: nauc_precision_at_1000_diff1
value: -28.081979732100532
- type: nauc_precision_at_1000_max
value: 35.055348014832916
- type: nauc_precision_at_1000_std
value: 59.61280468927384
- type: nauc_precision_at_100_diff1
value: -25.112740730587458
- type: nauc_precision_at_100_max
value: 38.26331300116496
- type: nauc_precision_at_100_std
value: 62.46316222328831
- type: nauc_precision_at_10_diff1
value: -2.6766206473658833
- type: nauc_precision_at_10_max
value: 45.95321867204845
- type: nauc_precision_at_10_std
value: 45.07212468670564
- type: nauc_precision_at_1_diff1
value: 80.0454932568644
- type: nauc_precision_at_1_max
value: 56.76038421319305
- type: nauc_precision_at_1_std
value: -4.101939392632653
- type: nauc_precision_at_20_diff1
value: -10.698911116738385
- type: nauc_precision_at_20_max
value: 43.467275950182994
- type: nauc_precision_at_20_std
value: 48.00467321991766
- type: nauc_precision_at_3_diff1
value: 33.6344708541193
- type: nauc_precision_at_3_max
value: 49.309242331670504
- type: nauc_precision_at_3_std
value: 21.02940391379915
- type: nauc_precision_at_5_diff1
value: 13.560415600596318
- type: nauc_precision_at_5_max
value: 48.918726500100085
- type: nauc_precision_at_5_std
value: 39.940930429172184
- type: nauc_recall_at_1000_diff1
value: .nan
- type: nauc_recall_at_1000_max
value: .nan
- type: nauc_recall_at_1000_std
value: .nan
- type: nauc_recall_at_100_diff1
value: 70.82166199813196
- type: nauc_recall_at_100_max
value: 76.6106442577042
- type: nauc_recall_at_100_std
value: 66.47992530345513
- type: nauc_recall_at_10_diff1
value: 62.68908885556092
- type: nauc_recall_at_10_max
value: 58.14262437741839
- type: nauc_recall_at_10_std
value: -12.946717875063369
- type: nauc_recall_at_1_diff1
value: 79.54189281784247
- type: nauc_recall_at_1_max
value: 46.630071622109526
- type: nauc_recall_at_1_std
value: -14.395943134644112
- type: nauc_recall_at_20_diff1
value: 65.79470497876567
- type: nauc_recall_at_20_max
value: 71.68308183488456
- type: nauc_recall_at_20_std
value: -12.556850697268453
- type: nauc_recall_at_3_diff1
value: 68.3240211318129
- type: nauc_recall_at_3_max
value: 45.05998217275036
- type: nauc_recall_at_3_std
value: -14.23179772593869
- type: nauc_recall_at_5_diff1
value: 67.53366869904056
- type: nauc_recall_at_5_max
value: 53.57935627081027
- type: nauc_recall_at_5_std
value: -3.3271112904853393
- type: ndcg_at_1
value: 64.667
- type: ndcg_at_10
value: 78.233
- type: ndcg_at_100
value: 79.806
- type: ndcg_at_1000
value: 79.92099999999999
- type: ndcg_at_20
value: 79.006
- type: ndcg_at_3
value: 74.018
- type: ndcg_at_5
value: 76.334
- type: precision_at_1
value: 64.667
- type: precision_at_10
value: 10.4
- type: precision_at_100
value: 1.1199999999999999
- type: precision_at_1000
value: 0.11299999999999999
- type: precision_at_20
value: 5.383
- type: precision_at_3
value: 29.444
- type: precision_at_5
value: 19.467000000000002
- type: recall_at_1
value: 61.49400000000001
- type: recall_at_10
value: 92.156
- type: recall_at_100
value: 99.167
- type: recall_at_1000
value: 100.0
- type: recall_at_20
value: 94.833
- type: recall_at_3
value: 80.833
- type: recall_at_5
value: 86.6
task:
type: Retrieval
- dataset:
config: default
name: MTEB SprintDuplicateQuestions
revision: d66bd1f72af766a5cc4b0ca5e00c162f89e8cc46
split: test
type: mteb/sprintduplicatequestions-pairclassification
metrics:
- type: cosine_accuracy
value: 99.8039603960396
- type: cosine_accuracy_threshold
value: 84.54211950302124
- type: cosine_ap
value: 95.59056372734358
- type: cosine_f1
value: 90.1394422310757
- type: cosine_f1_threshold
value: 84.54211950302124
- type: cosine_precision
value: 89.78174603174604
- type: cosine_recall
value: 90.5
- type: dot_accuracy
value: 99.80594059405941
- type: dot_accuracy_threshold
value: 85.57180166244507
- type: dot_ap
value: 95.53453431914399
- type: dot_f1
value: 90.10442565887618
- type: dot_f1_threshold
value: 84.59715843200684
- type: dot_precision
value: 89.61424332344214
- type: dot_recall
value: 90.60000000000001
- type: euclidean_accuracy
value: 99.8039603960396
- type: euclidean_accuracy_threshold
value: 53.253382444381714
- type: euclidean_ap
value: 95.5850992402159
- type: euclidean_f1
value: 90.09457441513192
- type: euclidean_f1_threshold
value: 55.725520849227905
- type: euclidean_precision
value: 89.69276511397423
- type: euclidean_recall
value: 90.5
- type: main_score
value: 95.7485189884476
- type: manhattan_accuracy
value: 99.81485148514851
- type: manhattan_accuracy_threshold
value: 3491.29638671875
- type: manhattan_ap
value: 95.7485189884476
- type: manhattan_f1
value: 90.464048954615
- type: manhattan_f1_threshold
value: 3491.29638671875
- type: manhattan_precision
value: 92.2996878251821
- type: manhattan_recall
value: 88.7
- type: max_ap
value: 95.7485189884476
- type: max_f1
value: 90.464048954615
- type: max_precision
value: 92.2996878251821
- type: max_recall
value: 90.60000000000001
- type: similarity_accuracy
value: 99.8039603960396
- type: similarity_accuracy_threshold
value: 84.54211950302124
- type: similarity_ap
value: 95.59056372734358
- type: similarity_f1
value: 90.1394422310757
- type: similarity_f1_threshold
value: 84.54211950302124
- type: similarity_precision
value: 89.78174603174604
- type: similarity_recall
value: 90.5
task:
type: PairClassification
- dataset:
config: default
name: MTEB StackExchangeClustering
revision: 6cbc1f7b2bc0622f2e39d2c77fa502909748c259
split: test
type: mteb/stackexchange-clustering
metrics:
- type: main_score
value: 78.49205191950675
- type: v_measure
value: 78.49205191950675
- type: v_measure_std
value: 2.84869550699959
task:
type: Clustering
- dataset:
config: default
name: MTEB StackExchangeClusteringP2P
revision: 815ca46b2622cec33ccafc3735d572c266efdb44
split: test
type: mteb/stackexchange-clustering-p2p
metrics:
- type: main_score
value: 48.90421736513028
- type: v_measure
value: 48.90421736513028
- type: v_measure_std
value: 1.6875865714471023
task:
type: Clustering
- dataset:
config: default
name: MTEB StackOverflowDupQuestions
revision: e185fbe320c72810689fc5848eb6114e1ef5ec69
split: test
type: mteb/stackoverflowdupquestions-reranking
metrics:
- type: main_score
value: 52.9874730481696
- type: map
value: 52.9874730481696
- type: mrr
value: 53.85867604617604
- type: nAUC_map_diff1
value: 39.633429293407616
- type: nAUC_map_max
value: 10.236807988858546
- type: nAUC_map_std
value: 10.276522217929674
- type: nAUC_mrr_diff1
value: 40.0543079218377
- type: nAUC_mrr_max
value: 10.96209807382042
- type: nAUC_mrr_std
value: 10.524400196109918
task:
type: Reranking
- dataset:
config: default
name: MTEB SummEval
revision: cda12ad7615edc362dbf25a00fdd61d3b1eaf93c
split: test
type: mteb/summeval
metrics:
- type: cosine_pearson
value: 30.727801109114232
- type: cosine_spearman
value: 31.66058223980157
- type: dot_pearson
value: 30.78818248622866
- type: dot_spearman
value: 31.525158776890265
- type: main_score
value: 31.66058223980157
- type: pearson
value: 30.727801109114232
- type: spearman
value: 31.66058223980157
task:
type: Summarization
- dataset:
config: default
name: MTEB TRECCOVID
revision: bb9466bac8153a0349341eb1b22e06409e78ef4e
split: test
type: mteb/trec-covid
metrics:
- type: main_score
value: 85.206
- type: map_at_1
value: 0.246
- type: map_at_10
value: 2.1950000000000003
- type: map_at_100
value: 14.179
- type: map_at_1000
value: 35.037
- type: map_at_20
value: 4.143
- type: map_at_3
value: 0.7100000000000001
- type: map_at_5
value: 1.135
- type: mrr_at_1
value: 94.0
- type: mrr_at_10
value: 96.66666666666666
- type: mrr_at_100
value: 96.66666666666666
- type: mrr_at_1000
value: 96.66666666666666
- type: mrr_at_20
value: 96.66666666666666
- type: mrr_at_3
value: 96.66666666666666
- type: mrr_at_5
value: 96.66666666666666
- type: nauc_map_at_1000_diff1
value: -4.6264497624527525
- type: nauc_map_at_1000_max
value: 44.594457564749355
- type: nauc_map_at_1000_std
value: 73.17642341400133
- type: nauc_map_at_100_diff1
value: 23.451335157405726
- type: nauc_map_at_100_max
value: 25.426398857299525
- type: nauc_map_at_100_std
value: 64.07416694472633
- type: nauc_map_at_10_diff1
value: 46.57568738568346
- type: nauc_map_at_10_max
value: 9.693233249079238
- type: nauc_map_at_10_std
value: 28.549530265164357
- type: nauc_map_at_1_diff1
value: 53.48238396620123
- type: nauc_map_at_1_max
value: 0.33476619393733076
- type: nauc_map_at_1_std
value: 8.906362219128463
- type: nauc_map_at_20_diff1
value: 39.40719602207749
- type: nauc_map_at_20_max
value: 9.635915072074045
- type: nauc_map_at_20_std
value: 35.15634791346394
- type: nauc_map_at_3_diff1
value: 53.11784737840137
- type: nauc_map_at_3_max
value: 3.059682761072153
- type: nauc_map_at_3_std
value: 21.310633086556617
- type: nauc_map_at_5_diff1
value: 49.91570701185436
- type: nauc_map_at_5_max
value: 8.045082896244576
- type: nauc_map_at_5_std
value: 20.597686235051647
- type: nauc_mrr_at_1000_diff1
value: 41.98412698412726
- type: nauc_mrr_at_1000_max
value: 78.24463118580779
- type: nauc_mrr_at_1000_std
value: 0.30812324930028195
- type: nauc_mrr_at_100_diff1
value: 41.98412698412726
- type: nauc_mrr_at_100_max
value: 78.24463118580779
- type: nauc_mrr_at_100_std
value: 0.30812324930028195
- type: nauc_mrr_at_10_diff1
value: 41.98412698412726
- type: nauc_mrr_at_10_max
value: 78.24463118580779
- type: nauc_mrr_at_10_std
value: 0.30812324930028195
- type: nauc_mrr_at_1_diff1
value: 38.62433862433873
- type: nauc_mrr_at_1_max
value: 80.78120136943666
- type: nauc_mrr_at_1_std
value: -10.768751945222197
- type: nauc_mrr_at_20_diff1
value: 41.98412698412726
- type: nauc_mrr_at_20_max
value: 78.24463118580779
- type: nauc_mrr_at_20_std
value: 0.30812324930028195
- type: nauc_mrr_at_3_diff1
value: 41.98412698412726
- type: nauc_mrr_at_3_max
value: 78.24463118580779
- type: nauc_mrr_at_3_std
value: 0.30812324930028195
- type: nauc_mrr_at_5_diff1
value: 41.98412698412726
- type: nauc_mrr_at_5_max
value: 78.24463118580779
- type: nauc_mrr_at_5_std
value: 0.30812324930028195
- type: nauc_ndcg_at_1000_diff1
value: 0.5174948602880207
- type: nauc_ndcg_at_1000_max
value: 48.60686602077053
- type: nauc_ndcg_at_1000_std
value: 75.72456343175277
- type: nauc_ndcg_at_100_diff1
value: -20.747252137999254
- type: nauc_ndcg_at_100_max
value: 49.985132618254994
- type: nauc_ndcg_at_100_std
value: 61.096383293836574
- type: nauc_ndcg_at_10_diff1
value: 6.791377920463332
- type: nauc_ndcg_at_10_max
value: 57.50019332833286
- type: nauc_ndcg_at_10_std
value: 49.201028841219426
- type: nauc_ndcg_at_1_diff1
value: 54.92683440362145
- type: nauc_ndcg_at_1_max
value: 83.8667228129276
- type: nauc_ndcg_at_1_std
value: 1.6738604063586122
- type: nauc_ndcg_at_20_diff1
value: -5.1948699196314925
- type: nauc_ndcg_at_20_max
value: 54.483087684806556
- type: nauc_ndcg_at_20_std
value: 50.54823818118781
- type: nauc_ndcg_at_3_diff1
value: 26.267246500164372
- type: nauc_ndcg_at_3_max
value: 63.0173212926611
- type: nauc_ndcg_at_3_std
value: 41.025597406368256
- type: nauc_ndcg_at_5_diff1
value: 16.910185454343036
- type: nauc_ndcg_at_5_max
value: 60.9328683868778
- type: nauc_ndcg_at_5_std
value: 36.70169905857712
- type: nauc_precision_at_1000_diff1
value: -46.374447765983525
- type: nauc_precision_at_1000_max
value: 35.36052337813863
- type: nauc_precision_at_1000_std
value: 14.219220668161018
- type: nauc_precision_at_100_diff1
value: -29.7838083657744
- type: nauc_precision_at_100_max
value: 43.93589400385112
- type: nauc_precision_at_100_std
value: 55.425045718579945
- type: nauc_precision_at_10_diff1
value: -12.016613405227687
- type: nauc_precision_at_10_max
value: 57.79924427743131
- type: nauc_precision_at_10_std
value: 49.022036703550675
- type: nauc_precision_at_1_diff1
value: 38.62433862433873
- type: nauc_precision_at_1_max
value: 80.78120136943666
- type: nauc_precision_at_1_std
value: -10.768751945222197
- type: nauc_precision_at_20_diff1
value: -23.95633847880195
- type: nauc_precision_at_20_max
value: 48.34715917258276
- type: nauc_precision_at_20_std
value: 48.82198285255887
- type: nauc_precision_at_3_diff1
value: 6.871296905858807
- type: nauc_precision_at_3_max
value: 70.54805793285054
- type: nauc_precision_at_3_std
value: 44.65108624094803
- type: nauc_precision_at_5_diff1
value: -9.074932448759695
- type: nauc_precision_at_5_max
value: 67.41284242437573
- type: nauc_precision_at_5_std
value: 23.876891983919577
- type: nauc_recall_at_1000_diff1
value: 8.142288830293255
- type: nauc_recall_at_1000_max
value: 38.85182826835104
- type: nauc_recall_at_1000_std
value: 68.60783819217335
- type: nauc_recall_at_100_diff1
value: 34.262914076287466
- type: nauc_recall_at_100_max
value: 12.87009658528838
- type: nauc_recall_at_100_std
value: 56.21330603762995
- type: nauc_recall_at_10_diff1
value: 49.33830945338758
- type: nauc_recall_at_10_max
value: 0.3539875530671406
- type: nauc_recall_at_10_std
value: 26.85864465557644
- type: nauc_recall_at_1_diff1
value: 53.48238396620123
- type: nauc_recall_at_1_max
value: 0.33476619393733076
- type: nauc_recall_at_1_std
value: 8.906362219128463
- type: nauc_recall_at_20_diff1
value: 44.21928181266254
- type: nauc_recall_at_20_max
value: -0.9198356057088594
- type: nauc_recall_at_20_std
value: 31.484376992896784
- type: nauc_recall_at_3_diff1
value: 53.038093080990876
- type: nauc_recall_at_3_max
value: -1.4170895916973003
- type: nauc_recall_at_3_std
value: 21.890202855574497
- type: nauc_recall_at_5_diff1
value: 49.39742214825278
- type: nauc_recall_at_5_max
value: 2.8412267611894517
- type: nauc_recall_at_5_std
value: 18.01598921859512
- type: ndcg_at_1
value: 91.0
- type: ndcg_at_10
value: 85.206
- type: ndcg_at_100
value: 67.29
- type: ndcg_at_1000
value: 60.584
- type: ndcg_at_20
value: 82.321
- type: ndcg_at_3
value: 88.642
- type: ndcg_at_5
value: 87.063
- type: precision_at_1
value: 94.0
- type: precision_at_10
value: 89.8
- type: precision_at_100
value: 69.78
- type: precision_at_1000
value: 26.738
- type: precision_at_20
value: 87.2
- type: precision_at_3
value: 92.0
- type: precision_at_5
value: 90.8
- type: recall_at_1
value: 0.246
- type: recall_at_10
value: 2.344
- type: recall_at_100
value: 16.962
- type: recall_at_1000
value: 57.325
- type: recall_at_20
value: 4.517
- type: recall_at_3
value: 0.731
- type: recall_at_5
value: 1.1780000000000002
task:
type: Retrieval
- dataset:
config: default
name: MTEB Touche2020
revision: a34f9a33db75fa0cbb21bb5cfc3dae8dc8bec93f
split: test
type: mteb/touche2020
metrics:
- type: main_score
value: 31.455
- type: map_at_1
value: 2.9739999999999998
- type: map_at_10
value: 12.183
- type: map_at_100
value: 18.772
- type: map_at_1000
value: 20.415
- type: map_at_20
value: 14.451
- type: map_at_3
value: 6.507000000000001
- type: map_at_5
value: 8.66
- type: mrr_at_1
value: 40.816326530612244
- type: mrr_at_10
value: 57.70975056689341
- type: mrr_at_100
value: 58.18379126542391
- type: mrr_at_1000
value: 58.18379126542391
- type: mrr_at_20
value: 57.85552316164561
- type: mrr_at_3
value: 54.08163265306123
- type: mrr_at_5
value: 56.42857142857143
- type: nauc_map_at_1000_diff1
value: 3.1567471051481437
- type: nauc_map_at_1000_max
value: -1.5882060729791523
- type: nauc_map_at_1000_std
value: 18.69622198722074
- type: nauc_map_at_100_diff1
value: 3.3449677678147536
- type: nauc_map_at_100_max
value: -2.8928606866168405
- type: nauc_map_at_100_std
value: 15.789984947653412
- type: nauc_map_at_10_diff1
value: 2.9696743570444264
- type: nauc_map_at_10_max
value: -9.096749212011876
- type: nauc_map_at_10_std
value: -5.38545817258353
- type: nauc_map_at_1_diff1
value: 20.680780404542546
- type: nauc_map_at_1_max
value: -7.04722927447817
- type: nauc_map_at_1_std
value: -7.062494733973898
- type: nauc_map_at_20_diff1
value: 4.070437790119271
- type: nauc_map_at_20_max
value: -4.84491434686032
- type: nauc_map_at_20_std
value: 0.5846341109021014
- type: nauc_map_at_3_diff1
value: 11.9634978045925
- type: nauc_map_at_3_max
value: -8.27834591046608
- type: nauc_map_at_3_std
value: -8.687615453381065
- type: nauc_map_at_5_diff1
value: 0.9195191526009436
- type: nauc_map_at_5_max
value: -1.673813362719489
- type: nauc_map_at_5_std
value: -6.67549753473631
- type: nauc_mrr_at_1000_diff1
value: 19.877993208719573
- type: nauc_mrr_at_1000_max
value: -10.37776706406218
- type: nauc_mrr_at_1000_std
value: 7.132169578056367
- type: nauc_mrr_at_100_diff1
value: 19.877993208719573
- type: nauc_mrr_at_100_max
value: -10.37776706406218
- type: nauc_mrr_at_100_std
value: 7.132169578056367
- type: nauc_mrr_at_10_diff1
value: 20.414285568401457
- type: nauc_mrr_at_10_max
value: -9.677800295687861
- type: nauc_mrr_at_10_std
value: 8.001103690180859
- type: nauc_mrr_at_1_diff1
value: 22.393284073955723
- type: nauc_mrr_at_1_max
value: -5.889370191243167
- type: nauc_mrr_at_1_std
value: -1.5183536173658247
- type: nauc_mrr_at_20_diff1
value: 20.455564720604055
- type: nauc_mrr_at_20_max
value: -10.230642830103074
- type: nauc_mrr_at_20_std
value: 7.863582453266621
- type: nauc_mrr_at_3_diff1
value: 17.554895390732618
- type: nauc_mrr_at_3_max
value: -15.618463505555052
- type: nauc_mrr_at_3_std
value: 5.913231577966864
- type: nauc_mrr_at_5_diff1
value: 18.393678507779914
- type: nauc_mrr_at_5_max
value: -11.903593353147762
- type: nauc_mrr_at_5_std
value: 7.580745996262831
- type: nauc_ndcg_at_1000_diff1
value: 13.746937095530473
- type: nauc_ndcg_at_1000_max
value: -0.9319249687895838
- type: nauc_ndcg_at_1000_std
value: 38.56328031451904
- type: nauc_ndcg_at_100_diff1
value: 13.854865944415895
- type: nauc_ndcg_at_100_max
value: -7.142142012591404
- type: nauc_ndcg_at_100_std
value: 35.61341954818848
- type: nauc_ndcg_at_10_diff1
value: 9.010144273248759
- type: nauc_ndcg_at_10_max
value: -15.320014897424574
- type: nauc_ndcg_at_10_std
value: 2.84883880489144
- type: nauc_ndcg_at_1_diff1
value: 20.939533945592967
- type: nauc_ndcg_at_1_max
value: -6.387319972188946
- type: nauc_ndcg_at_1_std
value: -0.5258673122126726
- type: nauc_ndcg_at_20_diff1
value: 14.660827309009496
- type: nauc_ndcg_at_20_max
value: -13.476196120145994
- type: nauc_ndcg_at_20_std
value: 8.22391881710838
- type: nauc_ndcg_at_3_diff1
value: 13.429985227235935
- type: nauc_ndcg_at_3_max
value: -14.904544592570247
- type: nauc_ndcg_at_3_std
value: 1.599779998183342
- type: nauc_ndcg_at_5_diff1
value: 8.085466231900622
- type: nauc_ndcg_at_5_max
value: -9.09591969526831
- type: nauc_ndcg_at_5_std
value: 3.5794092637248505
- type: nauc_precision_at_1000_diff1
value: -9.31941215946743
- type: nauc_precision_at_1000_max
value: 31.52913520470716
- type: nauc_precision_at_1000_std
value: 22.720784312185856
- type: nauc_precision_at_100_diff1
value: 8.958548406995279
- type: nauc_precision_at_100_max
value: 15.100597910674104
- type: nauc_precision_at_100_std
value: 71.04548238175113
- type: nauc_precision_at_10_diff1
value: 12.4698194690008
- type: nauc_precision_at_10_max
value: -15.84870544871496
- type: nauc_precision_at_10_std
value: 7.575297622501928
- type: nauc_precision_at_1_diff1
value: 22.393284073955723
- type: nauc_precision_at_1_max
value: -5.889370191243167
- type: nauc_precision_at_1_std
value: -1.5183536173658247
- type: nauc_precision_at_20_diff1
value: 15.393505718138758
- type: nauc_precision_at_20_max
value: -3.70684298539384
- type: nauc_precision_at_20_std
value: 29.426137824970304
- type: nauc_precision_at_3_diff1
value: 9.997768085465394
- type: nauc_precision_at_3_max
value: -17.12224314347674
- type: nauc_precision_at_3_std
value: -1.343018166772313
- type: nauc_precision_at_5_diff1
value: 3.8936997437913554
- type: nauc_precision_at_5_max
value: -5.689104289687632
- type: nauc_precision_at_5_std
value: 3.181098051304285
- type: nauc_recall_at_1000_diff1
value: 9.908303508158387
- type: nauc_recall_at_1000_max
value: 6.174506592699848
- type: nauc_recall_at_1000_std
value: 77.41931114780012
- type: nauc_recall_at_100_diff1
value: 10.286839241876192
- type: nauc_recall_at_100_max
value: -6.6138697026666815
- type: nauc_recall_at_100_std
value: 49.608313692633224
- type: nauc_recall_at_10_diff1
value: 2.215545846659851
- type: nauc_recall_at_10_max
value: -17.83025802478445
- type: nauc_recall_at_10_std
value: -3.3784768673705465
- type: nauc_recall_at_1_diff1
value: 20.680780404542546
- type: nauc_recall_at_1_max
value: -7.04722927447817
- type: nauc_recall_at_1_std
value: -7.062494733973898
- type: nauc_recall_at_20_diff1
value: 6.974410239251615
- type: nauc_recall_at_20_max
value: -14.161147924731646
- type: nauc_recall_at_20_std
value: 9.328412057721454
- type: nauc_recall_at_3_diff1
value: 7.904589805754212
- type: nauc_recall_at_3_max
value: -12.1912388648593
- type: nauc_recall_at_3_std
value: -9.221542013385555
- type: nauc_recall_at_5_diff1
value: -3.2604132752706914
- type: nauc_recall_at_5_max
value: -6.886351441658915
- type: nauc_recall_at_5_std
value: -7.014252851712789
- type: ndcg_at_1
value: 39.796
- type: ndcg_at_10
value: 31.455
- type: ndcg_at_100
value: 42.388999999999996
- type: ndcg_at_1000
value: 53.556000000000004
- type: ndcg_at_20
value: 30.808000000000003
- type: ndcg_at_3
value: 35.831
- type: ndcg_at_5
value: 32.845
- type: precision_at_1
value: 40.816
- type: precision_at_10
value: 27.143
- type: precision_at_100
value: 8.449
- type: precision_at_1000
value: 1.6179999999999999
- type: precision_at_20
value: 19.387999999999998
- type: precision_at_3
value: 35.374
- type: precision_at_5
value: 31.019999999999996
- type: recall_at_1
value: 2.9739999999999998
- type: recall_at_10
value: 19.39
- type: recall_at_100
value: 51.636
- type: recall_at_1000
value: 86.99900000000001
- type: recall_at_20
value: 26.478
- type: recall_at_3
value: 7.703
- type: recall_at_5
value: 11.42
task:
type: Retrieval
- dataset:
config: default
name: MTEB ToxicConversationsClassification
revision: edfaf9da55d3dd50d43143d90c1ac476895ae6de
split: test
type: mteb/toxic_conversations_50k
metrics:
- type: accuracy
value: 86.9384765625
- type: ap
value: 31.737513704141552
- type: ap_weighted
value: 31.737513704141552
- type: f1
value: 71.5490757306975
- type: f1_weighted
value: 89.14632533489856
- type: main_score
value: 86.9384765625
task:
type: Classification
- dataset:
config: default
name: MTEB TweetSentimentExtractionClassification
revision: d604517c81ca91fe16a244d1248fc021f9ecee7a
split: test
type: mteb/tweet_sentiment_extraction
metrics:
- type: accuracy
value: 73.57668364459535
- type: f1
value: 73.90467103648074
- type: f1_weighted
value: 73.42158415034704
- type: main_score
value: 73.57668364459535
task:
type: Classification
- dataset:
config: default
name: MTEB TwentyNewsgroupsClustering
revision: 6125ec4e24fa026cec8a478383ee943acfbd5449
split: test
type: mteb/twentynewsgroups-clustering
metrics:
- type: main_score
value: 58.574148097494685
- type: v_measure
value: 58.574148097494685
- type: v_measure_std
value: 0.9443161637490822
task:
type: Clustering
- dataset:
config: default
name: MTEB TwitterSemEval2015
revision: 70970daeab8776df92f5ea462b6173c0b46fd2d1
split: test
type: mteb/twittersemeval2015-pairclassification
metrics:
- type: cosine_accuracy
value: 88.1385229778864
- type: cosine_accuracy_threshold
value: 83.86307954788208
- type: cosine_ap
value: 80.17965893449055
- type: cosine_f1
value: 73.0614300100705
- type: cosine_f1_threshold
value: 80.7942807674408
- type: cosine_precision
value: 69.8603755416466
- type: cosine_recall
value: 76.56992084432717
- type: dot_accuracy
value: 88.2100494724921
- type: dot_accuracy_threshold
value: 83.84793996810913
- type: dot_ap
value: 80.18603932881858
- type: dot_f1
value: 73.07643714466204
- type: dot_f1_threshold
value: 80.87586164474487
- type: dot_precision
value: 70.10909090909091
- type: dot_recall
value: 76.3060686015831
- type: euclidean_accuracy
value: 88.1385229778864
- type: euclidean_accuracy_threshold
value: 56.77661895751953
- type: euclidean_ap
value: 80.1784070881624
- type: euclidean_f1
value: 73.04830369529574
- type: euclidean_f1_threshold
value: 61.91838979721069
- type: euclidean_precision
value: 69.96859144720948
- type: euclidean_recall
value: 76.41160949868075
- type: main_score
value: 80.18603932881858
- type: manhattan_accuracy
value: 88.0431543184121
- type: manhattan_accuracy_threshold
value: 3755.6137084960938
- type: manhattan_ap
value: 79.98270453664578
- type: manhattan_f1
value: 72.68242015061023
- type: manhattan_f1_threshold
value: 3892.494583129883
- type: manhattan_precision
value: 71.54907975460122
- type: manhattan_recall
value: 73.85224274406332
- type: max_ap
value: 80.18603932881858
- type: max_f1
value: 73.07643714466204
- type: max_precision
value: 71.54907975460122
- type: max_recall
value: 76.56992084432717
- type: similarity_accuracy
value: 88.1385229778864
- type: similarity_accuracy_threshold
value: 83.86307954788208
- type: similarity_ap
value: 80.17965893449055
- type: similarity_f1
value: 73.0614300100705
- type: similarity_f1_threshold
value: 80.7942807674408
- type: similarity_precision
value: 69.8603755416466
- type: similarity_recall
value: 76.56992084432717
task:
type: PairClassification
- dataset:
config: default
name: MTEB TwitterURLCorpus
revision: 8b6510b0b1fa4e4c4f879467980e9be563ec1cdf
split: test
type: mteb/twitterurlcorpus-pairclassification
metrics:
- type: cosine_accuracy
value: 89.7892653393876
- type: cosine_accuracy_threshold
value: 79.69566583633423
- type: cosine_ap
value: 87.4579867302024
- type: cosine_f1
value: 79.91620843152658
- type: cosine_f1_threshold
value: 78.53609323501587
- type: cosine_precision
value: 77.7155329210622
- type: cosine_recall
value: 82.24514936864799
- type: dot_accuracy
value: 89.78732487289945
- type: dot_accuracy_threshold
value: 80.05315661430359
- type: dot_ap
value: 87.44916182456272
- type: dot_f1
value: 79.90419878751591
- type: dot_f1_threshold
value: 78.57890725135803
- type: dot_precision
value: 77.73409057812728
- type: dot_recall
value: 82.19895287958116
- type: euclidean_accuracy
value: 89.78538440641131
- type: euclidean_accuracy_threshold
value: 62.29925751686096
- type: euclidean_ap
value: 87.45904868911386
- type: euclidean_f1
value: 79.93127404474657
- type: euclidean_f1_threshold
value: 65.61101078987122
- type: euclidean_precision
value: 77.62060210373595
- type: euclidean_recall
value: 82.38373883584848
- type: main_score
value: 87.46554314325058
- type: manhattan_accuracy
value: 89.76597974152986
- type: manhattan_accuracy_threshold
value: 3988.5299682617188
- type: manhattan_ap
value: 87.46554314325058
- type: manhattan_f1
value: 79.97181740645973
- type: manhattan_f1_threshold
value: 4235.905838012695
- type: manhattan_precision
value: 77.13713427283783
- type: manhattan_recall
value: 83.02279026793964
- type: max_ap
value: 87.46554314325058
- type: max_f1
value: 79.97181740645973
- type: max_precision
value: 77.73409057812728
- type: max_recall
value: 83.02279026793964
- type: similarity_accuracy
value: 89.7892653393876
- type: similarity_accuracy_threshold
value: 79.69566583633423
- type: similarity_ap
value: 87.4579867302024
- type: similarity_f1
value: 79.91620843152658
- type: similarity_f1_threshold
value: 78.53609323501587
- type: similarity_precision
value: 77.7155329210622
- type: similarity_recall
value: 82.24514936864799
task:
type: PairClassification
tags:
- mteb
- sentence-transformers
- transformers
- sentence-similarity
license: mit
---
# Updates
New open-source models and ToDoList will be listed on https://github.com/DunZhang/Stella/blob/main/news_and_todo.md.
You can also find these models on my [homepage](https://huggingface.co/infgrad).
# Introduction
The models are trained based on `Alibaba-NLP/gte-large-en-v1.5` and `Alibaba-NLP/gte-Qwen2-1.5B-instruct`. Thanks for
their contributions!
**We simplify usage of prompts, providing two prompts for most general tasks, one is for s2p, another one is for s2s.**
Prompt of s2p task(e.g. retrieve task):
```text
Instruct: Given a web search query, retrieve relevant passages that answer the query.\nQuery: {query}
```
Prompt of s2s task(e.g. semantic textual similarity task):
```text
Instruct: Retrieve semantically similar text.\nQuery: {query}
```
The models are finally trained by [MRL](https://arxiv.org/abs/2205.13147), so they have multiple dimensions: 512, 768,
1024, 2048, 4096, 6144 and 8192.
The higher the dimension, the better the performance.
**Generally speaking, 1024d is good enough.** The MTEB score of 1024d is only 0.001 lower than 8192d.
# Model directory structure
The model directory structure is very simple, it is a standard SentenceTransformer directory **with a series
of `2_Dense_{dims}`
folders**, where `dims` represents the final vector dimension.
For example, the `2_Dense_256` folder stores Linear weights that convert vector dimensions to 256 dimensions.
Please refer to the following chapters for specific instructions on how to use them.
# Usage
You can use `SentenceTransformers` or `transformers` library to encode text.
## Sentence Transformers
```python
from sentence_transformers import SentenceTransformer
# This model supports two prompts: "s2p_query" and "s2s_query" for sentence-to-passage and sentence-to-sentence tasks, respectively.
# They are defined in `config_sentence_transformers.json`
query_prompt_name = "s2p_query"
queries = [
"What are some ways to reduce stress?",
"What are the benefits of drinking green tea?",
]
# docs do not need any prompts
docs = [
"There are many effective ways to reduce stress. Some common techniques include deep breathing, meditation, and physical activity. Engaging in hobbies, spending time in nature, and connecting with loved ones can also help alleviate stress. Additionally, setting boundaries, practicing self-care, and learning to say no can prevent stress from building up.",
"Green tea has been consumed for centuries and is known for its potential health benefits. It contains antioxidants that may help protect the body against damage caused by free radicals. Regular consumption of green tea has been associated with improved heart health, enhanced cognitive function, and a reduced risk of certain types of cancer. The polyphenols in green tea may also have anti-inflammatory and weight loss properties.",
]
# !The default dimension is 1024, if you need other dimensions, please clone the model and modify `modules.json` to replace `2_Dense_1024` with another dimension, e.g. `2_Dense_256` or `2_Dense_8192` !
# on gpu
model = SentenceTransformer("dunzhang/stella_en_400M_v5", trust_remote_code=True).cuda()
# you can also use this model without the features of `use_memory_efficient_attention` and `unpad_inputs`. It can be worked in CPU.
# model = SentenceTransformer(
# "dunzhang/stella_en_400M_v5",
# trust_remote_code=True,
# device="cpu",
# config_kwargs={"use_memory_efficient_attention": False, "unpad_inputs": False}
# )
query_embeddings = model.encode(queries, prompt_name=query_prompt_name)
doc_embeddings = model.encode(docs)
print(query_embeddings.shape, doc_embeddings.shape)
# (2, 1024) (2, 1024)
similarities = model.similarity(query_embeddings, doc_embeddings)
print(similarities)
# tensor([[0.8398, 0.2990],
# [0.3282, 0.8095]])
```
## Transformers
```python
import os
import torch
from transformers import AutoModel, AutoTokenizer
from sklearn.preprocessing import normalize
query_prompt = "Instruct: Given a web search query, retrieve relevant passages that answer the query.\nQuery: "
queries = [
"What are some ways to reduce stress?",
"What are the benefits of drinking green tea?",
]
queries = [query_prompt + query for query in queries]
# docs do not need any prompts
docs = [
"There are many effective ways to reduce stress. Some common techniques include deep breathing, meditation, and physical activity. Engaging in hobbies, spending time in nature, and connecting with loved ones can also help alleviate stress. Additionally, setting boundaries, practicing self-care, and learning to say no can prevent stress from building up.",
"Green tea has been consumed for centuries and is known for its potential health benefits. It contains antioxidants that may help protect the body against damage caused by free radicals. Regular consumption of green tea has been associated with improved heart health, enhanced cognitive function, and a reduced risk of certain types of cancer. The polyphenols in green tea may also have anti-inflammatory and weight loss properties.",
]
# The path of your model after cloning it
model_dir = "{Your MODEL_PATH}"
vector_dim = 1024
vector_linear_directory = f"2_Dense_{vector_dim}"
model = AutoModel.from_pretrained(model_dir, trust_remote_code=True).cuda().eval()
# you can also use this model without the features of `use_memory_efficient_attention` and `unpad_inputs`. It can be worked in CPU.
# model = AutoModel.from_pretrained(model_dir, trust_remote_code=True,use_memory_efficient_attention=False,unpad_inputs=False).cuda().eval()
tokenizer = AutoTokenizer.from_pretrained(model_dir, trust_remote_code=True)
vector_linear = torch.nn.Linear(in_features=model.config.hidden_size, out_features=vector_dim)
vector_linear_dict = {
k.replace("linear.", ""): v for k, v in
torch.load(os.path.join(model_dir, f"{vector_linear_directory}/pytorch_model.bin")).items()
}
vector_linear.load_state_dict(vector_linear_dict)
vector_linear.cuda()
# Embed the queries
with torch.no_grad():
input_data = tokenizer(queries, padding="longest", truncation=True, max_length=512, return_tensors="pt")
input_data = {k: v.cuda() for k, v in input_data.items()}
attention_mask = input_data["attention_mask"]
last_hidden_state = model(**input_data)[0]
last_hidden = last_hidden_state.masked_fill(~attention_mask[..., None].bool(), 0.0)
query_vectors = last_hidden.sum(dim=1) / attention_mask.sum(dim=1)[..., None]
query_vectors = normalize(vector_linear(query_vectors).cpu().numpy())
# Embed the documents
with torch.no_grad():
input_data = tokenizer(docs, padding="longest", truncation=True, max_length=512, return_tensors="pt")
input_data = {k: v.cuda() for k, v in input_data.items()}
attention_mask = input_data["attention_mask"]
last_hidden_state = model(**input_data)[0]
last_hidden = last_hidden_state.masked_fill(~attention_mask[..., None].bool(), 0.0)
docs_vectors = last_hidden.sum(dim=1) / attention_mask.sum(dim=1)[..., None]
docs_vectors = normalize(vector_linear(docs_vectors).cpu().numpy())
print(query_vectors.shape, docs_vectors.shape)
# (2, 1024) (2, 1024)
similarities = query_vectors @ docs_vectors.T
print(similarities)
# [[0.8397531 0.29900077]
# [0.32818374 0.80954516]]
```
# FAQ
Q: The details of training?
A: The training method and datasets will be released in the future. (specific time unknown, may be provided in a paper)
Q: How to choose a suitable prompt for my own task?
A: In most cases, please use the s2p and s2s prompts. These two prompts account for the vast majority of the training
data.
Q: How to reproduce MTEB results?
A: Please use evaluation scripts in `Alibaba-NLP/gte-Qwen2-1.5B-instruct` or `intfloat/e5-mistral-7b-instruct`
Q: Why each dimension has a linear weight?
A: MRL has multiple training methods, we choose this method which has the best performance.
Q: What is the sequence length of models?
A: 512 is recommended, in our experiments, almost all models perform poorly on specialized long text retrieval datasets. Besides, the
model is trained on datasets of 512 length. This may be an optimization term.
If you have any questions, please start a discussion on community. |
charactr/vocos-mel-24khz | charactr | "2023-10-17T14:08:53Z" | 1,423,307 | 11 | null | [
"pytorch",
"arxiv:2306.00814",
"license:mit",
"region:us"
] | null | "2023-06-11T16:38:37Z" | ---
license: mit
---
# Vocos: Closing the gap between time-domain and Fourier-based neural vocoders for high-quality audio synthesis
[Audio samples](https://charactr-platform.github.io/vocos/) |
Paper [[abs]](https://arxiv.org/abs/2306.00814) [[pdf]](https://arxiv.org/pdf/2306.00814.pdf)
Vocos is a fast neural vocoder designed to synthesize audio waveforms from acoustic features. Trained using a Generative
Adversarial Network (GAN) objective, Vocos can generate waveforms in a single forward pass. Unlike other typical
GAN-based vocoders, Vocos does not model audio samples in the time domain. Instead, it generates spectral
coefficients, facilitating rapid audio reconstruction through inverse Fourier transform.
## Installation
To use Vocos only in inference mode, install it using:
```bash
pip install vocos
```
If you wish to train the model, install it with additional dependencies:
```bash
pip install vocos[train]
```
## Usage
### Reconstruct audio from mel-spectrogram
```python
import torch
from vocos import Vocos
vocos = Vocos.from_pretrained("charactr/vocos-mel-24khz")
mel = torch.randn(1, 100, 256) # B, C, T
audio = vocos.decode(mel)
```
Copy-synthesis from a file:
```python
import torchaudio
y, sr = torchaudio.load(YOUR_AUDIO_FILE)
if y.size(0) > 1: # mix to mono
y = y.mean(dim=0, keepdim=True)
y = torchaudio.functional.resample(y, orig_freq=sr, new_freq=24000)
y_hat = vocos(y)
```
## Citation
If this code contributes to your research, please cite our work:
```
@article{siuzdak2023vocos,
title={Vocos: Closing the gap between time-domain and Fourier-based neural vocoders for high-quality audio synthesis},
author={Siuzdak, Hubert},
journal={arXiv preprint arXiv:2306.00814},
year={2023}
}
```
## License
The code in this repository is released under the MIT license. |
coqui/XTTS-v2 | coqui | "2023-12-11T17:50:00Z" | 1,402,967 | 1,946 | coqui | [
"coqui",
"text-to-speech",
"license:other",
"region:us"
] | text-to-speech | "2023-10-31T10:11:33Z" | ---
license: other
license_name: coqui-public-model-license
license_link: https://coqui.ai/cpml
library_name: coqui
pipeline_tag: text-to-speech
widget:
- text: "Once when I was six years old I saw a magnificent picture"
---
# ⓍTTS
ⓍTTS is a Voice generation model that lets you clone voices into different languages by using just a quick 6-second audio clip. There is no need for an excessive amount of training data that spans countless hours.
This is the same or similar model to what powers [Coqui Studio](https://coqui.ai/) and [Coqui API](https://docs.coqui.ai/docs).
### Features
- Supports 17 languages.
- Voice cloning with just a 6-second audio clip.
- Emotion and style transfer by cloning.
- Cross-language voice cloning.
- Multi-lingual speech generation.
- 24khz sampling rate.
### Updates over XTTS-v1
- 2 new languages; Hungarian and Korean
- Architectural improvements for speaker conditioning.
- Enables the use of multiple speaker references and interpolation between speakers.
- Stability improvements.
- Better prosody and audio quality across the board.
### Languages
XTTS-v2 supports 17 languages: **English (en), Spanish (es), French (fr), German (de), Italian (it), Portuguese (pt),
Polish (pl), Turkish (tr), Russian (ru), Dutch (nl), Czech (cs), Arabic (ar), Chinese (zh-cn), Japanese (ja), Hungarian (hu), Korean (ko)
Hindi (hi)**.
Stay tuned as we continue to add support for more languages. If you have any language requests, feel free to reach out!
### Code
The [code-base](https://github.com/coqui-ai/TTS) supports inference and [fine-tuning](https://tts.readthedocs.io/en/latest/models/xtts.html#training).
### Demo Spaces
- [XTTS Space](https://huggingface.co/spaces/coqui/xtts) : You can see how model performs on supported languages, and try with your own reference or microphone input
- [XTTS Voice Chat with Mistral or Zephyr](https://huggingface.co/spaces/coqui/voice-chat-with-mistral) : You can experience streaming voice chat with Mistral 7B Instruct or Zephyr 7B Beta
| | |
| ------------------------------- | --------------------------------------- |
| 🐸💬 **CoquiTTS** | [coqui/TTS on Github](https://github.com/coqui-ai/TTS)|
| 💼 **Documentation** | [ReadTheDocs](https://tts.readthedocs.io/en/latest/)
| 👩💻 **Questions** | [GitHub Discussions](https://github.com/coqui-ai/TTS/discussions) |
| 🗯 **Community** | [Discord](https://discord.gg/5eXr5seRrv) |
### License
This model is licensed under [Coqui Public Model License](https://coqui.ai/cpml). There's a lot that goes into a license for generative models, and you can read more of [the origin story of CPML here](https://coqui.ai/blog/tts/cpml).
### Contact
Come and join in our 🐸Community. We're active on [Discord](https://discord.gg/fBC58unbKE) and [Twitter](https://twitter.com/coqui_ai).
You can also mail us at [email protected].
Using 🐸TTS API:
```python
from TTS.api import TTS
tts = TTS("tts_models/multilingual/multi-dataset/xtts_v2", gpu=True)
# generate speech by cloning a voice using default settings
tts.tts_to_file(text="It took me quite a long time to develop a voice, and now that I have it I'm not going to be silent.",
file_path="output.wav",
speaker_wav="/path/to/target/speaker.wav",
language="en")
```
Using 🐸TTS Command line:
```console
tts --model_name tts_models/multilingual/multi-dataset/xtts_v2 \
--text "Bugün okula gitmek istemiyorum." \
--speaker_wav /path/to/target/speaker.wav \
--language_idx tr \
--use_cuda true
```
Using the model directly:
```python
from TTS.tts.configs.xtts_config import XttsConfig
from TTS.tts.models.xtts import Xtts
config = XttsConfig()
config.load_json("/path/to/xtts/config.json")
model = Xtts.init_from_config(config)
model.load_checkpoint(config, checkpoint_dir="/path/to/xtts/", eval=True)
model.cuda()
outputs = model.synthesize(
"It took me quite a long time to develop a voice and now that I have it I am not going to be silent.",
config,
speaker_wav="/data/TTS-public/_refclips/3.wav",
gpt_cond_len=3,
language="en",
)
```
|
prithivida/parrot_paraphraser_on_T5 | prithivida | "2021-05-18T07:53:27Z" | 1,396,856 | 141 | transformers | [
"transformers",
"pytorch",
"t5",
"text2text-generation",
"autotrain_compatible",
"text-generation-inference",
"endpoints_compatible",
"region:us"
] | text2text-generation | "2022-03-02T23:29:05Z" | # Parrot
## 1. What is Parrot?
Parrot is a paraphrase based utterance augmentation framework purpose built to accelerate training NLU models. A paraphrase framework is more than just a paraphrasing model. For more details on the library and usage please refer to the [github page](https://github.com/PrithivirajDamodaran/Parrot)
### Installation
```python
pip install git+https://github.com/PrithivirajDamodaran/Parrot_Paraphraser.git
```
### Quickstart
```python
from parrot import Parrot
import torch
import warnings
warnings.filterwarnings("ignore")
'''
uncomment to get reproducable paraphrase generations
def random_state(seed):
torch.manual_seed(seed)
if torch.cuda.is_available():
torch.cuda.manual_seed_all(seed)
random_state(1234)
'''
#Init models (make sure you init ONLY once if you integrate this to your code)
parrot = Parrot(model_tag="prithivida/parrot_paraphraser_on_T5", use_gpu=False)
phrases = ["Can you recommed some upscale restaurants in Newyork?",
"What are the famous places we should not miss in Russia?"
]
for phrase in phrases:
print("-"*100)
print("Input_phrase: ", phrase)
print("-"*100)
para_phrases = parrot.augment(input_phrase=phrase)
for para_phrase in para_phrases:
print(para_phrase)
```
```
----------------------------------------------------------------------
Input_phrase: Can you recommed some upscale restaurants in Newyork?
----------------------------------------------------------------------
list some excellent restaurants to visit in new york city?
what upscale restaurants do you recommend in new york?
i want to try some upscale restaurants in new york?
recommend some upscale restaurants in newyork?
can you recommend some high end restaurants in newyork?
can you recommend some upscale restaurants in new york?
can you recommend some upscale restaurants in newyork?
----------------------------------------------------------------------
Input_phrase: What are the famous places we should not miss in Russia
----------------------------------------------------------------------
what should we not miss when visiting russia?
recommend some of the best places to visit in russia?
list some of the best places to visit in russia?
can you list the top places to visit in russia?
show the places that we should not miss in russia?
list some famous places which we should not miss in russia?
```
### Knobs
```python
para_phrases = parrot.augment(input_phrase=phrase,
diversity_ranker="levenshtein",
do_diverse=False,
max_return_phrases = 10,
max_length=32,
adequacy_threshold = 0.99,
fluency_threshold = 0.90)
```
## 2. Why Parrot?
**Huggingface** lists [12 paraphrase models,](https://huggingface.co/models?pipeline_tag=text2text-generation&search=paraphrase) **RapidAPI** lists 7 fremium and commercial paraphrasers like [QuillBot](https://rapidapi.com/search/paraphrase?section=apis&page=1), Rasa has discussed an experimental paraphraser for augmenting text data [here](https://forum.rasa.com/t/paraphrasing-for-nlu-data-augmentation-experimental/27744), Sentence-transfomers offers a [paraphrase mining utility](https://www.sbert.net/examples/applications/paraphrase-mining/README.html) and [NLPAug](https://github.com/makcedward/nlpaug) offers word level augmentation with a [PPDB](http://paraphrase.org/#/download) (a multi-million paraphrase database). While these attempts at paraphrasing are great, there are still some gaps and paraphrasing is NOT yet a mainstream option for text augmentation in building NLU models....Parrot is a humble attempt to fill some of these gaps.
**What is a good paraphrase?** Almost all conditioned text generation models are validated on 2 factors, (1) if the generated text conveys the same meaning as the original context (Adequacy) (2) if the text is fluent / grammatically correct english (Fluency). For instance Neural Machine Translation outputs are tested for Adequacy and Fluency. But [a good paraphrase](https://www.aclweb.org/anthology/D10-1090.pdf) should be adequate and fluent while being as different as possible on the surface lexical form. With respect to this definition, the **3 key metrics** that measures the quality of paraphrases are:
- **Adequacy** (Is the meaning preserved adequately?)
- **Fluency** (Is the paraphrase fluent English?)
- **Diversity (Lexical / Phrasal / Syntactical)** (How much has the paraphrase changed the original sentence?)
*Parrot offers knobs to control Adequacy, Fluency and Diversity as per your needs.*
**What makes a paraphraser a good augmentor?** For training a NLU model we just don't need a lot of utterances but utterances with intents and slots/entities annotated. Typical flow would be:
- Given an **input utterance + input annotations** a good augmentor spits out N **output paraphrases** while preserving the intent and slots.
- The output paraphrases are then converted into annotated data using the input annotations that we got in step 1.
- The annotated data created out of the output paraphrases then makes the training dataset for your NLU model.
But in general being a generative model paraphrasers doesn't guarantee to preserve the slots/entities. So the ability to generate high quality paraphrases in a constrained fashion without trading off the intents and slots for lexical dissimilarity makes a paraphraser a good augmentor. *More on this in section 3 below*
## 3. Scope
In the space of conversational engines, knowledge bots are to which **we ask questions** like *"when was the Berlin wall teared down?"*, transactional bots are to which **we give commands** like *"Turn on the music please"* and voice assistants are the ones which can do both answer questions and action our commands. Parrot mainly foucses on augmenting texts typed-into or spoken-to conversational interfaces for building robust NLU models. (*So usually people neither type out or yell out long paragraphs to conversational interfaces. Hence the pre-trained model is trained on text samples of maximum length of 32.*)
*While Parrot predominantly aims to be a text augmentor for building good NLU models, it can also be used as a pure-play paraphraser.*
|
llava-hf/llava-v1.6-mistral-7b-hf | llava-hf | "2024-10-21T10:19:34Z" | 1,389,909 | 231 | transformers | [
"transformers",
"safetensors",
"llava_next",
"image-text-to-text",
"vision",
"conversational",
"en",
"arxiv:2310.03744",
"license:apache-2.0",
"text-generation-inference",
"endpoints_compatible",
"region:us"
] | image-text-to-text | "2024-02-20T08:01:48Z" | ---
license: apache-2.0
tags:
- vision
- image-text-to-text
language:
- en
pipeline_tag: image-text-to-text
---
# LLaVa-Next, leveraging [mistralai/Mistral-7B-Instruct-v0.2](https://huggingface.co/mistralai/Mistral-7B-Instruct-v0.2) as LLM
The LLaVA-NeXT model was proposed in [LLaVA-NeXT: Improved reasoning, OCR, and world knowledge](https://llava-vl.github.io/blog/2024-01-30-llava-next/) by Haotian Liu, Chunyuan Li, Yuheng Li, Bo Li, Yuanhan Zhang, Sheng Shen, Yong Jae Lee. LLaVa-NeXT (also called LLaVa-1.6) improves upon [LLaVa-1.5](https://huggingface.co/transformers/main/model_doc/llava.html) by increasing the input image resolution and training on an improved visual instruction tuning dataset to improve OCR and common sense reasoning.
Disclaimer: The team releasing LLaVa-NeXT did not write a model card for this model so this model card has been written by the Hugging Face team.
## Model description
LLaVa combines a pre-trained large language model with a pre-trained vision encoder for multimodal chatbot use cases. LLaVA 1.6 improves on LLaVA 1.5 BY:
- Using [Mistral-7B](https://mistral.ai/news/announcing-mistral-7b/) (for this checkpoint) and [Nous-Hermes-2-Yi-34B](https://huggingface.co/NousResearch/Nous-Hermes-2-Yi-34B) which has better commercial licenses,
and bilingual support
- More diverse and high quality data mixture
- Dynamic high resolution

## Intended uses & limitations
You can use the raw model for tasks like image captioning, visual question answering, multimodal chatbot use cases. See the [model hub](https://huggingface.co/models?search=llava-hf) to look for
other versions on a task that interests you.
### How to use
Here's the prompt template for this model:
```
"[INST] <image>\nWhat is shown in this image? [/INST]"
```
You can load and use the model like following:
```python
from transformers import LlavaNextProcessor, LlavaNextForConditionalGeneration
import torch
from PIL import Image
import requests
processor = LlavaNextProcessor.from_pretrained("llava-hf/llava-v1.6-mistral-7b-hf")
model = LlavaNextForConditionalGeneration.from_pretrained("llava-hf/llava-v1.6-mistral-7b-hf", torch_dtype=torch.float16, low_cpu_mem_usage=True)
model.to("cuda:0")
# prepare image and text prompt, using the appropriate prompt template
url = "https://github.com/haotian-liu/LLaVA/blob/1a91fc274d7c35a9b50b3cb29c4247ae5837ce39/images/llava_v1_5_radar.jpg?raw=true"
image = Image.open(requests.get(url, stream=True).raw)
# Define a chat history and use `apply_chat_template` to get correctly formatted prompt
# Each value in "content" has to be a list of dicts with types ("text", "image")
conversation = [
{
"role": "user",
"content": [
{"type": "text", "text": "What is shown in this image?"},
{"type": "image"},
],
},
]
prompt = processor.apply_chat_template(conversation, add_generation_prompt=True)
inputs = processor(images=image, text=prompt, return_tensors="pt").to("cuda:0")
# autoregressively complete prompt
output = model.generate(**inputs, max_new_tokens=100)
print(processor.decode(output[0], skip_special_tokens=True))
```
### Model optimization
#### 4-bit quantization through `bitsandbytes` library
First make sure to install `bitsandbytes`, `pip install bitsandbytes` and make sure to have access to a CUDA compatible GPU device. Simply change the snippet above with:
```diff
model = LlavaNextForConditionalGeneration.from_pretrained(
model_id,
torch_dtype=torch.float16,
low_cpu_mem_usage=True,
+ load_in_4bit=True
)
```
#### Use Flash-Attention 2 to further speed-up generation
First make sure to install `flash-attn`. Refer to the [original repository of Flash Attention](https://github.com/Dao-AILab/flash-attention) regarding that package installation. Simply change the snippet above with:
```diff
model = LlavaNextForConditionalGeneration.from_pretrained(
model_id,
torch_dtype=torch.float16,
low_cpu_mem_usage=True,
+ use_flash_attention_2=True
).to(0)
```
### BibTeX entry and citation info
```bibtex
@misc{liu2023improved,
title={Improved Baselines with Visual Instruction Tuning},
author={Haotian Liu and Chunyuan Li and Yuheng Li and Yong Jae Lee},
year={2023},
eprint={2310.03744},
archivePrefix={arXiv},
primaryClass={cs.CV}
}
``` |
meta-llama/Llama-3.2-1B-Instruct | meta-llama | "2024-10-24T15:07:51Z" | 1,377,758 | 531 | transformers | [
"transformers",
"safetensors",
"llama",
"text-generation",
"facebook",
"meta",
"pytorch",
"llama-3",
"conversational",
"en",
"de",
"fr",
"it",
"pt",
"hi",
"es",
"th",
"arxiv:2204.05149",
"arxiv:2405.16406",
"license:llama3.2",
"autotrain_compatible",
"text-generation-inference",
"endpoints_compatible",
"region:us"
] | text-generation | "2024-09-18T15:12:47Z" | ---
language:
- en
- de
- fr
- it
- pt
- hi
- es
- th
library_name: transformers
pipeline_tag: text-generation
tags:
- facebook
- meta
- pytorch
- llama
- llama-3
license: llama3.2
extra_gated_prompt: >-
### LLAMA 3.2 COMMUNITY LICENSE AGREEMENT
Llama 3.2 Version Release Date: September 25, 2024
“Agreement” means the terms and conditions for use, reproduction, distribution
and modification of the Llama Materials set forth herein.
“Documentation” means the specifications, manuals and documentation accompanying Llama 3.2
distributed by Meta at https://llama.meta.com/doc/overview.
“Licensee” or “you” means you, or your employer or any other person or entity (if you are
entering into this Agreement on such person or entity’s behalf), of the age required under
applicable laws, rules or regulations to provide legal consent and that has legal authority
to bind your employer or such other person or entity if you are entering in this Agreement
on their behalf.
“Llama 3.2” means the foundational large language models and software and algorithms, including
machine-learning model code, trained model weights, inference-enabling code, training-enabling code,
fine-tuning enabling code and other elements of the foregoing distributed by Meta at
https://www.llama.com/llama-downloads.
“Llama Materials” means, collectively, Meta’s proprietary Llama 3.2 and Documentation (and
any portion thereof) made available under this Agreement.
“Meta” or “we” means Meta Platforms Ireland Limited (if you are located in or,
if you are an entity, your principal place of business is in the EEA or Switzerland)
and Meta Platforms, Inc. (if you are located outside of the EEA or Switzerland).
By clicking “I Accept” below or by using or distributing any portion or element of the Llama Materials,
you agree to be bound by this Agreement.
1. License Rights and Redistribution.
a. Grant of Rights. You are granted a non-exclusive, worldwide,
non-transferable and royalty-free limited license under Meta’s intellectual property or other rights
owned by Meta embodied in the Llama Materials to use, reproduce, distribute, copy, create derivative works
of, and make modifications to the Llama Materials.
b. Redistribution and Use.
i. If you distribute or make available the Llama Materials (or any derivative works thereof),
or a product or service (including another AI model) that contains any of them, you shall (A) provide
a copy of this Agreement with any such Llama Materials; and (B) prominently display “Built with Llama”
on a related website, user interface, blogpost, about page, or product documentation. If you use the
Llama Materials or any outputs or results of the Llama Materials to create, train, fine tune, or
otherwise improve an AI model, which is distributed or made available, you shall also include “Llama”
at the beginning of any such AI model name.
ii. If you receive Llama Materials, or any derivative works thereof, from a Licensee as part
of an integrated end user product, then Section 2 of this Agreement will not apply to you.
iii. You must retain in all copies of the Llama Materials that you distribute the
following attribution notice within a “Notice” text file distributed as a part of such copies:
“Llama 3.2 is licensed under the Llama 3.2 Community License, Copyright © Meta Platforms,
Inc. All Rights Reserved.”
iv. Your use of the Llama Materials must comply with applicable laws and regulations
(including trade compliance laws and regulations) and adhere to the Acceptable Use Policy for
the Llama Materials (available at https://www.llama.com/llama3_2/use-policy), which is hereby
incorporated by reference into this Agreement.
2. Additional Commercial Terms. If, on the Llama 3.2 version release date, the monthly active users
of the products or services made available by or for Licensee, or Licensee’s affiliates,
is greater than 700 million monthly active users in the preceding calendar month, you must request
a license from Meta, which Meta may grant to you in its sole discretion, and you are not authorized to
exercise any of the rights under this Agreement unless or until Meta otherwise expressly grants you such rights.
3. Disclaimer of Warranty. UNLESS REQUIRED BY APPLICABLE LAW, THE LLAMA MATERIALS AND ANY OUTPUT AND
RESULTS THEREFROM ARE PROVIDED ON AN “AS IS” BASIS, WITHOUT WARRANTIES OF ANY KIND, AND META DISCLAIMS
ALL WARRANTIES OF ANY KIND, BOTH EXPRESS AND IMPLIED, INCLUDING, WITHOUT LIMITATION, ANY WARRANTIES
OF TITLE, NON-INFRINGEMENT, MERCHANTABILITY, OR FITNESS FOR A PARTICULAR PURPOSE. YOU ARE SOLELY RESPONSIBLE
FOR DETERMINING THE APPROPRIATENESS OF USING OR REDISTRIBUTING THE LLAMA MATERIALS AND ASSUME ANY RISKS ASSOCIATED
WITH YOUR USE OF THE LLAMA MATERIALS AND ANY OUTPUT AND RESULTS.
4. Limitation of Liability. IN NO EVENT WILL META OR ITS AFFILIATES BE LIABLE UNDER ANY THEORY OF LIABILITY,
WHETHER IN CONTRACT, TORT, NEGLIGENCE, PRODUCTS LIABILITY, OR OTHERWISE, ARISING OUT OF THIS AGREEMENT,
FOR ANY LOST PROFITS OR ANY INDIRECT, SPECIAL, CONSEQUENTIAL, INCIDENTAL, EXEMPLARY OR PUNITIVE DAMAGES, EVEN
IF META OR ITS AFFILIATES HAVE BEEN ADVISED OF THE POSSIBILITY OF ANY OF THE FOREGOING.
5. Intellectual Property.
a. No trademark licenses are granted under this Agreement, and in connection with the Llama Materials,
neither Meta nor Licensee may use any name or mark owned by or associated with the other or any of its affiliates,
except as required for reasonable and customary use in describing and redistributing the Llama Materials or as
set forth in this Section 5(a). Meta hereby grants you a license to use “Llama” (the “Mark”) solely as required
to comply with the last sentence of Section 1.b.i. You will comply with Meta’s brand guidelines (currently accessible
at https://about.meta.com/brand/resources/meta/company-brand/). All goodwill arising out of your use of the Mark
will inure to the benefit of Meta.
b. Subject to Meta’s ownership of Llama Materials and derivatives made by or for Meta, with respect to any
derivative works and modifications of the Llama Materials that are made by you, as between you and Meta,
you are and will be the owner of such derivative works and modifications.
c. If you institute litigation or other proceedings against Meta or any entity (including a cross-claim or
counterclaim in a lawsuit) alleging that the Llama Materials or Llama 3.2 outputs or results, or any portion
of any of the foregoing, constitutes infringement of intellectual property or other rights owned or licensable
by you, then any licenses granted to you under this Agreement shall terminate as of the date such litigation or
claim is filed or instituted. You will indemnify and hold harmless Meta from and against any claim by any third
party arising out of or related to your use or distribution of the Llama Materials.
6. Term and Termination. The term of this Agreement will commence upon your acceptance of this Agreement or access
to the Llama Materials and will continue in full force and effect until terminated in accordance with the terms
and conditions herein. Meta may terminate this Agreement if you are in breach of any term or condition of this
Agreement. Upon termination of this Agreement, you shall delete and cease use of the Llama Materials. Sections 3,
4 and 7 shall survive the termination of this Agreement.
7. Governing Law and Jurisdiction. This Agreement will be governed and construed under the laws of the State of
California without regard to choice of law principles, and the UN Convention on Contracts for the International
Sale of Goods does not apply to this Agreement. The courts of California shall have exclusive jurisdiction of
any dispute arising out of this Agreement.
### Llama 3.2 Acceptable Use Policy
Meta is committed to promoting safe and fair use of its tools and features, including Llama 3.2.
If you access or use Llama 3.2, you agree to this Acceptable Use Policy (“**Policy**”).
The most recent copy of this policy can be found at
[https://www.llama.com/llama3_2/use-policy](https://www.llama.com/llama3_2/use-policy).
#### Prohibited Uses
We want everyone to use Llama 3.2 safely and responsibly. You agree you will not use, or allow others to use, Llama 3.2 to:
1. Violate the law or others’ rights, including to:
1. Engage in, promote, generate, contribute to, encourage, plan, incite, or further illegal or unlawful activity or content, such as:
1. Violence or terrorism
2. Exploitation or harm to children, including the solicitation, creation, acquisition, or dissemination of child exploitative content or failure to report Child Sexual Abuse Material
3. Human trafficking, exploitation, and sexual violence
4. The illegal distribution of information or materials to minors, including obscene materials, or failure to employ legally required age-gating in connection with such information or materials.
5. Sexual solicitation
6. Any other criminal activity
1. Engage in, promote, incite, or facilitate the harassment, abuse, threatening, or bullying of individuals or groups of individuals
2. Engage in, promote, incite, or facilitate discrimination or other unlawful or harmful conduct in the provision of employment, employment benefits, credit, housing, other economic benefits, or other essential goods and services
3. Engage in the unauthorized or unlicensed practice of any profession including, but not limited to, financial, legal, medical/health, or related professional practices
4. Collect, process, disclose, generate, or infer private or sensitive information about individuals, including information about individuals’ identity, health, or demographic information, unless you have obtained the right to do so in accordance with applicable law
5. Engage in or facilitate any action or generate any content that infringes, misappropriates, or otherwise violates any third-party rights, including the outputs or results of any products or services using the Llama Materials
6. Create, generate, or facilitate the creation of malicious code, malware, computer viruses or do anything else that could disable, overburden, interfere with or impair the proper working, integrity, operation or appearance of a website or computer system
7. Engage in any action, or facilitate any action, to intentionally circumvent or remove usage restrictions or other safety measures, or to enable functionality disabled by Meta
2. Engage in, promote, incite, facilitate, or assist in the planning or development of activities that present a risk of death or bodily harm to individuals, including use of Llama 3.2 related to the following:
8. Military, warfare, nuclear industries or applications, espionage, use for materials or activities that are subject to the International Traffic Arms Regulations (ITAR) maintained by the United States Department of State or to the U.S. Biological Weapons Anti-Terrorism Act of 1989 or the Chemical Weapons Convention Implementation Act of 1997
9. Guns and illegal weapons (including weapon development)
10. Illegal drugs and regulated/controlled substances
11. Operation of critical infrastructure, transportation technologies, or heavy machinery
12. Self-harm or harm to others, including suicide, cutting, and eating disorders
13. Any content intended to incite or promote violence, abuse, or any infliction of bodily harm to an individual
3. Intentionally deceive or mislead others, including use of Llama 3.2 related to the following:
14. Generating, promoting, or furthering fraud or the creation or promotion of disinformation
15. Generating, promoting, or furthering defamatory content, including the creation of defamatory statements, images, or other content
16. Generating, promoting, or further distributing spam
17. Impersonating another individual without consent, authorization, or legal right
18. Representing that the use of Llama 3.2 or outputs are human-generated
19. Generating or facilitating false online engagement, including fake reviews and other means of fake online engagement
4. Fail to appropriately disclose to end users any known dangers of your AI system
5. Interact with third party tools, models, or software designed to generate unlawful content or engage in unlawful or harmful conduct and/or represent that the outputs of such tools, models, or software are associated with Meta or Llama 3.2
With respect to any multimodal models included in Llama 3.2, the rights granted under Section 1(a) of the Llama 3.2 Community License Agreement are not being granted to you if you are an individual domiciled in, or a company with a principal place of business in, the European Union. This restriction does not apply to end users of a product or service that incorporates any such multimodal models.
Please report any violation of this Policy, software “bug,” or other problems that could lead to a violation of this Policy through one of the following means:
* Reporting issues with the model: [https://github.com/meta-llama/llama-models/issues](https://l.workplace.com/l.php?u=https%3A%2F%2Fgithub.com%2Fmeta-llama%2Fllama-models%2Fissues&h=AT0qV8W9BFT6NwihiOHRuKYQM_UnkzN_NmHMy91OT55gkLpgi4kQupHUl0ssR4dQsIQ8n3tfd0vtkobvsEvt1l4Ic6GXI2EeuHV8N08OG2WnbAmm0FL4ObkazC6G_256vN0lN9DsykCvCqGZ)
* Reporting risky content generated by the model: [developers.facebook.com/llama_output_feedback](http://developers.facebook.com/llama_output_feedback)
* Reporting bugs and security concerns: [facebook.com/whitehat/info](http://facebook.com/whitehat/info)
* Reporting violations of the Acceptable Use Policy or unlicensed uses of Llama 3.2: [email protected]
extra_gated_fields:
First Name: text
Last Name: text
Date of birth: date_picker
Country: country
Affiliation: text
Job title:
type: select
options:
- Student
- Research Graduate
- AI researcher
- AI developer/engineer
- Reporter
- Other
geo: ip_location
By clicking Submit below I accept the terms of the license and acknowledge that the information I provide will be collected stored processed and shared in accordance with the Meta Privacy Policy: checkbox
extra_gated_description: >-
The information you provide will be collected, stored, processed and shared in
accordance with the [Meta Privacy
Policy](https://www.facebook.com/privacy/policy/).
extra_gated_button_content: Submit
---
## Model Information
The Llama 3.2 collection of multilingual large language models (LLMs) is a collection of pretrained and instruction-tuned generative models in 1B and 3B sizes (text in/text out). The Llama 3.2 instruction-tuned text only models are optimized for multilingual dialogue use cases, including agentic retrieval and summarization tasks. They outperform many of the available open source and closed chat models on common industry benchmarks.
**Model Developer:** Meta
**Model Architecture:** Llama 3.2 is an auto-regressive language model that uses an optimized transformer architecture. The tuned versions use supervised fine-tuning (SFT) and reinforcement learning with human feedback (RLHF) to align with human preferences for helpfulness and safety.
| | Training Data | Params | Input modalities | Output modalities | Context Length | GQA | Shared Embeddings | Token count | Knowledge cutoff |
| :---- | :---- | :---- | :---- | :---- | :---- | :---- | :---- | :---- | :---- |
| Llama 3.2 (text only) | A new mix of publicly available online data. | 1B (1.23B) | Multilingual Text | Multilingual Text and code | 128k | Yes | Yes | Up to 9T tokens | December 2023 |
| | | 3B (3.21B) | Multilingual Text | Multilingual Text and code | | | | | |
| Llama 3.2 Quantized (text only) | A new mix of publicly available online data. | 1B (1.23B) | Multilingual Text | Multilingual Text and code | 8k | Yes | Yes | Up to 9T tokens | December 2023 |
| | | 3B (3.21B) | Multilingual Text | Multilingual Text and code | | | | | |
**Supported Languages:** English, German, French, Italian, Portuguese, Hindi, Spanish, and Thai are officially supported. Llama 3.2 has been trained on a broader collection of languages than these 8 supported languages. Developers may fine-tune Llama 3.2 models for languages beyond these supported languages, provided they comply with the Llama 3.2 Community License and the Acceptable Use Policy. Developers are always expected to ensure that their deployments, including those that involve additional languages, are completed safely and responsibly.
**Llama 3.2 Model Family:** Token counts refer to pretraining data only. All model versions use Grouped-Query Attention (GQA) for improved inference scalability.
**Model Release Date:** Sept 25, 2024
**Status:** This is a static model trained on an offline dataset. Future versions may be released that improve model capabilities and safety.
**License:** Use of Llama 3.2 is governed by the [Llama 3.2 Community License](https://github.com/meta-llama/llama-models/blob/main/models/llama3_2/LICENSE) (a custom, commercial license agreement).
**Feedback:** Instructions on how to provide feedback or comments on the model can be found in the Llama Models [README](https://github.com/meta-llama/llama-models/blob/main/README.md). For more technical information about generation parameters and recipes for how to use Llama 3.2 in applications, please go [here](https://github.com/meta-llama/llama-recipes).
## Intended Use
**Intended Use Cases:** Llama 3.2 is intended for commercial and research use in multiple languages. Instruction tuned text only models are intended for assistant-like chat and agentic applications like knowledge retrieval and summarization, mobile AI powered writing assistants and query and prompt rewriting. Pretrained models can be adapted for a variety of additional natural language generation tasks. Similarly, quantized models can be adapted for a variety of on-device use-cases with limited compute resources.
**Out of Scope:** Use in any manner that violates applicable laws or regulations (including trade compliance laws). Use in any other way that is prohibited by the Acceptable Use Policy and Llama 3.2 Community License. Use in languages beyond those explicitly referenced as supported in this model card.
## How to use
This repository contains two versions of Llama-3.2-1B-Instruct, for use with transformers and with the original `llama` codebase.
### Use with transformers
Starting with `transformers >= 4.43.0` onward, you can run conversational inference using the Transformers `pipeline` abstraction or by leveraging the Auto classes with the `generate()` function.
Make sure to update your transformers installation via `pip install --upgrade transformers`.
```python
import torch
from transformers import pipeline
model_id = "meta-llama/Llama-3.2-1B-Instruct"
pipe = pipeline(
"text-generation",
model=model_id,
torch_dtype=torch.bfloat16,
device_map="auto",
)
messages = [
{"role": "system", "content": "You are a pirate chatbot who always responds in pirate speak!"},
{"role": "user", "content": "Who are you?"},
]
outputs = pipe(
messages,
max_new_tokens=256,
)
print(outputs[0]["generated_text"][-1])
```
Note: You can also find detailed recipes on how to use the model locally, with `torch.compile()`, assisted generations, quantised and more at [`huggingface-llama-recipes`](https://github.com/huggingface/huggingface-llama-recipes)
### Use with `llama`
Please, follow the instructions in the [repository](https://github.com/meta-llama/llama)
To download Original checkpoints, see the example command below leveraging `huggingface-cli`:
```
huggingface-cli download meta-llama/Llama-3.2-1B-Instruct --include "original/*" --local-dir Llama-3.2-1B-Instruct
```
## Hardware and Software
**Training Factors:** We used custom training libraries, Meta's custom built GPU cluster, and production infrastructure for pretraining. Fine-tuning, quantization, annotation, and evaluation were also performed on production infrastructure.
**Training Energy Use:** Training utilized a cumulative of **916k** GPU hours of computation on H100-80GB (TDP of 700W) type hardware, per the table below. Training time is the total GPU time required for training each model and power consumption is the peak power capacity per GPU device used, adjusted for power usage efficiency.
**Training Greenhouse Gas Emissions:** Estimated total location-based greenhouse gas emissions were **240** tons CO2eq for training. Since 2020, Meta has maintained net zero greenhouse gas emissions in its global operations and matched 100% of its electricity use with renewable energy; therefore, the total market-based greenhouse gas emissions for training were 0 tons CO2eq.
| | Training Time (GPU hours) | Logit Generation Time (GPU Hours) | Training Power Consumption (W) | Training Location-Based Greenhouse Gas Emissions (tons CO2eq) | Training Market-Based Greenhouse Gas Emissions (tons CO2eq) |
| :---- | :---: | ----- | :---: | :---: | :---: |
| Llama 3.2 1B | 370k | \- | 700 | 107 | 0 |
| Llama 3.2 3B | 460k | \- | 700 | 133 | 0 |
| Llama 3.2 1B SpinQuant | 1.7 | 0 | 700 | *Negligible*\*\* | 0 |
| Llama 3.2 3B SpinQuant | 2.4 | 0 | 700 | *Negligible*\*\* | 0 |
| Llama 3.2 1B QLora | 1.3k | 0 | 700 | 0.381 | 0 |
| Llama 3.2 3B QLora | 1.6k | 0 | 700 | 0.461 | 0 |
| Total | 833k | 86k | | 240 | 0 |
\*\* The location-based CO2e emissions of Llama 3.2 1B SpinQuant and Llama 3.2 3B SpinQuant are less than 0.001 metric tonnes each. This is due to the minimal training GPU hours that are required.
The methodology used to determine training energy use and greenhouse gas emissions can be found [here](https://arxiv.org/pdf/2204.05149). Since Meta is openly releasing these models, the training energy use and greenhouse gas emissions will not be incurred by others.
## Training Data
**Overview:** Llama 3.2 was pretrained on up to 9 trillion tokens of data from publicly available sources. For the 1B and 3B Llama 3.2 models, we incorporated logits from the Llama 3.1 8B and 70B models into the pretraining stage of the model development, where outputs (logits) from these larger models were used as token-level targets. Knowledge distillation was used after pruning to recover performance. In post-training we used a similar recipe as Llama 3.1 and produced final chat models by doing several rounds of alignment on top of the pre-trained model. Each round involved Supervised Fine-Tuning (SFT), Rejection Sampling (RS), and Direct Preference Optimization (DPO).
**Data Freshness:** The pretraining data has a cutoff of December 2023\.
## Quantization
### Quantization Scheme
We designed the current quantization scheme with the [PyTorch’s ExecuTorch](https://github.com/pytorch/executorch) inference framework and Arm CPU backend in mind, taking into account metrics including model quality, prefill/decoding speed, and memory footprint. Our quantization scheme involves three parts:
- All linear layers in all transformer blocks are quantized to a 4-bit groupwise scheme (with a group size of 32) for weights and 8-bit per-token dynamic quantization for activations.
- The classification layer is quantized to 8-bit per-channel for weight and 8-bit per token dynamic quantization for activation.
- Similar to classification layer, an 8-bit per channel quantization is used for embedding layer.
### Quantization-Aware Training and LoRA
The quantization-aware training (QAT) with low-rank adaptation (LoRA) models went through only post-training stages, using the same data as the full precision models. To initialize QAT, we utilize BF16 Llama 3.2 model checkpoints obtained after supervised fine-tuning (SFT) and perform an additional full round of SFT training with QAT. We then freeze the backbone of the QAT model and perform another round of SFT with LoRA adaptors applied to all layers within the transformer block. Meanwhile, the LoRA adaptors' weights and activations are maintained in BF16. Because our approach is similar to QLoRA of Dettmers et al., (2023) (i.e., quantization followed by LoRA adapters), we refer this method as QLoRA. Finally, we fine-tune the resulting model (both backbone and LoRA adaptors) using direct preference optimization (DPO).
### SpinQuant
[SpinQuant](https://arxiv.org/abs/2405.16406) was applied, together with generative post-training quantization (GPTQ). For the SpinQuant rotation matrix fine-tuning, we optimized for 100 iterations, using 800 samples with sequence-length 2048 from the WikiText 2 dataset. For GPTQ, we used 128 samples from the same dataset with the same sequence-length.
## Benchmarks \- English Text
In this section, we report the results for Llama 3.2 models on standard automatic benchmarks. For all these evaluations, we used our internal evaluations library.
### Base Pretrained Models
| Category | Benchmark | \# Shots | Metric | Llama 3.2 1B | Llama 3.2 3B | Llama 3.1 8B |
| ----- | ----- | :---: | :---: | :---: | :---: | :---: |
| General | MMLU | 5 | macro\_avg/acc\_char | 32.2 | 58 | 66.7 |
| | AGIEval English | 3-5 | average/acc\_char | 23.3 | 39.2 | 47.8 |
| | ARC-Challenge | 25 | acc\_char | 32.8 | 69.1 | 79.7 |
| Reading comprehension | SQuAD | 1 | em | 49.2 | 67.7 | 77 |
| | QuAC (F1) | 1 | f1 | 37.9 | 42.9 | 44.9 |
| | DROP (F1) | 3 | f1 | 28.0 | 45.2 | 59.5 |
| Long Context | Needle in Haystack | 0 | em | 96.8 | 1 | 1 |
### Instruction Tuned Models
| Capability | | Benchmark | \# Shots | Metric | Llama 3.2 1B bf16 | Llama 3.2 1B Vanilla PTQ\*\* | Llama 3.2 1B Spin Quant | Llama 3.2 1B QLoRA | Llama 3.2 3B bf16 | Llama 3.2 3B Vanilla PTQ\*\* | Llama 3.2 3B Spin Quant | Llama 3.2 3B QLoRA | Llama 3.1 8B |
| :---: | ----- | :---: | :---: | :---: | :---: | :---: | :---: | :---: | :---: | :---: | :---: | :---: | :---: |
| General | | MMLU | 5 | macro\_avg/acc | 49.3 | 43.3 | 47.3 | 49.0 | 63.4 | 60.5 | 62 | 62.4 | 69.4 |
| Re-writing | | Open-rewrite eval | 0 | micro\_avg/rougeL | 41.6 | 39.2 | 40.9 | 41.2 | 40.1 | 40.3 | 40.8 | 40.7 | 40.9 |
| Summarization | | TLDR9+ (test) | 1 | rougeL | 16.8 | 14.9 | 16.7 | 16.8 | 19.0 | 19.1 | 19.2 | 19.1 | 17.2 |
| Instruction following | | IFEval | 0 | Avg(Prompt/Instruction acc Loose/Strict) | 59.5 | 51.5 | 58.4 | 55.6 | 77.4 | 73.9 | 73.5 | 75.9 | 80.4 |
| Math | | GSM8K (CoT) | 8 | em\_maj1@1 | 44.4 | 33.1 | 40.6 | 46.5 | 77.7 | 72.9 | 75.7 | 77.9 | 84.5 |
| | | MATH (CoT) | 0 | final\_em | 30.6 | 20.5 | 25.3 | 31.0 | 48.0 | 44.2 | 45.3 | 49.2 | 51.9 |
| Reasoning | | ARC-C | 0 | acc | 59.4 | 54.3 | 57 | 60.7 | 78.6 | 75.6 | 77.6 | 77.6 | 83.4 |
| | | GPQA | 0 | acc | 27.2 | 25.9 | 26.3 | 25.9 | 32.8 | 32.8 | 31.7 | 33.9 | 32.8 |
| | | Hellaswag | 0 | acc | 41.2 | 38.1 | 41.3 | 41.5 | 69.8 | 66.3 | 68 | 66.3 | 78.7 |
| Tool Use | | BFCL V2 | 0 | acc | 25.7 | 14.3 | 15.9 | 23.7 | 67.0 | 53.4 | 60.1 | 63.5 | 67.1 |
| | | Nexus | 0 | macro\_avg/acc | 13.5 | 5.2 | 9.6 | 12.5 | 34.3 | 32.4 | 31.5 | 30.1 | 38.5 |
| Long Context | | InfiniteBench/En.QA | 0 | longbook\_qa/f1 | 20.3 | N/A | N/A | N/A | 19.8 | N/A | N/A | N/A | 27.3 |
| | | InfiniteBench/En.MC | 0 | longbook\_choice/acc | 38.0 | N/A | N/A | N/A | 63.3 | N/A | N/A | N/A | 72.2 |
| | | NIH/Multi-needle | 0 | recall | 75.0 | N/A | N/A | N/A | 84.7 | N/A | N/A | N/A | 98.8 |
| Multilingual | | MGSM (CoT) | 0 | em | 24.5 | 13.7 | 18.2 | 24.4 | 58.2 | 48.9 | 54.3 | 56.8 | 68.9 |
\*\*for comparison purposes only. Model not released.
### Multilingual Benchmarks
| Category | Benchmark | Language | Llama 3.2 1B | Llama 3.2 1B Vanilla PTQ\*\* | Llama 3.2 1B Spin Quant | Llama 3.2 1B QLoRA | Llama 3.2 3B | Llama 3.2 3B Vanilla PTQ\*\* | Llama 3.2 3B Spin Quant | Llama 3.2 3B QLoRA | Llama 3.1 8B |
| :---: | :---: | :---: | :---: | :---: | :---: | :---: | :---: | :---: | :---: | :---: | :---: |
| General | MMLU (5-shot, macro_avg/acc) | Portuguese | 39.8 | 34.9 | 38.9 | 40.2 | 54.5 | 50.9 | 53.3 | 53.4 | 62.1 |
| | | Spanish | 41.5 | 36.0 | 39.8 | 41.8 | 55.1 | 51.9 | 53.6 | 53.6 | 62.5 |
| | | Italian | 39.8 | 34.9 | 38.1 | 40.6 | 53.8 | 49.9 | 52.1 | 51.7 | 61.6 |
| | | German | 39.2 | 34.9 | 37.5 | 39.6 | 53.3 | 50.0 | 52.2 | 51.3 | 60.6 |
| | | French | 40.5 | 34.8 | 39.2 | 40.8 | 54.6 | 51.2 | 53.3 | 53.3 | 62.3 |
| | | Hindi | 33.5 | 30.0 | 32.1 | 34.0 | 43.3 | 40.4 | 42.0 | 42.1 | 50.9 |
| | | Thai | 34.7 | 31.2 | 32.4 | 34.9 | 44.5 | 41.3 | 44.0 | 42.2 | 50.3 |
\*\*for comparison purposes only. Model not released.
## Inference time
In the below table, we compare the performance metrics of different quantization methods (SpinQuant and QAT \+ LoRA) with the BF16 baseline. The evaluation was done using the [ExecuTorch](https://github.com/pytorch/executorch) framework as the inference engine, with the ARM CPU as a backend using Android OnePlus 12 device.
| Category | Decode (tokens/sec) | Time-to-first-token (sec) | Prefill (tokens/sec) | Model size (PTE file size in MB) | Memory size (RSS in MB) |
| :---- | ----- | ----- | ----- | ----- | ----- |
| 1B BF16 (baseline) | 19.2 | 1.0 | 60.3 | 2358 | 3,185 |
| 1B SpinQuant | 50.2 (2.6x) | 0.3 (-76.9%) | 260.5 (4.3x) | 1083 (-54.1%) | 1,921 (-39.7%) |
| 1B QLoRA | 45.8 (2.4x) | 0.3 (-76.0%) | 252.0 (4.2x) | 1127 (-52.2%) | 2,255 (-29.2%) |
| 3B BF16 (baseline) | 7.6 | 3.0 | 21.2 | 6129 | 7,419 |
| 3B SpinQuant | 19.7 (2.6x) | 0.7 (-76.4%) | 89.7 (4.2x) | 2435 (-60.3%) | 3,726 (-49.8%) |
| 3B QLoRA | 18.5 (2.4x) | 0.7 (-76.1%) | 88.8 (4.2x) | 2529 (-58.7%) | 4,060 (-45.3%) |
(\*) The performance measurement is done using an adb binary-based approach.
(\*\*) It is measured on an Android OnePlus 12 device.
(\*\*\*) Time-to-first-token (TTFT) is measured with prompt length=64
*Footnote:*
- *Decode (tokens/second) is for how quickly it keeps generating. Higher is better.*
- *Time-to-first-token (TTFT for shorthand) is for how fast it generates the first token for a given prompt. Lower is better.*
- *Prefill is the inverse of TTFT (aka 1/TTFT) in tokens/second. Higher is better*
- *Model size \- how big is the model, measured by, PTE file, a binary file format for ExecuTorch*
- *RSS size \- Memory usage in resident set size (RSS)*
## Responsibility & Safety
As part of our Responsible release approach, we followed a three-pronged strategy to managing trust & safety risks:
1. Enable developers to deploy helpful, safe and flexible experiences for their target audience and for the use cases supported by Llama
2. Protect developers against adversarial users aiming to exploit Llama capabilities to potentially cause harm
3. Provide protections for the community to help prevent the misuse of our models
### Responsible Deployment
**Approach:** Llama is a foundational technology designed to be used in a variety of use cases. Examples on how Meta’s Llama models have been responsibly deployed can be found in our [Community Stories webpage](https://llama.meta.com/community-stories/). Our approach is to build the most helpful models, enabling the world to benefit from the technology power, by aligning our model safety for generic use cases and addressing a standard set of harms. Developers are then in the driver’s seat to tailor safety for their use cases, defining their own policies and deploying the models with the necessary safeguards in their Llama systems. Llama 3.2 was developed following the best practices outlined in our [Responsible Use Guide](https://llama.meta.com/responsible-use-guide/).
#### Llama 3.2 Instruct
**Objective:** Our main objectives for conducting safety fine-tuning are to provide the research community with a valuable resource for studying the robustness of safety fine-tuning, as well as to offer developers a readily available, safe, and powerful model for various applications to reduce the developer workload to deploy safe AI systems. We implemented the same set of safety mitigations as in Llama 3, and you can learn more about these in the Llama 3 [paper](https://ai.meta.com/research/publications/the-llama-3-herd-of-models/).
**Fine-Tuning Data:** We employ a multi-faceted approach to data collection, combining human-generated data from our vendors with synthetic data to mitigate potential safety risks. We’ve developed many large language model (LLM)-based classifiers that enable us to thoughtfully select high-quality prompts and responses, enhancing data quality control.
**Refusals and Tone:** Building on the work we started with Llama 3, we put a great emphasis on model refusals to benign prompts as well as refusal tone. We included both borderline and adversarial prompts in our safety data strategy, and modified our safety data responses to follow tone guidelines.
#### Llama 3.2 Systems
**Safety as a System:** Large language models, including Llama 3.2, **are not designed to be deployed in isolation** but instead should be deployed as part of an overall AI system with additional safety guardrails as required. Developers are expected to deploy system safeguards when building agentic systems. Safeguards are key to achieve the right helpfulness-safety alignment as well as mitigating safety and security risks inherent to the system and any integration of the model or system with external tools. As part of our responsible release approach, we provide the community with [safeguards](https://llama.meta.com/trust-and-safety/) that developers should deploy with Llama models or other LLMs, including Llama Guard, Prompt Guard and Code Shield. All our [reference implementations](https://github.com/meta-llama/llama-agentic-system) demos contain these safeguards by default so developers can benefit from system-level safety out-of-the-box.
### New Capabilities and Use Cases
**Technological Advancement:** Llama releases usually introduce new capabilities that require specific considerations in addition to the best practices that generally apply across all Generative AI use cases. For prior release capabilities also supported by Llama 3.2, see [Llama 3.1 Model Card](https://github.com/meta-llama/llama-models/blob/main/models/llama3_1/MODEL_CARD.md), as the same considerations apply here as well.
**Constrained Environments:** Llama 3.2 1B and 3B models are expected to be deployed in highly constrained environments, such as mobile devices. LLM Systems using smaller models will have a different alignment profile and safety/helpfulness tradeoff than more complex, larger systems. Developers should ensure the safety of their system meets the requirements of their use case. We recommend using lighter system safeguards for such use cases, like Llama Guard 3-1B or its mobile-optimized version.
### Evaluations
**Scaled Evaluations:** We built dedicated, adversarial evaluation datasets and evaluated systems composed of Llama models and Purple Llama safeguards to filter input prompt and output response. It is important to evaluate applications in context, and we recommend building dedicated evaluation dataset for your use case.
**Red Teaming:** We conducted recurring red teaming exercises with the goal of discovering risks via adversarial prompting and we used the learnings to improve our benchmarks and safety tuning datasets. We partnered early with subject-matter experts in critical risk areas to understand the nature of these real-world harms and how such models may lead to unintended harm for society. Based on these conversations, we derived a set of adversarial goals for the red team to attempt to achieve, such as extracting harmful information or reprogramming the model to act in a potentially harmful capacity. The red team consisted of experts in cybersecurity, adversarial machine learning, responsible AI, and integrity in addition to multilingual content specialists with background in integrity issues in specific geographic markets.
### Critical Risks
In addition to our safety work above, we took extra care on measuring and/or mitigating the following critical risk areas:
**1\. CBRNE (Chemical, Biological, Radiological, Nuclear, and Explosive Weapons):** Llama 3.2 1B and 3B models are smaller and less capable derivatives of Llama 3.1. For Llama 3.1 70B and 405B, to assess risks related to proliferation of chemical and biological weapons, we performed uplift testing designed to assess whether use of Llama 3.1 models could meaningfully increase the capabilities of malicious actors to plan or carry out attacks using these types of weapons and have determined that such testing also applies to the smaller 1B and 3B models.
**2\. Child Safety:** Child Safety risk assessments were conducted using a team of experts, to assess the model’s capability to produce outputs that could result in Child Safety risks and inform on any necessary and appropriate risk mitigations via fine tuning. We leveraged those expert red teaming sessions to expand the coverage of our evaluation benchmarks through Llama 3 model development. For Llama 3, we conducted new in-depth sessions using objective based methodologies to assess the model risks along multiple attack vectors including the additional languages Llama 3 is trained on. We also partnered with content specialists to perform red teaming exercises assessing potentially violating content while taking account of market specific nuances or experiences.
**3\. Cyber Attacks:** For Llama 3.1 405B, our cyber attack uplift study investigated whether LLMs can enhance human capabilities in hacking tasks, both in terms of skill level and speed.
Our attack automation study focused on evaluating the capabilities of LLMs when used as autonomous agents in cyber offensive operations, specifically in the context of ransomware attacks. This evaluation was distinct from previous studies that considered LLMs as interactive assistants. The primary objective was to assess whether these models could effectively function as independent agents in executing complex cyber-attacks without human intervention. Because Llama 3.2’s 1B and 3B models are smaller and less capable models than Llama 3.1 405B, we broadly believe that the testing conducted for the 405B model also applies to Llama 3.2 models.
### Community
**Industry Partnerships:** Generative AI safety requires expertise and tooling, and we believe in the strength of the open community to accelerate its progress. We are active members of open consortiums, including the AI Alliance, Partnership on AI and MLCommons, actively contributing to safety standardization and transparency. We encourage the community to adopt taxonomies like the MLCommons Proof of Concept evaluation to facilitate collaboration and transparency on safety and content evaluations. Our Purple Llama tools are open sourced for the community to use and widely distributed across ecosystem partners including cloud service providers. We encourage community contributions to our [Github repository](https://github.com/meta-llama/PurpleLlama).
**Grants:** We also set up the [Llama Impact Grants](https://llama.meta.com/llama-impact-grants/) program to identify and support the most compelling applications of Meta’s Llama model for societal benefit across three categories: education, climate and open innovation. The 20 finalists from the hundreds of applications can be found [here](https://llama.meta.com/llama-impact-grants/#finalists).
**Reporting:** Finally, we put in place a set of resources including an [output reporting mechanism](https://developers.facebook.com/llama_output_feedback) and [bug bounty program](https://www.facebook.com/whitehat) to continuously improve the Llama technology with the help of the community.
## Ethical Considerations and Limitations
**Values:** The core values of Llama 3.2 are openness, inclusivity and helpfulness. It is meant to serve everyone, and to work for a wide range of use cases. It is thus designed to be accessible to people across many different backgrounds, experiences and perspectives. Llama 3.2 addresses users and their needs as they are, without insertion unnecessary judgment or normativity, while reflecting the understanding that even content that may appear problematic in some cases can serve valuable purposes in others. It respects the dignity and autonomy of all users, especially in terms of the values of free thought and expression that power innovation and progress.
**Testing:** Llama 3.2 is a new technology, and like any new technology, there are risks associated with its use. Testing conducted to date has not covered, nor could it cover, all scenarios. For these reasons, as with all LLMs, Llama 3.2’s potential outputs cannot be predicted in advance, and the model may in some instances produce inaccurate, biased or other objectionable responses to user prompts. Therefore, before deploying any applications of Llama 3.2 models, developers should perform safety testing and tuning tailored to their specific applications of the model. Please refer to available resources including our [Responsible Use Guide](https://llama.meta.com/responsible-use-guide), [Trust and Safety](https://llama.meta.com/trust-and-safety/) solutions, and other [resources](https://llama.meta.com/docs/get-started/) to learn more about responsible development.
|
microsoft/Phi-3-mini-4k-instruct | microsoft | "2024-09-20T18:09:38Z" | 1,356,555 | 1,071 | transformers | [
"transformers",
"safetensors",
"phi3",
"text-generation",
"nlp",
"code",
"conversational",
"custom_code",
"en",
"fr",
"license:mit",
"autotrain_compatible",
"text-generation-inference",
"endpoints_compatible",
"region:us"
] | text-generation | "2024-04-22T16:18:17Z" | ---
license: mit
license_link: https://huggingface.co/microsoft/Phi-3-mini-4k-instruct/resolve/main/LICENSE
language:
- en
- fr
pipeline_tag: text-generation
tags:
- nlp
- code
inference:
parameters:
temperature: 0
widget:
- messages:
- role: user
content: Can you provide ways to eat combinations of bananas and dragonfruits?
---
🎉 **Phi-3.5**: [[mini-instruct]](https://huggingface.co/microsoft/Phi-3.5-mini-instruct); [[MoE-instruct]](https://huggingface.co/microsoft/Phi-3.5-MoE-instruct) ; [[vision-instruct]](https://huggingface.co/microsoft/Phi-3.5-vision-instruct)
## Model Summary
The Phi-3-Mini-4K-Instruct is a 3.8B parameters, lightweight, state-of-the-art open model trained with the Phi-3 datasets that includes both synthetic data and the filtered publicly available websites data with a focus on high-quality and reasoning dense properties.
The model belongs to the Phi-3 family with the Mini version in two variants [4K](https://huggingface.co/microsoft/Phi-3-mini-4k-instruct) and [128K](https://huggingface.co/microsoft/Phi-3-mini-128k-instruct) which is the context length (in tokens) that it can support.
The model has underwent a post-training process that incorporates both supervised fine-tuning and direct preference optimization for the instruction following and safety measures.
When assessed against benchmarks testing common sense, language understanding, math, code, long context and logical reasoning, Phi-3 Mini-4K-Instruct showcased a robust and state-of-the-art performance among models with less than 13 billion parameters.
Resources and Technical Documentation:
🏡 [Phi-3 Portal](https://azure.microsoft.com/en-us/products/phi-3) <br>
📰 [Phi-3 Microsoft Blog](https://aka.ms/Phi-3Build2024) <br>
📖 [Phi-3 Technical Report](https://aka.ms/phi3-tech-report) <br>
🛠️ [Phi-3 on Azure AI Studio](https://aka.ms/phi3-azure-ai) <br>
👩🍳 [Phi-3 Cookbook](https://github.com/microsoft/Phi-3CookBook) <br>
🖥️ [Try It](https://aka.ms/try-phi3)
| | Short Context | Long Context |
| :------- | :------------- | :------------ |
| Mini | 4K [[HF]](https://huggingface.co/microsoft/Phi-3-mini-4k-instruct) ; [[ONNX]](https://huggingface.co/microsoft/Phi-3-mini-4k-instruct-onnx) ; [[GGUF]](https://huggingface.co/microsoft/Phi-3-mini-4k-instruct-gguf) | 128K [[HF]](https://huggingface.co/microsoft/Phi-3-mini-128k-instruct) ; [[ONNX]](https://huggingface.co/microsoft/Phi-3-mini-128k-instruct-onnx)|
| Small | 8K [[HF]](https://huggingface.co/microsoft/Phi-3-small-8k-instruct) ; [[ONNX]](https://huggingface.co/microsoft/Phi-3-small-8k-instruct-onnx-cuda) | 128K [[HF]](https://huggingface.co/microsoft/Phi-3-small-128k-instruct) ; [[ONNX]](https://huggingface.co/microsoft/Phi-3-small-128k-instruct-onnx-cuda)|
| Medium | 4K [[HF]](https://huggingface.co/microsoft/Phi-3-medium-4k-instruct) ; [[ONNX]](https://huggingface.co/microsoft/Phi-3-medium-4k-instruct-onnx-cuda) | 128K [[HF]](https://huggingface.co/microsoft/Phi-3-medium-128k-instruct) ; [[ONNX]](https://huggingface.co/microsoft/Phi-3-medium-128k-instruct-onnx-cuda)|
| Vision | | 128K [[HF]](https://huggingface.co/microsoft/Phi-3-vision-128k-instruct) ; [[ONNX]](https://huggingface.co/microsoft/Phi-3-vision-128k-instruct-onnx-cuda)|
## Intended Uses
**Primary use cases**
The model is intended for broad commercial and research use in English. The model provides uses for general purpose AI systems and applications which require
1) memory/compute constrained environments;
2) latency bound scenarios;
3) strong reasoning (especially math and logic).
Our model is designed to accelerate research on language and multimodal models, for use as a building block for generative AI powered features.
**Out-of-scope use cases**
Our models are not specifically designed or evaluated for all downstream purposes. Developers should consider common limitations of language models as they select use cases, and evaluate and mitigate for accuracy, safety, and fairness before using within a specific downstream use case, particularly for high-risk scenarios.
Developers should be aware of and adhere to applicable laws or regulations (including privacy, trade compliance laws, etc.) that are relevant to their use case.
**Nothing contained in this Model Card should be interpreted as or deemed a restriction or modification to the license the model is released under.**
## Release Notes
This is an update over the original instruction-tuned Phi-3-mini release based on valuable customer feedback.
The model used additional post-training data leading to substantial gains on instruction following and structure output.
We also improve multi-turn conversation quality, explicitly support <|system|> tag, and significantly improve reasoning capability.
We believe most use cases will benefit from this release, but we encourage users to test in their particular AI applications.
We appreciate the enthusiastic adoption of the Phi-3 model family, and continue to welcome all feedback from the community.
The table below highlights improvements on instruction following, structure output, and reasoning of the new release on publich and internal benchmark datasets.
| Benchmarks | Original | June 2024 Update |
|:------------|:----------|:------------------|
| Instruction Extra Hard | 5.7 | 6.0 |
| Instruction Hard | 4.9 | 5.1 |
| Instructions Challenge | 24.6 | 42.3 |
| JSON Structure Output | 11.5 | 52.3 |
| XML Structure Output | 14.4 | 49.8 |
| GPQA | 23.7 | 30.6 |
| MMLU | 68.8 | 70.9 |
| **Average** | **21.9** | **36.7** |
Notes: if users would like to check out the previous version, use the git commit id **ff07dc01615f8113924aed013115ab2abd32115b**. For the model conversion, e.g. GGUF and other formats, we invite the community to experiment with various approaches and share your valuable feedback. Let's innovate together!
## How to Use
Phi-3 Mini-4K-Instruct has been integrated in the `4.41.2` version of `transformers`. The current `transformers` version can be verified with: `pip list | grep transformers`.
Examples of required packages:
```
flash_attn==2.5.8
torch==2.3.1
accelerate==0.31.0
transformers==4.41.2
```
Phi-3 Mini-4K-Instruct is also available in [Azure AI Studio](https://aka.ms/try-phi3)
### Tokenizer
Phi-3 Mini-4K-Instruct supports a vocabulary size of up to `32064` tokens. The [tokenizer files](https://huggingface.co/microsoft/Phi-3-mini-4k-instruct/blob/main/added_tokens.json) already provide placeholder tokens that can be used for downstream fine-tuning, but they can also be extended up to the model's vocabulary size.
### Chat Format
Given the nature of the training data, the Phi-3 Mini-4K-Instruct model is best suited for prompts using the chat format as follows.
You can provide the prompt as a question with a generic template as follow:
```markdown
<|system|>
You are a helpful assistant.<|end|>
<|user|>
Question?<|end|>
<|assistant|>
```
For example:
```markdown
<|system|>
You are a helpful assistant.<|end|>
<|user|>
How to explain Internet for a medieval knight?<|end|>
<|assistant|>
```
where the model generates the text after `<|assistant|>` . In case of few-shots prompt, the prompt can be formatted as the following:
```markdown
<|system|>
You are a helpful travel assistant.<|end|>
<|user|>
I am going to Paris, what should I see?<|end|>
<|assistant|>
Paris, the capital of France, is known for its stunning architecture, art museums, historical landmarks, and romantic atmosphere. Here are some of the top attractions to see in Paris:\n\n1. The Eiffel Tower: The iconic Eiffel Tower is one of the most recognizable landmarks in the world and offers breathtaking views of the city.\n2. The Louvre Museum: The Louvre is one of the world's largest and most famous museums, housing an impressive collection of art and artifacts, including the Mona Lisa.\n3. Notre-Dame Cathedral: This beautiful cathedral is one of the most famous landmarks in Paris and is known for its Gothic architecture and stunning stained glass windows.\n\nThese are just a few of the many attractions that Paris has to offer. With so much to see and do, it's no wonder that Paris is one of the most popular tourist destinations in the world."<|end|>
<|user|>
What is so great about #1?<|end|>
<|assistant|>
```
### Sample inference code
This code snippets show how to get quickly started with running the model on a GPU:
```python
import torch
from transformers import AutoModelForCausalLM, AutoTokenizer, pipeline
torch.random.manual_seed(0)
model = AutoModelForCausalLM.from_pretrained(
"microsoft/Phi-3-mini-4k-instruct",
device_map="cuda",
torch_dtype="auto",
trust_remote_code=True,
)
tokenizer = AutoTokenizer.from_pretrained("microsoft/Phi-3-mini-4k-instruct")
messages = [
{"role": "system", "content": "You are a helpful AI assistant."},
{"role": "user", "content": "Can you provide ways to eat combinations of bananas and dragonfruits?"},
{"role": "assistant", "content": "Sure! Here are some ways to eat bananas and dragonfruits together: 1. Banana and dragonfruit smoothie: Blend bananas and dragonfruits together with some milk and honey. 2. Banana and dragonfruit salad: Mix sliced bananas and dragonfruits together with some lemon juice and honey."},
{"role": "user", "content": "What about solving an 2x + 3 = 7 equation?"},
]
pipe = pipeline(
"text-generation",
model=model,
tokenizer=tokenizer,
)
generation_args = {
"max_new_tokens": 500,
"return_full_text": False,
"temperature": 0.0,
"do_sample": False,
}
output = pipe(messages, **generation_args)
print(output[0]['generated_text'])
```
Note: If you want to use flash attention, call _AutoModelForCausalLM.from_pretrained()_ with _attn_implementation="flash_attention_2"_
## Responsible AI Considerations
Like other language models, the Phi series models can potentially behave in ways that are unfair, unreliable, or offensive. Some of the limiting behaviors to be aware of include:
+ Quality of Service: the Phi models are trained primarily on English text. Languages other than English will experience worse performance. English language varieties with less representation in the training data might experience worse performance than standard American English.
+ Representation of Harms & Perpetuation of Stereotypes: These models can over- or under-represent groups of people, erase representation of some groups, or reinforce demeaning or negative stereotypes. Despite safety post-training, these limitations may still be present due to differing levels of representation of different groups or prevalence of examples of negative stereotypes in training data that reflect real-world patterns and societal biases.
+ Inappropriate or Offensive Content: these models may produce other types of inappropriate or offensive content, which may make it inappropriate to deploy for sensitive contexts without additional mitigations that are specific to the use case.
+ Information Reliability: Language models can generate nonsensical content or fabricate content that might sound reasonable but is inaccurate or outdated.
+ Limited Scope for Code: Majority of Phi-3 training data is based in Python and use common packages such as "typing, math, random, collections, datetime, itertools". If the model generates Python scripts that utilize other packages or scripts in other languages, we strongly recommend users manually verify all API uses.
Developers should apply responsible AI best practices and are responsible for ensuring that a specific use case complies with relevant laws and regulations (e.g. privacy, trade, etc.). Important areas for consideration include:
+ Allocation: Models may not be suitable for scenarios that could have consequential impact on legal status or the allocation of resources or life opportunities (ex: housing, employment, credit, etc.) without further assessments and additional debiasing techniques.
+ High-Risk Scenarios: Developers should assess suitability of using models in high-risk scenarios where unfair, unreliable or offensive outputs might be extremely costly or lead to harm. This includes providing advice in sensitive or expert domains where accuracy and reliability are critical (ex: legal or health advice). Additional safeguards should be implemented at the application level according to the deployment context.
+ Misinformation: Models may produce inaccurate information. Developers should follow transparency best practices and inform end-users they are interacting with an AI system. At the application level, developers can build feedback mechanisms and pipelines to ground responses in use-case specific, contextual information, a technique known as Retrieval Augmented Generation (RAG).
+ Generation of Harmful Content: Developers should assess outputs for their context and use available safety classifiers or custom solutions appropriate for their use case.
+ Misuse: Other forms of misuse such as fraud, spam, or malware production may be possible, and developers should ensure that their applications do not violate applicable laws and regulations.
## Training
### Model
* Architecture: Phi-3 Mini-4K-Instruct has 3.8B parameters and is a dense decoder-only Transformer model. The model is fine-tuned with Supervised fine-tuning (SFT) and Direct Preference Optimization (DPO) to ensure alignment with human preferences and safety guidlines.
* Inputs: Text. It is best suited for prompts using chat format.
* Context length: 4K tokens
* GPUs: 512 H100-80G
* Training time: 10 days
* Training data: 4.9T tokens
* Outputs: Generated text in response to the input
* Dates: Our models were trained between May and June 2024
* Status: This is a static model trained on an offline dataset with cutoff date October 2023. Future versions of the tuned models may be released as we improve models.
* Release dates: June, 2024.
### Datasets
Our training data includes a wide variety of sources, totaling 4.9 trillion tokens, and is a combination of
1) Publicly available documents filtered rigorously for quality, selected high-quality educational data, and code;
2) Newly created synthetic, “textbook-like” data for the purpose of teaching math, coding, common sense reasoning, general knowledge of the world (science, daily activities, theory of mind, etc.);
3) High quality chat format supervised data covering various topics to reflect human preferences on different aspects such as instruct-following, truthfulness, honesty and helpfulness.
We are focusing on the quality of data that could potentially improve the reasoning ability for the model, and we filter the publicly available documents to contain the correct level of knowledge. As an example, the result of a game in premier league in a particular day might be good training data for frontier models, but we need to remove such information to leave more model capacity for reasoning for the small size models. More details about data can be found in the [Phi-3 Technical Report](https://aka.ms/phi3-tech-report).
### Fine-tuning
A basic example of multi-GPUs supervised fine-tuning (SFT) with TRL and Accelerate modules is provided [here](https://huggingface.co/microsoft/Phi-3-mini-4k-instruct/resolve/main/sample_finetune.py).
## Benchmarks
We report the results under completion format for Phi-3-Mini-4K-Instruct on standard open-source benchmarks measuring the model's reasoning ability (both common sense reasoning and logical reasoning). We compare to Mistral-7b-v0.1, Mixtral-8x7b, Gemma 7B, Llama-3-8B-Instruct, and GPT3.5-Turbo-1106.
All the reported numbers are produced with the exact same pipeline to ensure that the numbers are comparable. These numbers might differ from other published numbers due to slightly different choices in the evaluation.
As is now standard, we use few-shot prompts to evaluate the models, at temperature 0.
The prompts and number of shots are part of a Microsoft internal tool to evaluate language models, and in particular we did no optimization to the pipeline for Phi-3.
More specifically, we do not change prompts, pick different few-shot examples, change prompt format, or do any other form of optimization for the model.
The number of k–shot examples is listed per-benchmark.
| Category | Benchmark | Phi-3-Mini-4K-Ins | Gemma-7B | Mistral-7b | Mixtral-8x7b | Llama-3-8B-Ins | GPT3.5-Turbo-1106 |
|:----------|:-----------|:-------------------|:----------|:------------|:--------------|:----------------|:-------------------|
| Popular aggregated benchmark | AGI Eval <br>5-shot| 39.0 | 42.1 | 35.1 | 45.2 | 42 | 48.4 |
| | MMLU <br>5-shot | 70.9 | 63.6 | 61.7 | 70.5 | 66.5 | 71.4 |
| | BigBench Hard CoT<br>3-shot| 73.5 | 59.6 | 57.3 | 69.7 | 51.5 | 68.3 |
| Language Understanding | ANLI <br>7-shot | 53.6 | 48.7 | 47.1 | 55.2 | 57.3 | 58.1 |
| | HellaSwag <br>5-shot| 75.3 | 49.8 | 58.5 | 70.4 | 71.1 | 78.8 |
| Reasoning | ARC Challenge <br>10-shot | 86.3 | 78.3 | 78.6 | 87.3 | 82.8 | 87.4 |
| | BoolQ <br>0-shot | 78.1 | 66 | 72.2 | 76.6 | 80.9 | 79.1 |
| | MedQA <br>2-shot| 56.5 | 49.6 | 50 | 62.2 | 60.5 | 63.4 |
| | OpenBookQA <br>10-shot| 82.2 | 78.6 | 79.8 | 85.8 | 82.6 | 86 |
| | PIQA <br>5-shot| 83.5 | 78.1 | 77.7 | 86 | 75.7 | 86.6 |
| | GPQA <br>0-shot| 30.6 | 2.9 | 15 | 6.9 | 32.4 | 30.8 |
| | Social IQA <br>5-shot| 77.6 | 65.5 | 74.6 | 75.9 | 73.9 | 68.3 |
| | TruthfulQA (MC2) <br>10-shot| 64.7 | 52.1 | 53 | 60.1 | 63.2 | 67.7 |
| | WinoGrande <br>5-shot| 71.6 | 55.6 | 54.2 | 62 | 65 | 68.8 |
| Factual Knowledge | TriviaQA <br>5-shot| 61.4 | 72.3 | 75.2 | 82.2 | 67.7 | 85.8 |
| Math | GSM8K CoT <br>8-shot| 85.7 | 59.8 | 46.4 | 64.7 | 77.4 | 78.1 |
| Code Generation | HumanEval <br>0-shot| 57.3 | 34.1 | 28.0 | 37.8 | 60.4 | 62.2 |
| | MBPP <br>3-shot| 69.8 | 51.5 | 50.8 | 60.2 | 67.7 | 77.8 |
| **Average** | | **67.6** | **56.0** | **56.4** | **64.4** | **65.5** | **70.4** |
We take a closer look at different categories across 100 public benchmark datasets at the table below:
| Category | Phi-3-Mini-4K-Instruct | Gemma-7B | Mistral-7B | Mixtral 8x7B | Llama-3-8B-Instruct | GPT-3.5-Turbo |
|:----------|:------------------------|:----------|:------------|:--------------|:---------------------|:---------------|
| Popular aggregated benchmark | 61.1 | 59.4 | 56.5 | 66.2 | 59.9 | 67.0 |
| Reasoning | 70.8 | 60.3 | 62.8 | 68.1 | 69.6 | 71.8 |
| Language understanding | 60.5 | 57.6 | 52.5 | 66.1 | 63.2 | 67.7 |
| Code generation | 60.7 | 45.6 | 42.9 | 52.7 | 56.4 | 70.4 |
| Math | 50.6 | 35.8 | 25.4 | 40.3 | 41.1 | 52.8 |
| Factual knowledge | 38.4 | 46.7 | 49.8 | 58.6 | 43.1 | 63.4 |
| Multilingual | 56.7 | 66.5 | 57.4 | 66.7 | 66.6 | 71.0 |
| Robustness | 61.1 | 38.4 | 40.6 | 51.0 | 64.5 | 69.3 |
Overall, the model with only 3.8B-param achieves a similar level of language understanding and reasoning ability as much larger models. However, it is still fundamentally limited by its size for certain tasks. The model simply does not have the capacity to store too much world knowledge, which can be seen for example with low performance on TriviaQA. However, we believe such weakness can be resolved by augmenting Phi-3-Mini with a search engine.
## Cross Platform Support
[ONNX runtime](https://onnxruntime.ai/blogs/accelerating-phi-3) now supports Phi-3 mini models across platforms and hardware.
Optimized phi-3 models are also published here in ONNX format, to run with ONNX Runtime on CPU and GPU across devices, including server platforms, Windows, Linux and Mac desktops, and mobile CPUs, with the precision best suited to each of these targets. DirectML GPU acceleration is supported for Windows desktops GPUs (AMD, Intel, and NVIDIA).
Along with DML, ONNX Runtime provides cross platform support for Phi3 mini across a range of devices CPU, GPU, and mobile.
Here are some of the optimized configurations we have added:
1. ONNX models for int4 DML: Quantized to int4 via AWQ
2. ONNX model for fp16 CUDA
3. ONNX model for int4 CUDA: Quantized to int4 via RTN
4. ONNX model for int4 CPU and Mobile: Quantized to int4 via R
## Software
* [PyTorch](https://github.com/pytorch/pytorch)
* [Transformers](https://github.com/huggingface/transformers)
* [Flash-Attention](https://github.com/HazyResearch/flash-attention)
## Hardware
Note that by default, the Phi-3 Mini-4K-Instruct model uses flash attention, which requires certain types of GPU hardware to run. We have tested on the following GPU types:
* NVIDIA A100
* NVIDIA A6000
* NVIDIA H100
If you want to run the model on:
* NVIDIA V100 or earlier generation GPUs: call AutoModelForCausalLM.from_pretrained() with attn_implementation="eager"
* CPU: use the **GGUF** quantized models [4K](https://aka.ms/Phi3-mini-4k-instruct-gguf)
+ Optimized inference on GPU, CPU, and Mobile: use the **ONNX** models [4K](https://aka.ms/Phi3-mini-4k-instruct-onnx)
## License
The model is licensed under the [MIT license](https://huggingface.co/microsoft/Phi-3-mini-4k/resolve/main/LICENSE).
## Trademarks
This project may contain trademarks or logos for projects, products, or services. Authorized use of Microsoft trademarks or logos is subject to and must follow [Microsoft’s Trademark & Brand Guidelines](https://www.microsoft.com/en-us/legal/intellectualproperty/trademarks). Use of Microsoft trademarks or logos in modified versions of this project must not cause confusion or imply Microsoft sponsorship. Any use of third-party trademarks or logos are subject to those third-party’s policies. |
TheBloke/galactica-30B-evol-instruct-70K-GPTQ | TheBloke | "2023-08-21T08:01:38Z" | 1,352,341 | 11 | transformers | [
"transformers",
"safetensors",
"opt",
"text-generation",
"galactica",
"wizardlm",
"alpaca",
"dataset:WizardLM/WizardLM_evol_instruct_70k",
"arxiv:2304.12244",
"license:other",
"autotrain_compatible",
"text-generation-inference",
"4-bit",
"gptq",
"region:us"
] | text-generation | "2023-06-17T19:52:11Z" | ---
inference: false
license: other
datasets:
- WizardLM/WizardLM_evol_instruct_70k
library_name: transformers
pipeline_tag: text-generation
tags:
- galactica
- wizardlm
- alpaca
- opt
---
<!-- header start -->
<!-- 200823 -->
<div style="width: auto; margin-left: auto; margin-right: auto">
<img src="https://i.imgur.com/EBdldam.jpg" alt="TheBlokeAI" style="width: 100%; min-width: 400px; display: block; margin: auto;">
</div>
<div style="display: flex; justify-content: space-between; width: 100%;">
<div style="display: flex; flex-direction: column; align-items: flex-start;">
<p style="margin-top: 0.5em; margin-bottom: 0em;"><a href="https://discord.gg/theblokeai">Chat & support: TheBloke's Discord server</a></p>
</div>
<div style="display: flex; flex-direction: column; align-items: flex-end;">
<p style="margin-top: 0.5em; margin-bottom: 0em;"><a href="https://www.patreon.com/TheBlokeAI">Want to contribute? TheBloke's Patreon page</a></p>
</div>
</div>
<div style="text-align:center; margin-top: 0em; margin-bottom: 0em"><p style="margin-top: 0.25em; margin-bottom: 0em;">TheBloke's LLM work is generously supported by a grant from <a href="https://a16z.com">andreessen horowitz (a16z)</a></p></div>
<hr style="margin-top: 1.0em; margin-bottom: 1.0em;">
<!-- header end -->
# Georgia Tech Research Institute's Galactica 30B Evol Instruct 70K GPTQ
These files are GPTQ 4bit model files for [Georgia Tech Research Institute's Galactica 30B Evol Instruct 70K](https://huggingface.co/GeorgiaTechResearchInstitute/galactica-30b-evol-instruct-70k).
It is the result of quantising to 4bit using [AutoGPTQ](https://github.com/PanQiWei/AutoGPTQ).
## Repositories available
* [4-bit GPTQ models for GPU inference](https://huggingface.co/TheBloke/galactica-30B-evol-instruct-70K-GPTQ)
* [Unquantised fp16 model in pytorch format, for GPU inference and for further conversions](https://huggingface.co/GeorgiaTechResearchInstitute/galactica-30b-evol-instruct-70k)
## Prompt template
```
### Instruction:
prompt
### Response:
```
## How to easily download and use this model in text-generation-webui
Please make sure you're using the latest version of text-generation-webui
1. Click the **Model tab**.
2. Under **Download custom model or LoRA**, enter `TheBloke/galactica-30B-evol-instruct-70K-GPTQ`.
3. Click **Download**.
4. The model will start downloading. Once it's finished it will say "Done"
5. In the top left, click the refresh icon next to **Model**.
6. In the **Model** dropdown, choose the model you just downloaded: `galactica-30B-evol-instruct-70K-GPTQ`
7. The model will automatically load, and is now ready for use!
8. If you want any custom settings, set them and then click **Save settings for this model** followed by **Reload the Model** in the top right.
* Note that you do not need to and should not set manual GPTQ parameters any more. These are set automatically from the file `quantize_config.json`.
9. Once you're ready, click the **Text Generation tab** and enter a prompt to get started!
## How to use this GPTQ model from Python code
First make sure you have [AutoGPTQ](https://github.com/PanQiWei/AutoGPTQ) installed:
`pip install auto-gptq`
Then try the following example code:
```python
from transformers import AutoTokenizer, pipeline, logging
from auto_gptq import AutoGPTQForCausalLM, BaseQuantizeConfig
import argparse
model_name_or_path = "TheBloke/galactica-30B-evol-instruct-70K-GPTQ"
model_basename = "model"
use_triton = False
tokenizer = AutoTokenizer.from_pretrained(model_name_or_path, use_fast=True)
model = AutoGPTQForCausalLM.from_quantized(model_name_or_path,
model_basename=model_basename,
use_safetensors=True,
trust_remote_code=False,
device="cuda:0",
use_triton=use_triton,
quantize_config=None)
# Note: check the prompt template is correct for this model.
prompt = "Tell me about AI"
prompt_template=f'''### Instruction: {prompt}
### Response:'''
print("\n\n*** Generate:")
input_ids = tokenizer(prompt_template, return_tensors='pt').input_ids.cuda()
output = model.generate(inputs=input_ids, temperature=0.7, max_new_tokens=512)
print(tokenizer.decode(output[0]))
# Inference can also be done using transformers' pipeline
# Prevent printing spurious transformers error when using pipeline with AutoGPTQ
logging.set_verbosity(logging.CRITICAL)
print("*** Pipeline:")
pipe = pipeline(
"text-generation",
model=model,
tokenizer=tokenizer,
max_new_tokens=512,
temperature=0.7,
top_p=0.95,
repetition_penalty=1.15
)
print(pipe(prompt_template)[0]['generated_text'])
```
## Provided files
**gptq_model-4bit--1g.safetensors**
This will work with AutoGPTQ and CUDA versions of GPTQ-for-LLaMa. There are reports of issues with Triton mode of recent GPTQ-for-LLaMa. If you have issues, please use AutoGPTQ instead.
It was created without group_size to lower VRAM requirements, and with --act-order (desc_act) to boost inference accuracy as much as possible.
* `gptq_model-4bit--1g.safetensors`
* Works with AutoGPTQ in CUDA or Triton modes.
* Works with GPTQ-for-LLaMa in CUDA mode. May have issues with GPTQ-for-LLaMa Triton mode.
* Works with text-generation-webui, including one-click-installers.
* Parameters: Groupsize = -1. Act Order / desc_act = True.
<!-- footer start -->
<!-- 200823 -->
## Discord
For further support, and discussions on these models and AI in general, join us at:
[TheBloke AI's Discord server](https://discord.gg/theblokeai)
## Thanks, and how to contribute.
Thanks to the [chirper.ai](https://chirper.ai) team!
I've had a lot of people ask if they can contribute. I enjoy providing models and helping people, and would love to be able to spend even more time doing it, as well as expanding into new projects like fine tuning/training.
If you're able and willing to contribute it will be most gratefully received and will help me to keep providing more models, and to start work on new AI projects.
Donaters will get priority support on any and all AI/LLM/model questions and requests, access to a private Discord room, plus other benefits.
* Patreon: https://patreon.com/TheBlokeAI
* Ko-Fi: https://ko-fi.com/TheBlokeAI
**Special thanks to**: Aemon Algiz.
**Patreon special mentions**: Sam, theTransient, Jonathan Leane, Steven Wood, webtim, Johann-Peter Hartmann, Geoffrey Montalvo, Gabriel Tamborski, Willem Michiel, John Villwock, Derek Yates, Mesiah Bishop, Eugene Pentland, Pieter, Chadd, Stephen Murray, Daniel P. Andersen, terasurfer, Brandon Frisco, Thomas Belote, Sid, Nathan LeClaire, Magnesian, Alps Aficionado, Stanislav Ovsiannikov, Alex, Joseph William Delisle, Nikolai Manek, Michael Davis, Junyu Yang, K, J, Spencer Kim, Stefan Sabev, Olusegun Samson, transmissions 11, Michael Levine, Cory Kujawski, Rainer Wilmers, zynix, Kalila, Luke @flexchar, Ajan Kanaga, Mandus, vamX, Ai Maven, Mano Prime, Matthew Berman, subjectnull, Vitor Caleffi, Clay Pascal, biorpg, alfie_i, 阿明, Jeffrey Morgan, ya boyyy, Raymond Fosdick, knownsqashed, Olakabola, Leonard Tan, ReadyPlayerEmma, Enrico Ros, Dave, Talal Aujan, Illia Dulskyi, Sean Connelly, senxiiz, Artur Olbinski, Elle, Raven Klaugh, Fen Risland, Deep Realms, Imad Khwaja, Fred von Graf, Will Dee, usrbinkat, SuperWojo, Alexandros Triantafyllidis, Swaroop Kallakuri, Dan Guido, John Detwiler, Pedro Madruga, Iucharbius, Viktor Bowallius, Asp the Wyvern, Edmond Seymore, Trenton Dambrowitz, Space Cruiser, Spiking Neurons AB, Pyrater, LangChain4j, Tony Hughes, Kacper Wikieł, Rishabh Srivastava, David Ziegler, Luke Pendergrass, Andrey, Gabriel Puliatti, Lone Striker, Sebastain Graf, Pierre Kircher, Randy H, NimbleBox.ai, Vadim, danny, Deo Leter
Thank you to all my generous patrons and donaters!
And thank you again to a16z for their generous grant.
<!-- footer end -->
# Original model card: Georgia Tech Research Institute's Galactica 30B Evol Instruct 70K
# GALACTICA 30B Evol-Instruct
GALACTICA 30B fine-tuned on the Evol-Instruct 70k dataset.
The model card from the original Galactica repo can be found [here](https://github.com/paperswithcode/galai/blob/main/docs/model_card.md), and the original paper [here](https://galactica.org/paper.pdf).
The HF dataset for Evol-Instruct-70k can be found [here](https://huggingface.co/datasets/WizardLM/WizardLM_evol_instruct_70k), and the original GitHub repo for WizardLM is [here](https://github.com/nlpxucan/WizardLM).
## Model Details
The GALACTICA models are trained on a large-scale scientific corpus and are designed to perform scientific tasks.
Evol-instruct-70k is a dataset of evolved instruction-response pairs generated via the Evol-Instruct framework using gpt-3.5-turbo.
## Model Use
The GALACTICA model card specifies that the primary indended users of the GALACTICA models are researchers studying language models applied to the scientific domain, and it cautions against production use of GALACTICA without safeguards due to the potential for the model to produce inaccurate information.
The original GALACTICA models are available under a non-commercial CC BY-NC 4.0 license, and models based on the Evol-Instruct-70k dataset are additionally subject to the [OpenAI Terms of Service](https://openai.com/policies/terms-of-use).
<details>
<summary> Example Outputs </summary>
*__Full Prompt:__*
```
### Instruction:
Write out and explain Maxwell's equations using markdown and latex syntax. Highlight the key implication of each equation.
### Response:
```
*__Response:__*
Maxwell's equations are a set of four equations that describe the behavior of electric and magnetic fields. Here are the equations in both markdown and latex syntax:
- **Gauss's Law for Electric Fields:**
$$\nabla \cdot \mathbf{E} = \frac{\rho}{\epsilon_0}$$
This equation states that the divergence of the electric field E is proportional to the charge density ρ divided by the vacuum permittivity ε0. This means that if there is a non-zero charge density, there must be a non-zero electric field surrounding it.
- **Gauss's Law for Magnetic Fields:**
$$\nabla \cdot \mathbf{B} = 0$$
This equation states that the divergence of the magnetic field B is always zero. This means that magnetic monopoles do not exist, and magnetic fields are always created by the motion of electric charges.
- **Maxwell-Faraday Equation:**
$$\nabla \times \mathbf{E} = -\frac{\partial \mathbf{B}}{\partial t}$$
This equation states that the curl of the electric field is equal to the negative of the time derivative of the magnetic field. This means that changing magnetic fields can create electric fields, and vice versa.
- **Ampere's Law with Maxwell's Addition:**
$$\nabla \times \mathbf{B} = \mu_0 \left( \mathbf{J} + \epsilon_0 \frac{\partial \mathbf{E}}{\partial t} \right)$$
This equation states that the curl of the magnetic field is equal to the product of the vacuum permeability μ0 and the sum of the electric current density J and the time derivative of the electric field. This means that changing electric fields can create magnetic fields, and that electric currents can also create magnetic fields.
**Implications**
- Gauss's Laws imply that electric charges must be conserved, since the net charge in a closed system must always be zero.
- The Maxwell-Faraday Equation implies that electromagnetic waves can travel through space, since changing magnetic fields can create electric fields, which can create changing magnetic fields, and so on. This is the basis for radio waves, microwaves, and other forms of electromagnetic radiation.
- Ampere's Law with Maxwell's Addition implies that electric currents can create magnetic fields, which can create electric currents, and so on. This is the basis for electrical generators and transformers.
Overall, Maxwell's equations are a fundamental framework for understanding the behavior of electric and magnetic fields, and have important applications in fields such as electromagnetism, radio technology, and electrical engineering.
</details>
## Training Data
This model was trained by fine-tuning pre-trained GALACTICA 30B on the Evol-Instruct-70k dataset. GALACTICA models were trained on 106 billion tokens of open-access scientific text and data, including papers, textbooks, scientific websites, encyclopedias, and more.
Fine-tuning the base GALACTICA models on the 70k instruction-response pairs in the Evol-Instruct-70k dataset allows users to query the resulting model in an instruct-response fashion.
## How to Use
The GALACTICA Evol-Instruct-70K weights are made available for use with the `transformers` library.
<details>
<summary> Click to expand </summary>
```python
# pip install accelerate
from transformers import AutoTokenizer, AutoModelForCausalLM
tokenizer = AutoTokenizer.from_pretrained("GeorgiaTechResearchInstitute/galactica-30b-evol-instruct-70k")
model = AutoModelForCausalLM.from_pretrained("GeorgiaTechResearchInstitute/galactica-30b-evol-instruct-70k", device_map="auto", torch_dtype=torch.bfloat16)
# the evol-instruct models were fine-tuned with the same hidden prompts as the Alpaca project
no_input_prompt_template = ("### Instruction:\n{instruction}\n\n### Response:")
prompt = "Write out Maxwell's equations and explain the meaning of each one."
formatted_prompt = no_input_prompt_template.format_map({'instruction': prompt})
tokenized_prompt = tokenizer(formatted_prompt, return_tensors="pt").input_ids.to(model.device)
out_tokens = model.generate(tokenized_prompt)
print(tokenizer.batch_decode(out_tokens, skip_special_tokens=False, clean_up_tokenization_spaces=False))
```
</details>
## Training Resources
GALACTICA 30B Evol-Instruct was fine-tuned in 196 hours using 16 A100 80GB GPUs, 16-bit mixed-precision, an effective batch-size of 128, and with a maximum context window of 1024 tokens. This model was trained using DeepSpeed ZeRO-3 optimizations.
## Performance and Limitations
Qualitative evaluation suggests that the evol-instruct-70k fine-tuned Galactica models are signficantly more controllable and attentive to user prompts than the Alpaca fine-tuned GALPACA models.
## Works Cited
```bibtex
@inproceedings{GALACTICA,
title={GALACTICA: A Large Language Model for Science},
author={Ross Taylor and Marcin Kardas and Guillem Cucurull and Thomas Scialom and Anthony Hartshorn and Elvis Saravia and Andrew Poulton and Viktor Kerkez and Robert Stojnic},
year={2022}
}
```
```bibtex
@misc{xu2023wizardlm,
title={WizardLM: Empowering Large Language Models to Follow Complex Instructions},
author={Can Xu and Qingfeng Sun and Kai Zheng and Xiubo Geng and Pu Zhao and Jiazhan Feng and Chongyang Tao and Daxin Jiang},
year={2023},
eprint={2304.12244},
archivePrefix={arXiv},
primaryClass={cs.CL}
}
```
|
meta-llama/Llama-3.2-1B | meta-llama | "2024-10-24T15:08:03Z" | 1,344,828 | 872 | transformers | [
"transformers",
"safetensors",
"llama",
"text-generation",
"facebook",
"meta",
"pytorch",
"llama-3",
"en",
"de",
"fr",
"it",
"pt",
"hi",
"es",
"th",
"arxiv:2204.05149",
"arxiv:2405.16406",
"license:llama3.2",
"autotrain_compatible",
"text-generation-inference",
"endpoints_compatible",
"region:us"
] | text-generation | "2024-09-18T15:03:14Z" | ---
language:
- en
- de
- fr
- it
- pt
- hi
- es
- th
library_name: transformers
pipeline_tag: text-generation
tags:
- facebook
- meta
- pytorch
- llama
- llama-3
license: llama3.2
extra_gated_prompt: >-
### LLAMA 3.2 COMMUNITY LICENSE AGREEMENT
Llama 3.2 Version Release Date: September 25, 2024
“Agreement” means the terms and conditions for use, reproduction, distribution
and modification of the Llama Materials set forth herein.
“Documentation” means the specifications, manuals and documentation accompanying Llama 3.2
distributed by Meta at https://llama.meta.com/doc/overview.
“Licensee” or “you” means you, or your employer or any other person or entity (if you are
entering into this Agreement on such person or entity’s behalf), of the age required under
applicable laws, rules or regulations to provide legal consent and that has legal authority
to bind your employer or such other person or entity if you are entering in this Agreement
on their behalf.
“Llama 3.2” means the foundational large language models and software and algorithms, including
machine-learning model code, trained model weights, inference-enabling code, training-enabling code,
fine-tuning enabling code and other elements of the foregoing distributed by Meta at
https://www.llama.com/llama-downloads.
“Llama Materials” means, collectively, Meta’s proprietary Llama 3.2 and Documentation (and
any portion thereof) made available under this Agreement.
“Meta” or “we” means Meta Platforms Ireland Limited (if you are located in or,
if you are an entity, your principal place of business is in the EEA or Switzerland)
and Meta Platforms, Inc. (if you are located outside of the EEA or Switzerland).
By clicking “I Accept” below or by using or distributing any portion or element of the Llama Materials,
you agree to be bound by this Agreement.
1. License Rights and Redistribution.
a. Grant of Rights. You are granted a non-exclusive, worldwide,
non-transferable and royalty-free limited license under Meta’s intellectual property or other rights
owned by Meta embodied in the Llama Materials to use, reproduce, distribute, copy, create derivative works
of, and make modifications to the Llama Materials.
b. Redistribution and Use.
i. If you distribute or make available the Llama Materials (or any derivative works thereof),
or a product or service (including another AI model) that contains any of them, you shall (A) provide
a copy of this Agreement with any such Llama Materials; and (B) prominently display “Built with Llama”
on a related website, user interface, blogpost, about page, or product documentation. If you use the
Llama Materials or any outputs or results of the Llama Materials to create, train, fine tune, or
otherwise improve an AI model, which is distributed or made available, you shall also include “Llama”
at the beginning of any such AI model name.
ii. If you receive Llama Materials, or any derivative works thereof, from a Licensee as part
of an integrated end user product, then Section 2 of this Agreement will not apply to you.
iii. You must retain in all copies of the Llama Materials that you distribute the
following attribution notice within a “Notice” text file distributed as a part of such copies:
“Llama 3.2 is licensed under the Llama 3.2 Community License, Copyright © Meta Platforms,
Inc. All Rights Reserved.”
iv. Your use of the Llama Materials must comply with applicable laws and regulations
(including trade compliance laws and regulations) and adhere to the Acceptable Use Policy for
the Llama Materials (available at https://www.llama.com/llama3_2/use-policy), which is hereby
incorporated by reference into this Agreement.
2. Additional Commercial Terms. If, on the Llama 3.2 version release date, the monthly active users
of the products or services made available by or for Licensee, or Licensee’s affiliates,
is greater than 700 million monthly active users in the preceding calendar month, you must request
a license from Meta, which Meta may grant to you in its sole discretion, and you are not authorized to
exercise any of the rights under this Agreement unless or until Meta otherwise expressly grants you such rights.
3. Disclaimer of Warranty. UNLESS REQUIRED BY APPLICABLE LAW, THE LLAMA MATERIALS AND ANY OUTPUT AND
RESULTS THEREFROM ARE PROVIDED ON AN “AS IS” BASIS, WITHOUT WARRANTIES OF ANY KIND, AND META DISCLAIMS
ALL WARRANTIES OF ANY KIND, BOTH EXPRESS AND IMPLIED, INCLUDING, WITHOUT LIMITATION, ANY WARRANTIES
OF TITLE, NON-INFRINGEMENT, MERCHANTABILITY, OR FITNESS FOR A PARTICULAR PURPOSE. YOU ARE SOLELY RESPONSIBLE
FOR DETERMINING THE APPROPRIATENESS OF USING OR REDISTRIBUTING THE LLAMA MATERIALS AND ASSUME ANY RISKS ASSOCIATED
WITH YOUR USE OF THE LLAMA MATERIALS AND ANY OUTPUT AND RESULTS.
4. Limitation of Liability. IN NO EVENT WILL META OR ITS AFFILIATES BE LIABLE UNDER ANY THEORY OF LIABILITY,
WHETHER IN CONTRACT, TORT, NEGLIGENCE, PRODUCTS LIABILITY, OR OTHERWISE, ARISING OUT OF THIS AGREEMENT,
FOR ANY LOST PROFITS OR ANY INDIRECT, SPECIAL, CONSEQUENTIAL, INCIDENTAL, EXEMPLARY OR PUNITIVE DAMAGES, EVEN
IF META OR ITS AFFILIATES HAVE BEEN ADVISED OF THE POSSIBILITY OF ANY OF THE FOREGOING.
5. Intellectual Property.
a. No trademark licenses are granted under this Agreement, and in connection with the Llama Materials,
neither Meta nor Licensee may use any name or mark owned by or associated with the other or any of its affiliates,
except as required for reasonable and customary use in describing and redistributing the Llama Materials or as
set forth in this Section 5(a). Meta hereby grants you a license to use “Llama” (the “Mark”) solely as required
to comply with the last sentence of Section 1.b.i. You will comply with Meta’s brand guidelines (currently accessible
at https://about.meta.com/brand/resources/meta/company-brand/). All goodwill arising out of your use of the Mark
will inure to the benefit of Meta.
b. Subject to Meta’s ownership of Llama Materials and derivatives made by or for Meta, with respect to any
derivative works and modifications of the Llama Materials that are made by you, as between you and Meta,
you are and will be the owner of such derivative works and modifications.
c. If you institute litigation or other proceedings against Meta or any entity (including a cross-claim or
counterclaim in a lawsuit) alleging that the Llama Materials or Llama 3.2 outputs or results, or any portion
of any of the foregoing, constitutes infringement of intellectual property or other rights owned or licensable
by you, then any licenses granted to you under this Agreement shall terminate as of the date such litigation or
claim is filed or instituted. You will indemnify and hold harmless Meta from and against any claim by any third
party arising out of or related to your use or distribution of the Llama Materials.
6. Term and Termination. The term of this Agreement will commence upon your acceptance of this Agreement or access
to the Llama Materials and will continue in full force and effect until terminated in accordance with the terms
and conditions herein. Meta may terminate this Agreement if you are in breach of any term or condition of this
Agreement. Upon termination of this Agreement, you shall delete and cease use of the Llama Materials. Sections 3,
4 and 7 shall survive the termination of this Agreement.
7. Governing Law and Jurisdiction. This Agreement will be governed and construed under the laws of the State of
California without regard to choice of law principles, and the UN Convention on Contracts for the International
Sale of Goods does not apply to this Agreement. The courts of California shall have exclusive jurisdiction of
any dispute arising out of this Agreement.
### Llama 3.2 Acceptable Use Policy
Meta is committed to promoting safe and fair use of its tools and features, including Llama 3.2.
If you access or use Llama 3.2, you agree to this Acceptable Use Policy (“**Policy**”).
The most recent copy of this policy can be found at
[https://www.llama.com/llama3_2/use-policy](https://www.llama.com/llama3_2/use-policy).
#### Prohibited Uses
We want everyone to use Llama 3.2 safely and responsibly. You agree you will not use, or allow others to use, Llama 3.2 to:
1. Violate the law or others’ rights, including to:
1. Engage in, promote, generate, contribute to, encourage, plan, incite, or further illegal or unlawful activity or content, such as:
1. Violence or terrorism
2. Exploitation or harm to children, including the solicitation, creation, acquisition, or dissemination of child exploitative content or failure to report Child Sexual Abuse Material
3. Human trafficking, exploitation, and sexual violence
4. The illegal distribution of information or materials to minors, including obscene materials, or failure to employ legally required age-gating in connection with such information or materials.
5. Sexual solicitation
6. Any other criminal activity
1. Engage in, promote, incite, or facilitate the harassment, abuse, threatening, or bullying of individuals or groups of individuals
2. Engage in, promote, incite, or facilitate discrimination or other unlawful or harmful conduct in the provision of employment, employment benefits, credit, housing, other economic benefits, or other essential goods and services
3. Engage in the unauthorized or unlicensed practice of any profession including, but not limited to, financial, legal, medical/health, or related professional practices
4. Collect, process, disclose, generate, or infer private or sensitive information about individuals, including information about individuals’ identity, health, or demographic information, unless you have obtained the right to do so in accordance with applicable law
5. Engage in or facilitate any action or generate any content that infringes, misappropriates, or otherwise violates any third-party rights, including the outputs or results of any products or services using the Llama Materials
6. Create, generate, or facilitate the creation of malicious code, malware, computer viruses or do anything else that could disable, overburden, interfere with or impair the proper working, integrity, operation or appearance of a website or computer system
7. Engage in any action, or facilitate any action, to intentionally circumvent or remove usage restrictions or other safety measures, or to enable functionality disabled by Meta
2. Engage in, promote, incite, facilitate, or assist in the planning or development of activities that present a risk of death or bodily harm to individuals, including use of Llama 3.2 related to the following:
8. Military, warfare, nuclear industries or applications, espionage, use for materials or activities that are subject to the International Traffic Arms Regulations (ITAR) maintained by the United States Department of State or to the U.S. Biological Weapons Anti-Terrorism Act of 1989 or the Chemical Weapons Convention Implementation Act of 1997
9. Guns and illegal weapons (including weapon development)
10. Illegal drugs and regulated/controlled substances
11. Operation of critical infrastructure, transportation technologies, or heavy machinery
12. Self-harm or harm to others, including suicide, cutting, and eating disorders
13. Any content intended to incite or promote violence, abuse, or any infliction of bodily harm to an individual
3. Intentionally deceive or mislead others, including use of Llama 3.2 related to the following:
14. Generating, promoting, or furthering fraud or the creation or promotion of disinformation
15. Generating, promoting, or furthering defamatory content, including the creation of defamatory statements, images, or other content
16. Generating, promoting, or further distributing spam
17. Impersonating another individual without consent, authorization, or legal right
18. Representing that the use of Llama 3.2 or outputs are human-generated
19. Generating or facilitating false online engagement, including fake reviews and other means of fake online engagement
4. Fail to appropriately disclose to end users any known dangers of your AI system
5. Interact with third party tools, models, or software designed to generate unlawful content or engage in unlawful or harmful conduct and/or represent that the outputs of such tools, models, or software are associated with Meta or Llama 3.2
With respect to any multimodal models included in Llama 3.2, the rights granted under Section 1(a) of the Llama 3.2 Community License Agreement are not being granted to you if you are an individual domiciled in, or a company with a principal place of business in, the European Union. This restriction does not apply to end users of a product or service that incorporates any such multimodal models.
Please report any violation of this Policy, software “bug,” or other problems that could lead to a violation of this Policy through one of the following means:
* Reporting issues with the model: [https://github.com/meta-llama/llama-models/issues](https://l.workplace.com/l.php?u=https%3A%2F%2Fgithub.com%2Fmeta-llama%2Fllama-models%2Fissues&h=AT0qV8W9BFT6NwihiOHRuKYQM_UnkzN_NmHMy91OT55gkLpgi4kQupHUl0ssR4dQsIQ8n3tfd0vtkobvsEvt1l4Ic6GXI2EeuHV8N08OG2WnbAmm0FL4ObkazC6G_256vN0lN9DsykCvCqGZ)
* Reporting risky content generated by the model: [developers.facebook.com/llama_output_feedback](http://developers.facebook.com/llama_output_feedback)
* Reporting bugs and security concerns: [facebook.com/whitehat/info](http://facebook.com/whitehat/info)
* Reporting violations of the Acceptable Use Policy or unlicensed uses of Llama 3.2: [email protected]
extra_gated_fields:
First Name: text
Last Name: text
Date of birth: date_picker
Country: country
Affiliation: text
Job title:
type: select
options:
- Student
- Research Graduate
- AI researcher
- AI developer/engineer
- Reporter
- Other
geo: ip_location
By clicking Submit below I accept the terms of the license and acknowledge that the information I provide will be collected stored processed and shared in accordance with the Meta Privacy Policy: checkbox
extra_gated_description: >-
The information you provide will be collected, stored, processed and shared in
accordance with the [Meta Privacy
Policy](https://www.facebook.com/privacy/policy/).
extra_gated_button_content: Submit
---
## Model Information
The Llama 3.2 collection of multilingual large language models (LLMs) is a collection of pretrained and instruction-tuned generative models in 1B and 3B sizes (text in/text out). The Llama 3.2 instruction-tuned text only models are optimized for multilingual dialogue use cases, including agentic retrieval and summarization tasks. They outperform many of the available open source and closed chat models on common industry benchmarks.
**Model Developer:** Meta
**Model Architecture:** Llama 3.2 is an auto-regressive language model that uses an optimized transformer architecture. The tuned versions use supervised fine-tuning (SFT) and reinforcement learning with human feedback (RLHF) to align with human preferences for helpfulness and safety.
| | Training Data | Params | Input modalities | Output modalities | Context Length | GQA | Shared Embeddings | Token count | Knowledge cutoff |
| :---- | :---- | :---- | :---- | :---- | :---- | :---- | :---- | :---- | :---- |
| Llama 3.2 (text only) | A new mix of publicly available online data. | 1B (1.23B) | Multilingual Text | Multilingual Text and code | 128k | Yes | Yes | Up to 9T tokens | December 2023 |
| | | 3B (3.21B) | Multilingual Text | Multilingual Text and code | | | | | |
| Llama 3.2 Quantized (text only) | A new mix of publicly available online data. | 1B (1.23B) | Multilingual Text | Multilingual Text and code | 8k | Yes | Yes | Up to 9T tokens | December 2023 |
| | | 3B (3.21B) | Multilingual Text | Multilingual Text and code | | | | | |
**Supported Languages:** English, German, French, Italian, Portuguese, Hindi, Spanish, and Thai are officially supported. Llama 3.2 has been trained on a broader collection of languages than these 8 supported languages. Developers may fine-tune Llama 3.2 models for languages beyond these supported languages, provided they comply with the Llama 3.2 Community License and the Acceptable Use Policy. Developers are always expected to ensure that their deployments, including those that involve additional languages, are completed safely and responsibly.
**Llama 3.2 Model Family:** Token counts refer to pretraining data only. All model versions use Grouped-Query Attention (GQA) for improved inference scalability.
**Model Release Date:** Sept 25, 2024
**Status:** This is a static model trained on an offline dataset. Future versions may be released that improve model capabilities and safety.
**License:** Use of Llama 3.2 is governed by the [Llama 3.2 Community License](https://github.com/meta-llama/llama-models/blob/main/models/llama3_2/LICENSE) (a custom, commercial license agreement).
**Feedback:** Instructions on how to provide feedback or comments on the model can be found in the Llama Models [README](https://github.com/meta-llama/llama-models/blob/main/README.md). For more technical information about generation parameters and recipes for how to use Llama 3.2 in applications, please go [here](https://github.com/meta-llama/llama-recipes).
## Intended Use
**Intended Use Cases:** Llama 3.2 is intended for commercial and research use in multiple languages. Instruction tuned text only models are intended for assistant-like chat and agentic applications like knowledge retrieval and summarization, mobile AI powered writing assistants and query and prompt rewriting. Pretrained models can be adapted for a variety of additional natural language generation tasks. Similarly, quantized models can be adapted for a variety of on-device use-cases with limited compute resources.
**Out of Scope:** Use in any manner that violates applicable laws or regulations (including trade compliance laws). Use in any other way that is prohibited by the Acceptable Use Policy and Llama 3.2 Community License. Use in languages beyond those explicitly referenced as supported in this model card.
## How to use
This repository contains two versions of Llama-3.2-1B, for use with transformers and with the original `llama` codebase.
### Use with transformers
Starting with transformers >= 4.43.0 onward, you can run conversational inference using the Transformers pipeline abstraction or by leveraging the Auto classes with the generate() function.
Make sure to update your transformers installation via pip install --upgrade transformers.
```python
import torch
from transformers import pipeline
model_id = "meta-llama/Llama-3.2-1B"
pipe = pipeline(
"text-generation",
model=model_id,
torch_dtype=torch.bfloat16,
device_map="auto"
)
pipe("The key to life is")
```
### Use with `llama`
Please, follow the instructions in the [repository](https://github.com/meta-llama/llama).
To download Original checkpoints, see the example command below leveraging `huggingface-cli`:
```
huggingface-cli download meta-llama/Llama-3.2-1B --include "original/*" --local-dir Llama-3.2-1B
```
## Hardware and Software
**Training Factors:** We used custom training libraries, Meta's custom built GPU cluster, and production infrastructure for pretraining. Fine-tuning, quantization, annotation, and evaluation were also performed on production infrastructure.
**Training Energy Use:** Training utilized a cumulative of **916k** GPU hours of computation on H100-80GB (TDP of 700W) type hardware, per the table below. Training time is the total GPU time required for training each model and power consumption is the peak power capacity per GPU device used, adjusted for power usage efficiency.
**Training Greenhouse Gas Emissions:** Estimated total location-based greenhouse gas emissions were **240** tons CO2eq for training. Since 2020, Meta has maintained net zero greenhouse gas emissions in its global operations and matched 100% of its electricity use with renewable energy; therefore, the total market-based greenhouse gas emissions for training were 0 tons CO2eq.
| | Training Time (GPU hours) | Logit Generation Time (GPU Hours) | Training Power Consumption (W) | Training Location-Based Greenhouse Gas Emissions (tons CO2eq) | Training Market-Based Greenhouse Gas Emissions (tons CO2eq) |
| :---- | :---: | ----- | :---: | :---: | :---: |
| Llama 3.2 1B | 370k | \- | 700 | 107 | 0 |
| Llama 3.2 3B | 460k | \- | 700 | 133 | 0 |
| Llama 3.2 1B SpinQuant | 1.7 | 0 | 700 | *Negligible*\*\* | 0 |
| Llama 3.2 3B SpinQuant | 2.4 | 0 | 700 | *Negligible*\*\* | 0 |
| Llama 3.2 1B QLora | 1.3k | 0 | 700 | 0.381 | 0 |
| Llama 3.2 3B QLora | 1.6k | 0 | 700 | 0.461 | 0 |
| Total | 833k | 86k | | 240 | 0 |
\*\* The location-based CO2e emissions of Llama 3.2 1B SpinQuant and Llama 3.2 3B SpinQuant are less than 0.001 metric tonnes each. This is due to the minimal training GPU hours that are required.
The methodology used to determine training energy use and greenhouse gas emissions can be found [here](https://arxiv.org/pdf/2204.05149). Since Meta is openly releasing these models, the training energy use and greenhouse gas emissions will not be incurred by others.
## Training Data
**Overview:** Llama 3.2 was pretrained on up to 9 trillion tokens of data from publicly available sources. For the 1B and 3B Llama 3.2 models, we incorporated logits from the Llama 3.1 8B and 70B models into the pretraining stage of the model development, where outputs (logits) from these larger models were used as token-level targets. Knowledge distillation was used after pruning to recover performance. In post-training we used a similar recipe as Llama 3.1 and produced final chat models by doing several rounds of alignment on top of the pre-trained model. Each round involved Supervised Fine-Tuning (SFT), Rejection Sampling (RS), and Direct Preference Optimization (DPO).
**Data Freshness:** The pretraining data has a cutoff of December 2023\.
## Quantization
### Quantization Scheme
We designed the current quantization scheme with the [PyTorch’s ExecuTorch](https://github.com/pytorch/executorch) inference framework and Arm CPU backend in mind, taking into account metrics including model quality, prefill/decoding speed, and memory footprint. Our quantization scheme involves three parts:
- All linear layers in all transformer blocks are quantized to a 4-bit groupwise scheme (with a group size of 32) for weights and 8-bit per-token dynamic quantization for activations.
- The classification layer is quantized to 8-bit per-channel for weight and 8-bit per token dynamic quantization for activation.
- Similar to classification layer, an 8-bit per channel quantization is used for embedding layer.
### Quantization-Aware Training and LoRA
The quantization-aware training (QAT) with low-rank adaptation (LoRA) models went through only post-training stages, using the same data as the full precision models. To initialize QAT, we utilize BF16 Llama 3.2 model checkpoints obtained after supervised fine-tuning (SFT) and perform an additional full round of SFT training with QAT. We then freeze the backbone of the QAT model and perform another round of SFT with LoRA adaptors applied to all layers within the transformer block. Meanwhile, the LoRA adaptors' weights and activations are maintained in BF16. Because our approach is similar to QLoRA of Dettmers et al., (2023) (i.e., quantization followed by LoRA adapters), we refer this method as QLoRA. Finally, we fine-tune the resulting model (both backbone and LoRA adaptors) using direct preference optimization (DPO).
### SpinQuant
[SpinQuant](https://arxiv.org/abs/2405.16406) was applied, together with generative post-training quantization (GPTQ). For the SpinQuant rotation matrix fine-tuning, we optimized for 100 iterations, using 800 samples with sequence-length 2048 from the WikiText 2 dataset. For GPTQ, we used 128 samples from the same dataset with the same sequence-length.
## Benchmarks \- English Text
In this section, we report the results for Llama 3.2 models on standard automatic benchmarks. For all these evaluations, we used our internal evaluations library.
### Base Pretrained Models
| Category | Benchmark | \# Shots | Metric | Llama 3.2 1B | Llama 3.2 3B | Llama 3.1 8B |
| ----- | ----- | :---: | :---: | :---: | :---: | :---: |
| General | MMLU | 5 | macro\_avg/acc\_char | 32.2 | 58 | 66.7 |
| | AGIEval English | 3-5 | average/acc\_char | 23.3 | 39.2 | 47.8 |
| | ARC-Challenge | 25 | acc\_char | 32.8 | 69.1 | 79.7 |
| Reading comprehension | SQuAD | 1 | em | 49.2 | 67.7 | 77 |
| | QuAC (F1) | 1 | f1 | 37.9 | 42.9 | 44.9 |
| | DROP (F1) | 3 | f1 | 28.0 | 45.2 | 59.5 |
| Long Context | Needle in Haystack | 0 | em | 96.8 | 1 | 1 |
### Instruction Tuned Models
| Capability | | Benchmark | \# Shots | Metric | Llama 3.2 1B bf16 | Llama 3.2 1B Vanilla PTQ\*\* | Llama 3.2 1B Spin Quant | Llama 3.2 1B QLoRA | Llama 3.2 3B bf16 | Llama 3.2 3B Vanilla PTQ\*\* | Llama 3.2 3B Spin Quant | Llama 3.2 3B QLoRA | Llama 3.1 8B |
| :---: | ----- | :---: | :---: | :---: | :---: | :---: | :---: | :---: | :---: | :---: | :---: | :---: | :---: |
| General | | MMLU | 5 | macro\_avg/acc | 49.3 | 43.3 | 47.3 | 49.0 | 63.4 | 60.5 | 62 | 62.4 | 69.4 |
| Re-writing | | Open-rewrite eval | 0 | micro\_avg/rougeL | 41.6 | 39.2 | 40.9 | 41.2 | 40.1 | 40.3 | 40.8 | 40.7 | 40.9 |
| Summarization | | TLDR9+ (test) | 1 | rougeL | 16.8 | 14.9 | 16.7 | 16.8 | 19.0 | 19.1 | 19.2 | 19.1 | 17.2 |
| Instruction following | | IFEval | 0 | Avg(Prompt/Instruction acc Loose/Strict) | 59.5 | 51.5 | 58.4 | 55.6 | 77.4 | 73.9 | 73.5 | 75.9 | 80.4 |
| Math | | GSM8K (CoT) | 8 | em\_maj1@1 | 44.4 | 33.1 | 40.6 | 46.5 | 77.7 | 72.9 | 75.7 | 77.9 | 84.5 |
| | | MATH (CoT) | 0 | final\_em | 30.6 | 20.5 | 25.3 | 31.0 | 48.0 | 44.2 | 45.3 | 49.2 | 51.9 |
| Reasoning | | ARC-C | 0 | acc | 59.4 | 54.3 | 57 | 60.7 | 78.6 | 75.6 | 77.6 | 77.6 | 83.4 |
| | | GPQA | 0 | acc | 27.2 | 25.9 | 26.3 | 25.9 | 32.8 | 32.8 | 31.7 | 33.9 | 32.8 |
| | | Hellaswag | 0 | acc | 41.2 | 38.1 | 41.3 | 41.5 | 69.8 | 66.3 | 68 | 66.3 | 78.7 |
| Tool Use | | BFCL V2 | 0 | acc | 25.7 | 14.3 | 15.9 | 23.7 | 67.0 | 53.4 | 60.1 | 63.5 | 67.1 |
| | | Nexus | 0 | macro\_avg/acc | 13.5 | 5.2 | 9.6 | 12.5 | 34.3 | 32.4 | 31.5 | 30.1 | 38.5 |
| Long Context | | InfiniteBench/En.QA | 0 | longbook\_qa/f1 | 20.3 | N/A | N/A | N/A | 19.8 | N/A | N/A | N/A | 27.3 |
| | | InfiniteBench/En.MC | 0 | longbook\_choice/acc | 38.0 | N/A | N/A | N/A | 63.3 | N/A | N/A | N/A | 72.2 |
| | | NIH/Multi-needle | 0 | recall | 75.0 | N/A | N/A | N/A | 84.7 | N/A | N/A | N/A | 98.8 |
| Multilingual | | MGSM (CoT) | 0 | em | 24.5 | 13.7 | 18.2 | 24.4 | 58.2 | 48.9 | 54.3 | 56.8 | 68.9 |
\*\*for comparison purposes only. Model not released.
### Multilingual Benchmarks
| Category | Benchmark | Language | Llama 3.2 1B | Llama 3.2 1B Vanilla PTQ\*\* | Llama 3.2 1B Spin Quant | Llama 3.2 1B QLoRA | Llama 3.2 3B | Llama 3.2 3B Vanilla PTQ\*\* | Llama 3.2 3B Spin Quant | Llama 3.2 3B QLoRA | Llama 3.1 8B |
| :---: | :---: | :---: | :---: | :---: | :---: | :---: | :---: | :---: | :---: | :---: | :---: |
| General | MMLU (5-shot, macro_avg/acc) | Portuguese | 39.8 | 34.9 | 38.9 | 40.2 | 54.5 | 50.9 | 53.3 | 53.4 | 62.1 |
| | | Spanish | 41.5 | 36.0 | 39.8 | 41.8 | 55.1 | 51.9 | 53.6 | 53.6 | 62.5 |
| | | Italian | 39.8 | 34.9 | 38.1 | 40.6 | 53.8 | 49.9 | 52.1 | 51.7 | 61.6 |
| | | German | 39.2 | 34.9 | 37.5 | 39.6 | 53.3 | 50.0 | 52.2 | 51.3 | 60.6 |
| | | French | 40.5 | 34.8 | 39.2 | 40.8 | 54.6 | 51.2 | 53.3 | 53.3 | 62.3 |
| | | Hindi | 33.5 | 30.0 | 32.1 | 34.0 | 43.3 | 40.4 | 42.0 | 42.1 | 50.9 |
| | | Thai | 34.7 | 31.2 | 32.4 | 34.9 | 44.5 | 41.3 | 44.0 | 42.2 | 50.3 |
\*\*for comparison purposes only. Model not released.
## Inference time
In the below table, we compare the performance metrics of different quantization methods (SpinQuant and QAT \+ LoRA) with the BF16 baseline. The evaluation was done using the [ExecuTorch](https://github.com/pytorch/executorch) framework as the inference engine, with the ARM CPU as a backend using Android OnePlus 12 device.
| Category | Decode (tokens/sec) | Time-to-first-token (sec) | Prefill (tokens/sec) | Model size (PTE file size in MB) | Memory size (RSS in MB) |
| :---- | ----- | ----- | ----- | ----- | ----- |
| 1B BF16 (baseline) | 19.2 | 1.0 | 60.3 | 2358 | 3,185 |
| 1B SpinQuant | 50.2 (2.6x) | 0.3 (-76.9%) | 260.5 (4.3x) | 1083 (-54.1%) | 1,921 (-39.7%) |
| 1B QLoRA | 45.8 (2.4x) | 0.3 (-76.0%) | 252.0 (4.2x) | 1127 (-52.2%) | 2,255 (-29.2%) |
| 3B BF16 (baseline) | 7.6 | 3.0 | 21.2 | 6129 | 7,419 |
| 3B SpinQuant | 19.7 (2.6x) | 0.7 (-76.4%) | 89.7 (4.2x) | 2435 (-60.3%) | 3,726 (-49.8%) |
| 3B QLoRA | 18.5 (2.4x) | 0.7 (-76.1%) | 88.8 (4.2x) | 2529 (-58.7%) | 4,060 (-45.3%) |
(\*) The performance measurement is done using an adb binary-based approach.
(\*\*) It is measured on an Android OnePlus 12 device.
(\*\*\*) Time-to-first-token (TTFT) is measured with prompt length=64
*Footnote:*
- *Decode (tokens/second) is for how quickly it keeps generating. Higher is better.*
- *Time-to-first-token (TTFT for shorthand) is for how fast it generates the first token for a given prompt. Lower is better.*
- *Prefill is the inverse of TTFT (aka 1/TTFT) in tokens/second. Higher is better*
- *Model size \- how big is the model, measured by, PTE file, a binary file format for ExecuTorch*
- *RSS size \- Memory usage in resident set size (RSS)*
## Responsibility & Safety
As part of our Responsible release approach, we followed a three-pronged strategy to managing trust & safety risks:
1. Enable developers to deploy helpful, safe and flexible experiences for their target audience and for the use cases supported by Llama
2. Protect developers against adversarial users aiming to exploit Llama capabilities to potentially cause harm
3. Provide protections for the community to help prevent the misuse of our models
### Responsible Deployment
**Approach:** Llama is a foundational technology designed to be used in a variety of use cases. Examples on how Meta’s Llama models have been responsibly deployed can be found in our [Community Stories webpage](https://llama.meta.com/community-stories/). Our approach is to build the most helpful models, enabling the world to benefit from the technology power, by aligning our model safety for generic use cases and addressing a standard set of harms. Developers are then in the driver’s seat to tailor safety for their use cases, defining their own policies and deploying the models with the necessary safeguards in their Llama systems. Llama 3.2 was developed following the best practices outlined in our [Responsible Use Guide](https://llama.meta.com/responsible-use-guide/).
#### Llama 3.2 Instruct
**Objective:** Our main objectives for conducting safety fine-tuning are to provide the research community with a valuable resource for studying the robustness of safety fine-tuning, as well as to offer developers a readily available, safe, and powerful model for various applications to reduce the developer workload to deploy safe AI systems. We implemented the same set of safety mitigations as in Llama 3, and you can learn more about these in the Llama 3 [paper](https://ai.meta.com/research/publications/the-llama-3-herd-of-models/).
**Fine-Tuning Data:** We employ a multi-faceted approach to data collection, combining human-generated data from our vendors with synthetic data to mitigate potential safety risks. We’ve developed many large language model (LLM)-based classifiers that enable us to thoughtfully select high-quality prompts and responses, enhancing data quality control.
**Refusals and Tone:** Building on the work we started with Llama 3, we put a great emphasis on model refusals to benign prompts as well as refusal tone. We included both borderline and adversarial prompts in our safety data strategy, and modified our safety data responses to follow tone guidelines.
#### Llama 3.2 Systems
**Safety as a System:** Large language models, including Llama 3.2, **are not designed to be deployed in isolation** but instead should be deployed as part of an overall AI system with additional safety guardrails as required. Developers are expected to deploy system safeguards when building agentic systems. Safeguards are key to achieve the right helpfulness-safety alignment as well as mitigating safety and security risks inherent to the system and any integration of the model or system with external tools. As part of our responsible release approach, we provide the community with [safeguards](https://llama.meta.com/trust-and-safety/) that developers should deploy with Llama models or other LLMs, including Llama Guard, Prompt Guard and Code Shield. All our [reference implementations](https://github.com/meta-llama/llama-agentic-system) demos contain these safeguards by default so developers can benefit from system-level safety out-of-the-box.
### New Capabilities and Use Cases
**Technological Advancement:** Llama releases usually introduce new capabilities that require specific considerations in addition to the best practices that generally apply across all Generative AI use cases. For prior release capabilities also supported by Llama 3.2, see [Llama 3.1 Model Card](https://github.com/meta-llama/llama-models/blob/main/models/llama3_1/MODEL_CARD.md), as the same considerations apply here as well.
**Constrained Environments:** Llama 3.2 1B and 3B models are expected to be deployed in highly constrained environments, such as mobile devices. LLM Systems using smaller models will have a different alignment profile and safety/helpfulness tradeoff than more complex, larger systems. Developers should ensure the safety of their system meets the requirements of their use case. We recommend using lighter system safeguards for such use cases, like Llama Guard 3-1B or its mobile-optimized version.
### Evaluations
**Scaled Evaluations:** We built dedicated, adversarial evaluation datasets and evaluated systems composed of Llama models and Purple Llama safeguards to filter input prompt and output response. It is important to evaluate applications in context, and we recommend building dedicated evaluation dataset for your use case.
**Red Teaming:** We conducted recurring red teaming exercises with the goal of discovering risks via adversarial prompting and we used the learnings to improve our benchmarks and safety tuning datasets. We partnered early with subject-matter experts in critical risk areas to understand the nature of these real-world harms and how such models may lead to unintended harm for society. Based on these conversations, we derived a set of adversarial goals for the red team to attempt to achieve, such as extracting harmful information or reprogramming the model to act in a potentially harmful capacity. The red team consisted of experts in cybersecurity, adversarial machine learning, responsible AI, and integrity in addition to multilingual content specialists with background in integrity issues in specific geographic markets.
### Critical Risks
In addition to our safety work above, we took extra care on measuring and/or mitigating the following critical risk areas:
**1\. CBRNE (Chemical, Biological, Radiological, Nuclear, and Explosive Weapons):** Llama 3.2 1B and 3B models are smaller and less capable derivatives of Llama 3.1. For Llama 3.1 70B and 405B, to assess risks related to proliferation of chemical and biological weapons, we performed uplift testing designed to assess whether use of Llama 3.1 models could meaningfully increase the capabilities of malicious actors to plan or carry out attacks using these types of weapons and have determined that such testing also applies to the smaller 1B and 3B models.
**2\. Child Safety:** Child Safety risk assessments were conducted using a team of experts, to assess the model’s capability to produce outputs that could result in Child Safety risks and inform on any necessary and appropriate risk mitigations via fine tuning. We leveraged those expert red teaming sessions to expand the coverage of our evaluation benchmarks through Llama 3 model development. For Llama 3, we conducted new in-depth sessions using objective based methodologies to assess the model risks along multiple attack vectors including the additional languages Llama 3 is trained on. We also partnered with content specialists to perform red teaming exercises assessing potentially violating content while taking account of market specific nuances or experiences.
**3\. Cyber Attacks:** For Llama 3.1 405B, our cyber attack uplift study investigated whether LLMs can enhance human capabilities in hacking tasks, both in terms of skill level and speed.
Our attack automation study focused on evaluating the capabilities of LLMs when used as autonomous agents in cyber offensive operations, specifically in the context of ransomware attacks. This evaluation was distinct from previous studies that considered LLMs as interactive assistants. The primary objective was to assess whether these models could effectively function as independent agents in executing complex cyber-attacks without human intervention. Because Llama 3.2’s 1B and 3B models are smaller and less capable models than Llama 3.1 405B, we broadly believe that the testing conducted for the 405B model also applies to Llama 3.2 models.
### Community
**Industry Partnerships:** Generative AI safety requires expertise and tooling, and we believe in the strength of the open community to accelerate its progress. We are active members of open consortiums, including the AI Alliance, Partnership on AI and MLCommons, actively contributing to safety standardization and transparency. We encourage the community to adopt taxonomies like the MLCommons Proof of Concept evaluation to facilitate collaboration and transparency on safety and content evaluations. Our Purple Llama tools are open sourced for the community to use and widely distributed across ecosystem partners including cloud service providers. We encourage community contributions to our [Github repository](https://github.com/meta-llama/PurpleLlama).
**Grants:** We also set up the [Llama Impact Grants](https://llama.meta.com/llama-impact-grants/) program to identify and support the most compelling applications of Meta’s Llama model for societal benefit across three categories: education, climate and open innovation. The 20 finalists from the hundreds of applications can be found [here](https://llama.meta.com/llama-impact-grants/#finalists).
**Reporting:** Finally, we put in place a set of resources including an [output reporting mechanism](https://developers.facebook.com/llama_output_feedback) and [bug bounty program](https://www.facebook.com/whitehat) to continuously improve the Llama technology with the help of the community.
## Ethical Considerations and Limitations
**Values:** The core values of Llama 3.2 are openness, inclusivity and helpfulness. It is meant to serve everyone, and to work for a wide range of use cases. It is thus designed to be accessible to people across many different backgrounds, experiences and perspectives. Llama 3.2 addresses users and their needs as they are, without insertion unnecessary judgment or normativity, while reflecting the understanding that even content that may appear problematic in some cases can serve valuable purposes in others. It respects the dignity and autonomy of all users, especially in terms of the values of free thought and expression that power innovation and progress.
**Testing:** Llama 3.2 is a new technology, and like any new technology, there are risks associated with its use. Testing conducted to date has not covered, nor could it cover, all scenarios. For these reasons, as with all LLMs, Llama 3.2’s potential outputs cannot be predicted in advance, and the model may in some instances produce inaccurate, biased or other objectionable responses to user prompts. Therefore, before deploying any applications of Llama 3.2 models, developers should perform safety testing and tuning tailored to their specific applications of the model. Please refer to available resources including our [Responsible Use Guide](https://llama.meta.com/responsible-use-guide), [Trust and Safety](https://llama.meta.com/trust-and-safety/) solutions, and other [resources](https://llama.meta.com/docs/get-started/) to learn more about responsible development.
|
CompVis/stable-diffusion-safety-checker | CompVis | "2022-11-25T17:21:38Z" | 1,333,291 | 119 | transformers | [
"transformers",
"pytorch",
"clip",
"arxiv:2103.00020",
"arxiv:1910.09700",
"endpoints_compatible",
"region:us"
] | null | "2022-08-22T10:22:34Z" | ---
tags:
- clip
---
# Model Card for stable-diffusion-safety-checker
# Model Details
## Model Description
More information needed
- **Developed by:** More information needed
- **Shared by [Optional]:** CompVis
- **Model type:** Image Identification
- **Language(s) (NLP):** More information needed
- **License:** More information needed
- **Parent Model:** [CLIP](https://huggingface.co/openai/clip-vit-large-patch14)
- **Resources for more information:**
- [CLIP Paper](https://arxiv.org/abs/2103.00020)
- [Stable Diffusion Model Card](https://github.com/CompVis/stable-diffusion/blob/main/Stable_Diffusion_v1_Model_Card.md)
# Uses
## Direct Use
This model can be used for identifying NSFW image
The CLIP model devlopers note in their [model card](https://huggingface.co/openai/clip-vit-large-patch14) :
>The primary intended users of these models are AI researchers.
We primarily imagine the model will be used by researchers to better understand robustness, generalization, and other capabilities, biases, and constraints of computer vision models.
## Downstream Use [Optional]
More information needed.
## Out-of-Scope Use
The model is not intended to be used with transformers but with diffusers. This model should also not be used to intentionally create hostile or alienating environments for people.
# Bias, Risks, and Limitations
Significant research has explored bias and fairness issues with language models (see, e.g., [Sheng et al. (2021)](https://aclanthology.org/2021.acl-long.330.pdf) and [Bender et al. (2021)](https://dl.acm.org/doi/pdf/10.1145/3442188.3445922)). Predictions generated by the model may include disturbing and harmful stereotypes across protected classes; identity characteristics; and sensitive, social, and occupational groups.
The CLIP model devlopers note in their [model card](https://huggingface.co/openai/clip-vit-large-patch14) :
> We find that the performance of CLIP - and the specific biases it exhibits - can depend significantly on class design and the choices one makes for categories to include and exclude. We tested the risk of certain kinds of denigration with CLIP by classifying images of people from Fairface into crime-related and non-human animal categories. We found significant disparities with respect to race and gender. Additionally, we found that these disparities could shift based on how the classes were constructed.
> We also tested the performance of CLIP on gender, race and age classification using the Fairface dataset (We default to using race categories as they are constructed in the Fairface dataset.) in order to assess quality of performance across different demographics. We found accuracy >96% across all races for gender classification with ‘Middle Eastern’ having the highest accuracy (98.4%) and ‘White’ having the lowest (96.5%). Additionally, CLIP averaged ~93% for racial classification and ~63% for age classification
## Recommendations
Users (both direct and downstream) should be made aware of the risks, biases and limitations of the model. More information needed for further recommendations.
# Training Details
## Training Data
More information needed
## Training Procedure
### Preprocessing
More information needed
### Speeds, Sizes, Times
More information needed
# Evaluation
## Testing Data, Factors & Metrics
### Testing Data
More information needed
### Factors
More information needed
### Metrics
More information needed
## Results
More information needed
# Model Examination
More information needed
# Environmental Impact
Carbon emissions can be estimated using the [Machine Learning Impact calculator](https://mlco2.github.io/impact#compute) presented in [Lacoste et al. (2019)](https://arxiv.org/abs/1910.09700).
- **Hardware Type:** More information needed
- **Hours used:** More information needed
- **Cloud Provider:** More information needed
- **Compute Region:** More information needed
- **Carbon Emitted:** More information needed
# Technical Specifications [optional]
## Model Architecture and Objective
The CLIP model devlopers note in their [model card](https://huggingface.co/openai/clip-vit-large-patch14) :
> The base model uses a ViT-L/14 Transformer architecture as an image encoder and uses a masked self-attention Transformer as a text encoder. These encoders are trained to maximize the similarity of (image, text) pairs via a contrastive loss.
## Compute Infrastructure
More information needed
### Hardware
More information needed
### Software
More information needed.
# Citation
**BibTeX:**
More information needed
**APA:**
More information needed
# Glossary [optional]
More information needed
# More Information [optional]
More information needed
# Model Card Authors [optional]
CompVis in collaboration with Ezi Ozoani and the Hugging Face team
# Model Card Contact
More information needed
# How to Get Started with the Model
Use the code below to get started with the model.
<details>
<summary> Click to expand </summary>
```python
from transformers import AutoProcessor, SafetyChecker
processor = AutoProcessor.from_pretrained("CompVis/stable-diffusion-safety-checker")
safety_checker = SafetyChecker.from_pretrained("CompVis/stable-diffusion-safety-checker")
```
</details>
|
MaziyarPanahi/Llama-3.2-3B-Instruct-GGUF | MaziyarPanahi | "2024-09-25T19:18:32Z" | 1,332,772 | 6 | null | [
"gguf",
"mistral",
"quantized",
"2-bit",
"3-bit",
"4-bit",
"5-bit",
"6-bit",
"8-bit",
"GGUF",
"text-generation",
"base_model:meta-llama/Llama-3.2-3B-Instruct",
"base_model:quantized:meta-llama/Llama-3.2-3B-Instruct",
"region:us",
"imatrix",
"conversational"
] | text-generation | "2024-09-25T19:00:39Z" | ---
tags:
- quantized
- 2-bit
- 3-bit
- 4-bit
- 5-bit
- 6-bit
- 8-bit
- GGUF
- text-generation
- text-generation
model_name: Llama-3.2-3B-Instruct-GGUF
base_model: meta-llama/Llama-3.2-3B-Instruct
inference: false
model_creator: meta-llama
pipeline_tag: text-generation
quantized_by: MaziyarPanahi
---
# [MaziyarPanahi/Llama-3.2-3B-Instruct-GGUF](https://huggingface.co/MaziyarPanahi/Llama-3.2-3B-Instruct-GGUF)
- Model creator: [meta-llama](https://huggingface.co/meta-llama)
- Original model: [meta-llama/Llama-3.2-3B-Instruct](https://huggingface.co/meta-llama/Llama-3.2-3B-Instruct)
## Description
[MaziyarPanahi/Llama-3.2-3B-Instruct-GGUF](https://huggingface.co/MaziyarPanahi/Llama-3.2-3B-Instruct-GGUF) contains GGUF format model files for [meta-llama/Llama-3.2-3B-Instruct](https://huggingface.co/meta-llama/Llama-3.2-3B-Instruct).
### About GGUF
GGUF is a new format introduced by the llama.cpp team on August 21st 2023. It is a replacement for GGML, which is no longer supported by llama.cpp.
Here is an incomplete list of clients and libraries that are known to support GGUF:
* [llama.cpp](https://github.com/ggerganov/llama.cpp). The source project for GGUF. Offers a CLI and a server option.
* [llama-cpp-python](https://github.com/abetlen/llama-cpp-python), a Python library with GPU accel, LangChain support, and OpenAI-compatible API server.
* [LM Studio](https://lmstudio.ai/), an easy-to-use and powerful local GUI for Windows and macOS (Silicon), with GPU acceleration. Linux available, in beta as of 27/11/2023.
* [text-generation-webui](https://github.com/oobabooga/text-generation-webui), the most widely used web UI, with many features and powerful extensions. Supports GPU acceleration.
* [KoboldCpp](https://github.com/LostRuins/koboldcpp), a fully featured web UI, with GPU accel across all platforms and GPU architectures. Especially good for story telling.
* [GPT4All](https://gpt4all.io/index.html), a free and open source local running GUI, supporting Windows, Linux and macOS with full GPU accel.
* [LoLLMS Web UI](https://github.com/ParisNeo/lollms-webui), a great web UI with many interesting and unique features, including a full model library for easy model selection.
* [Faraday.dev](https://faraday.dev/), an attractive and easy to use character-based chat GUI for Windows and macOS (both Silicon and Intel), with GPU acceleration.
* [candle](https://github.com/huggingface/candle), a Rust ML framework with a focus on performance, including GPU support, and ease of use.
* [ctransformers](https://github.com/marella/ctransformers), a Python library with GPU accel, LangChain support, and OpenAI-compatible AI server. Note, as of time of writing (November 27th 2023), ctransformers has not been updated in a long time and does not support many recent models.
## Special thanks
🙏 Special thanks to [Georgi Gerganov](https://github.com/ggerganov) and the whole team working on [llama.cpp](https://github.com/ggerganov/llama.cpp/) for making all of this possible. |
facebook/m2m100_418M | facebook | "2024-02-29T09:08:42Z" | 1,331,587 | 254 | transformers | [
"transformers",
"pytorch",
"rust",
"m2m_100",
"text2text-generation",
"multilingual",
"af",
"am",
"ar",
"ast",
"az",
"ba",
"be",
"bg",
"bn",
"br",
"bs",
"ca",
"ceb",
"cs",
"cy",
"da",
"de",
"el",
"en",
"es",
"et",
"fa",
"ff",
"fi",
"fr",
"fy",
"ga",
"gd",
"gl",
"gu",
"ha",
"he",
"hi",
"hr",
"ht",
"hu",
"hy",
"id",
"ig",
"ilo",
"is",
"it",
"ja",
"jv",
"ka",
"kk",
"km",
"kn",
"ko",
"lb",
"lg",
"ln",
"lo",
"lt",
"lv",
"mg",
"mk",
"ml",
"mn",
"mr",
"ms",
"my",
"ne",
"nl",
"no",
"ns",
"oc",
"or",
"pa",
"pl",
"ps",
"pt",
"ro",
"ru",
"sd",
"si",
"sk",
"sl",
"so",
"sq",
"sr",
"ss",
"su",
"sv",
"sw",
"ta",
"th",
"tl",
"tn",
"tr",
"uk",
"ur",
"uz",
"vi",
"wo",
"xh",
"yi",
"yo",
"zh",
"zu",
"arxiv:2010.11125",
"license:mit",
"autotrain_compatible",
"endpoints_compatible",
"region:us"
] | text2text-generation | "2022-03-02T23:29:05Z" | ---
language:
- multilingual
- af
- am
- ar
- ast
- az
- ba
- be
- bg
- bn
- br
- bs
- ca
- ceb
- cs
- cy
- da
- de
- el
- en
- es
- et
- fa
- ff
- fi
- fr
- fy
- ga
- gd
- gl
- gu
- ha
- he
- hi
- hr
- ht
- hu
- hy
- id
- ig
- ilo
- is
- it
- ja
- jv
- ka
- kk
- km
- kn
- ko
- lb
- lg
- ln
- lo
- lt
- lv
- mg
- mk
- ml
- mn
- mr
- ms
- my
- ne
- nl
- no
- ns
- oc
- or
- pa
- pl
- ps
- pt
- ro
- ru
- sd
- si
- sk
- sl
- so
- sq
- sr
- ss
- su
- sv
- sw
- ta
- th
- tl
- tn
- tr
- uk
- ur
- uz
- vi
- wo
- xh
- yi
- yo
- zh
- zu
license: mit
---
# M2M100 418M
M2M100 is a multilingual encoder-decoder (seq-to-seq) model trained for Many-to-Many multilingual translation.
It was introduced in this [paper](https://arxiv.org/abs/2010.11125) and first released in [this](https://github.com/pytorch/fairseq/tree/master/examples/m2m_100) repository.
The model that can directly translate between the 9,900 directions of 100 languages.
To translate into a target language, the target language id is forced as the first generated token.
To force the target language id as the first generated token, pass the `forced_bos_token_id` parameter to the `generate` method.
*Note: `M2M100Tokenizer` depends on `sentencepiece`, so make sure to install it before running the example.*
To install `sentencepiece` run `pip install sentencepiece`
```python
from transformers import M2M100ForConditionalGeneration, M2M100Tokenizer
hi_text = "जीवन एक चॉकलेट बॉक्स की तरह है।"
chinese_text = "生活就像一盒巧克力。"
model = M2M100ForConditionalGeneration.from_pretrained("facebook/m2m100_418M")
tokenizer = M2M100Tokenizer.from_pretrained("facebook/m2m100_418M")
# translate Hindi to French
tokenizer.src_lang = "hi"
encoded_hi = tokenizer(hi_text, return_tensors="pt")
generated_tokens = model.generate(**encoded_hi, forced_bos_token_id=tokenizer.get_lang_id("fr"))
tokenizer.batch_decode(generated_tokens, skip_special_tokens=True)
# => "La vie est comme une boîte de chocolat."
# translate Chinese to English
tokenizer.src_lang = "zh"
encoded_zh = tokenizer(chinese_text, return_tensors="pt")
generated_tokens = model.generate(**encoded_zh, forced_bos_token_id=tokenizer.get_lang_id("en"))
tokenizer.batch_decode(generated_tokens, skip_special_tokens=True)
# => "Life is like a box of chocolate."
```
See the [model hub](https://huggingface.co/models?filter=m2m_100) to look for more fine-tuned versions.
## Languages covered
Afrikaans (af), Amharic (am), Arabic (ar), Asturian (ast), Azerbaijani (az), Bashkir (ba), Belarusian (be), Bulgarian (bg), Bengali (bn), Breton (br), Bosnian (bs), Catalan; Valencian (ca), Cebuano (ceb), Czech (cs), Welsh (cy), Danish (da), German (de), Greeek (el), English (en), Spanish (es), Estonian (et), Persian (fa), Fulah (ff), Finnish (fi), French (fr), Western Frisian (fy), Irish (ga), Gaelic; Scottish Gaelic (gd), Galician (gl), Gujarati (gu), Hausa (ha), Hebrew (he), Hindi (hi), Croatian (hr), Haitian; Haitian Creole (ht), Hungarian (hu), Armenian (hy), Indonesian (id), Igbo (ig), Iloko (ilo), Icelandic (is), Italian (it), Japanese (ja), Javanese (jv), Georgian (ka), Kazakh (kk), Central Khmer (km), Kannada (kn), Korean (ko), Luxembourgish; Letzeburgesch (lb), Ganda (lg), Lingala (ln), Lao (lo), Lithuanian (lt), Latvian (lv), Malagasy (mg), Macedonian (mk), Malayalam (ml), Mongolian (mn), Marathi (mr), Malay (ms), Burmese (my), Nepali (ne), Dutch; Flemish (nl), Norwegian (no), Northern Sotho (ns), Occitan (post 1500) (oc), Oriya (or), Panjabi; Punjabi (pa), Polish (pl), Pushto; Pashto (ps), Portuguese (pt), Romanian; Moldavian; Moldovan (ro), Russian (ru), Sindhi (sd), Sinhala; Sinhalese (si), Slovak (sk), Slovenian (sl), Somali (so), Albanian (sq), Serbian (sr), Swati (ss), Sundanese (su), Swedish (sv), Swahili (sw), Tamil (ta), Thai (th), Tagalog (tl), Tswana (tn), Turkish (tr), Ukrainian (uk), Urdu (ur), Uzbek (uz), Vietnamese (vi), Wolof (wo), Xhosa (xh), Yiddish (yi), Yoruba (yo), Chinese (zh), Zulu (zu)
## BibTeX entry and citation info
```
@misc{fan2020englishcentric,
title={Beyond English-Centric Multilingual Machine Translation},
author={Angela Fan and Shruti Bhosale and Holger Schwenk and Zhiyi Ma and Ahmed El-Kishky and Siddharth Goyal and Mandeep Baines and Onur Celebi and Guillaume Wenzek and Vishrav Chaudhary and Naman Goyal and Tom Birch and Vitaliy Liptchinsky and Sergey Edunov and Edouard Grave and Michael Auli and Armand Joulin},
year={2020},
eprint={2010.11125},
archivePrefix={arXiv},
primaryClass={cs.CL}
}
``` |
MaziyarPanahi/Mistral-7B-Instruct-v0.3-GGUF | MaziyarPanahi | "2024-05-22T20:26:06Z" | 1,320,291 | 62 | transformers | [
"transformers",
"gguf",
"mistral",
"quantized",
"2-bit",
"3-bit",
"4-bit",
"5-bit",
"6-bit",
"8-bit",
"GGUF",
"safetensors",
"text-generation",
"conversational",
"license:apache-2.0",
"autotrain_compatible",
"endpoints_compatible",
"text-generation-inference",
"region:us",
"base_model:mistralai/Mistral-7B-Instruct-v0.3",
"base_model:quantized:mistralai/Mistral-7B-Instruct-v0.3",
"imatrix"
] | text-generation | "2024-05-22T17:27:45Z" | ---
tags:
- quantized
- 2-bit
- 3-bit
- 4-bit
- 5-bit
- 6-bit
- 8-bit
- GGUF
- transformers
- safetensors
- mistral
- text-generation
- conversational
- license:apache-2.0
- autotrain_compatible
- endpoints_compatible
- text-generation-inference
- region:us
- text-generation
model_name: Mistral-7B-Instruct-v0.3-GGUF
base_model: mistralai/Mistral-7B-Instruct-v0.3
inference: false
model_creator: mistralai
pipeline_tag: text-generation
quantized_by: MaziyarPanahi
---
# [MaziyarPanahi/Mistral-7B-Instruct-v0.3-GGUF](https://huggingface.co/MaziyarPanahi/Mistral-7B-Instruct-v0.3-GGUF)
- Model creator: [mistralai](https://huggingface.co/mistralai)
- Original model: [mistralai/Mistral-7B-Instruct-v0.3](https://huggingface.co/mistralai/Mistral-7B-Instruct-v0.3)
## Description
[MaziyarPanahi/Mistral-7B-Instruct-v0.3-GGUF](https://huggingface.co/MaziyarPanahi/Mistral-7B-Instruct-v0.3-GGUF) contains GGUF format model files for [mistralai/Mistral-7B-Instruct-v0.3](https://huggingface.co/mistralai/Mistral-7B-Instruct-v0.3).
### About GGUF
GGUF is a new format introduced by the llama.cpp team on August 21st 2023. It is a replacement for GGML, which is no longer supported by llama.cpp.
Here is an incomplete list of clients and libraries that are known to support GGUF:
* [llama.cpp](https://github.com/ggerganov/llama.cpp). The source project for GGUF. Offers a CLI and a server option.
* [llama-cpp-python](https://github.com/abetlen/llama-cpp-python), a Python library with GPU accel, LangChain support, and OpenAI-compatible API server.
* [LM Studio](https://lmstudio.ai/), an easy-to-use and powerful local GUI for Windows and macOS (Silicon), with GPU acceleration. Linux available, in beta as of 27/11/2023.
* [text-generation-webui](https://github.com/oobabooga/text-generation-webui), the most widely used web UI, with many features and powerful extensions. Supports GPU acceleration.
* [KoboldCpp](https://github.com/LostRuins/koboldcpp), a fully featured web UI, with GPU accel across all platforms and GPU architectures. Especially good for story telling.
* [GPT4All](https://gpt4all.io/index.html), a free and open source local running GUI, supporting Windows, Linux and macOS with full GPU accel.
* [LoLLMS Web UI](https://github.com/ParisNeo/lollms-webui), a great web UI with many interesting and unique features, including a full model library for easy model selection.
* [Faraday.dev](https://faraday.dev/), an attractive and easy to use character-based chat GUI for Windows and macOS (both Silicon and Intel), with GPU acceleration.
* [candle](https://github.com/huggingface/candle), a Rust ML framework with a focus on performance, including GPU support, and ease of use.
* [ctransformers](https://github.com/marella/ctransformers), a Python library with GPU accel, LangChain support, and OpenAI-compatible AI server. Note, as of time of writing (November 27th 2023), ctransformers has not been updated in a long time and does not support many recent models.
## Special thanks
🙏 Special thanks to [Georgi Gerganov](https://github.com/ggerganov) and the whole team working on [llama.cpp](https://github.com/ggerganov/llama.cpp/) for making all of this possible. |
black-forest-labs/FLUX.1-dev | black-forest-labs | "2024-08-16T14:38:19Z" | 1,316,333 | 6,276 | diffusers | [
"diffusers",
"safetensors",
"text-to-image",
"image-generation",
"flux",
"en",
"license:other",
"endpoints_compatible",
"diffusers:FluxPipeline",
"region:us"
] | text-to-image | "2024-07-31T21:13:44Z" | ---
language:
- en
license: other
license_name: flux-1-dev-non-commercial-license
license_link: LICENSE.md
extra_gated_prompt: By clicking "Agree", you agree to the [FluxDev Non-Commercial License Agreement](https://huggingface.co/black-forest-labs/FLUX.1-dev/blob/main/LICENSE.md)
and acknowledge the [Acceptable Use Policy](https://huggingface.co/black-forest-labs/FLUX.1-dev/blob/main/POLICY.md).
tags:
- text-to-image
- image-generation
- flux
---
![FLUX.1 [dev] Grid](./dev_grid.jpg)
`FLUX.1 [dev]` is a 12 billion parameter rectified flow transformer capable of generating images from text descriptions.
For more information, please read our [blog post](https://blackforestlabs.ai/announcing-black-forest-labs/).
# Key Features
1. Cutting-edge output quality, second only to our state-of-the-art model `FLUX.1 [pro]`.
2. Competitive prompt following, matching the performance of closed source alternatives .
3. Trained using guidance distillation, making `FLUX.1 [dev]` more efficient.
4. Open weights to drive new scientific research, and empower artists to develop innovative workflows.
5. Generated outputs can be used for personal, scientific, and commercial purposes as described in the [`FLUX.1 [dev]` Non-Commercial License](https://huggingface.co/black-forest-labs/FLUX.1-dev/blob/main/LICENSE.md).
# Usage
We provide a reference implementation of `FLUX.1 [dev]`, as well as sampling code, in a dedicated [github repository](https://github.com/black-forest-labs/flux).
Developers and creatives looking to build on top of `FLUX.1 [dev]` are encouraged to use this as a starting point.
## API Endpoints
The FLUX.1 models are also available via API from the following sources
- [bfl.ml](https://docs.bfl.ml/) (currently `FLUX.1 [pro]`)
- [replicate.com](https://replicate.com/collections/flux)
- [fal.ai](https://fal.ai/models/fal-ai/flux/dev)
- [mystic.ai](https://www.mystic.ai/black-forest-labs/flux1-dev)
## ComfyUI
`FLUX.1 [dev]` is also available in [Comfy UI](https://github.com/comfyanonymous/ComfyUI) for local inference with a node-based workflow.
## Diffusers
To use `FLUX.1 [dev]` with the 🧨 diffusers python library, first install or upgrade diffusers
```shell
pip install -U diffusers
```
Then you can use `FluxPipeline` to run the model
```python
import torch
from diffusers import FluxPipeline
pipe = FluxPipeline.from_pretrained("black-forest-labs/FLUX.1-dev", torch_dtype=torch.bfloat16)
pipe.enable_model_cpu_offload() #save some VRAM by offloading the model to CPU. Remove this if you have enough GPU power
prompt = "A cat holding a sign that says hello world"
image = pipe(
prompt,
height=1024,
width=1024,
guidance_scale=3.5,
num_inference_steps=50,
max_sequence_length=512,
generator=torch.Generator("cpu").manual_seed(0)
).images[0]
image.save("flux-dev.png")
```
To learn more check out the [diffusers](https://huggingface.co/docs/diffusers/main/en/api/pipelines/flux) documentation
---
# Limitations
- This model is not intended or able to provide factual information.
- As a statistical model this checkpoint might amplify existing societal biases.
- The model may fail to generate output that matches the prompts.
- Prompt following is heavily influenced by the prompting-style.
# Out-of-Scope Use
The model and its derivatives may not be used
- In any way that violates any applicable national, federal, state, local or international law or regulation.
- For the purpose of exploiting, harming or attempting to exploit or harm minors in any way; including but not limited to the solicitation, creation, acquisition, or dissemination of child exploitative content.
- To generate or disseminate verifiably false information and/or content with the purpose of harming others.
- To generate or disseminate personal identifiable information that can be used to harm an individual.
- To harass, abuse, threaten, stalk, or bully individuals or groups of individuals.
- To create non-consensual nudity or illegal pornographic content.
- For fully automated decision making that adversely impacts an individual's legal rights or otherwise creates or modifies a binding, enforceable obligation.
- Generating or facilitating large-scale disinformation campaigns.
# License
This model falls under the [`FLUX.1 [dev]` Non-Commercial License](https://huggingface.co/black-forest-labs/FLUX.1-dev/blob/main/LICENSE.md). |
MaziyarPanahi/Meta-Llama-3-8B-Instruct-GGUF | MaziyarPanahi | "2024-04-23T12:55:06Z" | 1,315,767 | 77 | transformers | [
"transformers",
"gguf",
"mistral",
"facebook",
"meta",
"pytorch",
"llama",
"llama-3",
"quantized",
"2-bit",
"3-bit",
"4-bit",
"5-bit",
"6-bit",
"8-bit",
"16-bit",
"GGUF",
"text-generation",
"en",
"base_model:meta-llama/Meta-Llama-3-8B-Instruct",
"base_model:quantized:meta-llama/Meta-Llama-3-8B-Instruct",
"region:us",
"conversational"
] | text-generation | "2024-04-18T16:43:25Z" | ---
language:
- en
pipeline_tag: text-generation
tags:
- facebook
- meta
- pytorch
- llama
- llama-3
- quantized
- 2-bit
- 3-bit
- 4-bit
- 5-bit
- 6-bit
- 8-bit
- 16-bit
- GGUF
base_model: meta-llama/Meta-Llama-3-8B-Instruct
inference: false
model_creator: MaziyarPanahi
model_name: Meta-Llama-3-8B-Instruct-GGUF
quantized_by: MaziyarPanahi
license_name: llama3
---
# [MaziyarPanahi/Meta-Llama-3-8B-Instruct-GGUF](https://huggingface.co/MaziyarPanahi/Meta-Llama-3-8B-Instruct-GGUF)
- Model creator: [meta-llama](https://huggingface.co/meta-llama)
- Original model: [meta-llama/Meta-Llama-3-8B-Instruct](https://huggingface.co/meta-llama/Meta-Llama-3-8B-Instruct)
The GGUF and quantized models here are based on [meta-llama/Meta-Llama-3-8B-Instruct](https://huggingface.co/meta-llama/Meta-Llama-3-8B-Instruct) model
## How to download
You can download only the quants you need instead of cloning the entire repository as follows:
```
huggingface-cli download MaziyarPanahi/Meta-Llama-3-8B-Instruct-GGUF --local-dir . --include '*Q2_K*gguf'
```
## Load GGUF models
You `MUST` follow the prompt template provided by Llama-3:
```sh
./llama.cpp/main -m Meta-Llama-3-8B-Instruct.Q2_K.gguf -r '<|eot_id|>' --in-prefix "\n<|start_header_id|>user<|end_header_id|>\n\n" --in-suffix "<|eot_id|><|start_header_id|>assistant<|end_header_id|>\n\n" -p "<|begin_of_text|><|start_header_id|>system<|end_header_id|>\n\nYou are a helpful, smart, kind, and efficient AI assistant. You always fulfill the user's requests to the best of your ability.<|eot_id|>\n<|start_header_id|>user<|end_header_id|>\n\nHi! How are you?<|eot_id|>\n<|start_header_id|>assistant<|end_header_id|>\n\n" -n 1024
```
Original README
---
## Model Details
Meta developed and released the Meta Llama 3 family of large language models (LLMs), a collection of pretrained and instruction tuned generative text models in 8 and 70B sizes. The Llama 3 instruction tuned models are optimized for dialogue use cases and outperform many of the available open source chat models on common industry benchmarks. Further, in developing these models, we took great care to optimize helpfulness and safety.
**Model developers** Meta
**Variations** Llama 3 comes in two sizes — 8B and 70B parameters — in pre-trained and instruction tuned variants.
**Input** Models input text only.
**Output** Models generate text and code only.
**Model Architecture** Llama 3 is an auto-regressive language model that uses an optimized transformer architecture. The tuned versions use supervised fine-tuning (SFT) and reinforcement learning with human feedback (RLHF) to align with human preferences for helpfulness and safety.
<table>
<tr>
<td>
</td>
<td><strong>Training Data</strong>
</td>
<td><strong>Params</strong>
</td>
<td><strong>Context length</strong>
</td>
<td><strong>GQA</strong>
</td>
<td><strong>Token count</strong>
</td>
<td><strong>Knowledge cutoff</strong>
</td>
</tr>
<tr>
<td rowspan="2" >Llama 3
</td>
<td rowspan="2" >A new mix of publicly available online data.
</td>
<td>8B
</td>
<td>8k
</td>
<td>Yes
</td>
<td rowspan="2" >15T+
</td>
<td>March, 2023
</td>
</tr>
<tr>
<td>70B
</td>
<td>8k
</td>
<td>Yes
</td>
<td>December, 2023
</td>
</tr>
</table>
**Llama 3 family of models**. Token counts refer to pretraining data only. Both the 8 and 70B versions use Grouped-Query Attention (GQA) for improved inference scalability.
**Model Release Date** April 18, 2024.
**Status** This is a static model trained on an offline dataset. Future versions of the tuned models will be released as we improve model safety with community feedback.
**License** A custom commercial license is available at: [https://llama.meta.com/llama3/license](https://llama.meta.com/llama3/license)
Where to send questions or comments about the model Instructions on how to provide feedback or comments on the model can be found in the model [README](https://github.com/meta-llama/llama3). For more technical information about generation parameters and recipes for how to use Llama 3 in applications, please go [here](https://github.com/meta-llama/llama-recipes).
## Intended Use
**Intended Use Cases** Llama 3 is intended for commercial and research use in English. Instruction tuned models are intended for assistant-like chat, whereas pretrained models can be adapted for a variety of natural language generation tasks.
**Out-of-scope** Use in any manner that violates applicable laws or regulations (including trade compliance laws). Use in any other way that is prohibited by the Acceptable Use Policy and Llama 3 Community License. Use in languages other than English**.
**Note: Developers may fine-tune Llama 3 models for languages beyond English provided they comply with the Llama 3 Community License and the Acceptable Use Policy.
## How to use
This repository contains two versions of Meta-Llama-3-70B-Instruct, for use with transformers and with the original `llama3` codebase.
### Use with transformers
See the snippet below for usage with Transformers:
```python
import transformers
import torch
model_id = "meta-llama/Meta-Llama-3-70B-Instruct"
pipeline = transformers.pipeline(
"text-generation",
model=model_id,
model_kwargs={"torch_dtype": torch.bfloat16},
device="cuda",
)
messages = [
{"role": "system", "content": "You are a pirate chatbot who always responds in pirate speak!"},
{"role": "user", "content": "Who are you?"},
]
prompt = pipeline.tokenizer.apply_chat_template(
messages,
tokenize=False,
add_generation_prompt=True
)
terminators = [
tokenizer.eos_token_id,
tokenizer.convert_tokens_to_ids("<|eot_id|>")
]
outputs = pipeline(
prompt,
max_new_tokens=256,
eos_token_id=terminators,
do_sample=True,
temperature=0.6,
top_p=0.9,
)
print(outputs[0]["generated_text"][len(prompt):])
```
### Use with `llama3`
Please, follow the instructions in the [repository](https://github.com/meta-llama/llama3).
To download Original checkpoints, see the example command below leveraging `huggingface-cli`:
```
huggingface-cli download meta-llama/Meta-Llama-3-70B-Instruct --include "original/*" --local-dir Meta-Llama-3-70B-Instruct
```
For Hugging Face support, we recommend using transformers or TGI, but a similar command works.
## Hardware and Software
**Training Factors** We used custom training libraries, Meta's Research SuperCluster, and production clusters for pretraining. Fine-tuning, annotation, and evaluation were also performed on third-party cloud compute.
**Carbon Footprint Pretraining utilized a cumulative** 7.7M GPU hours of computation on hardware of type H100-80GB (TDP of 700W). Estimated total emissions were 2290 tCO2eq, 100% of which were offset by Meta’s sustainability program.
<table>
<tr>
<td>
</td>
<td><strong>Time (GPU hours)</strong>
</td>
<td><strong>Power Consumption (W)</strong>
</td>
<td><strong>Carbon Emitted(tCO2eq)</strong>
</td>
</tr>
<tr>
<td>Llama 3 8B
</td>
<td>1.3M
</td>
<td>700
</td>
<td>390
</td>
</tr>
<tr>
<td>Llama 3 70B
</td>
<td>6.4M
</td>
<td>700
</td>
<td>1900
</td>
</tr>
<tr>
<td>Total
</td>
<td>7.7M
</td>
<td>
</td>
<td>2290
</td>
</tr>
</table>
**CO2 emissions during pre-training**. Time: total GPU time required for training each model. Power Consumption: peak power capacity per GPU device for the GPUs used adjusted for power usage efficiency. 100% of the emissions are directly offset by Meta's sustainability program, and because we are openly releasing these models, the pretraining costs do not need to be incurred by others.
## Training Data
**Overview** Llama 3 was pretrained on over 15 trillion tokens of data from publicly available sources. The fine-tuning data includes publicly available instruction datasets, as well as over 10M human-annotated examples. Neither the pretraining nor the fine-tuning datasets include Meta user data.
**Data Freshness** The pretraining data has a cutoff of March 2023 for the 7B and December 2023 for the 70B models respectively.
## Benchmarks
In this section, we report the results for Llama 3 models on standard automatic benchmarks. For all the evaluations, we use our internal evaluations library. For details on the methodology see [here](https://github.com/meta-llama/llama3/blob/main/eval_methodology.md).
### Base pretrained models
<table>
<tr>
<td><strong>Category</strong>
</td>
<td><strong>Benchmark</strong>
</td>
<td><strong>Llama 3 8B</strong>
</td>
<td><strong>Llama2 7B</strong>
</td>
<td><strong>Llama2 13B</strong>
</td>
<td><strong>Llama 3 70B</strong>
</td>
<td><strong>Llama2 70B</strong>
</td>
</tr>
<tr>
<td rowspan="6" >General
</td>
<td>MMLU (5-shot)
</td>
<td>66.6
</td>
<td>45.7
</td>
<td>53.8
</td>
<td>79.5
</td>
<td>69.7
</td>
</tr>
<tr>
<td>AGIEval English (3-5 shot)
</td>
<td>45.9
</td>
<td>28.8
</td>
<td>38.7
</td>
<td>63.0
</td>
<td>54.8
</td>
</tr>
<tr>
<td>CommonSenseQA (7-shot)
</td>
<td>72.6
</td>
<td>57.6
</td>
<td>67.6
</td>
<td>83.8
</td>
<td>78.7
</td>
</tr>
<tr>
<td>Winogrande (5-shot)
</td>
<td>76.1
</td>
<td>73.3
</td>
<td>75.4
</td>
<td>83.1
</td>
<td>81.8
</td>
</tr>
<tr>
<td>BIG-Bench Hard (3-shot, CoT)
</td>
<td>61.1
</td>
<td>38.1
</td>
<td>47.0
</td>
<td>81.3
</td>
<td>65.7
</td>
</tr>
<tr>
<td>ARC-Challenge (25-shot)
</td>
<td>78.6
</td>
<td>53.7
</td>
<td>67.6
</td>
<td>93.0
</td>
<td>85.3
</td>
</tr>
<tr>
<td>Knowledge reasoning
</td>
<td>TriviaQA-Wiki (5-shot)
</td>
<td>78.5
</td>
<td>72.1
</td>
<td>79.6
</td>
<td>89.7
</td>
<td>87.5
</td>
</tr>
<tr>
<td rowspan="4" >Reading comprehension
</td>
<td>SQuAD (1-shot)
</td>
<td>76.4
</td>
<td>72.2
</td>
<td>72.1
</td>
<td>85.6
</td>
<td>82.6
</td>
</tr>
<tr>
<td>QuAC (1-shot, F1)
</td>
<td>44.4
</td>
<td>39.6
</td>
<td>44.9
</td>
<td>51.1
</td>
<td>49.4
</td>
</tr>
<tr>
<td>BoolQ (0-shot)
</td>
<td>75.7
</td>
<td>65.5
</td>
<td>66.9
</td>
<td>79.0
</td>
<td>73.1
</td>
</tr>
<tr>
<td>DROP (3-shot, F1)
</td>
<td>58.4
</td>
<td>37.9
</td>
<td>49.8
</td>
<td>79.7
</td>
<td>70.2
</td>
</tr>
</table>
### Instruction tuned models
<table>
<tr>
<td><strong>Benchmark</strong>
</td>
<td><strong>Llama 3 8B</strong>
</td>
<td><strong>Llama 2 7B</strong>
</td>
<td><strong>Llama 2 13B</strong>
</td>
<td><strong>Llama 3 70B</strong>
</td>
<td><strong>Llama 2 70B</strong>
</td>
</tr>
<tr>
<td>MMLU (5-shot)
</td>
<td>68.4
</td>
<td>34.1
</td>
<td>47.8
</td>
<td>82.0
</td>
<td>52.9
</td>
</tr>
<tr>
<td>GPQA (0-shot)
</td>
<td>34.2
</td>
<td>21.7
</td>
<td>22.3
</td>
<td>39.5
</td>
<td>21.0
</td>
</tr>
<tr>
<td>HumanEval (0-shot)
</td>
<td>62.2
</td>
<td>7.9
</td>
<td>14.0
</td>
<td>81.7
</td>
<td>25.6
</td>
</tr>
<tr>
<td>GSM-8K (8-shot, CoT)
</td>
<td>79.6
</td>
<td>25.7
</td>
<td>77.4
</td>
<td>93.0
</td>
<td>57.5
</td>
</tr>
<tr>
<td>MATH (4-shot, CoT)
</td>
<td>30.0
</td>
<td>3.8
</td>
<td>6.7
</td>
<td>50.4
</td>
<td>11.6
</td>
</tr>
</table>
### Responsibility & Safety
We believe that an open approach to AI leads to better, safer products, faster innovation, and a bigger overall market. We are committed to Responsible AI development and took a series of steps to limit misuse and harm and support the open source community.
Foundation models are widely capable technologies that are built to be used for a diverse range of applications. They are not designed to meet every developer preference on safety levels for all use cases, out-of-the-box, as those by their nature will differ across different applications.
Rather, responsible LLM-application deployment is achieved by implementing a series of safety best practices throughout the development of such applications, from the model pre-training, fine-tuning and the deployment of systems composed of safeguards to tailor the safety needs specifically to the use case and audience.
As part of the Llama 3 release, we updated our [Responsible Use Guide](https://llama.meta.com/responsible-use-guide/) to outline the steps and best practices for developers to implement model and system level safety for their application. We also provide a set of resources including [Meta Llama Guard 2](https://llama.meta.com/purple-llama/) and [Code Shield](https://llama.meta.com/purple-llama/) safeguards. These tools have proven to drastically reduce residual risks of LLM Systems, while maintaining a high level of helpfulness. We encourage developers to tune and deploy these safeguards according to their needs and we provide a [reference implementation](https://github.com/meta-llama/llama-recipes/tree/main/recipes/responsible_ai) to get you started.
#### Llama 3-Instruct
As outlined in the Responsible Use Guide, some trade-off between model helpfulness and model alignment is likely unavoidable. Developers should exercise discretion about how to weigh the benefits of alignment and helpfulness for their specific use case and audience. Developers should be mindful of residual risks when using Llama models and leverage additional safety tools as needed to reach the right safety bar for their use case.
<span style="text-decoration:underline;">Safety</span>
For our instruction tuned model, we conducted extensive red teaming exercises, performed adversarial evaluations and implemented safety mitigations techniques to lower residual risks. As with any Large Language Model, residual risks will likely remain and we recommend that developers assess these risks in the context of their use case. In parallel, we are working with the community to make AI safety benchmark standards transparent, rigorous and interpretable.
<span style="text-decoration:underline;">Refusals</span>
In addition to residual risks, we put a great emphasis on model refusals to benign prompts. Over-refusing not only can impact the user experience but could even be harmful in certain contexts as well. We’ve heard the feedback from the developer community and improved our fine tuning to ensure that Llama 3 is significantly less likely to falsely refuse to answer prompts than Llama 2.
We built internal benchmarks and developed mitigations to limit false refusals making Llama 3 our most helpful model to date.
#### Responsible release
In addition to responsible use considerations outlined above, we followed a rigorous process that requires us to take extra measures against misuse and critical risks before we make our release decision.
Misuse
If you access or use Llama 3, you agree to the Acceptable Use Policy. The most recent copy of this policy can be found at [https://llama.meta.com/llama3/use-policy/](https://llama.meta.com/llama3/use-policy/).
#### Critical risks
<span style="text-decoration:underline;">CBRNE</span> (Chemical, Biological, Radiological, Nuclear, and high yield Explosives)
We have conducted a two fold assessment of the safety of the model in this area:
* Iterative testing during model training to assess the safety of responses related to CBRNE threats and other adversarial risks.
* Involving external CBRNE experts to conduct an uplift test assessing the ability of the model to accurately provide expert knowledge and reduce barriers to potential CBRNE misuse, by reference to what can be achieved using web search (without the model).
### <span style="text-decoration:underline;">Cyber Security </span>
We have evaluated Llama 3 with CyberSecEval, Meta’s cybersecurity safety eval suite, measuring Llama 3’s propensity to suggest insecure code when used as a coding assistant, and Llama 3’s propensity to comply with requests to help carry out cyber attacks, where attacks are defined by the industry standard MITRE ATT&CK cyber attack ontology. On our insecure coding and cyber attacker helpfulness tests, Llama 3 behaved in the same range or safer than models of [equivalent coding capability](https://huggingface.co/spaces/facebook/CyberSecEval).
### <span style="text-decoration:underline;">Child Safety</span>
Child Safety risk assessments were conducted using a team of experts, to assess the model’s capability to produce outputs that could result in Child Safety risks and inform on any necessary and appropriate risk mitigations via fine tuning. We leveraged those expert red teaming sessions to expand the coverage of our evaluation benchmarks through Llama 3 model development. For Llama 3, we conducted new in-depth sessions using objective based methodologies to assess the model risks along multiple attack vectors. We also partnered with content specialists to perform red teaming exercises assessing potentially violating content while taking account of market specific nuances or experiences.
### Community
Generative AI safety requires expertise and tooling, and we believe in the strength of the open community to accelerate its progress. We are active members of open consortiums, including the AI Alliance, Partnership in AI and MLCommons, actively contributing to safety standardization and transparency. We encourage the community to adopt taxonomies like the MLCommons Proof of Concept evaluation to facilitate collaboration and transparency on safety and content evaluations. Our Purple Llama tools are open sourced for the community to use and widely distributed across ecosystem partners including cloud service providers. We encourage community contributions to our [Github repository](https://github.com/meta-llama/PurpleLlama).
Finally, we put in place a set of resources including an [output reporting mechanism](https://developers.facebook.com/llama_output_feedback) and [bug bounty program](https://www.facebook.com/whitehat) to continuously improve the Llama technology with the help of the community.
## Ethical Considerations and Limitations
The core values of Llama 3 are openness, inclusivity and helpfulness. It is meant to serve everyone, and to work for a wide range of use cases. It is thus designed to be accessible to people across many different backgrounds, experiences and perspectives. Llama 3 addresses users and their needs as they are, without insertion unnecessary judgment or normativity, while reflecting the understanding that even content that may appear problematic in some cases can serve valuable purposes in others. It respects the dignity and autonomy of all users, especially in terms of the values of free thought and expression that power innovation and progress.
But Llama 3 is a new technology, and like any new technology, there are risks associated with its use. Testing conducted to date has been in English, and has not covered, nor could it cover, all scenarios. For these reasons, as with all LLMs, Llama 3’s potential outputs cannot be predicted in advance, and the model may in some instances produce inaccurate, biased or other objectionable responses to user prompts. Therefore, before deploying any applications of Llama 3 models, developers should perform safety testing and tuning tailored to their specific applications of the model. As outlined in the Responsible Use Guide, we recommend incorporating [Purple Llama](https://github.com/facebookresearch/PurpleLlama) solutions into your workflows and specifically [Llama Guard](https://ai.meta.com/research/publications/llama-guard-llm-based-input-output-safeguard-for-human-ai-conversations/) which provides a base model to filter input and output prompts to layer system-level safety on top of model-level safety.
Please see the Responsible Use Guide available at [http://llama.meta.com/responsible-use-guide](http://llama.meta.com/responsible-use-guide)
## Citation instructions
@article{llama3modelcard,
title={Llama 3 Model Card},
author={AI@Meta},
year={2024},
url = {https://github.com/meta-llama/llama3/blob/main/MODEL_CARD.md}
}
## Contributors
Aaditya Singh; Aaron Grattafiori; Abhimanyu Dubey; Abhinav Jauhri; Abhinav Pandey; Abhishek Kadian; Adam Kelsey; Adi Gangidi; Ahmad Al-Dahle; Ahuva Goldstand; Aiesha Letman; Ajay Menon; Akhil Mathur; Alan Schelten; Alex Vaughan; Amy Yang; Andrei Lupu; Andres Alvarado; Andrew Gallagher; Andrew Gu; Andrew Ho; Andrew Poulton; Andrew Ryan; Angela Fan; Ankit Ramchandani; Anthony Hartshorn; Archi Mitra; Archie Sravankumar; Artem Korenev; Arun Rao; Ashley Gabriel; Ashwin Bharambe; Assaf Eisenman; Aston Zhang; Aurelien Rodriguez; Austen Gregerson; Ava Spataru; Baptiste Roziere; Ben Maurer; Benjamin Leonhardi; Bernie Huang; Bhargavi Paranjape; Bing Liu; Binh Tang; Bobbie Chern; Brani Stojkovic; Brian Fuller; Catalina Mejia Arenas; Chao Zhou; Charlotte Caucheteux; Chaya Nayak; Ching-Hsiang Chu; Chloe Bi; Chris Cai; Chris Cox; Chris Marra; Chris McConnell; Christian Keller; Christoph Feichtenhofer; Christophe Touret; Chunyang Wu; Corinne Wong; Cristian Canton Ferrer; Damien Allonsius; Daniel Kreymer; Daniel Haziza; Daniel Li; Danielle Pintz; Danny Livshits; Danny Wyatt; David Adkins; David Esiobu; David Xu; Davide Testuggine; Delia David; Devi Parikh; Dhruv Choudhary; Dhruv Mahajan; Diana Liskovich; Diego Garcia-Olano; Diego Perino; Dieuwke Hupkes; Dingkang Wang; Dustin Holland; Egor Lakomkin; Elina Lobanova; Xiaoqing Ellen Tan; Emily Dinan; Eric Smith; Erik Brinkman; Esteban Arcaute; Filip Radenovic; Firat Ozgenel; Francesco Caggioni; Frank Seide; Frank Zhang; Gabriel Synnaeve; Gabriella Schwarz; Gabrielle Lee; Gada Badeer; Georgia Anderson; Graeme Nail; Gregoire Mialon; Guan Pang; Guillem Cucurell; Hailey Nguyen; Hannah Korevaar; Hannah Wang; Haroun Habeeb; Harrison Rudolph; Henry Aspegren; Hu Xu; Hugo Touvron; Iga Kozlowska; Igor Molybog; Igor Tufanov; Iliyan Zarov; Imanol Arrieta Ibarra; Irina-Elena Veliche; Isabel Kloumann; Ishan Misra; Ivan Evtimov; Jacob Xu; Jade Copet; Jake Weissman; Jan Geffert; Jana Vranes; Japhet Asher; Jason Park; Jay Mahadeokar; Jean-Baptiste Gaya; Jeet Shah; Jelmer van der Linde; Jennifer Chan; Jenny Hong; Jenya Lee; Jeremy Fu; Jeremy Teboul; Jianfeng Chi; Jianyu Huang; Jie Wang; Jiecao Yu; Joanna Bitton; Joe Spisak; Joelle Pineau; Jon Carvill; Jongsoo Park; Joseph Rocca; Joshua Johnstun; Junteng Jia; Kalyan Vasuden Alwala; Kam Hou U; Kate Plawiak; Kartikeya Upasani; Kaushik Veeraraghavan; Ke Li; Kenneth Heafield; Kevin Stone; Khalid El-Arini; Krithika Iyer; Kshitiz Malik; Kuenley Chiu; Kunal Bhalla; Kyle Huang; Lakshya Garg; Lauren Rantala-Yeary; Laurens van der Maaten; Lawrence Chen; Leandro Silva; Lee Bell; Lei Zhang; Liang Tan; Louis Martin; Lovish Madaan; Luca Wehrstedt; Lukas Blecher; Luke de Oliveira; Madeline Muzzi; Madian Khabsa; Manav Avlani; Mannat Singh; Manohar Paluri; Mark Zuckerberg; Marcin Kardas; Martynas Mankus; Mathew Oldham; Mathieu Rita; Matthew Lennie; Maya Pavlova; Meghan Keneally; Melanie Kambadur; Mihir Patel; Mikayel Samvelyan; Mike Clark; Mike Lewis; Min Si; Mitesh Kumar Singh; Mo Metanat; Mona Hassan; Naman Goyal; Narjes Torabi; Nicolas Usunier; Nikolay Bashlykov; Nikolay Bogoychev; Niladri Chatterji; Ning Dong; Oliver Aobo Yang; Olivier Duchenne; Onur Celebi; Parth Parekh; Patrick Alrassy; Paul Saab; Pavan Balaji; Pedro Rittner; Pengchuan Zhang; Pengwei Li; Petar Vasic; Peter Weng; Polina Zvyagina; Prajjwal Bhargava; Pratik Dubal; Praveen Krishnan; Punit Singh Koura; Qing He; Rachel Rodriguez; Ragavan Srinivasan; Rahul Mitra; Ramon Calderer; Raymond Li; Robert Stojnic; Roberta Raileanu; Robin Battey; Rocky Wang; Rohit Girdhar; Rohit Patel; Romain Sauvestre; Ronnie Polidoro; Roshan Sumbaly; Ross Taylor; Ruan Silva; Rui Hou; Rui Wang; Russ Howes; Ruty Rinott; Saghar Hosseini; Sai Jayesh Bondu; Samyak Datta; Sanjay Singh; Sara Chugh; Sargun Dhillon; Satadru Pan; Sean Bell; Sergey Edunov; Shaoliang Nie; Sharan Narang; Sharath Raparthy; Shaun Lindsay; Sheng Feng; Sheng Shen; Shenghao Lin; Shiva Shankar; Shruti Bhosale; Shun Zhang; Simon Vandenhende; Sinong Wang; Seohyun Sonia Kim; Soumya Batra; Sten Sootla; Steve Kehoe; Suchin Gururangan; Sumit Gupta; Sunny Virk; Sydney Borodinsky; Tamar Glaser; Tamar Herman; Tamara Best; Tara Fowler; Thomas Georgiou; Thomas Scialom; Tianhe Li; Todor Mihaylov; Tong Xiao; Ujjwal Karn; Vedanuj Goswami; Vibhor Gupta; Vignesh Ramanathan; Viktor Kerkez; Vinay Satish Kumar; Vincent Gonguet; Vish Vogeti; Vlad Poenaru; Vlad Tiberiu Mihailescu; Vladan Petrovic; Vladimir Ivanov; Wei Li; Weiwei Chu; Wenhan Xiong; Wenyin Fu; Wes Bouaziz; Whitney Meers; Will Constable; Xavier Martinet; Xiaojian Wu; Xinbo Gao; Xinfeng Xie; Xuchao Jia; Yaelle Goldschlag; Yann LeCun; Yashesh Gaur; Yasmine Babaei; Ye Qi; Yenda Li; Yi Wen; Yiwen Song; Youngjin Nam; Yuchen Hao; Yuchen Zhang; Yun Wang; Yuning Mao; Yuzi He; Zacharie Delpierre Coudert; Zachary DeVito; Zahra Hankir; Zhaoduo Wen; Zheng Yan; Zhengxing Chen; Zhenyu Yang; Zoe Papakipos
---
|
flair/ner-english-fast | flair | "2024-07-21T19:24:44Z" | 1,314,791 | 20 | flair | [
"flair",
"pytorch",
"token-classification",
"sequence-tagger-model",
"en",
"dataset:conll2003",
"region:us"
] | token-classification | "2022-03-02T23:29:05Z" | ---
tags:
- flair
- token-classification
- sequence-tagger-model
language: en
datasets:
- conll2003
widget:
- text: "George Washington went to Washington"
---
## English NER in Flair (fast model)
This is the fast 4-class NER model for English that ships with [Flair](https://github.com/flairNLP/flair/).
F1-Score: **92,92** (corrected CoNLL-03)
Predicts 4 tags:
| **tag** | **meaning** |
|---------------------------------|-----------|
| PER | person name |
| LOC | location name |
| ORG | organization name |
| MISC | other name |
Based on [Flair embeddings](https://www.aclweb.org/anthology/C18-1139/) and LSTM-CRF.
---
### Demo: How to use in Flair
Requires: **[Flair](https://github.com/flairNLP/flair/)** (`pip install flair`)
```python
from flair.data import Sentence
from flair.models import SequenceTagger
# load tagger
tagger = SequenceTagger.load("flair/ner-english-fast")
# make example sentence
sentence = Sentence("George Washington went to Washington")
# predict NER tags
tagger.predict(sentence)
# print sentence
print(sentence)
# print predicted NER spans
print('The following NER tags are found:')
# iterate over entities and print
for entity in sentence.get_spans('ner'):
print(entity)
```
This yields the following output:
```
Span [1,2]: "George Washington" [− Labels: PER (0.9515)]
Span [5]: "Washington" [− Labels: LOC (0.992)]
```
So, the entities "*George Washington*" (labeled as a **person**) and "*Washington*" (labeled as a **location**) are found in the sentence "*George Washington went to Washington*".
---
### Training: Script to train this model
The following Flair script was used to train this model:
```python
from flair.data import Corpus
from flair.datasets import CONLL_03
from flair.embeddings import WordEmbeddings, StackedEmbeddings, FlairEmbeddings
# 1. get the corpus
corpus: Corpus = CONLL_03()
# 2. what tag do we want to predict?
tag_type = 'ner'
# 3. make the tag dictionary from the corpus
tag_dictionary = corpus.make_tag_dictionary(tag_type=tag_type)
# 4. initialize each embedding we use
embedding_types = [
# GloVe embeddings
WordEmbeddings('glove'),
# contextual string embeddings, forward
FlairEmbeddings('news-forward-fast'),
# contextual string embeddings, backward
FlairEmbeddings('news-backward-fast'),
]
# embedding stack consists of Flair and GloVe embeddings
embeddings = StackedEmbeddings(embeddings=embedding_types)
# 5. initialize sequence tagger
from flair.models import SequenceTagger
tagger = SequenceTagger(hidden_size=256,
embeddings=embeddings,
tag_dictionary=tag_dictionary,
tag_type=tag_type)
# 6. initialize trainer
from flair.trainers import ModelTrainer
trainer = ModelTrainer(tagger, corpus)
# 7. run training
trainer.train('resources/taggers/ner-english',
train_with_dev=True,
max_epochs=150)
```
---
### Cite
Please cite the following paper when using this model.
```
@inproceedings{akbik2018coling,
title={Contextual String Embeddings for Sequence Labeling},
author={Akbik, Alan and Blythe, Duncan and Vollgraf, Roland},
booktitle = {{COLING} 2018, 27th International Conference on Computational Linguistics},
pages = {1638--1649},
year = {2018}
}
```
---
### Issues?
The Flair issue tracker is available [here](https://github.com/flairNLP/flair/issues/).
|
facebook/dinov2-small | facebook | "2023-09-06T11:24:10Z" | 1,314,553 | 22 | transformers | [
"transformers",
"pytorch",
"safetensors",
"dinov2",
"image-feature-extraction",
"dino",
"vision",
"arxiv:2304.07193",
"license:apache-2.0",
"endpoints_compatible",
"region:us"
] | image-feature-extraction | "2023-07-31T16:53:09Z" | ---
license: apache-2.0
tags:
- dino
- vision
---
# Vision Transformer (small-sized model) trained using DINOv2
Vision Transformer (ViT) model trained using the DINOv2 method. It was introduced in the paper [DINOv2: Learning Robust Visual Features without Supervision](https://arxiv.org/abs/2304.07193) by Oquab et al. and first released in [this repository](https://github.com/facebookresearch/dinov2).
Disclaimer: The team releasing DINOv2 did not write a model card for this model so this model card has been written by the Hugging Face team.
## Model description
The Vision Transformer (ViT) is a transformer encoder model (BERT-like) pretrained on a large collection of images in a self-supervised fashion.
Images are presented to the model as a sequence of fixed-size patches, which are linearly embedded. One also adds a [CLS] token to the beginning of a sequence to use it for classification tasks. One also adds absolute position embeddings before feeding the sequence to the layers of the Transformer encoder.
Note that this model does not include any fine-tuned heads.
By pre-training the model, it learns an inner representation of images that can then be used to extract features useful for downstream tasks: if you have a dataset of labeled images for instance, you can train a standard classifier by placing a linear layer on top of the pre-trained encoder. One typically places a linear layer on top of the [CLS] token, as the last hidden state of this token can be seen as a representation of an entire image.
## Intended uses & limitations
You can use the raw model for feature extraction. See the [model hub](https://huggingface.co/models?search=facebook/dinov2) to look for
fine-tuned versions on a task that interests you.
### How to use
Here is how to use this model:
```python
from transformers import AutoImageProcessor, AutoModel
from PIL import Image
import requests
url = 'http://images.cocodataset.org/val2017/000000039769.jpg'
image = Image.open(requests.get(url, stream=True).raw)
processor = AutoImageProcessor.from_pretrained('facebook/dinov2-small')
model = AutoModel.from_pretrained('facebook/dinov2-small')
inputs = processor(images=image, return_tensors="pt")
outputs = model(**inputs)
last_hidden_states = outputs.last_hidden_state
```
### BibTeX entry and citation info
```bibtex
misc{oquab2023dinov2,
title={DINOv2: Learning Robust Visual Features without Supervision},
author={Maxime Oquab and Timothée Darcet and Théo Moutakanni and Huy Vo and Marc Szafraniec and Vasil Khalidov and Pierre Fernandez and Daniel Haziza and Francisco Massa and Alaaeldin El-Nouby and Mahmoud Assran and Nicolas Ballas and Wojciech Galuba and Russell Howes and Po-Yao Huang and Shang-Wen Li and Ishan Misra and Michael Rabbat and Vasu Sharma and Gabriel Synnaeve and Hu Xu and Hervé Jegou and Julien Mairal and Patrick Labatut and Armand Joulin and Piotr Bojanowski},
year={2023},
eprint={2304.07193},
archivePrefix={arXiv},
primaryClass={cs.CV}
}
``` |
MaziyarPanahi/Llama-3.2-1B-Instruct-GGUF | MaziyarPanahi | "2024-09-25T19:31:01Z" | 1,314,390 | 4 | null | [
"gguf",
"mistral",
"quantized",
"2-bit",
"3-bit",
"4-bit",
"5-bit",
"6-bit",
"8-bit",
"GGUF",
"text-generation",
"base_model:meta-llama/Llama-3.2-1B-Instruct",
"base_model:quantized:meta-llama/Llama-3.2-1B-Instruct",
"region:us",
"imatrix",
"conversational"
] | text-generation | "2024-09-25T19:26:01Z" | ---
tags:
- quantized
- 2-bit
- 3-bit
- 4-bit
- 5-bit
- 6-bit
- 8-bit
- GGUF
- text-generation
- text-generation
model_name: Llama-3.2-1B-Instruct-GGUF
base_model: meta-llama/Llama-3.2-1B-Instruct
inference: false
model_creator: meta-llama
pipeline_tag: text-generation
quantized_by: MaziyarPanahi
---
# [MaziyarPanahi/Llama-3.2-1B-Instruct-GGUF](https://huggingface.co/MaziyarPanahi/Llama-3.2-1B-Instruct-GGUF)
- Model creator: [meta-llama](https://huggingface.co/meta-llama)
- Original model: [meta-llama/Llama-3.2-1B-Instruct](https://huggingface.co/meta-llama/Llama-3.2-1B-Instruct)
## Description
[MaziyarPanahi/Llama-3.2-1B-Instruct-GGUF](https://huggingface.co/MaziyarPanahi/Llama-3.2-1B-Instruct-GGUF) contains GGUF format model files for [meta-llama/Llama-3.2-1B-Instruct](https://huggingface.co/meta-llama/Llama-3.2-1B-Instruct).
### About GGUF
GGUF is a new format introduced by the llama.cpp team on August 21st 2023. It is a replacement for GGML, which is no longer supported by llama.cpp.
Here is an incomplete list of clients and libraries that are known to support GGUF:
* [llama.cpp](https://github.com/ggerganov/llama.cpp). The source project for GGUF. Offers a CLI and a server option.
* [llama-cpp-python](https://github.com/abetlen/llama-cpp-python), a Python library with GPU accel, LangChain support, and OpenAI-compatible API server.
* [LM Studio](https://lmstudio.ai/), an easy-to-use and powerful local GUI for Windows and macOS (Silicon), with GPU acceleration. Linux available, in beta as of 27/11/2023.
* [text-generation-webui](https://github.com/oobabooga/text-generation-webui), the most widely used web UI, with many features and powerful extensions. Supports GPU acceleration.
* [KoboldCpp](https://github.com/LostRuins/koboldcpp), a fully featured web UI, with GPU accel across all platforms and GPU architectures. Especially good for story telling.
* [GPT4All](https://gpt4all.io/index.html), a free and open source local running GUI, supporting Windows, Linux and macOS with full GPU accel.
* [LoLLMS Web UI](https://github.com/ParisNeo/lollms-webui), a great web UI with many interesting and unique features, including a full model library for easy model selection.
* [Faraday.dev](https://faraday.dev/), an attractive and easy to use character-based chat GUI for Windows and macOS (both Silicon and Intel), with GPU acceleration.
* [candle](https://github.com/huggingface/candle), a Rust ML framework with a focus on performance, including GPU support, and ease of use.
* [ctransformers](https://github.com/marella/ctransformers), a Python library with GPU accel, LangChain support, and OpenAI-compatible AI server. Note, as of time of writing (November 27th 2023), ctransformers has not been updated in a long time and does not support many recent models.
## Special thanks
🙏 Special thanks to [Georgi Gerganov](https://github.com/ggerganov) and the whole team working on [llama.cpp](https://github.com/ggerganov/llama.cpp/) for making all of this possible. |
MaziyarPanahi/Llama-3-8B-Instruct-32k-v0.1-GGUF | MaziyarPanahi | "2024-06-28T10:42:58Z" | 1,314,367 | 56 | transformers | [
"transformers",
"gguf",
"mistral",
"quantized",
"2-bit",
"3-bit",
"4-bit",
"5-bit",
"6-bit",
"8-bit",
"GGUF",
"text-generation",
"base_model:MaziyarPanahi/Llama-3-8B-Instruct-32k-v0.1",
"base_model:quantized:MaziyarPanahi/Llama-3-8B-Instruct-32k-v0.1",
"region:us",
"imatrix",
"conversational"
] | text-generation | "2024-04-24T16:01:52Z" | ---
tags:
- quantized
- 2-bit
- 3-bit
- 4-bit
- 5-bit
- 6-bit
- 8-bit
- GGUF
- text-generation
- text-generation
model_name: Llama-3-8B-Instruct-32k-v0.1-GGUF
base_model: MaziyarPanahi/Llama-3-8B-Instruct-32k-v0.1
inference: false
model_creator: MaziyarPanahi
pipeline_tag: text-generation
quantized_by: MaziyarPanahi
---
# [MaziyarPanahi/Llama-3-8B-Instruct-32k-v0.1-GGUF](https://huggingface.co/MaziyarPanahi/Llama-3-8B-Instruct-32k-v0.1-GGUF)
- Model creator: [MaziyarPanahi](https://huggingface.co/MaziyarPanahi)
- Original model: [MaziyarPanahi/Llama-3-8B-Instruct-32k-v0.1](https://huggingface.co/MaziyarPanahi/Llama-3-8B-Instruct-32k-v0.1)
## Description
[MaziyarPanahi/Llama-3-8B-Instruct-32k-v0.1-GGUF](https://huggingface.co/MaziyarPanahi/Llama-3-8B-Instruct-32k-v0.1-GGUF) contains GGUF format model files for [MaziyarPanahi/Llama-3-8B-Instruct-32k-v0.1](https://huggingface.co/MaziyarPanahi/Llama-3-8B-Instruct-32k-v0.1).
### About GGUF
GGUF is a new format introduced by the llama.cpp team on August 21st 2023. It is a replacement for GGML, which is no longer supported by llama.cpp.
Here is an incomplete list of clients and libraries that are known to support GGUF:
* [llama.cpp](https://github.com/ggerganov/llama.cpp). The source project for GGUF. Offers a CLI and a server option.
* [llama-cpp-python](https://github.com/abetlen/llama-cpp-python), a Python library with GPU accel, LangChain support, and OpenAI-compatible API server.
* [LM Studio](https://lmstudio.ai/), an easy-to-use and powerful local GUI for Windows and macOS (Silicon), with GPU acceleration. Linux available, in beta as of 27/11/2023.
* [text-generation-webui](https://github.com/oobabooga/text-generation-webui), the most widely used web UI, with many features and powerful extensions. Supports GPU acceleration.
* [KoboldCpp](https://github.com/LostRuins/koboldcpp), a fully featured web UI, with GPU accel across all platforms and GPU architectures. Especially good for story telling.
* [GPT4All](https://gpt4all.io/index.html), a free and open source local running GUI, supporting Windows, Linux and macOS with full GPU accel.
* [LoLLMS Web UI](https://github.com/ParisNeo/lollms-webui), a great web UI with many interesting and unique features, including a full model library for easy model selection.
* [Faraday.dev](https://faraday.dev/), an attractive and easy to use character-based chat GUI for Windows and macOS (both Silicon and Intel), with GPU acceleration.
* [candle](https://github.com/huggingface/candle), a Rust ML framework with a focus on performance, including GPU support, and ease of use.
* [ctransformers](https://github.com/marella/ctransformers), a Python library with GPU accel, LangChain support, and OpenAI-compatible AI server. Note, as of time of writing (November 27th 2023), ctransformers has not been updated in a long time and does not support many recent models.
## Special thanks
🙏 Special thanks to [Georgi Gerganov](https://github.com/ggerganov) and the whole team working on [llama.cpp](https://github.com/ggerganov/llama.cpp/) for making all of this possible. |
dmis-lab/biobert-v1.1 | dmis-lab | "2021-05-19T16:03:17Z" | 1,303,741 | 68 | transformers | [
"transformers",
"pytorch",
"jax",
"bert",
"feature-extraction",
"endpoints_compatible",
"region:us"
] | feature-extraction | "2022-03-02T23:29:05Z" | Entry not found |
jonatasgrosman/wav2vec2-large-xlsr-53-japanese | jonatasgrosman | "2022-12-14T01:58:09Z" | 1,294,222 | 27 | transformers | [
"transformers",
"pytorch",
"jax",
"wav2vec2",
"automatic-speech-recognition",
"audio",
"speech",
"xlsr-fine-tuning-week",
"ja",
"dataset:common_voice",
"license:apache-2.0",
"model-index",
"endpoints_compatible",
"region:us"
] | automatic-speech-recognition | "2022-03-02T23:29:05Z" | ---
language: ja
datasets:
- common_voice
metrics:
- wer
- cer
tags:
- audio
- automatic-speech-recognition
- speech
- xlsr-fine-tuning-week
license: apache-2.0
model-index:
- name: XLSR Wav2Vec2 Japanese by Jonatas Grosman
results:
- task:
name: Speech Recognition
type: automatic-speech-recognition
dataset:
name: Common Voice ja
type: common_voice
args: ja
metrics:
- name: Test WER
type: wer
value: 81.80
- name: Test CER
type: cer
value: 20.16
---
# Fine-tuned XLSR-53 large model for speech recognition in Japanese
Fine-tuned [facebook/wav2vec2-large-xlsr-53](https://huggingface.co/facebook/wav2vec2-large-xlsr-53) on Japanese using the train and validation splits of [Common Voice 6.1](https://huggingface.co/datasets/common_voice), [CSS10](https://github.com/Kyubyong/css10) and [JSUT](https://sites.google.com/site/shinnosuketakamichi/publication/jsut).
When using this model, make sure that your speech input is sampled at 16kHz.
This model has been fine-tuned thanks to the GPU credits generously given by the [OVHcloud](https://www.ovhcloud.com/en/public-cloud/ai-training/) :)
The script used for training can be found here: https://github.com/jonatasgrosman/wav2vec2-sprint
## Usage
The model can be used directly (without a language model) as follows...
Using the [HuggingSound](https://github.com/jonatasgrosman/huggingsound) library:
```python
from huggingsound import SpeechRecognitionModel
model = SpeechRecognitionModel("jonatasgrosman/wav2vec2-large-xlsr-53-japanese")
audio_paths = ["/path/to/file.mp3", "/path/to/another_file.wav"]
transcriptions = model.transcribe(audio_paths)
```
Writing your own inference script:
```python
import torch
import librosa
from datasets import load_dataset
from transformers import Wav2Vec2ForCTC, Wav2Vec2Processor
LANG_ID = "ja"
MODEL_ID = "jonatasgrosman/wav2vec2-large-xlsr-53-japanese"
SAMPLES = 10
test_dataset = load_dataset("common_voice", LANG_ID, split=f"test[:{SAMPLES}]")
processor = Wav2Vec2Processor.from_pretrained(MODEL_ID)
model = Wav2Vec2ForCTC.from_pretrained(MODEL_ID)
# Preprocessing the datasets.
# We need to read the audio files as arrays
def speech_file_to_array_fn(batch):
speech_array, sampling_rate = librosa.load(batch["path"], sr=16_000)
batch["speech"] = speech_array
batch["sentence"] = batch["sentence"].upper()
return batch
test_dataset = test_dataset.map(speech_file_to_array_fn)
inputs = processor(test_dataset["speech"], sampling_rate=16_000, return_tensors="pt", padding=True)
with torch.no_grad():
logits = model(inputs.input_values, attention_mask=inputs.attention_mask).logits
predicted_ids = torch.argmax(logits, dim=-1)
predicted_sentences = processor.batch_decode(predicted_ids)
for i, predicted_sentence in enumerate(predicted_sentences):
print("-" * 100)
print("Reference:", test_dataset[i]["sentence"])
print("Prediction:", predicted_sentence)
```
| Reference | Prediction |
| ------------- | ------------- |
| 祖母は、おおむね機嫌よく、サイコロをころがしている。 | 人母は重にきね起くさいがしている |
| 財布をなくしたので、交番へ行きます。 | 財布をなく手端ので勾番へ行きます |
| 飲み屋のおやじ、旅館の主人、医者をはじめ、交際のある人にきいてまわったら、みんな、私より収入が多いはずなのに、税金は安い。 | ノ宮屋のお親じ旅館の主に医者をはじめ交際のアル人トに聞いて回ったらみんな私より収入が多いはなうに税金は安い |
| 新しい靴をはいて出かけます。 | だらしい靴をはいて出かけます |
| このためプラズマ中のイオンや電子の持つ平均運動エネルギーを温度で表現することがある | このためプラズマ中のイオンや電子の持つ平均運動エネルギーを温度で表弁することがある |
| 松井さんはサッカーより野球のほうが上手です。 | 松井さんはサッカーより野球のほうが上手です |
| 新しいお皿を使います。 | 新しいお皿を使います |
| 結婚以来三年半ぶりの東京も、旧友とのお酒も、夜行列車も、駅で寝て、朝を待つのも久しぶりだ。 | 結婚ル二来三年半降りの東京も吸とのお酒も野越者も駅で寝て朝を待つの久しぶりた |
| これまで、少年野球、ママさんバレーなど、地域スポーツを支え、市民に密着してきたのは、無数のボランティアだった。 | これまで少年野球<unk>三バレーなど地域スポーツを支え市民に満着してきたのは娘数のボランティアだった |
| 靴を脱いで、スリッパをはきます。 | 靴を脱いでスイパーをはきます |
## Evaluation
The model can be evaluated as follows on the Japanese test data of Common Voice.
```python
import torch
import re
import librosa
from datasets import load_dataset, load_metric
from transformers import Wav2Vec2ForCTC, Wav2Vec2Processor
LANG_ID = "ja"
MODEL_ID = "jonatasgrosman/wav2vec2-large-xlsr-53-japanese"
DEVICE = "cuda"
CHARS_TO_IGNORE = [",", "?", "¿", ".", "!", "¡", ";", ";", ":", '""', "%", '"', "�", "ʿ", "·", "჻", "~", "՞",
"؟", "،", "।", "॥", "«", "»", "„", "“", "”", "「", "」", "‘", "’", "《", "》", "(", ")", "[", "]",
"{", "}", "=", "`", "_", "+", "<", ">", "…", "–", "°", "´", "ʾ", "‹", "›", "©", "®", "—", "→", "。",
"、", "﹂", "﹁", "‧", "~", "﹏", ",", "{", "}", "(", ")", "[", "]", "【", "】", "‥", "〽",
"『", "』", "〝", "〟", "⟨", "⟩", "〜", ":", "!", "?", "♪", "؛", "/", "\\", "º", "−", "^", "'", "ʻ", "ˆ"]
test_dataset = load_dataset("common_voice", LANG_ID, split="test")
wer = load_metric("wer.py") # https://github.com/jonatasgrosman/wav2vec2-sprint/blob/main/wer.py
cer = load_metric("cer.py") # https://github.com/jonatasgrosman/wav2vec2-sprint/blob/main/cer.py
chars_to_ignore_regex = f"[{re.escape(''.join(CHARS_TO_IGNORE))}]"
processor = Wav2Vec2Processor.from_pretrained(MODEL_ID)
model = Wav2Vec2ForCTC.from_pretrained(MODEL_ID)
model.to(DEVICE)
# Preprocessing the datasets.
# We need to read the audio files as arrays
def speech_file_to_array_fn(batch):
with warnings.catch_warnings():
warnings.simplefilter("ignore")
speech_array, sampling_rate = librosa.load(batch["path"], sr=16_000)
batch["speech"] = speech_array
batch["sentence"] = re.sub(chars_to_ignore_regex, "", batch["sentence"]).upper()
return batch
test_dataset = test_dataset.map(speech_file_to_array_fn)
# Preprocessing the datasets.
# We need to read the audio files as arrays
def evaluate(batch):
inputs = processor(batch["speech"], sampling_rate=16_000, return_tensors="pt", padding=True)
with torch.no_grad():
logits = model(inputs.input_values.to(DEVICE), attention_mask=inputs.attention_mask.to(DEVICE)).logits
pred_ids = torch.argmax(logits, dim=-1)
batch["pred_strings"] = processor.batch_decode(pred_ids)
return batch
result = test_dataset.map(evaluate, batched=True, batch_size=8)
predictions = [x.upper() for x in result["pred_strings"]]
references = [x.upper() for x in result["sentence"]]
print(f"WER: {wer.compute(predictions=predictions, references=references, chunk_size=1000) * 100}")
print(f"CER: {cer.compute(predictions=predictions, references=references, chunk_size=1000) * 100}")
```
**Test Result**:
In the table below I report the Word Error Rate (WER) and the Character Error Rate (CER) of the model. I ran the evaluation script described above on other models as well (on 2021-05-10). Note that the table below may show different results from those already reported, this may have been caused due to some specificity of the other evaluation scripts used.
| Model | WER | CER |
| ------------- | ------------- | ------------- |
| jonatasgrosman/wav2vec2-large-xlsr-53-japanese | **81.80%** | **20.16%** |
| vumichien/wav2vec2-large-xlsr-japanese | 1108.86% | 23.40% |
| qqhann/w2v_hf_jsut_xlsr53 | 1012.18% | 70.77% |
## Citation
If you want to cite this model you can use this:
```bibtex
@misc{grosman2021xlsr53-large-japanese,
title={Fine-tuned {XLSR}-53 large model for speech recognition in {J}apanese},
author={Grosman, Jonatas},
howpublished={\url{https://huggingface.co/jonatasgrosman/wav2vec2-large-xlsr-53-japanese}},
year={2021}
}
``` |
trl-internal-testing/dummy-GPT2-correct-vocab | trl-internal-testing | "2024-09-24T11:26:20Z" | 1,288,961 | 0 | transformers | [
"transformers",
"safetensors",
"gpt2",
"text-generation",
"conversational",
"autotrain_compatible",
"text-generation-inference",
"endpoints_compatible",
"region:us"
] | text-generation | "2023-02-08T15:12:33Z" | ---
library_name: transformers
tags: []
---
# Dummy GPT2 for TRL testing
```python
from transformers import AutoTokenizer, GPT2Config, GPT2LMHeadModel
config = GPT2Config(n_positions=512, n_embd=32, n_layer=5, n_head=4, n_inner=37, pad_token_id=1023, is_decoder=True)
model = GPT2LMHeadModel(config)
tokenizer = AutoTokenizer.from_pretrained("openai-community/gpt2")
model_id = "trl-internal-testing/dummy-GPT2-correct-vocab"
model.push_to_hub(model_id)
tokenizer.chat_template = "{% for message in messages %}{% if message['role'] == 'user' %}{{ ' ' }}{% endif %}{{ message['content'] }}{% if not loop.last %}{{ ' ' }}{% endif %}{% endfor %}{{ eos_token }}"
tokenizer.push_to_hub(model_id)
config.push_to_hub(model_id)
```
|
MaziyarPanahi/WizardLM-2-7B-GGUF | MaziyarPanahi | "2024-04-15T18:39:24Z" | 1,286,738 | 74 | transformers | [
"transformers",
"gguf",
"mistral",
"quantized",
"2-bit",
"3-bit",
"4-bit",
"5-bit",
"6-bit",
"8-bit",
"GGUF",
"safetensors",
"text-generation",
"arxiv:2304.12244",
"arxiv:2306.08568",
"arxiv:2308.09583",
"license:apache-2.0",
"autotrain_compatible",
"endpoints_compatible",
"text-generation-inference",
"region:us",
"base_model:microsoft/WizardLM-2-7B",
"base_model:quantized:microsoft/WizardLM-2-7B"
] | text-generation | "2024-04-15T16:51:17Z" | ---
tags:
- quantized
- 2-bit
- 3-bit
- 4-bit
- 5-bit
- 6-bit
- 8-bit
- GGUF
- transformers
- safetensors
- mistral
- text-generation
- arxiv:2304.12244
- arxiv:2306.08568
- arxiv:2308.09583
- license:apache-2.0
- autotrain_compatible
- endpoints_compatible
- text-generation-inference
- region:us
- text-generation
model_name: WizardLM-2-7B-GGUF
base_model: microsoft/WizardLM-2-7B
inference: false
model_creator: microsoft
pipeline_tag: text-generation
quantized_by: MaziyarPanahi
---
# [MaziyarPanahi/WizardLM-2-7B-GGUF](https://huggingface.co/MaziyarPanahi/WizardLM-2-7B-GGUF)
- Model creator: [microsoft](https://huggingface.co/microsoft)
- Original model: [microsoft/WizardLM-2-7B](https://huggingface.co/microsoft/WizardLM-2-7B)
## Description
[MaziyarPanahi/WizardLM-2-7B-GGUF](https://huggingface.co/MaziyarPanahi/WizardLM-2-7B-GGUF) contains GGUF format model files for [microsoft/WizardLM-2-7B](https://huggingface.co/microsoft/WizardLM-2-7B).
## Prompt template
```
{system_prompt}
USER: {prompt}
ASSISTANT: </s>
```
or
```
A chat between a curious user and an artificial intelligence assistant. The assistant gives helpful,
detailed, and polite answers to the user's questions. USER: Hi ASSISTANT: Hello.</s>
USER: {prompt} ASSISTANT: </s>......
```
Taken from the original README
---
---
license: apache-2.0
---
<p style="font-size:20px;" align="center">
🏠 <a href="https://wizardlm.github.io/WizardLM2" target="_blank">WizardLM-2 Release Blog</a> </p>
<p align="center">
🤗 <a href="https://huggingface.co/collections/microsoft/wizardlm-2-661d403f71e6c8257dbd598a" target="_blank">HF Repo</a> •🐱 <a href="https://github.com/victorsungo/WizardLM/tree/main/WizardLM-2" target="_blank">Github Repo</a> • 🐦 <a href="https://twitter.com/WizardLM_AI" target="_blank">Twitter</a> • 📃 <a href="https://arxiv.org/abs/2304.12244" target="_blank">[WizardLM]</a> • 📃 <a href="https://arxiv.org/abs/2306.08568" target="_blank">[WizardCoder]</a> • 📃 <a href="https://arxiv.org/abs/2308.09583" target="_blank">[WizardMath]</a> <br>
</p>
<p align="center">
👋 Join our <a href="https://discord.gg/VZjjHtWrKs" target="_blank">Discord</a>
</p>
## News 🔥🔥🔥 [2024/04/15]
We introduce and opensource WizardLM-2, our next generation state-of-the-art large language models,
which have improved performance on complex chat, multilingual, reasoning and agent.
New family includes three cutting-edge models: WizardLM-2 8x22B, WizardLM-2 70B, and WizardLM-2 7B.
- WizardLM-2 8x22B is our most advanced model, demonstrates highly competitive performance compared to those leading proprietary works
and consistently outperforms all the existing state-of-the-art opensource models.
- WizardLM-2 70B reaches top-tier reasoning capabilities and is the first choice in the same size.
- WizardLM-2 7B is the fastest and achieves comparable performance with existing 10x larger opensource leading models.
For more details of WizardLM-2 please read our [release blog post](https://wizardlm.github.io/WizardLM2) and upcoming paper.
## Model Details
* **Model name**: WizardLM-2 7B
* **Developed by**: WizardLM@Microsoft AI
* **Base model**: [mistralai/Mistral-7B-v0.1](https://huggingface.co/mistralai/Mistral-7B-v0.1)
* **Parameters**: 7B
* **Language(s)**: Multilingual
* **Blog**: [Introducing WizardLM-2](https://wizardlm.github.io/WizardLM2)
* **Repository**: [https://github.com/nlpxucan/WizardLM](https://github.com/nlpxucan/WizardLM)
* **Paper**: WizardLM-2 (Upcoming)
* **License**: Apache2.0
## Model Capacities
**MT-Bench**
We also adopt the automatic MT-Bench evaluation framework based on GPT-4 proposed by lmsys to assess the performance of models.
The WizardLM-2 8x22B even demonstrates highly competitive performance compared to the most advanced proprietary models.
Meanwhile, WizardLM-2 7B and WizardLM-2 70B are all the top-performing models among the other leading baselines at 7B to 70B model scales.
<p align="center" width="100%">
<a ><img src="https://raw.githubusercontent.com/WizardLM/WizardLM2/main/static/images/mtbench.png" alt="MTBench" style="width: 96%; min-width: 300px; display: block; margin: auto;"></a>
</p>
**Human Preferences Evaluation**
We carefully collected a complex and challenging set consisting of real-world instructions, which includes main requirements of humanity, such as writing, coding, math, reasoning, agent, and multilingual.
We report the win:loss rate without tie:
- WizardLM-2 8x22B is just slightly falling behind GPT-4-1106-preview, and significantly stronger than Command R Plus and GPT4-0314.
- WizardLM-2 70B is better than GPT4-0613, Mistral-Large, and Qwen1.5-72B-Chat.
- WizardLM-2 7B is comparable with Qwen1.5-32B-Chat, and surpasses Qwen1.5-14B-Chat and Starling-LM-7B-beta.
<p align="center" width="100%">
<a ><img src="https://raw.githubusercontent.com/WizardLM/WizardLM2/main/static/images/winall.png" alt="Win" style="width: 96%; min-width: 300px; display: block; margin: auto;"></a>
</p>
## Method Overview
We built a **fully AI powered synthetic training system** to train WizardLM-2 models, please refer to our [blog](https://wizardlm.github.io/WizardLM2) for more details of this system.
<p align="center" width="100%">
<a ><img src="https://raw.githubusercontent.com/WizardLM/WizardLM2/main/static/images/exp_1.png" alt="Method" style="width: 96%; min-width: 300px; display: block; margin: auto;"></a>
</p>
## Usage
❗<b>Note for model system prompts usage:</b>
<b>WizardLM-2</b> adopts the prompt format from <b>Vicuna</b> and supports **multi-turn** conversation. The prompt should be as following:
```
A chat between a curious user and an artificial intelligence assistant. The assistant gives helpful,
detailed, and polite answers to the user's questions. USER: Hi ASSISTANT: Hello.</s>
USER: Who are you? ASSISTANT: I am WizardLM.</s>......
```
<b> Inference WizardLM-2 Demo Script</b>
We provide a WizardLM-2 inference demo [code](https://github.com/nlpxucan/WizardLM/tree/main/demo) on our github.
---
## How to use
Thanks to [TheBloke](https://huggingface.co/TheBloke) for preparing an amazing README on how to use GGUF models:
### About GGUF
GGUF is a new format introduced by the llama.cpp team on August 21st 2023. It is a replacement for GGML, which is no longer supported by llama.cpp.
Here is an incomplete list of clients and libraries that are known to support GGUF:
* [llama.cpp](https://github.com/ggerganov/llama.cpp). The source project for GGUF. Offers a CLI and a server option.
* [text-generation-webui](https://github.com/oobabooga/text-generation-webui), the most widely used web UI, with many features and powerful extensions. Supports GPU acceleration.
* [KoboldCpp](https://github.com/LostRuins/koboldcpp), a fully featured web UI, with GPU accel across all platforms and GPU architectures. Especially good for story telling.
* [GPT4All](https://gpt4all.io/index.html), a free and open source local running GUI, supporting Windows, Linux and macOS with full GPU accel.
* [LM Studio](https://lmstudio.ai/), an easy-to-use and powerful local GUI for Windows and macOS (Silicon), with GPU acceleration. Linux available, in beta as of 27/11/2023.
* [LoLLMS Web UI](https://github.com/ParisNeo/lollms-webui), a great web UI with many interesting and unique features, including a full model library for easy model selection.
* [Faraday.dev](https://faraday.dev/), an attractive and easy to use character-based chat GUI for Windows and macOS (both Silicon and Intel), with GPU acceleration.
* [llama-cpp-python](https://github.com/abetlen/llama-cpp-python), a Python library with GPU accel, LangChain support, and OpenAI-compatible API server.
* [candle](https://github.com/huggingface/candle), a Rust ML framework with a focus on performance, including GPU support, and ease of use.
* [ctransformers](https://github.com/marella/ctransformers), a Python library with GPU accel, LangChain support, and OpenAI-compatible AI server. Note, as of time of writing (November 27th 2023), ctransformers has not been updated in a long time and does not support many recent models.
### Explanation of quantisation methods
<details>
<summary>Click to see details</summary>
The new methods available are:
* GGML_TYPE_Q2_K - "type-1" 2-bit quantization in super-blocks containing 16 blocks, each block having 16 weight. Block scales and mins are quantized with 4 bits. This ends up effectively using 2.5625 bits per weight (bpw)
* GGML_TYPE_Q3_K - "type-0" 3-bit quantization in super-blocks containing 16 blocks, each block having 16 weights. Scales are quantized with 6 bits. This end up using 3.4375 bpw.
* GGML_TYPE_Q4_K - "type-1" 4-bit quantization in super-blocks containing 8 blocks, each block having 32 weights. Scales and mins are quantized with 6 bits. This ends up using 4.5 bpw.
* GGML_TYPE_Q5_K - "type-1" 5-bit quantization. Same super-block structure as GGML_TYPE_Q4_K resulting in 5.5 bpw
* GGML_TYPE_Q6_K - "type-0" 6-bit quantization. Super-blocks with 16 blocks, each block having 16 weights. Scales are quantized with 8 bits. This ends up using 6.5625 bpw
## How to download GGUF files
**Note for manual downloaders:** You almost never want to clone the entire repo! Multiple different quantisation formats are provided, and most users only want to pick and download a single file.
The following clients/libraries will automatically download models for you, providing a list of available models to choose from:
* LM Studio
* LoLLMS Web UI
* Faraday.dev
### In `text-generation-webui`
Under Download Model, you can enter the model repo: [MaziyarPanahi/WizardLM-2-7B-GGUF](https://huggingface.co/MaziyarPanahi/WizardLM-2-7B-GGUF) and below it, a specific filename to download, such as: WizardLM-2-7B-GGUF.Q4_K_M.gguf.
Then click Download.
### On the command line, including multiple files at once
I recommend using the `huggingface-hub` Python library:
```shell
pip3 install huggingface-hub
```
Then you can download any individual model file to the current directory, at high speed, with a command like this:
```shell
huggingface-cli download MaziyarPanahi/WizardLM-2-7B-GGUF WizardLM-2-7B.Q4_K_M.gguf --local-dir . --local-dir-use-symlinks False
```
</details>
<details>
<summary>More advanced huggingface-cli download usage (click to read)</summary>
You can also download multiple files at once with a pattern:
```shell
huggingface-cli download [MaziyarPanahi/WizardLM-2-7B-GGUF](https://huggingface.co/MaziyarPanahi/WizardLM-2-7B-GGUF) --local-dir . --local-dir-use-symlinks False --include='*Q4_K*gguf'
```
For more documentation on downloading with `huggingface-cli`, please see: [HF -> Hub Python Library -> Download files -> Download from the CLI](https://huggingface.co/docs/huggingface_hub/guides/download#download-from-the-cli).
To accelerate downloads on fast connections (1Gbit/s or higher), install `hf_transfer`:
```shell
pip3 install hf_transfer
```
And set environment variable `HF_HUB_ENABLE_HF_TRANSFER` to `1`:
```shell
HF_HUB_ENABLE_HF_TRANSFER=1 huggingface-cli download MaziyarPanahi/WizardLM-2-7B-GGUF WizardLM-2-7B.Q4_K_M.gguf --local-dir . --local-dir-use-symlinks False
```
Windows Command Line users: You can set the environment variable by running `set HF_HUB_ENABLE_HF_TRANSFER=1` before the download command.
</details>
## Example `llama.cpp` command
Make sure you are using `llama.cpp` from commit [d0cee0d](https://github.com/ggerganov/llama.cpp/commit/d0cee0d36d5be95a0d9088b674dbb27354107221) or later.
```shell
./main -ngl 35 -m WizardLM-2-7B.Q4_K_M.gguf --color -c 32768 --temp 0.7 --repeat_penalty 1.1 -n -1 -p "<|im_start|>system
{system_message}<|im_end|>
<|im_start|>user
{prompt}<|im_end|>
<|im_start|>assistant"
```
Change `-ngl 32` to the number of layers to offload to GPU. Remove it if you don't have GPU acceleration.
Change `-c 32768` to the desired sequence length. For extended sequence models - eg 8K, 16K, 32K - the necessary RoPE scaling parameters are read from the GGUF file and set by llama.cpp automatically. Note that longer sequence lengths require much more resources, so you may need to reduce this value.
If you want to have a chat-style conversation, replace the `-p <PROMPT>` argument with `-i -ins`
For other parameters and how to use them, please refer to [the llama.cpp documentation](https://github.com/ggerganov/llama.cpp/blob/master/examples/main/README.md)
## How to run in `text-generation-webui`
Further instructions can be found in the text-generation-webui documentation, here: [text-generation-webui/docs/04 ‐ Model Tab.md](https://github.com/oobabooga/text-generation-webui/blob/main/docs/04%20-%20Model%20Tab.md#llamacpp).
## How to run from Python code
You can use GGUF models from Python using the [llama-cpp-python](https://github.com/abetlen/llama-cpp-python) or [ctransformers](https://github.com/marella/ctransformers) libraries. Note that at the time of writing (Nov 27th 2023), ctransformers has not been updated for some time and is not compatible with some recent models. Therefore I recommend you use llama-cpp-python.
### How to load this model in Python code, using llama-cpp-python
For full documentation, please see: [llama-cpp-python docs](https://github.com/abetlen/llama-cpp-python/).
#### First install the package
Run one of the following commands, according to your system:
```shell
# Base ctransformers with no GPU acceleration
pip install llama-cpp-python
# With NVidia CUDA acceleration
CMAKE_ARGS="-DLLAMA_CUBLAS=on" pip install llama-cpp-python
# Or with OpenBLAS acceleration
CMAKE_ARGS="-DLLAMA_BLAS=ON -DLLAMA_BLAS_VENDOR=OpenBLAS" pip install llama-cpp-python
# Or with CLBLast acceleration
CMAKE_ARGS="-DLLAMA_CLBLAST=on" pip install llama-cpp-python
# Or with AMD ROCm GPU acceleration (Linux only)
CMAKE_ARGS="-DLLAMA_HIPBLAS=on" pip install llama-cpp-python
# Or with Metal GPU acceleration for macOS systems only
CMAKE_ARGS="-DLLAMA_METAL=on" pip install llama-cpp-python
# In windows, to set the variables CMAKE_ARGS in PowerShell, follow this format; eg for NVidia CUDA:
$env:CMAKE_ARGS = "-DLLAMA_OPENBLAS=on"
pip install llama-cpp-python
```
#### Simple llama-cpp-python example code
```python
from llama_cpp import Llama
# Set gpu_layers to the number of layers to offload to GPU. Set to 0 if no GPU acceleration is available on your system.
llm = Llama(
model_path="./WizardLM-2-7B.Q4_K_M.gguf", # Download the model file first
n_ctx=32768, # The max sequence length to use - note that longer sequence lengths require much more resources
n_threads=8, # The number of CPU threads to use, tailor to your system and the resulting performance
n_gpu_layers=35 # The number of layers to offload to GPU, if you have GPU acceleration available
)
# Simple inference example
output = llm(
"<|im_start|>system
{system_message}<|im_end|>
<|im_start|>user
{prompt}<|im_end|>
<|im_start|>assistant", # Prompt
max_tokens=512, # Generate up to 512 tokens
stop=["</s>"], # Example stop token - not necessarily correct for this specific model! Please check before using.
echo=True # Whether to echo the prompt
)
# Chat Completion API
llm = Llama(model_path="./WizardLM-2-7B.Q4_K_M.gguf", chat_format="llama-2") # Set chat_format according to the model you are using
llm.create_chat_completion(
messages = [
{"role": "system", "content": "You are a story writing assistant."},
{
"role": "user",
"content": "Write a story about llamas."
}
]
)
```
## How to use with LangChain
Here are guides on using llama-cpp-python and ctransformers with LangChain:
* [LangChain + llama-cpp-python](https://python.langchain.com/docs/integrations/llms/llamacpp)
* [LangChain + ctransformers](https://python.langchain.com/docs/integrations/providers/ctransformers) |
TinyLlama/TinyLlama-1.1B-Chat-v1.0 | TinyLlama | "2024-03-17T05:07:08Z" | 1,286,527 | 1,088 | transformers | [
"transformers",
"safetensors",
"llama",
"text-generation",
"conversational",
"en",
"dataset:cerebras/SlimPajama-627B",
"dataset:bigcode/starcoderdata",
"dataset:HuggingFaceH4/ultrachat_200k",
"dataset:HuggingFaceH4/ultrafeedback_binarized",
"license:apache-2.0",
"autotrain_compatible",
"text-generation-inference",
"endpoints_compatible",
"region:us"
] | text-generation | "2023-12-30T06:27:30Z" | ---
license: apache-2.0
datasets:
- cerebras/SlimPajama-627B
- bigcode/starcoderdata
- HuggingFaceH4/ultrachat_200k
- HuggingFaceH4/ultrafeedback_binarized
language:
- en
widget:
- example_title: Fibonacci (Python)
messages:
- role: system
content: You are a chatbot who can help code!
- role: user
content: Write me a function to calculate the first 10 digits of the fibonacci sequence in Python and print it out to the CLI.
---
<div align="center">
# TinyLlama-1.1B
</div>
https://github.com/jzhang38/TinyLlama
The TinyLlama project aims to **pretrain** a **1.1B Llama model on 3 trillion tokens**. With some proper optimization, we can achieve this within a span of "just" 90 days using 16 A100-40G GPUs 🚀🚀. The training has started on 2023-09-01.
We adopted exactly the same architecture and tokenizer as Llama 2. This means TinyLlama can be plugged and played in many open-source projects built upon Llama. Besides, TinyLlama is compact with only 1.1B parameters. This compactness allows it to cater to a multitude of applications demanding a restricted computation and memory footprint.
#### This Model
This is the chat model finetuned on top of [TinyLlama/TinyLlama-1.1B-intermediate-step-1431k-3T](https://huggingface.co/TinyLlama/TinyLlama-1.1B-intermediate-step-1431k-3T). **We follow [HF's Zephyr](https://huggingface.co/HuggingFaceH4/zephyr-7b-alpha)'s training recipe.** The model was " initially fine-tuned on a variant of the [`UltraChat`](https://huggingface.co/datasets/stingning/ultrachat) dataset, which contains a diverse range of synthetic dialogues generated by ChatGPT.
We then further aligned the model with [🤗 TRL's](https://github.com/huggingface/trl) `DPOTrainer` on the [openbmb/UltraFeedback](https://huggingface.co/datasets/openbmb/UltraFeedback) dataset, which contain 64k prompts and model completions that are ranked by GPT-4."
#### How to use
You will need the transformers>=4.34
Do check the [TinyLlama](https://github.com/jzhang38/TinyLlama) github page for more information.
```python
# Install transformers from source - only needed for versions <= v4.34
# pip install git+https://github.com/huggingface/transformers.git
# pip install accelerate
import torch
from transformers import pipeline
pipe = pipeline("text-generation", model="TinyLlama/TinyLlama-1.1B-Chat-v1.0", torch_dtype=torch.bfloat16, device_map="auto")
# We use the tokenizer's chat template to format each message - see https://huggingface.co/docs/transformers/main/en/chat_templating
messages = [
{
"role": "system",
"content": "You are a friendly chatbot who always responds in the style of a pirate",
},
{"role": "user", "content": "How many helicopters can a human eat in one sitting?"},
]
prompt = pipe.tokenizer.apply_chat_template(messages, tokenize=False, add_generation_prompt=True)
outputs = pipe(prompt, max_new_tokens=256, do_sample=True, temperature=0.7, top_k=50, top_p=0.95)
print(outputs[0]["generated_text"])
# <|system|>
# You are a friendly chatbot who always responds in the style of a pirate.</s>
# <|user|>
# How many helicopters can a human eat in one sitting?</s>
# <|assistant|>
# ...
``` |
MaziyarPanahi/Llama-3-Smaug-8B-GGUF | MaziyarPanahi | "2024-04-20T07:42:51Z" | 1,280,914 | 4 | transformers | [
"transformers",
"gguf",
"mistral",
"quantized",
"2-bit",
"3-bit",
"4-bit",
"5-bit",
"6-bit",
"8-bit",
"GGUF",
"text-generation",
"mixtral",
"base_model:abacusai/Llama-3-Smaug-8B",
"base_model:quantized:abacusai/Llama-3-Smaug-8B",
"region:us",
"conversational"
] | text-generation | "2024-04-20T06:09:07Z" | ---
tags:
- quantized
- 2-bit
- 3-bit
- 4-bit
- 5-bit
- 6-bit
- 8-bit
- GGUF
- text-generation
- mixtral
- text-generation
model_name: Llama-3-Smaug-8B-GGUF
base_model: abacusai/Llama-3-Smaug-8B
inference: false
model_creator: abacusai
pipeline_tag: text-generation
quantized_by: MaziyarPanahi
---
# [MaziyarPanahi/Llama-3-Smaug-8B-GGUF](https://huggingface.co/MaziyarPanahi/Llama-3-Smaug-8B-GGUF)
- Model creator: [abacusai](https://huggingface.co/abacusai)
- Original model: [abacusai/Llama-3-Smaug-8B](https://huggingface.co/abacusai/Llama-3-Smaug-8B)
## Description
[MaziyarPanahi/Llama-3-Smaug-8B-GGUF](https://huggingface.co/MaziyarPanahi/Llama-3-Smaug-8B-GGUF) contains GGUF format model files for [abacusai/Llama-3-Smaug-8B](https://huggingface.co/abacusai/Llama-3-Smaug-8B).
## How to use
## Load GGUF models
You `MUST` follow the prompt template provided by Llama-3:
```sh
./llama.cpp/main -m Llama-3-Smaug-8B.Q2_K.gguf -r '<|eot_id|>' --in-prefix "\n<|start_header_id|>user<|end_header_id|>\n\n" --in-suffix "<|eot_id|><|start_header_id|>assistant<|end_header_id|>\n\n" -p "<|begin_of_text|><|start_header_id|>system<|end_header_id|>\n\nYou are a helpful, smart, kind, and efficient AI assistant. You always fulfill the user's requests to the best of your ability.<|eot_id|>\n<|start_header_id|>user<|end_header_id|>\n\nHi! How are you?<|eot_id|>\n<|start_header_id|>assistant<|end_header_id|>\n\n" -n 1024
```
### About GGUF
GGUF is a new format introduced by the llama.cpp team on August 21st 2023. It is a replacement for GGML, which is no longer supported by llama.cpp.
Here is an incomplete list of clients and libraries that are known to support GGUF:
* [llama.cpp](https://github.com/ggerganov/llama.cpp). The source project for GGUF. Offers a CLI and a server option.
* [text-generation-webui](https://github.com/oobabooga/text-generation-webui), the most widely used web UI, with many features and powerful extensions. Supports GPU acceleration.
* [KoboldCpp](https://github.com/LostRuins/koboldcpp), a fully featured web UI, with GPU accel across all platforms and GPU architectures. Especially good for story telling.
* [GPT4All](https://gpt4all.io/index.html), a free and open source local running GUI, supporting Windows, Linux and macOS with full GPU accel.
* [LM Studio](https://lmstudio.ai/), an easy-to-use and powerful local GUI for Windows and macOS (Silicon), with GPU acceleration. Linux available, in beta as of 27/11/2023.
* [LoLLMS Web UI](https://github.com/ParisNeo/lollms-webui), a great web UI with many interesting and unique features, including a full model library for easy model selection.
* [Faraday.dev](https://faraday.dev/), an attractive and easy to use character-based chat GUI for Windows and macOS (both Silicon and Intel), with GPU acceleration.
* [llama-cpp-python](https://github.com/abetlen/llama-cpp-python), a Python library with GPU accel, LangChain support, and OpenAI-compatible API server.
* [candle](https://github.com/huggingface/candle), a Rust ML framework with a focus on performance, including GPU support, and ease of use.
* [ctransformers](https://github.com/marella/ctransformers), a Python library with GPU accel, LangChain support, and OpenAI-compatible AI server. Note, as of time of writing (November 27th 2023), ctransformers has not been updated in a long time and does not support many recent models. |
nlptown/bert-base-multilingual-uncased-sentiment | nlptown | "2023-07-27T18:14:29Z" | 1,276,471 | 317 | transformers | [
"transformers",
"pytorch",
"tf",
"jax",
"bert",
"text-classification",
"en",
"nl",
"de",
"fr",
"it",
"es",
"doi:10.57967/hf/1515",
"license:mit",
"autotrain_compatible",
"endpoints_compatible",
"region:us"
] | text-classification | "2022-03-02T23:29:05Z" | ---
language:
- en
- nl
- de
- fr
- it
- es
license: mit
---
# bert-base-multilingual-uncased-sentiment
This is a bert-base-multilingual-uncased model finetuned for sentiment analysis on product reviews in six languages: English, Dutch, German, French, Spanish, and Italian. It predicts the sentiment of the review as a number of stars (between 1 and 5).
This model is intended for direct use as a sentiment analysis model for product reviews in any of the six languages above or for further finetuning on related sentiment analysis tasks.
## Training data
Here is the number of product reviews we used for finetuning the model:
| Language | Number of reviews |
| -------- | ----------------- |
| English | 150k |
| Dutch | 80k |
| German | 137k |
| French | 140k |
| Italian | 72k |
| Spanish | 50k |
## Accuracy
The fine-tuned model obtained the following accuracy on 5,000 held-out product reviews in each of the languages:
- Accuracy (exact) is the exact match for the number of stars.
- Accuracy (off-by-1) is the percentage of reviews where the number of stars the model predicts differs by a maximum of 1 from the number given by the human reviewer.
| Language | Accuracy (exact) | Accuracy (off-by-1) |
| -------- | ---------------------- | ------------------- |
| English | 67% | 95%
| Dutch | 57% | 93%
| German | 61% | 94%
| French | 59% | 94%
| Italian | 59% | 95%
| Spanish | 58% | 95%
## Contact
If you found this model useful, you can buy me a coffee at https://www.buymeacoffee.com/yvespeirsman.
In addition to this model, [NLP Town](http://nlp.town) offers custom models for many languages and NLP tasks.
Feel free to contact me for questions, feedback and/or requests for similar models. |
MaziyarPanahi/solar-pro-preview-instruct-GGUF | MaziyarPanahi | "2024-09-13T15:58:01Z" | 1,276,353 | 18 | null | [
"gguf",
"mistral",
"quantized",
"2-bit",
"3-bit",
"4-bit",
"5-bit",
"6-bit",
"8-bit",
"GGUF",
"text-generation",
"base_model:upstage/solar-pro-preview-instruct",
"base_model:quantized:upstage/solar-pro-preview-instruct",
"region:us",
"imatrix",
"conversational"
] | text-generation | "2024-09-13T13:44:40Z" | ---
tags:
- quantized
- 2-bit
- 3-bit
- 4-bit
- 5-bit
- 6-bit
- 8-bit
- GGUF
- text-generation
- text-generation
model_name: solar-pro-preview-instruct-GGUF
base_model: upstage/solar-pro-preview-instruct
inference: false
model_creator: upstage
pipeline_tag: text-generation
quantized_by: MaziyarPanahi
---
# [arcee-train/solar-pro-preview-instruct-GGUF](https://huggingface.co/arcee-train/solar-pro-preview-instruct-GGUF)
- Model creator: [upstage](https://huggingface.co/upstage)
- Original model: [upstage/solar-pro-preview-instruct](https://huggingface.co/upstage/solar-pro-preview-instruct)
## Description
[arcee-train/solar-pro-preview-instruct-GGUF](https://huggingface.co/arcee-train/solar-pro-preview-instruct-GGUF) contains GGUF format model files for [upstage/solar-pro-preview-instruct](https://huggingface.co/upstage/solar-pro-preview-instruct).
### About GGUF
GGUF is a new format introduced by the llama.cpp team on August 21st 2023. It is a replacement for GGML, which is no longer supported by llama.cpp.
Here is an incomplete list of clients and libraries that are known to support GGUF:
* [llama.cpp](https://github.com/ggerganov/llama.cpp). The source project for GGUF. Offers a CLI and a server option.
* [llama-cpp-python](https://github.com/abetlen/llama-cpp-python), a Python library with GPU accel, LangChain support, and OpenAI-compatible API server.
* [LM Studio](https://lmstudio.ai/), an easy-to-use and powerful local GUI for Windows and macOS (Silicon), with GPU acceleration. Linux available, in beta as of 27/11/2023.
* [text-generation-webui](https://github.com/oobabooga/text-generation-webui), the most widely used web UI, with many features and powerful extensions. Supports GPU acceleration.
* [KoboldCpp](https://github.com/LostRuins/koboldcpp), a fully featured web UI, with GPU accel across all platforms and GPU architectures. Especially good for story telling.
* [GPT4All](https://gpt4all.io/index.html), a free and open source local running GUI, supporting Windows, Linux and macOS with full GPU accel.
* [LoLLMS Web UI](https://github.com/ParisNeo/lollms-webui), a great web UI with many interesting and unique features, including a full model library for easy model selection.
* [Faraday.dev](https://faraday.dev/), an attractive and easy to use character-based chat GUI for Windows and macOS (both Silicon and Intel), with GPU acceleration.
* [candle](https://github.com/huggingface/candle), a Rust ML framework with a focus on performance, including GPU support, and ease of use.
* [ctransformers](https://github.com/marella/ctransformers), a Python library with GPU accel, LangChain support, and OpenAI-compatible AI server. Note, as of time of writing (November 27th 2023), ctransformers has not been updated in a long time and does not support many recent models.
## Special thanks
🙏 Special thanks to [Georgi Gerganov](https://github.com/ggerganov) and the whole team working on [llama.cpp](https://github.com/ggerganov/llama.cpp/) for making all of this possible. |
Qwen/Qwen2-0.5B | Qwen | "2024-10-22T13:47:30Z" | 1,271,059 | 110 | transformers | [
"transformers",
"safetensors",
"qwen2",
"text-generation",
"pretrained",
"conversational",
"en",
"license:apache-2.0",
"autotrain_compatible",
"text-generation-inference",
"endpoints_compatible",
"region:us"
] | text-generation | "2024-05-31T08:38:11Z" | ---
language:
- en
pipeline_tag: text-generation
tags:
- pretrained
license: apache-2.0
new_version: Qwen/Qwen2.5-0.5B
---
# Qwen2-0.5B
## Introduction
Qwen2 is the new series of Qwen large language models. For Qwen2, we release a number of base language models and instruction-tuned language models ranging from 0.5 to 72 billion parameters, including a Mixture-of-Experts model. This repo contains the 0.5B Qwen2 base language model.
Compared with the state-of-the-art opensource language models, including the previous released Qwen1.5, Qwen2 has generally surpassed most opensource models and demonstrated competitiveness against proprietary models across a series of benchmarks targeting for language understanding, language generation, multilingual capability, coding, mathematics, reasoning, etc.
For more details, please refer to our [blog](https://qwenlm.github.io/blog/qwen2/), [GitHub](https://github.com/QwenLM/Qwen2), and [Documentation](https://qwen.readthedocs.io/en/latest/).
<br>
## Model Details
Qwen2 is a language model series including decoder language models of different model sizes. For each size, we release the base language model and the aligned chat model. It is based on the Transformer architecture with SwiGLU activation, attention QKV bias, group query attention, etc. Additionally, we have an improved tokenizer adaptive to multiple natural languages and codes.
## Requirements
The code of Qwen2 has been in the latest Hugging face transformers and we advise you to install `transformers>=4.37.0`, or you might encounter the following error:
```
KeyError: 'qwen2'
```
## Usage
We do not advise you to use base language models for text generation. Instead, you can apply post-training, e.g., SFT, RLHF, continued pretraining, etc., on this model.
## Performance
The evaluation of base models mainly focuses on the model performance of natural language understanding, general question answering, coding, mathematics, scientific knowledge, reasoning, multilingual capability, etc.
The datasets for evaluation include:
**English Tasks**: MMLU (5-shot), MMLU-Pro (5-shot), GPQA (5shot), Theorem QA (5-shot), BBH (3-shot), HellaSwag (10-shot), Winogrande (5-shot), TruthfulQA (0-shot), ARC-C (25-shot)
**Coding Tasks**: EvalPlus (0-shot) (HumanEval, MBPP, HumanEval+, MBPP+), MultiPL-E (0-shot) (Python, C++, JAVA, PHP, TypeScript, C#, Bash, JavaScript)
**Math Tasks**: GSM8K (4-shot), MATH (4-shot)
**Chinese Tasks**: C-Eval(5-shot), CMMLU (5-shot)
**Multilingual Tasks**: Multi-Exam (M3Exam 5-shot, IndoMMLU 3-shot, ruMMLU 5-shot, mMMLU 5-shot), Multi-Understanding (BELEBELE 5-shot, XCOPA 5-shot, XWinograd 5-shot, XStoryCloze 0-shot, PAWS-X 5-shot), Multi-Mathematics (MGSM 8-shot), Multi-Translation (Flores-101 5-shot)
#### Qwen2-0.5B & Qwen2-1.5B performances
| Datasets | Phi-2 | Gemma-2B | MiniCPM | Qwen1.5-1.8B | Qwen2-0.5B | Qwen2-1.5B |
| :--------| :---------: | :------------: | :------------: |:------------: | :------------: | :------------: |
|#Non-Emb Params | 2.5B | 2.0B | 2.4B | 1.3B | 0.35B | 1.3B |
|MMLU | 52.7 | 42.3 | 53.5 | 46.8 | 45.4 | **56.5** |
|MMLU-Pro | - | 15.9 | - | - | 14.7 | 21.8 |
|Theorem QA | - | - | - |- | 8.9 | **15.0** |
|HumanEval | 47.6 | 22.0 |**50.0**| 20.1 | 22.0 | 31.1 |
|MBPP | **55.0** | 29.2 | 47.3 | 18.0 | 22.0 | 37.4 |
|GSM8K | 57.2 | 17.7 | 53.8 | 38.4 | 36.5 | **58.5** |
|MATH | 3.5 | 11.8 | 10.2 | 10.1 | 10.7 | **21.7** |
|BBH | **43.4** | 35.2 | 36.9 | 24.2 | 28.4 | 37.2 |
|HellaSwag | **73.1** | 71.4 | 68.3 | 61.4 | 49.3 | 66.6 |
|Winogrande | **74.4** | 66.8 | -| 60.3 | 56.8 | 66.2 |
|ARC-C | **61.1** | 48.5 | -| 37.9 | 31.5 | 43.9 |
|TruthfulQA | 44.5 | 33.1 | -| 39.4 | 39.7 | **45.9** |
|C-Eval | 23.4 | 28.0 | 51.1| 59.7 | 58.2 | **70.6** |
|CMMLU | 24.2 | - | 51.1 | 57.8 | 55.1 | **70.3** |
## Citation
If you find our work helpful, feel free to give us a cite.
```
@article{qwen2,
title={Qwen2 Technical Report},
year={2024}
}
``` |
CompVis/stable-diffusion-v1-4 | CompVis | "2023-08-23T21:15:42Z" | 1,270,675 | 6,560 | diffusers | [
"diffusers",
"safetensors",
"stable-diffusion",
"stable-diffusion-diffusers",
"text-to-image",
"arxiv:2207.12598",
"arxiv:2112.10752",
"arxiv:2103.00020",
"arxiv:2205.11487",
"arxiv:1910.09700",
"license:creativeml-openrail-m",
"autotrain_compatible",
"endpoints_compatible",
"diffusers:StableDiffusionPipeline",
"region:us"
] | text-to-image | "2022-08-20T13:26:13Z" | ---
license: creativeml-openrail-m
tags:
- stable-diffusion
- stable-diffusion-diffusers
- text-to-image
widget:
- text: "A high tech solarpunk utopia in the Amazon rainforest"
example_title: Amazon rainforest
- text: "A pikachu fine dining with a view to the Eiffel Tower"
example_title: Pikachu in Paris
- text: "A mecha robot in a favela in expressionist style"
example_title: Expressionist robot
- text: "an insect robot preparing a delicious meal"
example_title: Insect robot
- text: "A small cabin on top of a snowy mountain in the style of Disney, artstation"
example_title: Snowy disney cabin
extra_gated_prompt: |-
This model is open access and available to all, with a CreativeML OpenRAIL-M license further specifying rights and usage.
The CreativeML OpenRAIL License specifies:
1. You can't use the model to deliberately produce nor share illegal or harmful outputs or content
2. The authors claim no rights on the outputs you generate, you are free to use them and are accountable for their use which must not go against the provisions set in the license
3. You may re-distribute the weights and use the model commercially and/or as a service. If you do, please be aware you have to include the same use restrictions as the ones in the license and share a copy of the CreativeML OpenRAIL-M to all your users (please read the license entirely and carefully)
Please read the full license carefully here: https://huggingface.co/spaces/CompVis/stable-diffusion-license
extra_gated_heading: Please read the LICENSE to access this model
---
# Stable Diffusion v1-4 Model Card
Stable Diffusion is a latent text-to-image diffusion model capable of generating photo-realistic images given any text input.
For more information about how Stable Diffusion functions, please have a look at [🤗's Stable Diffusion with 🧨Diffusers blog](https://huggingface.co/blog/stable_diffusion).
The **Stable-Diffusion-v1-4** checkpoint was initialized with the weights of the [Stable-Diffusion-v1-2](https:/steps/huggingface.co/CompVis/stable-diffusion-v1-2)
checkpoint and subsequently fine-tuned on 225k steps at resolution 512x512 on "laion-aesthetics v2 5+" and 10% dropping of the text-conditioning to improve [classifier-free guidance sampling](https://arxiv.org/abs/2207.12598).
This weights here are intended to be used with the 🧨 Diffusers library. If you are looking for the weights to be loaded into the CompVis Stable Diffusion codebase, [come here](https://huggingface.co/CompVis/stable-diffusion-v-1-4-original)
## Model Details
- **Developed by:** Robin Rombach, Patrick Esser
- **Model type:** Diffusion-based text-to-image generation model
- **Language(s):** English
- **License:** [The CreativeML OpenRAIL M license](https://huggingface.co/spaces/CompVis/stable-diffusion-license) is an [Open RAIL M license](https://www.licenses.ai/blog/2022/8/18/naming-convention-of-responsible-ai-licenses), adapted from the work that [BigScience](https://bigscience.huggingface.co/) and [the RAIL Initiative](https://www.licenses.ai/) are jointly carrying in the area of responsible AI licensing. See also [the article about the BLOOM Open RAIL license](https://bigscience.huggingface.co/blog/the-bigscience-rail-license) on which our license is based.
- **Model Description:** This is a model that can be used to generate and modify images based on text prompts. It is a [Latent Diffusion Model](https://arxiv.org/abs/2112.10752) that uses a fixed, pretrained text encoder ([CLIP ViT-L/14](https://arxiv.org/abs/2103.00020)) as suggested in the [Imagen paper](https://arxiv.org/abs/2205.11487).
- **Resources for more information:** [GitHub Repository](https://github.com/CompVis/stable-diffusion), [Paper](https://arxiv.org/abs/2112.10752).
- **Cite as:**
@InProceedings{Rombach_2022_CVPR,
author = {Rombach, Robin and Blattmann, Andreas and Lorenz, Dominik and Esser, Patrick and Ommer, Bj\"orn},
title = {High-Resolution Image Synthesis With Latent Diffusion Models},
booktitle = {Proceedings of the IEEE/CVF Conference on Computer Vision and Pattern Recognition (CVPR)},
month = {June},
year = {2022},
pages = {10684-10695}
}
## Examples
We recommend using [🤗's Diffusers library](https://github.com/huggingface/diffusers) to run Stable Diffusion.
### PyTorch
```bash
pip install --upgrade diffusers transformers scipy
```
Running the pipeline with the default PNDM scheduler:
```python
import torch
from diffusers import StableDiffusionPipeline
model_id = "CompVis/stable-diffusion-v1-4"
device = "cuda"
pipe = StableDiffusionPipeline.from_pretrained(model_id, torch_dtype=torch.float16)
pipe = pipe.to(device)
prompt = "a photo of an astronaut riding a horse on mars"
image = pipe(prompt).images[0]
image.save("astronaut_rides_horse.png")
```
**Note**:
If you are limited by GPU memory and have less than 4GB of GPU RAM available, please make sure to load the StableDiffusionPipeline in float16 precision instead of the default float32 precision as done above. You can do so by telling diffusers to expect the weights to be in float16 precision:
```py
import torch
pipe = StableDiffusionPipeline.from_pretrained(model_id, torch_dtype=torch.float16)
pipe = pipe.to(device)
pipe.enable_attention_slicing()
prompt = "a photo of an astronaut riding a horse on mars"
image = pipe(prompt).images[0]
image.save("astronaut_rides_horse.png")
```
To swap out the noise scheduler, pass it to `from_pretrained`:
```python
from diffusers import StableDiffusionPipeline, EulerDiscreteScheduler
model_id = "CompVis/stable-diffusion-v1-4"
# Use the Euler scheduler here instead
scheduler = EulerDiscreteScheduler.from_pretrained(model_id, subfolder="scheduler")
pipe = StableDiffusionPipeline.from_pretrained(model_id, scheduler=scheduler, torch_dtype=torch.float16)
pipe = pipe.to("cuda")
prompt = "a photo of an astronaut riding a horse on mars"
image = pipe(prompt).images[0]
image.save("astronaut_rides_horse.png")
```
### JAX/Flax
To use StableDiffusion on TPUs and GPUs for faster inference you can leverage JAX/Flax.
Running the pipeline with default PNDMScheduler
```python
import jax
import numpy as np
from flax.jax_utils import replicate
from flax.training.common_utils import shard
from diffusers import FlaxStableDiffusionPipeline
pipeline, params = FlaxStableDiffusionPipeline.from_pretrained(
"CompVis/stable-diffusion-v1-4", revision="flax", dtype=jax.numpy.bfloat16
)
prompt = "a photo of an astronaut riding a horse on mars"
prng_seed = jax.random.PRNGKey(0)
num_inference_steps = 50
num_samples = jax.device_count()
prompt = num_samples * [prompt]
prompt_ids = pipeline.prepare_inputs(prompt)
# shard inputs and rng
params = replicate(params)
prng_seed = jax.random.split(prng_seed, num_samples)
prompt_ids = shard(prompt_ids)
images = pipeline(prompt_ids, params, prng_seed, num_inference_steps, jit=True).images
images = pipeline.numpy_to_pil(np.asarray(images.reshape((num_samples,) + images.shape[-3:])))
```
**Note**:
If you are limited by TPU memory, please make sure to load the `FlaxStableDiffusionPipeline` in `bfloat16` precision instead of the default `float32` precision as done above. You can do so by telling diffusers to load the weights from "bf16" branch.
```python
import jax
import numpy as np
from flax.jax_utils import replicate
from flax.training.common_utils import shard
from diffusers import FlaxStableDiffusionPipeline
pipeline, params = FlaxStableDiffusionPipeline.from_pretrained(
"CompVis/stable-diffusion-v1-4", revision="bf16", dtype=jax.numpy.bfloat16
)
prompt = "a photo of an astronaut riding a horse on mars"
prng_seed = jax.random.PRNGKey(0)
num_inference_steps = 50
num_samples = jax.device_count()
prompt = num_samples * [prompt]
prompt_ids = pipeline.prepare_inputs(prompt)
# shard inputs and rng
params = replicate(params)
prng_seed = jax.random.split(prng_seed, num_samples)
prompt_ids = shard(prompt_ids)
images = pipeline(prompt_ids, params, prng_seed, num_inference_steps, jit=True).images
images = pipeline.numpy_to_pil(np.asarray(images.reshape((num_samples,) + images.shape[-3:])))
```
# Uses
## Direct Use
The model is intended for research purposes only. Possible research areas and
tasks include
- Safe deployment of models which have the potential to generate harmful content.
- Probing and understanding the limitations and biases of generative models.
- Generation of artworks and use in design and other artistic processes.
- Applications in educational or creative tools.
- Research on generative models.
Excluded uses are described below.
### Misuse, Malicious Use, and Out-of-Scope Use
_Note: This section is taken from the [DALLE-MINI model card](https://huggingface.co/dalle-mini/dalle-mini), but applies in the same way to Stable Diffusion v1_.
The model should not be used to intentionally create or disseminate images that create hostile or alienating environments for people. This includes generating images that people would foreseeably find disturbing, distressing, or offensive; or content that propagates historical or current stereotypes.
#### Out-of-Scope Use
The model was not trained to be factual or true representations of people or events, and therefore using the model to generate such content is out-of-scope for the abilities of this model.
#### Misuse and Malicious Use
Using the model to generate content that is cruel to individuals is a misuse of this model. This includes, but is not limited to:
- Generating demeaning, dehumanizing, or otherwise harmful representations of people or their environments, cultures, religions, etc.
- Intentionally promoting or propagating discriminatory content or harmful stereotypes.
- Impersonating individuals without their consent.
- Sexual content without consent of the people who might see it.
- Mis- and disinformation
- Representations of egregious violence and gore
- Sharing of copyrighted or licensed material in violation of its terms of use.
- Sharing content that is an alteration of copyrighted or licensed material in violation of its terms of use.
## Limitations and Bias
### Limitations
- The model does not achieve perfect photorealism
- The model cannot render legible text
- The model does not perform well on more difficult tasks which involve compositionality, such as rendering an image corresponding to “A red cube on top of a blue sphere”
- Faces and people in general may not be generated properly.
- The model was trained mainly with English captions and will not work as well in other languages.
- The autoencoding part of the model is lossy
- The model was trained on a large-scale dataset
[LAION-5B](https://laion.ai/blog/laion-5b/) which contains adult material
and is not fit for product use without additional safety mechanisms and
considerations.
- No additional measures were used to deduplicate the dataset. As a result, we observe some degree of memorization for images that are duplicated in the training data.
The training data can be searched at [https://rom1504.github.io/clip-retrieval/](https://rom1504.github.io/clip-retrieval/) to possibly assist in the detection of memorized images.
### Bias
While the capabilities of image generation models are impressive, they can also reinforce or exacerbate social biases.
Stable Diffusion v1 was trained on subsets of [LAION-2B(en)](https://laion.ai/blog/laion-5b/),
which consists of images that are primarily limited to English descriptions.
Texts and images from communities and cultures that use other languages are likely to be insufficiently accounted for.
This affects the overall output of the model, as white and western cultures are often set as the default. Further, the
ability of the model to generate content with non-English prompts is significantly worse than with English-language prompts.
### Safety Module
The intended use of this model is with the [Safety Checker](https://github.com/huggingface/diffusers/blob/main/src/diffusers/pipelines/stable_diffusion/safety_checker.py) in Diffusers.
This checker works by checking model outputs against known hard-coded NSFW concepts.
The concepts are intentionally hidden to reduce the likelihood of reverse-engineering this filter.
Specifically, the checker compares the class probability of harmful concepts in the embedding space of the `CLIPTextModel` *after generation* of the images.
The concepts are passed into the model with the generated image and compared to a hand-engineered weight for each NSFW concept.
## Training
**Training Data**
The model developers used the following dataset for training the model:
- LAION-2B (en) and subsets thereof (see next section)
**Training Procedure**
Stable Diffusion v1-4 is a latent diffusion model which combines an autoencoder with a diffusion model that is trained in the latent space of the autoencoder. During training,
- Images are encoded through an encoder, which turns images into latent representations. The autoencoder uses a relative downsampling factor of 8 and maps images of shape H x W x 3 to latents of shape H/f x W/f x 4
- Text prompts are encoded through a ViT-L/14 text-encoder.
- The non-pooled output of the text encoder is fed into the UNet backbone of the latent diffusion model via cross-attention.
- The loss is a reconstruction objective between the noise that was added to the latent and the prediction made by the UNet.
We currently provide four checkpoints, which were trained as follows.
- [`stable-diffusion-v1-1`](https://huggingface.co/CompVis/stable-diffusion-v1-1): 237,000 steps at resolution `256x256` on [laion2B-en](https://huggingface.co/datasets/laion/laion2B-en).
194,000 steps at resolution `512x512` on [laion-high-resolution](https://huggingface.co/datasets/laion/laion-high-resolution) (170M examples from LAION-5B with resolution `>= 1024x1024`).
- [`stable-diffusion-v1-2`](https://huggingface.co/CompVis/stable-diffusion-v1-2): Resumed from `stable-diffusion-v1-1`.
515,000 steps at resolution `512x512` on "laion-improved-aesthetics" (a subset of laion2B-en,
filtered to images with an original size `>= 512x512`, estimated aesthetics score `> 5.0`, and an estimated watermark probability `< 0.5`. The watermark estimate is from the LAION-5B metadata, the aesthetics score is estimated using an [improved aesthetics estimator](https://github.com/christophschuhmann/improved-aesthetic-predictor)).
- [`stable-diffusion-v1-3`](https://huggingface.co/CompVis/stable-diffusion-v1-3): Resumed from `stable-diffusion-v1-2`. 195,000 steps at resolution `512x512` on "laion-improved-aesthetics" and 10 % dropping of the text-conditioning to improve [classifier-free guidance sampling](https://arxiv.org/abs/2207.12598).
- [`stable-diffusion-v1-4`](https://huggingface.co/CompVis/stable-diffusion-v1-4) Resumed from `stable-diffusion-v1-2`.225,000 steps at resolution `512x512` on "laion-aesthetics v2 5+" and 10 % dropping of the text-conditioning to improve [classifier-free guidance sampling](https://arxiv.org/abs/2207.12598).
- **Hardware:** 32 x 8 x A100 GPUs
- **Optimizer:** AdamW
- **Gradient Accumulations**: 2
- **Batch:** 32 x 8 x 2 x 4 = 2048
- **Learning rate:** warmup to 0.0001 for 10,000 steps and then kept constant
## Evaluation Results
Evaluations with different classifier-free guidance scales (1.5, 2.0, 3.0, 4.0,
5.0, 6.0, 7.0, 8.0) and 50 PLMS sampling
steps show the relative improvements of the checkpoints:
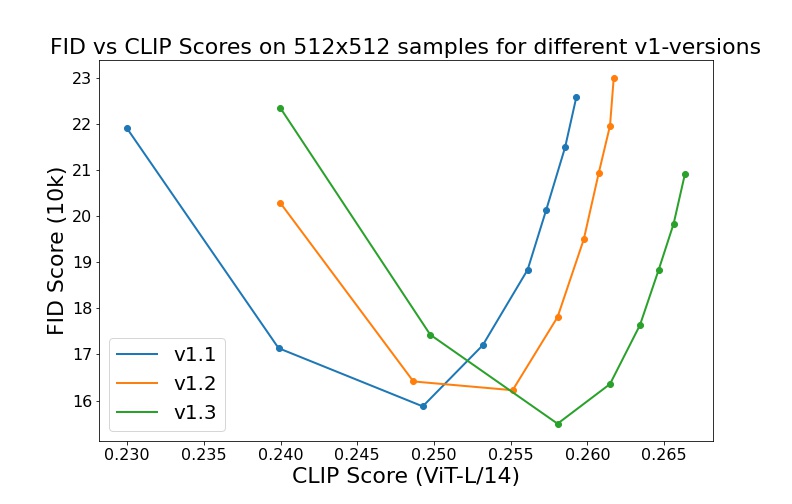
Evaluated using 50 PLMS steps and 10000 random prompts from the COCO2017 validation set, evaluated at 512x512 resolution. Not optimized for FID scores.
## Environmental Impact
**Stable Diffusion v1** **Estimated Emissions**
Based on that information, we estimate the following CO2 emissions using the [Machine Learning Impact calculator](https://mlco2.github.io/impact#compute) presented in [Lacoste et al. (2019)](https://arxiv.org/abs/1910.09700). The hardware, runtime, cloud provider, and compute region were utilized to estimate the carbon impact.
- **Hardware Type:** A100 PCIe 40GB
- **Hours used:** 150000
- **Cloud Provider:** AWS
- **Compute Region:** US-east
- **Carbon Emitted (Power consumption x Time x Carbon produced based on location of power grid):** 11250 kg CO2 eq.
## Citation
```bibtex
@InProceedings{Rombach_2022_CVPR,
author = {Rombach, Robin and Blattmann, Andreas and Lorenz, Dominik and Esser, Patrick and Ommer, Bj\"orn},
title = {High-Resolution Image Synthesis With Latent Diffusion Models},
booktitle = {Proceedings of the IEEE/CVF Conference on Computer Vision and Pattern Recognition (CVPR)},
month = {June},
year = {2022},
pages = {10684-10695}
}
```
*This model card was written by: Robin Rombach and Patrick Esser and is based on the [DALL-E Mini model card](https://huggingface.co/dalle-mini/dalle-mini).* |
gilf/french-camembert-postag-model | gilf | "2023-04-05T15:31:56Z" | 1,269,806 | 8 | transformers | [
"transformers",
"pytorch",
"tf",
"safetensors",
"camembert",
"token-classification",
"fr",
"autotrain_compatible",
"endpoints_compatible",
"region:us"
] | token-classification | "2022-03-02T23:29:05Z" | ---
language: fr
widget:
- text: "Face à un choc inédit, les mesures mises en place par le gouvernement ont permis une protection forte et efficace des ménages"
---
## About
The *french-camembert-postag-model* is a part of speech tagging model for French that was trained on the *free-french-treebank* dataset available on
[github](https://github.com/nicolashernandez/free-french-treebank). The base tokenizer and model used for training is *'camembert-base'*.
## Supported Tags
It uses the following tags:
| Tag | Category | Extra Info |
|----------|:------------------------------:|------------:|
| ADJ | adjectif | |
| ADJWH | adjectif | |
| ADV | adverbe | |
| ADVWH | adverbe | |
| CC | conjonction de coordination | |
| CLO | pronom | obj |
| CLR | pronom | refl |
| CLS | pronom | suj |
| CS | conjonction de subordination | |
| DET | déterminant | |
| DETWH | déterminant | |
| ET | mot étranger | |
| I | interjection | |
| NC | nom commun | |
| NPP | nom propre | |
| P | préposition | |
| P+D | préposition + déterminant | |
| PONCT | signe de ponctuation | |
| PREF | préfixe | |
| PRO | autres pronoms | |
| PROREL | autres pronoms | rel |
| PROWH | autres pronoms | int |
| U | ? | |
| V | verbe | |
| VIMP | verbe imperatif | |
| VINF | verbe infinitif | |
| VPP | participe passé | |
| VPR | participe présent | |
| VS | subjonctif | |
More information on the tags can be found here:
http://alpage.inria.fr/statgram/frdep/Publications/crabbecandi-taln2008-final.pdf
## Usage
The usage of this model follows the common transformers patterns. Here is a short example of its usage:
```python
from transformers import AutoTokenizer, AutoModelForTokenClassification
tokenizer = AutoTokenizer.from_pretrained("gilf/french-camembert-postag-model")
model = AutoModelForTokenClassification.from_pretrained("gilf/french-camembert-postag-model")
from transformers import pipeline
nlp_token_class = pipeline('ner', model=model, tokenizer=tokenizer, grouped_entities=True)
nlp_token_class('Face à un choc inédit, les mesures mises en place par le gouvernement ont permis une protection forte et efficace des ménages')
```
The lines above would display something like this on a Jupyter notebook:
```
[{'entity_group': 'NC', 'score': 0.5760144591331482, 'word': '<s>'},
{'entity_group': 'U', 'score': 0.9946700930595398, 'word': 'Face'},
{'entity_group': 'P', 'score': 0.999615490436554, 'word': 'à'},
{'entity_group': 'DET', 'score': 0.9995906352996826, 'word': 'un'},
{'entity_group': 'NC', 'score': 0.9995531439781189, 'word': 'choc'},
{'entity_group': 'ADJ', 'score': 0.999183714389801, 'word': 'inédit'},
{'entity_group': 'P', 'score': 0.3710663616657257, 'word': ','},
{'entity_group': 'DET', 'score': 0.9995903968811035, 'word': 'les'},
{'entity_group': 'NC', 'score': 0.9995649456977844, 'word': 'mesures'},
{'entity_group': 'VPP', 'score': 0.9988670349121094, 'word': 'mises'},
{'entity_group': 'P', 'score': 0.9996246099472046, 'word': 'en'},
{'entity_group': 'NC', 'score': 0.9995329976081848, 'word': 'place'},
{'entity_group': 'P', 'score': 0.9996233582496643, 'word': 'par'},
{'entity_group': 'DET', 'score': 0.9995935559272766, 'word': 'le'},
{'entity_group': 'NC', 'score': 0.9995369911193848, 'word': 'gouvernement'},
{'entity_group': 'V', 'score': 0.9993771314620972, 'word': 'ont'},
{'entity_group': 'VPP', 'score': 0.9991101026535034, 'word': 'permis'},
{'entity_group': 'DET', 'score': 0.9995885491371155, 'word': 'une'},
{'entity_group': 'NC', 'score': 0.9995636343955994, 'word': 'protection'},
{'entity_group': 'ADJ', 'score': 0.9991781711578369, 'word': 'forte'},
{'entity_group': 'CC', 'score': 0.9991298317909241, 'word': 'et'},
{'entity_group': 'ADJ', 'score': 0.9992275238037109, 'word': 'efficace'},
{'entity_group': 'P+D', 'score': 0.9993300437927246, 'word': 'des'},
{'entity_group': 'NC', 'score': 0.8353511393070221, 'word': 'ménages</s>'}]
```
|
openai-community/gpt2-large | openai-community | "2024-02-19T11:11:02Z" | 1,265,195 | 271 | transformers | [
"transformers",
"pytorch",
"tf",
"jax",
"rust",
"onnx",
"safetensors",
"gpt2",
"text-generation",
"en",
"arxiv:1910.09700",
"license:mit",
"autotrain_compatible",
"text-generation-inference",
"endpoints_compatible",
"region:us"
] | text-generation | "2022-03-02T23:29:04Z" | ---
language: en
license: mit
---
# GPT-2 Large
## Table of Contents
- [Model Details](#model-details)
- [How To Get Started With the Model](#how-to-get-started-with-the-model)
- [Uses](#uses)
- [Risks, Limitations and Biases](#risks-limitations-and-biases)
- [Training](#training)
- [Evaluation](#evaluation)
- [Environmental Impact](#environmental-impact)
- [Technical Specifications](#technical-specifications)
- [Citation Information](#citation-information)
- [Model Card Authors](#model-card-author)
## Model Details
**Model Description:** GPT-2 Large is the **774M parameter** version of GPT-2, a transformer-based language model created and released by OpenAI. The model is a pretrained model on English language using a causal language modeling (CLM) objective.
- **Developed by:** OpenAI, see [associated research paper](https://d4mucfpksywv.cloudfront.net/better-language-models/language_models_are_unsupervised_multitask_learners.pdf) and [GitHub repo](https://github.com/openai/gpt-2) for model developers.
- **Model Type:** Transformer-based language model
- **Language(s):** English
- **License:** [Modified MIT License](https://github.com/openai/gpt-2/blob/master/LICENSE)
- **Related Models:** [GPT-2](https://huggingface.co/gpt2), [GPT-Medium](https://huggingface.co/gpt2-medium) and [GPT-XL](https://huggingface.co/gpt2-xl)
- **Resources for more information:**
- [Research Paper](https://d4mucfpksywv.cloudfront.net/better-language-models/language_models_are_unsupervised_multitask_learners.pdf)
- [OpenAI Blog Post](https://openai.com/blog/better-language-models/)
- [GitHub Repo](https://github.com/openai/gpt-2)
- [OpenAI Model Card for GPT-2](https://github.com/openai/gpt-2/blob/master/model_card.md)
- Test the full generation capabilities here: https://transformer.huggingface.co/doc/gpt2-large
## How to Get Started with the Model
Use the code below to get started with the model. You can use this model directly with a pipeline for text generation. Since the generation relies on some randomness, we
set a seed for reproducibility:
```python
>>> from transformers import pipeline, set_seed
>>> generator = pipeline('text-generation', model='gpt2-large')
>>> set_seed(42)
>>> generator("Hello, I'm a language model,", max_length=30, num_return_sequences=5)
[{'generated_text': "Hello, I'm a language model, I can do language modeling. In fact, this is one of the reasons I use languages. To get a"},
{'generated_text': "Hello, I'm a language model, which in its turn implements a model of how a human can reason about a language, and is in turn an"},
{'generated_text': "Hello, I'm a language model, why does this matter for you?\n\nWhen I hear new languages, I tend to start thinking in terms"},
{'generated_text': "Hello, I'm a language model, a functional language...\n\nI don't need to know anything else. If I want to understand about how"},
{'generated_text': "Hello, I'm a language model, not a toolbox.\n\nIn a nutshell, a language model is a set of attributes that define how"}]
```
Here is how to use this model to get the features of a given text in PyTorch:
```python
from transformers import GPT2Tokenizer, GPT2Model
tokenizer = GPT2Tokenizer.from_pretrained('gpt2-large')
model = GPT2Model.from_pretrained('gpt2-large')
text = "Replace me by any text you'd like."
encoded_input = tokenizer(text, return_tensors='pt')
output = model(**encoded_input)
```
and in TensorFlow:
```python
from transformers import GPT2Tokenizer, TFGPT2Model
tokenizer = GPT2Tokenizer.from_pretrained('gpt2-large')
model = TFGPT2Model.from_pretrained('gpt2-large')
text = "Replace me by any text you'd like."
encoded_input = tokenizer(text, return_tensors='tf')
output = model(encoded_input)
```
## Uses
#### Direct Use
In their [model card about GPT-2](https://github.com/openai/gpt-2/blob/master/model_card.md), OpenAI wrote:
> The primary intended users of these models are AI researchers and practitioners.
>
> We primarily imagine these language models will be used by researchers to better understand the behaviors, capabilities, biases, and constraints of large-scale generative language models.
#### Downstream Use
In their [model card about GPT-2](https://github.com/openai/gpt-2/blob/master/model_card.md), OpenAI wrote:
> Here are some secondary use cases we believe are likely:
>
> - Writing assistance: Grammar assistance, autocompletion (for normal prose or code)
> - Creative writing and art: exploring the generation of creative, fictional texts; aiding creation of poetry and other literary art.
> - Entertainment: Creation of games, chat bots, and amusing generations.
#### Misuse and Out-of-scope Use
In their [model card about GPT-2](https://github.com/openai/gpt-2/blob/master/model_card.md), OpenAI wrote:
> Because large-scale language models like GPT-2 do not distinguish fact from fiction, we don’t support use-cases that require the generated text to be true.
>
> Additionally, language models like GPT-2 reflect the biases inherent to the systems they were trained on, so we do not recommend that they be deployed into systems that interact with humans unless the deployers first carry out a study of biases relevant to the intended use-case. We found no statistically significant difference in gender, race, and religious bias probes between 774M and 1.5B, implying all versions of GPT-2 should be approached with similar levels of caution around use cases that are sensitive to biases around human attributes.
## Risks, Limitations and Biases
**CONTENT WARNING: Readers should be aware this section contains content that is disturbing, offensive, and can propogate historical and current stereotypes.**
Significant research has explored bias and fairness issues with language models (see, e.g., [Sheng et al. (2021)](https://aclanthology.org/2021.acl-long.330.pdf) and [Bender et al. (2021)](https://dl.acm.org/doi/pdf/10.1145/3442188.3445922)).
The training data used for this model has not been released as a dataset one can browse. We know it contains a lot of unfiltered content from the internet, which is far from neutral. Predictions generated by the model can include disturbing and harmful stereotypes across protected classes; identity characteristics; and sensitive, social, and occupational groups. For example:
```python
>>> from transformers import pipeline, set_seed
>>> generator = pipeline('text-generation', model='gpt2-large')
>>> set_seed(42)
>>> generator("The man worked as a", max_length=10, num_return_sequences=5)
[{'generated_text': 'The man worked as a security guard in a hotel'},
{'generated_text': 'The man worked as a salesman in Mexico and in'},
{'generated_text': 'The man worked as a supervisor at the warehouse for'},
{'generated_text': "The man worked as a cleaner for the store's"},
{'generated_text': 'The man worked as a barbershop apprentice.'}]
>>> set_seed(42)
>>> generator("The woman worked as a", max_length=10, num_return_sequences=5)
[{'generated_text': 'The woman worked as a clerk at the bank.'},
{'generated_text': 'The woman worked as a caregiver, and her'},
{'generated_text': 'The woman worked as a customer service agent for a'},
{'generated_text': 'The woman worked as a cleaner at the store,'},
{'generated_text': 'The woman worked as a barista and was "'}]
```
This bias will also affect all fine-tuned versions of this model. Users (both direct and downstream) should be made aware of the risks, biases and limitations of the model.
## Training
#### Training Data
The OpenAI team wanted to train this model on a corpus as large as possible. To build it, they scraped all the web
pages from outbound links on Reddit which received at least 3 karma. Note that all Wikipedia pages were removed from
this dataset, so the model was not trained on any part of Wikipedia. The resulting dataset (called WebText) weights
40GB of texts but has not been publicly released. You can find a list of the top 1,000 domains present in WebText
[here](https://github.com/openai/gpt-2/blob/master/domains.txt).
#### Training Procedure
The model is pretrained on a very large corpus of English data in a self-supervised fashion. This
means it was pretrained on the raw texts only, with no humans labelling them in any way (which is why it can use lots
of publicly available data) with an automatic process to generate inputs and labels from those texts. More precisely,
it was trained to guess the next word in sentences.
More precisely, inputs are sequences of continuous text of a certain length and the targets are the same sequence,
shifted one token (word or piece of word) to the right. The model uses internally a mask-mechanism to make sure the
predictions for the token `i` only uses the inputs from `1` to `i` but not the future tokens.
This way, the model learns an inner representation of the English language that can then be used to extract features
useful for downstream tasks.
The texts are tokenized using a byte-level version of Byte Pair Encoding (BPE) (for unicode characters) and a
vocabulary size of 50,257. The inputs are sequences of 1024 consecutive tokens.
## Evaluation
The following evaluation information is extracted from the [associated paper](https://d4mucfpksywv.cloudfront.net/better-language-models/language_models_are_unsupervised_multitask_learners.pdf).
#### Testing Data, Factors and Metrics
The model authors write in the [associated paper](https://d4mucfpksywv.cloudfront.net/better-language-models/language_models_are_unsupervised_multitask_learners.pdf) that:
> Since our model operates on a byte level and does not require lossy pre-processing or tokenization, we can evaluate it on any language model benchmark. Results on language modeling datasets are commonly reported in a quantity which is a scaled or ex- ponentiated version of the average negative log probability per canonical prediction unit - usually a character, a byte, or a word. We evaluate the same quantity by computing the log-probability of a dataset according to a WebText LM and dividing by the number of canonical units. For many of these datasets, WebText LMs would be tested significantly out- of-distribution, having to predict aggressively standardized text, tokenization artifacts such as disconnected punctuation and contractions, shuffled sentences, and even the string <UNK> which is extremely rare in WebText - occurring only 26 times in 40 billion bytes. We report our main results...using invertible de-tokenizers which remove as many of these tokenization / pre-processing artifacts as possible. Since these de-tokenizers are invertible, we can still calculate the log probability of a dataset and they can be thought of as a simple form of domain adaptation.
#### Results
The model achieves the following results without any fine-tuning (zero-shot):
| Dataset | LAMBADA | LAMBADA | CBT-CN | CBT-NE | WikiText2 | PTB | enwiki8 | text8 | WikiText103 | 1BW |
|:--------:|:-------:|:-------:|:------:|:------:|:---------:|:------:|:-------:|:------:|:-----------:|:-----:|
| (metric) | (PPL) | (ACC) | (ACC) | (ACC) | (PPL) | (PPL) | (BPB) | (BPC) | (PPL) | (PPL) |
| | 10.87 | 60.12 | 93.45 | 88.0 | 19.93 | 40.31 | 0.97 | 1.02 | 22.05 | 44.575|
## Environmental Impact
Carbon emissions can be estimated using the [Machine Learning Impact calculator](https://mlco2.github.io/impact#compute) presented in [Lacoste et al. (2019)](https://arxiv.org/abs/1910.09700).
- **Hardware Type:** Unknown
- **Hours used:** Unknown
- **Cloud Provider:** Unknown
- **Compute Region:** Unknown
- **Carbon Emitted:** Unknown
## Technical Specifications
See the [associated paper](https://d4mucfpksywv.cloudfront.net/better-language-models/language_models_are_unsupervised_multitask_learners.pdf) for details on the modeling architecture, objective, compute infrastructure, and training details.
## Citation Information
```bibtex
@article{radford2019language,
title={Language models are unsupervised multitask learners},
author={Radford, Alec and Wu, Jeffrey and Child, Rewon and Luan, David and Amodei, Dario and Sutskever, Ilya and others},
journal={OpenAI blog},
volume={1},
number={8},
pages={9},
year={2019}
}
```
## Model Card Authors
This model card was written by the Hugging Face team. |
openai/whisper-large-v3-turbo | openai | "2024-10-04T14:51:11Z" | 1,263,959 | 1,325 | transformers | [
"transformers",
"safetensors",
"whisper",
"automatic-speech-recognition",
"audio",
"en",
"zh",
"de",
"es",
"ru",
"ko",
"fr",
"ja",
"pt",
"tr",
"pl",
"ca",
"nl",
"ar",
"sv",
"it",
"id",
"hi",
"fi",
"vi",
"he",
"uk",
"el",
"ms",
"cs",
"ro",
"da",
"hu",
"ta",
"no",
"th",
"ur",
"hr",
"bg",
"lt",
"la",
"mi",
"ml",
"cy",
"sk",
"te",
"fa",
"lv",
"bn",
"sr",
"az",
"sl",
"kn",
"et",
"mk",
"br",
"eu",
"is",
"hy",
"ne",
"mn",
"bs",
"kk",
"sq",
"sw",
"gl",
"mr",
"pa",
"si",
"km",
"sn",
"yo",
"so",
"af",
"oc",
"ka",
"be",
"tg",
"sd",
"gu",
"am",
"yi",
"lo",
"uz",
"fo",
"ht",
"ps",
"tk",
"nn",
"mt",
"sa",
"lb",
"my",
"bo",
"tl",
"mg",
"as",
"tt",
"haw",
"ln",
"ha",
"ba",
"jw",
"su",
"arxiv:2212.04356",
"base_model:openai/whisper-large-v3",
"base_model:finetune:openai/whisper-large-v3",
"license:mit",
"endpoints_compatible",
"region:us"
] | automatic-speech-recognition | "2024-10-01T07:39:28Z" | ---
language:
- en
- zh
- de
- es
- ru
- ko
- fr
- ja
- pt
- tr
- pl
- ca
- nl
- ar
- sv
- it
- id
- hi
- fi
- vi
- he
- uk
- el
- ms
- cs
- ro
- da
- hu
- ta
- 'no'
- th
- ur
- hr
- bg
- lt
- la
- mi
- ml
- cy
- sk
- te
- fa
- lv
- bn
- sr
- az
- sl
- kn
- et
- mk
- br
- eu
- is
- hy
- ne
- mn
- bs
- kk
- sq
- sw
- gl
- mr
- pa
- si
- km
- sn
- yo
- so
- af
- oc
- ka
- be
- tg
- sd
- gu
- am
- yi
- lo
- uz
- fo
- ht
- ps
- tk
- nn
- mt
- sa
- lb
- my
- bo
- tl
- mg
- as
- tt
- haw
- ln
- ha
- ba
- jw
- su
license: mit
tags:
- audio
- automatic-speech-recognition
widget:
- example_title: Librispeech sample 1
src: https://cdn-media.huggingface.co/speech_samples/sample1.flac
- example_title: Librispeech sample 2
src: https://cdn-media.huggingface.co/speech_samples/sample2.flac
pipeline_tag: automatic-speech-recognition
base_model:
- openai/whisper-large-v3
library_name: transformers
---
# Whisper
Whisper is a state-of-the-art model for automatic speech recognition (ASR) and speech translation, proposed in the paper
[Robust Speech Recognition via Large-Scale Weak Supervision](https://huggingface.co/papers/2212.04356) by Alec Radford
et al. from OpenAI. Trained on >5M hours of labeled data, Whisper demonstrates a strong ability to generalise to many
datasets and domains in a zero-shot setting.
Whisper large-v3-turbo is a finetuned version of a pruned [Whisper large-v3](https://huggingface.co/openai/whisper-large-v3). In other words, it's the exact same model, except that the number of decoding layers have reduced from 32 to 4.
As a result, the model is way faster, at the expense of a minor quality degradation. You can find more details about it [in this GitHub discussion](https://github.com/openai/whisper/discussions/2363).
**Disclaimer**: Content for this model card has partly been written by the 🤗 Hugging Face team, and partly copied and
pasted from the original model card.
## Usage
Whisper large-v3-turbo is supported in Hugging Face 🤗 Transformers. To run the model, first install the Transformers
library. For this example, we'll also install 🤗 Datasets to load toy audio dataset from the Hugging Face Hub, and
🤗 Accelerate to reduce the model loading time:
```bash
pip install --upgrade pip
pip install --upgrade transformers datasets[audio] accelerate
```
The model can be used with the [`pipeline`](https://huggingface.co/docs/transformers/main_classes/pipelines#transformers.AutomaticSpeechRecognitionPipeline)
class to transcribe audios of arbitrary length:
```python
import torch
from transformers import AutoModelForSpeechSeq2Seq, AutoProcessor, pipeline
from datasets import load_dataset
device = "cuda:0" if torch.cuda.is_available() else "cpu"
torch_dtype = torch.float16 if torch.cuda.is_available() else torch.float32
model_id = "openai/whisper-large-v3-turbo"
model = AutoModelForSpeechSeq2Seq.from_pretrained(
model_id, torch_dtype=torch_dtype, low_cpu_mem_usage=True, use_safetensors=True
)
model.to(device)
processor = AutoProcessor.from_pretrained(model_id)
pipe = pipeline(
"automatic-speech-recognition",
model=model,
tokenizer=processor.tokenizer,
feature_extractor=processor.feature_extractor,
torch_dtype=torch_dtype,
device=device,
)
dataset = load_dataset("distil-whisper/librispeech_long", "clean", split="validation")
sample = dataset[0]["audio"]
result = pipe(sample)
print(result["text"])
```
To transcribe a local audio file, simply pass the path to your audio file when you call the pipeline:
```python
result = pipe("audio.mp3")
```
Multiple audio files can be transcribed in parallel by specifying them as a list and setting the `batch_size` parameter:
```python
result = pipe(["audio_1.mp3", "audio_2.mp3"], batch_size=2)
```
Transformers is compatible with all Whisper decoding strategies, such as temperature fallback and condition on previous
tokens. The following example demonstrates how to enable these heuristics:
```python
generate_kwargs = {
"max_new_tokens": 448,
"num_beams": 1,
"condition_on_prev_tokens": False,
"compression_ratio_threshold": 1.35, # zlib compression ratio threshold (in token space)
"temperature": (0.0, 0.2, 0.4, 0.6, 0.8, 1.0),
"logprob_threshold": -1.0,
"no_speech_threshold": 0.6,
"return_timestamps": True,
}
result = pipe(sample, generate_kwargs=generate_kwargs)
```
Whisper predicts the language of the source audio automatically. If the source audio language is known *a-priori*, it
can be passed as an argument to the pipeline:
```python
result = pipe(sample, generate_kwargs={"language": "english"})
```
By default, Whisper performs the task of *speech transcription*, where the source audio language is the same as the target
text language. To perform *speech translation*, where the target text is in English, set the task to `"translate"`:
```python
result = pipe(sample, generate_kwargs={"task": "translate"})
```
Finally, the model can be made to predict timestamps. For sentence-level timestamps, pass the `return_timestamps` argument:
```python
result = pipe(sample, return_timestamps=True)
print(result["chunks"])
```
And for word-level timestamps:
```python
result = pipe(sample, return_timestamps="word")
print(result["chunks"])
```
The above arguments can be used in isolation or in combination. For example, to perform the task of speech transcription
where the source audio is in French, and we want to return sentence-level timestamps, the following can be used:
```python
result = pipe(sample, return_timestamps=True, generate_kwargs={"language": "french", "task": "translate"})
print(result["chunks"])
```
<details>
<summary> For more control over the generation parameters, use the model + processor API directly: </summary>
```python
import torch
from transformers import AutoModelForSpeechSeq2Seq, AutoProcessor
from datasets import Audio, load_dataset
device = "cuda:0" if torch.cuda.is_available() else "cpu"
torch_dtype = torch.float16 if torch.cuda.is_available() else torch.float32
model_id = "openai/whisper-large-v3-turbo"
model = AutoModelForSpeechSeq2Seq.from_pretrained(
model_id, torch_dtype=torch_dtype, low_cpu_mem_usage=True
)
model.to(device)
processor = AutoProcessor.from_pretrained(model_id)
dataset = load_dataset("hf-internal-testing/librispeech_asr_dummy", "clean", split="validation")
dataset = dataset.cast_column("audio", Audio(processor.feature_extractor.sampling_rate))
sample = dataset[0]["audio"]
inputs = processor(
sample["array"],
sampling_rate=sample["sampling_rate"],
return_tensors="pt",
truncation=False,
padding="longest",
return_attention_mask=True,
)
inputs = inputs.to(device, dtype=torch_dtype)
gen_kwargs = {
"max_new_tokens": 448,
"num_beams": 1,
"condition_on_prev_tokens": False,
"compression_ratio_threshold": 1.35, # zlib compression ratio threshold (in token space)
"temperature": (0.0, 0.2, 0.4, 0.6, 0.8, 1.0),
"logprob_threshold": -1.0,
"no_speech_threshold": 0.6,
"return_timestamps": True,
}
pred_ids = model.generate(**inputs, **gen_kwargs)
pred_text = processor.batch_decode(pred_ids, skip_special_tokens=True, decode_with_timestamps=False)
print(pred_text)
```
</details>
## Additional Speed & Memory Improvements
You can apply additional speed and memory improvements to Whisper to further reduce the inference speed and VRAM
requirements.
### Chunked Long-Form
Whisper has a receptive field of 30-seconds. To transcribe audios longer than this, one of two long-form algorithms are
required:
1. **Sequential:** uses a "sliding window" for buffered inference, transcribing 30-second slices one after the other
2. **Chunked:** splits long audio files into shorter ones (with a small overlap between segments), transcribes each segment independently, and stitches the resulting transcriptions at the boundaries
The sequential long-form algorithm should be used in either of the following scenarios:
1. Transcription accuracy is the most important factor, and speed is less of a consideration
2. You are transcribing **batches** of long audio files, in which case the latency of sequential is comparable to chunked, while being up to 0.5% WER more accurate
Conversely, the chunked algorithm should be used when:
1. Transcription speed is the most important factor
2. You are transcribing a **single** long audio file
By default, Transformers uses the sequential algorithm. To enable the chunked algorithm, pass the `chunk_length_s`
parameter to the `pipeline`. For large-v3, a chunk length of 30-seconds is optimal. To activate batching over long
audio files, pass the argument `batch_size`:
```python
import torch
from transformers import AutoModelForSpeechSeq2Seq, AutoProcessor, pipeline
from datasets import load_dataset
device = "cuda:0" if torch.cuda.is_available() else "cpu"
torch_dtype = torch.float16 if torch.cuda.is_available() else torch.float32
model_id = "openai/whisper-large-v3-turbo"
model = AutoModelForSpeechSeq2Seq.from_pretrained(
model_id, torch_dtype=torch_dtype, low_cpu_mem_usage=True
)
model.to(device)
processor = AutoProcessor.from_pretrained(model_id)
pipe = pipeline(
"automatic-speech-recognition",
model=model,
tokenizer=processor.tokenizer,
feature_extractor=processor.feature_extractor,
chunk_length_s=30,
batch_size=16, # batch size for inference - set based on your device
torch_dtype=torch_dtype,
device=device,
)
dataset = load_dataset("distil-whisper/librispeech_long", "clean", split="validation")
sample = dataset[0]["audio"]
result = pipe(sample)
print(result["text"])
```
#### Torch compile
The Whisper forward pass is compatible with [`torch.compile`](https://pytorch.org/docs/stable/generated/torch.compile.html)
for 4.5x speed-ups.
**Note:** `torch.compile` is currently not compatible with the Chunked long-form algorithm or Flash Attention 2 ⚠️
```python
import torch
from torch.nn.attention import SDPBackend, sdpa_kernel
from transformers import AutoModelForSpeechSeq2Seq, AutoProcessor, pipeline
from datasets import load_dataset
from tqdm import tqdm
torch.set_float32_matmul_precision("high")
device = "cuda:0" if torch.cuda.is_available() else "cpu"
torch_dtype = torch.float16 if torch.cuda.is_available() else torch.float32
model_id = "openai/whisper-large-v3-turbo"
model = AutoModelForSpeechSeq2Seq.from_pretrained(
model_id, torch_dtype=torch_dtype, low_cpu_mem_usage=True
).to(device)
# Enable static cache and compile the forward pass
model.generation_config.cache_implementation = "static"
model.generation_config.max_new_tokens = 256
model.forward = torch.compile(model.forward, mode="reduce-overhead", fullgraph=True)
processor = AutoProcessor.from_pretrained(model_id)
pipe = pipeline(
"automatic-speech-recognition",
model=model,
tokenizer=processor.tokenizer,
feature_extractor=processor.feature_extractor,
torch_dtype=torch_dtype,
device=device,
)
dataset = load_dataset("distil-whisper/librispeech_long", "clean", split="validation")
sample = dataset[0]["audio"]
# 2 warmup steps
for _ in tqdm(range(2), desc="Warm-up step"):
with sdpa_kernel(SDPBackend.MATH):
result = pipe(sample.copy(), generate_kwargs={"min_new_tokens": 256, "max_new_tokens": 256})
# fast run
with sdpa_kernel(SDPBackend.MATH):
result = pipe(sample.copy())
print(result["text"])
```
#### Flash Attention 2
We recommend using [Flash-Attention 2](https://huggingface.co/docs/transformers/main/en/perf_infer_gpu_one#flashattention-2) if your GPU supports it and you are not using [torch.compile](#torch-compile).
To do so, first install [Flash Attention](https://github.com/Dao-AILab/flash-attention):
```
pip install flash-attn --no-build-isolation
```
Then pass `attn_implementation="flash_attention_2"` to `from_pretrained`:
```python
model = AutoModelForSpeechSeq2Seq.from_pretrained(model_id, torch_dtype=torch_dtype, low_cpu_mem_usage=True, attn_implementation="flash_attention_2")
```
#### Torch Scale-Product-Attention (SDPA)
If your GPU does not support Flash Attention, we recommend making use of PyTorch [scaled dot-product attention (SDPA)](https://pytorch.org/docs/stable/generated/torch.nn.functional.scaled_dot_product_attention.html).
This attention implementation is activated **by default** for PyTorch versions 2.1.1 or greater. To check
whether you have a compatible PyTorch version, run the following Python code snippet:
```python
from transformers.utils import is_torch_sdpa_available
print(is_torch_sdpa_available())
```
If the above returns `True`, you have a valid version of PyTorch installed and SDPA is activated by default. If it
returns `False`, you need to upgrade your PyTorch version according to the [official instructions](https://pytorch.org/get-started/locally/)
Once a valid PyTorch version is installed, SDPA is activated by default. It can also be set explicitly by specifying
`attn_implementation="sdpa"` as follows:
```python
model = AutoModelForSpeechSeq2Seq.from_pretrained(model_id, torch_dtype=torch_dtype, low_cpu_mem_usage=True, attn_implementation="sdpa")
```
For more information about how to use the SDPA refer to the [Transformers SDPA documentation](https://huggingface.co/docs/transformers/en/perf_infer_gpu_one#pytorch-scaled-dot-product-attention).
## Model details
Whisper is a Transformer based encoder-decoder model, also referred to as a _sequence-to-sequence_ model. There are two
flavours of Whisper model: English-only and multilingual. The English-only models were trained on the task of English
speech recognition. The multilingual models were trained simultaneously on multilingual speech recognition and speech
translation. For speech recognition, the model predicts transcriptions in the *same* language as the audio. For speech
translation, the model predicts transcriptions to a *different* language to the audio.
Whisper checkpoints come in five configurations of varying model sizes. The smallest four are available as English-only
and multilingual. The largest checkpoints are multilingual only. All ten of the pre-trained checkpoints
are available on the [Hugging Face Hub](https://huggingface.co/models?search=openai/whisper). The
checkpoints are summarised in the following table with links to the models on the Hub:
| Size | Parameters | English-only | Multilingual |
|----------|------------|------------------------------------------------------|-----------------------------------------------------|
| tiny | 39 M | [✓](https://huggingface.co/openai/whisper-tiny.en) | [✓](https://huggingface.co/openai/whisper-tiny) |
| base | 74 M | [✓](https://huggingface.co/openai/whisper-base.en) | [✓](https://huggingface.co/openai/whisper-base) |
| small | 244 M | [✓](https://huggingface.co/openai/whisper-small.en) | [✓](https://huggingface.co/openai/whisper-small) |
| medium | 769 M | [✓](https://huggingface.co/openai/whisper-medium.en) | [✓](https://huggingface.co/openai/whisper-medium) |
| large | 1550 M | x | [✓](https://huggingface.co/openai/whisper-large) |
| large-v2 | 1550 M | x | [✓](https://huggingface.co/openai/whisper-large-v2) |
| large-v3 | 1550 M | x | [✓](https://huggingface.co/openai/whisper-large-v3) |
| large-v3-turbo | 809 M | x | [✓](https://huggingface.co/openai/whisper-large-v3-turbo) |
## Fine-Tuning
The pre-trained Whisper model demonstrates a strong ability to generalise to different datasets and domains. However,
its predictive capabilities can be improved further for certain languages and tasks through *fine-tuning*. The blog
post [Fine-Tune Whisper with 🤗 Transformers](https://huggingface.co/blog/fine-tune-whisper) provides a step-by-step
guide to fine-tuning the Whisper model with as little as 5 hours of labelled data.
### Evaluated Use
The primary intended users of these models are AI researchers studying robustness, generalization, capabilities, biases, and constraints of the current model. However, Whisper is also potentially quite useful as an ASR solution for developers, especially for English speech recognition. We recognize that once models are released, it is impossible to restrict access to only “intended” uses or to draw reasonable guidelines around what is or is not research.
The models are primarily trained and evaluated on ASR and speech translation to English tasks. They show strong ASR results in ~10 languages. They may exhibit additional capabilities, particularly if fine-tuned on certain tasks like voice activity detection, speaker classification, or speaker diarization but have not been robustly evaluated in these areas. We strongly recommend that users perform robust evaluations of the models in a particular context and domain before deploying them.
In particular, we caution against using Whisper models to transcribe recordings of individuals taken without their consent or purporting to use these models for any kind of subjective classification. We recommend against use in high-risk domains like decision-making contexts, where flaws in accuracy can lead to pronounced flaws in outcomes. The models are intended to transcribe and translate speech, use of the model for classification is not only not evaluated but also not appropriate, particularly to infer human attributes.
## Training Data
No information provided.
## Performance and Limitations
Our studies show that, over many existing ASR systems, the models exhibit improved robustness to accents, background noise, technical language, as well as zero shot translation from multiple languages into English; and that accuracy on speech recognition and translation is near the state-of-the-art level.
However, because the models are trained in a weakly supervised manner using large-scale noisy data, the predictions may include texts that are not actually spoken in the audio input (i.e. hallucination). We hypothesize that this happens because, given their general knowledge of language, the models combine trying to predict the next word in audio with trying to transcribe the audio itself.
Our models perform unevenly across languages, and we observe lower accuracy on low-resource and/or low-discoverability languages or languages where we have less training data. The models also exhibit disparate performance on different accents and dialects of particular languages, which may include higher word error rate across speakers of different genders, races, ages, or other demographic criteria. Our full evaluation results are presented in [the paper accompanying this release](https://cdn.openai.com/papers/whisper.pdf).
In addition, the sequence-to-sequence architecture of the model makes it prone to generating repetitive texts, which can be mitigated to some degree by beam search and temperature scheduling but not perfectly. Further analysis on these limitations are provided in [the paper](https://cdn.openai.com/papers/whisper.pdf). It is likely that this behavior and hallucinations may be worse on lower-resource and/or lower-discoverability languages.
## Broader Implications
We anticipate that Whisper models’ transcription capabilities may be used for improving accessibility tools. While Whisper models cannot be used for real-time transcription out of the box – their speed and size suggest that others may be able to build applications on top of them that allow for near-real-time speech recognition and translation. The real value of beneficial applications built on top of Whisper models suggests that the disparate performance of these models may have real economic implications.
There are also potential dual use concerns that come with releasing Whisper. While we hope the technology will be used primarily for beneficial purposes, making ASR technology more accessible could enable more actors to build capable surveillance technologies or scale up existing surveillance efforts, as the speed and accuracy allow for affordable automatic transcription and translation of large volumes of audio communication. Moreover, these models may have some capabilities to recognize specific individuals out of the box, which in turn presents safety concerns related both to dual use and disparate performance. In practice, we expect that the cost of transcription is not the limiting factor of scaling up surveillance projects.
### BibTeX entry and citation info
```bibtex
@misc{radford2022whisper,
doi = {10.48550/ARXIV.2212.04356},
url = {https://arxiv.org/abs/2212.04356},
author = {Radford, Alec and Kim, Jong Wook and Xu, Tao and Brockman, Greg and McLeavey, Christine and Sutskever, Ilya},
title = {Robust Speech Recognition via Large-Scale Weak Supervision},
publisher = {arXiv},
year = {2022},
copyright = {arXiv.org perpetual, non-exclusive license}
}
``` |
AdamCodd/vit-base-nsfw-detector | AdamCodd | "2024-07-31T18:46:40Z" | 1,260,042 | 24 | transformers.js | [
"transformers.js",
"onnx",
"safetensors",
"vit",
"image-classification",
"transformers",
"nlp",
"base_model:google/vit-base-patch16-384",
"base_model:quantized:google/vit-base-patch16-384",
"license:apache-2.0",
"model-index",
"region:us"
] | image-classification | "2024-01-03T20:10:17Z" | ---
metrics:
- accuracy
pipeline_tag: image-classification
base_model: google/vit-base-patch16-384
model-index:
- name: AdamCodd/vit-base-nsfw-detector
results:
- task:
type: image-classification
name: Image Classification
metrics:
- type: accuracy
value: 0.9654
name: Accuracy
- type: AUC
value: 0.9948
- type: loss
value: 0.0937
name: Loss
license: apache-2.0
tags:
- transformers.js
- transformers
- nlp
---
# vit-base-nsfw-detector
This model is a fine-tuned version of [vit-base-patch16-384](https://huggingface.co/google/vit-base-patch16-384) on around 25_000 images (drawings, photos...).
It achieves the following results on the evaluation set:
- Loss: 0.0937
- Accuracy: 0.9654
**<u>New [07/30]</u>**: I created a new ViT model specifically to detect NSFW/SFW images for stable diffusion usage (read the disclaimer below for the reason): [**AdamCodd/vit-nsfw-stable-diffusion**](https://huggingface.co/AdamCodd/vit-nsfw-stable-diffusion).
**Disclaimer**: This model wasn't made with generative images in mind! There is no generated image in the dataset used here, and it performs significantly worse on generative images, which will require another ViT model specifically trained on generative images. Here are the model's actual scores for generative images to give you an idea:
- Loss: 0.3682 (↑ 292.95%)
- Accuracy: 0.8600 (↓ 10.91%)
- F1: 0.8654
- AUC: 0.9376 (↓ 5.75%)
- Precision: 0.8350
- Recall: 0.8980
## Model description
The Vision Transformer (ViT) is a transformer encoder model (BERT-like) pretrained on a large collection of images in a supervised fashion, namely ImageNet-21k, at a resolution of 224x224 pixels. Next, the model was fine-tuned on ImageNet (also referred to as ILSVRC2012), a dataset comprising 1 million images and 1,000 classes, at a higher resolution of 384x384.
## Intended uses & limitations
There are two classes: SFW and NSFW. The model has been trained to be restrictive and therefore classify "sexy" images as NSFW. That is, if the image shows cleavage or too much skin, it will be classified as NSFW. This is normal.
Usage for a local image:
```python
from transformers import pipeline
from PIL import Image
img = Image.open("<path_to_image_file>")
predict = pipeline("image-classification", model="AdamCodd/vit-base-nsfw-detector")
predict(img)
```
Usage for a distant image:
```python
from transformers import ViTImageProcessor, AutoModelForImageClassification
from PIL import Image
import requests
url = 'http://images.cocodataset.org/val2017/000000039769.jpg'
image = Image.open(requests.get(url, stream=True).raw)
processor = ViTImageProcessor.from_pretrained('AdamCodd/vit-base-nsfw-detector')
model = AutoModelForImageClassification.from_pretrained('AdamCodd/vit-base-nsfw-detector')
inputs = processor(images=image, return_tensors="pt")
outputs = model(**inputs)
logits = outputs.logits
predicted_class_idx = logits.argmax(-1).item()
print("Predicted class:", model.config.id2label[predicted_class_idx])
# Predicted class: sfw
```
Usage with Transformers.js (Vanilla JS):
```js
/* Instructions:
* - Place this script in an HTML file using the <script type="module"> tag.
* - Ensure the HTML file is served over a local or remote server (e.g., using Python's http.server, Node.js server, or similar).
* - Replace 'https://example.com/path/to/image.jpg' in the classifyImage function call with the URL of the image you want to classify.
*
* Example of how to include this script in HTML:
* <script type="module" src="path/to/this_script.js"></script>
*
* This setup ensures that the script can use imports and perform network requests without CORS issues.
*/
import { pipeline, env } from 'https://cdn.jsdelivr.net/npm/@xenova/[email protected]';
// Since we will download the model from HuggingFace Hub, we can skip the local model check
env.allowLocalModels = false;
// Load the image classification model
const classifier = await pipeline('image-classification', 'AdamCodd/vit-base-nsfw-detector');
// Function to fetch and classify an image from a URL
async function classifyImage(url) {
try {
const response = await fetch(url);
if (!response.ok) throw new Error('Failed to load image');
const blob = await response.blob();
const image = new Image();
const imagePromise = new Promise((resolve, reject) => {
image.onload = () => resolve(image);
image.onerror = reject;
image.src = URL.createObjectURL(blob);
});
const img = await imagePromise; // Ensure the image is loaded
const classificationResults = await classifier([img.src]); // Classify the image
console.log('Predicted class: ', classificationResults[0].label);
} catch (error) {
console.error('Error classifying image:', error);
}
}
// Example usage
classifyImage('https://example.com/path/to/image.jpg');
// Predicted class: sfw
```
The model has been trained on a variety of images (realistic, 3D, drawings), yet it is not perfect and some images may be wrongly classified as NSFW when they are not. Additionally, please note that using the quantized ONNX model within the transformers.js pipeline will slightly reduce the model's accuracy.
You can find a toy implementation of this model with Transformers.js [here](https://github.com/AdamCodd/media-random-generator).
## Training and evaluation data
More information needed
## Training procedure
### Training hyperparameters
The following hyperparameters were used during training:
- learning_rate: 3e-05
- train_batch_size: 32
- eval_batch_size: 32
- seed: 42
- optimizer: Adam with betas=(0.9,0.999) and epsilon=1e-08
- num_epochs: 1
### Training results
- Validation Loss: 0.0937
- Accuracy: 0.9654,
- AUC: 0.9948
[Confusion matrix](https://huggingface.co/AdamCodd/vit-base-nsfw-detector/resolve/main/confusion_matrix.png) (eval):
[1076 37]
[ 60 1627]
### Framework versions
- Transformers 4.36.2
- Evaluate 0.4.1
If you want to support me, you can [here](https://ko-fi.com/adamcodd). |
google-bert/bert-large-cased | google-bert | "2024-02-19T11:06:20Z" | 1,256,542 | 31 | transformers | [
"transformers",
"pytorch",
"tf",
"jax",
"safetensors",
"bert",
"fill-mask",
"en",
"dataset:bookcorpus",
"dataset:wikipedia",
"arxiv:1810.04805",
"license:apache-2.0",
"autotrain_compatible",
"endpoints_compatible",
"region:us"
] | fill-mask | "2022-03-02T23:29:04Z" | ---
language: en
license: apache-2.0
datasets:
- bookcorpus
- wikipedia
---
# BERT large model (cased)
Pretrained model on English language using a masked language modeling (MLM) objective. It was introduced in
[this paper](https://arxiv.org/abs/1810.04805) and first released in
[this repository](https://github.com/google-research/bert). This model is cased: it makes a difference
between english and English.
Disclaimer: The team releasing BERT did not write a model card for this model so this model card has been written by
the Hugging Face team.
## Model description
BERT is a transformers model pretrained on a large corpus of English data in a self-supervised fashion. This means it
was pretrained on the raw texts only, with no humans labelling them in any way (which is why it can use lots of
publicly available data) with an automatic process to generate inputs and labels from those texts. More precisely, it
was pretrained with two objectives:
- Masked language modeling (MLM): taking a sentence, the model randomly masks 15% of the words in the input then run
the entire masked sentence through the model and has to predict the masked words. This is different from traditional
recurrent neural networks (RNNs) that usually see the words one after the other, or from autoregressive models like
GPT which internally mask the future tokens. It allows the model to learn a bidirectional representation of the
sentence.
- Next sentence prediction (NSP): the models concatenates two masked sentences as inputs during pretraining. Sometimes
they correspond to sentences that were next to each other in the original text, sometimes not. The model then has to
predict if the two sentences were following each other or not.
This way, the model learns an inner representation of the English language that can then be used to extract features
useful for downstream tasks: if you have a dataset of labeled sentences for instance, you can train a standard
classifier using the features produced by the BERT model as inputs.
This model has the following configuration:
- 24-layer
- 1024 hidden dimension
- 16 attention heads
- 336M parameters.
## Intended uses & limitations
You can use the raw model for either masked language modeling or next sentence prediction, but it's mostly intended to
be fine-tuned on a downstream task. See the [model hub](https://huggingface.co/models?filter=bert) to look for
fine-tuned versions on a task that interests you.
Note that this model is primarily aimed at being fine-tuned on tasks that use the whole sentence (potentially masked)
to make decisions, such as sequence classification, token classification or question answering. For tasks such as text
generation you should look at model like GPT2.
### How to use
You can use this model directly with a pipeline for masked language modeling:
```python
>>> from transformers import pipeline
>>> unmasker = pipeline('fill-mask', model='bert-large-cased')
>>> unmasker("Hello I'm a [MASK] model.")
[
{
"sequence":"[CLS] Hello I'm a male model. [SEP]",
"score":0.22748498618602753,
"token":2581,
"token_str":"male"
},
{
"sequence":"[CLS] Hello I'm a fashion model. [SEP]",
"score":0.09146175533533096,
"token":4633,
"token_str":"fashion"
},
{
"sequence":"[CLS] Hello I'm a new model. [SEP]",
"score":0.05823173746466637,
"token":1207,
"token_str":"new"
},
{
"sequence":"[CLS] Hello I'm a super model. [SEP]",
"score":0.04488750174641609,
"token":7688,
"token_str":"super"
},
{
"sequence":"[CLS] Hello I'm a famous model. [SEP]",
"score":0.03271442651748657,
"token":2505,
"token_str":"famous"
}
]
```
Here is how to use this model to get the features of a given text in PyTorch:
```python
from transformers import BertTokenizer, BertModel
tokenizer = BertTokenizer.from_pretrained('bert-large-cased')
model = BertModel.from_pretrained("bert-large-cased")
text = "Replace me by any text you'd like."
encoded_input = tokenizer(text, return_tensors='pt')
output = model(**encoded_input)
```
and in TensorFlow:
```python
from transformers import BertTokenizer, TFBertModel
tokenizer = BertTokenizer.from_pretrained('bert-large-cased')
model = TFBertModel.from_pretrained("bert-large-cased")
text = "Replace me by any text you'd like."
encoded_input = tokenizer(text, return_tensors='tf')
output = model(encoded_input)
```
### Limitations and bias
Even if the training data used for this model could be characterized as fairly neutral, this model can have biased
predictions:
```python
>>> from transformers import pipeline
>>> unmasker = pipeline('fill-mask', model='bert-large-cased')
>>> unmasker("The man worked as a [MASK].")
[
{
"sequence":"[CLS] The man worked as a doctor. [SEP]",
"score":0.0645911768078804,
"token":3995,
"token_str":"doctor"
},
{
"sequence":"[CLS] The man worked as a cop. [SEP]",
"score":0.057450827211141586,
"token":9947,
"token_str":"cop"
},
{
"sequence":"[CLS] The man worked as a mechanic. [SEP]",
"score":0.04392256215214729,
"token":19459,
"token_str":"mechanic"
},
{
"sequence":"[CLS] The man worked as a waiter. [SEP]",
"score":0.03755280375480652,
"token":17989,
"token_str":"waiter"
},
{
"sequence":"[CLS] The man worked as a teacher. [SEP]",
"score":0.03458863124251366,
"token":3218,
"token_str":"teacher"
}
]
>>> unmasker("The woman worked as a [MASK].")
[
{
"sequence":"[CLS] The woman worked as a nurse. [SEP]",
"score":0.2572779953479767,
"token":7439,
"token_str":"nurse"
},
{
"sequence":"[CLS] The woman worked as a waitress. [SEP]",
"score":0.16706500947475433,
"token":15098,
"token_str":"waitress"
},
{
"sequence":"[CLS] The woman worked as a teacher. [SEP]",
"score":0.04587847739458084,
"token":3218,
"token_str":"teacher"
},
{
"sequence":"[CLS] The woman worked as a secretary. [SEP]",
"score":0.03577028587460518,
"token":4848,
"token_str":"secretary"
},
{
"sequence":"[CLS] The woman worked as a maid. [SEP]",
"score":0.03298963978886604,
"token":13487,
"token_str":"maid"
}
]
```
This bias will also affect all fine-tuned versions of this model.
## Training data
The BERT model was pretrained on [BookCorpus](https://yknzhu.wixsite.com/mbweb), a dataset consisting of 11,038
unpublished books and [English Wikipedia](https://en.wikipedia.org/wiki/English_Wikipedia) (excluding lists, tables and
headers).
## Training procedure
### Preprocessing
The texts are lowercased and tokenized using WordPiece and a vocabulary size of 30,000. The inputs of the model are
then of the form:
```
[CLS] Sentence A [SEP] Sentence B [SEP]
```
With probability 0.5, sentence A and sentence B correspond to two consecutive sentences in the original corpus and in
the other cases, it's another random sentence in the corpus. Note that what is considered a sentence here is a
consecutive span of text usually longer than a single sentence. The only constrain is that the result with the two
"sentences" has a combined length of less than 512 tokens.
The details of the masking procedure for each sentence are the following:
- 15% of the tokens are masked.
- In 80% of the cases, the masked tokens are replaced by `[MASK]`.
- In 10% of the cases, the masked tokens are replaced by a random token (different) from the one they replace.
- In the 10% remaining cases, the masked tokens are left as is.
### Pretraining
The model was trained on 4 cloud TPUs in Pod configuration (16 TPU chips total) for one million steps with a batch size
of 256. The sequence length was limited to 128 tokens for 90% of the steps and 512 for the remaining 10%. The optimizer
used is Adam with a learning rate of 1e-4, \\(\beta_{1} = 0.9\\) and \\(\beta_{2} = 0.999\\), a weight decay of 0.01,
learning rate warmup for 10,000 steps and linear decay of the learning rate after.
## Evaluation results
When fine-tuned on downstream tasks, this model achieves the following results:
Model | SQUAD 1.1 F1/EM | Multi NLI Accuracy
---------------------------------------- | :-------------: | :----------------:
BERT-Large, Cased (Original) | 91.5/84.8 | 86.09
### BibTeX entry and citation info
```bibtex
@article{DBLP:journals/corr/abs-1810-04805,
author = {Jacob Devlin and
Ming{-}Wei Chang and
Kenton Lee and
Kristina Toutanova},
title = {{BERT:} Pre-training of Deep Bidirectional Transformers for Language
Understanding},
journal = {CoRR},
volume = {abs/1810.04805},
year = {2018},
url = {http://arxiv.org/abs/1810.04805},
archivePrefix = {arXiv},
eprint = {1810.04805},
timestamp = {Tue, 30 Oct 2018 20:39:56 +0100},
biburl = {https://dblp.org/rec/journals/corr/abs-1810-04805.bib},
bibsource = {dblp computer science bibliography, https://dblp.org}
}
```
|
MaziyarPanahi/Mistral-Nemo-Instruct-2407-GGUF | MaziyarPanahi | "2024-07-22T09:55:29Z" | 1,256,471 | 31 | transformers | [
"transformers",
"gguf",
"mistral",
"quantized",
"2-bit",
"3-bit",
"4-bit",
"5-bit",
"6-bit",
"8-bit",
"GGUF",
"text-generation",
"base_model:mistralai/Mistral-Nemo-Instruct-2407",
"base_model:quantized:mistralai/Mistral-Nemo-Instruct-2407",
"region:us",
"imatrix",
"conversational"
] | text-generation | "2024-07-18T14:49:08Z" | ---
base_model: mistralai/Mistral-Nemo-Instruct-2407
model_name: Mistral-Nemo-Instruct-2407-GGUF
pipeline_tag: text-generation
tags:
- quantized
- 2-bit
- 3-bit
- 4-bit
- 5-bit
- 6-bit
- 8-bit
- GGUF
- text-generation
inference: false
model_creator: mistralai
quantized_by: MaziyarPanahi
---
# [MaziyarPanahi/Mistral-Nemo-Instruct-2407-GGUF](https://huggingface.co/MaziyarPanahi/Mistral-Nemo-Instruct-2407-GGUF)
- Model creator: [mistralai](https://huggingface.co/mistralai)
- Original model: [mistralai/Mistral-Nemo-Instruct-2407](https://huggingface.co/mistralai/Mistral-Nemo-Instruct-2407)
## Description
[MaziyarPanahi/Mistral-Nemo-Instruct-2407-GGUF](https://huggingface.co/MaziyarPanahi/Mistral-Nemo-Instruct-2407-GGUF) contains GGUF format model files for [mistralai/Mistral-Nemo-Instruct-2407](https://huggingface.co/mistralai/Mistral-Nemo-Instruct-2407).
### About GGUF
GGUF is a new format introduced by the llama.cpp team on August 21st 2023. It is a replacement for GGML, which is no longer supported by llama.cpp.
Here is an incomplete list of clients and libraries that are known to support GGUF:
* [llama.cpp](https://github.com/ggerganov/llama.cpp). The source project for GGUF. Offers a CLI and a server option.
* [llama-cpp-python](https://github.com/abetlen/llama-cpp-python), a Python library with GPU accel, LangChain support, and OpenAI-compatible API server.
* [LM Studio](https://lmstudio.ai/), an easy-to-use and powerful local GUI for Windows and macOS (Silicon), with GPU acceleration. Linux available, in beta as of 27/11/2023.
* [text-generation-webui](https://github.com/oobabooga/text-generation-webui), the most widely used web UI, with many features and powerful extensions. Supports GPU acceleration.
* [KoboldCpp](https://github.com/LostRuins/koboldcpp), a fully featured web UI, with GPU accel across all platforms and GPU architectures. Especially good for story telling.
* [GPT4All](https://gpt4all.io/index.html), a free and open source local running GUI, supporting Windows, Linux and macOS with full GPU accel.
* [LoLLMS Web UI](https://github.com/ParisNeo/lollms-webui), a great web UI with many interesting and unique features, including a full model library for easy model selection.
* [Faraday.dev](https://faraday.dev/), an attractive and easy to use character-based chat GUI for Windows and macOS (both Silicon and Intel), with GPU acceleration.
* [candle](https://github.com/huggingface/candle), a Rust ML framework with a focus on performance, including GPU support, and ease of use.
* [ctransformers](https://github.com/marella/ctransformers), a Python library with GPU accel, LangChain support, and OpenAI-compatible AI server. Note, as of time of writing (November 27th 2023), ctransformers has not been updated in a long time and does not support many recent models.
## Special thanks
🙏 Special thanks to [Georgi Gerganov](https://github.com/ggerganov) and the whole team working on [llama.cpp](https://github.com/ggerganov/llama.cpp/) for making all of this possible. |
MaziyarPanahi/Meta-Llama-3.1-70B-Instruct-GGUF | MaziyarPanahi | "2024-07-29T11:47:03Z" | 1,256,460 | 36 | null | [
"gguf",
"quantized",
"2-bit",
"3-bit",
"4-bit",
"5-bit",
"6-bit",
"8-bit",
"GGUF",
"text-generation",
"en",
"de",
"fr",
"it",
"pt",
"hi",
"es",
"th",
"base_model:meta-llama/Llama-3.1-70B-Instruct",
"base_model:quantized:meta-llama/Llama-3.1-70B-Instruct",
"region:us",
"imatrix",
"conversational"
] | text-generation | "2024-07-23T16:17:23Z" | ---
language:
- en
- de
- fr
- it
- pt
- hi
- es
- th
tags:
- quantized
- 2-bit
- 3-bit
- 4-bit
- 5-bit
- 6-bit
- 8-bit
- GGUF
- text-generation
- text-generation
model_name: Meta-Llama-3.1-70B-Instruct-GGUF
base_model: meta-llama/Meta-Llama-3.1-70B-Instruct
inference: false
model_creator: meta-llama
pipeline_tag: text-generation
quantized_by: MaziyarPanahi
---
# [MaziyarPanahi/Meta-Llama-3.1-70B-Instruct-GGUF](https://huggingface.co/MaziyarPanahi/Meta-Llama-3.1-70B-Instruct-GGUF)
- Model creator: [meta-llama](https://huggingface.co/meta-llama)
- Original model: [meta-llama/Meta-Llama-3.1-70B-Instruct](https://huggingface.co/meta-llama/Meta-Llama-3.1-70B-Instruct)
## Description
[MaziyarPanahi/Meta-Llama-3.1-70B-Instruct-GGUF](https://huggingface.co/MaziyarPanahi/Meta-Llama-3.1-70B-Instruct-GGUF) contains GGUF format model files for [meta-llama/Meta-Llama-3.1-70B-Instruct](https://huggingface.co/meta-llama/Meta-Llama-3.1-70B-Instruct).
### About GGUF
GGUF is a new format introduced by the llama.cpp team on August 21st 2023. It is a replacement for GGML, which is no longer supported by llama.cpp.
Here is an incomplete list of clients and libraries that are known to support GGUF:
* [llama.cpp](https://github.com/ggerganov/llama.cpp). The source project for GGUF. Offers a CLI and a server option.
* [llama-cpp-python](https://github.com/abetlen/llama-cpp-python), a Python library with GPU accel, LangChain support, and OpenAI-compatible API server.
* [LM Studio](https://lmstudio.ai/), an easy-to-use and powerful local GUI for Windows and macOS (Silicon), with GPU acceleration. Linux available, in beta as of 27/11/2023.
* [text-generation-webui](https://github.com/oobabooga/text-generation-webui), the most widely used web UI, with many features and powerful extensions. Supports GPU acceleration.
* [KoboldCpp](https://github.com/LostRuins/koboldcpp), a fully featured web UI, with GPU accel across all platforms and GPU architectures. Especially good for story telling.
* [GPT4All](https://gpt4all.io/index.html), a free and open source local running GUI, supporting Windows, Linux and macOS with full GPU accel.
* [LoLLMS Web UI](https://github.com/ParisNeo/lollms-webui), a great web UI with many interesting and unique features, including a full model library for easy model selection.
* [Faraday.dev](https://faraday.dev/), an attractive and easy to use character-based chat GUI for Windows and macOS (both Silicon and Intel), with GPU acceleration.
* [candle](https://github.com/huggingface/candle), a Rust ML framework with a focus on performance, including GPU support, and ease of use.
* [ctransformers](https://github.com/marella/ctransformers), a Python library with GPU accel, LangChain support, and OpenAI-compatible AI server. Note, as of time of writing (November 27th 2023), ctransformers has not been updated in a long time and does not support many recent models.
## Special thanks
🙏 Special thanks to [Georgi Gerganov](https://github.com/ggerganov) and the whole team working on [llama.cpp](https://github.com/ggerganov/llama.cpp/) for making all of this possible. |
owkin/phikon | owkin | "2024-09-26T12:45:48Z" | 1,256,275 | 27 | transformers | [
"transformers",
"pytorch",
"safetensors",
"vit",
"image-feature-extraction",
"biology",
"medical",
"cancer",
"feature-extraction",
"en",
"dataset:owkin/nct-crc-he",
"dataset:owkin/camelyon16-features",
"license:other",
"endpoints_compatible",
"region:us"
] | feature-extraction | "2023-09-21T13:17:37Z" | ---
license: other
language:
- en
tags:
- biology
- medical
- cancer
datasets:
- owkin/nct-crc-he
- owkin/camelyon16-features
pipeline_tag: feature-extraction
---
# Model Card for Phikon
---
> [!IMPORTANT]
> 🎉 Check out the latest version of Phikon here: [Phikon-v2](https://huggingface.co/owkin/phikon-v2)
>
>
Phikon is a self-supervised learning model for histopathology trained with iBOT.
To learn more about how to use the model, we encourage you to read our blog post and view this Colab notebook.
### Model Description
- **Developed by:** Owkin
- **Funded by:** Owkin and IDRIS
- **Model type:** Vision Transformer Base
- **Model Stats:**
- Params (M): 85.8
- Image size: 224 x 224 x 3
- **Paper:**
- Scaling Self-Supervised Learning for Histopathology with Masked Image Modeling. A. Filiot et al., medRxiv 2023.07.21.23292757; doi: [https://doi.org/10.1101/2023.07.21.23292757](https://www.medrxiv.org/content/10.1101/2023.07.21.23292757v2)
- **Pretrain Dataset:** 40 million pan-cancer tiles extracted from [TGCA](https://portal.gdc.cancer.gov/)
- **Original:** https://github.com/owkin/HistoSSLscaling/
- **License:** [Owkin non-commercial license](https://github.com/owkin/HistoSSLscaling/blob/main/LICENSE.txt)
## Uses
### Direct Use
The primary use of the Phikon model can be used for feature extraction from histology image tiles.
### Downstream Use
The model can be used for cancer classification on a variety of cancer subtypes. The model can also be finetuned to specialise on cancer subtypes.
## Technical Specifications
### Compute Infrastructure
All the models we built were trained on the French Jean Zay cluster.
### Hardware
NVIDIA V100 GPUs with 32Gb RAM
### Software
PyTorch 1.13.1
---
### BibTeX entry and citation info
```bibtex
@article{Filiot2023ScalingSSLforHistoWithMIM,
author = {Alexandre Filiot and Ridouane Ghermi and Antoine Olivier and Paul Jacob and Lucas Fidon and Alice Mac Kain and Charlie Saillard and Jean-Baptiste Schiratti},
title = {Scaling Self-Supervised Learning for Histopathology with Masked Image Modeling},
elocation-id = {2023.07.21.23292757},
year = {2023},
doi = {10.1101/2023.07.21.23292757},
publisher = {Cold Spring Harbor Laboratory Press},
url = {https://www.medrxiv.org/content/early/2023/07/26/2023.07.21.23292757},
eprint = {https://www.medrxiv.org/content/early/2023/07/26/2023.07.21.23292757.full.pdf},
journal = {medRxiv}
}
``` |
trl-internal-testing/tiny-random-LlamaForCausalLM | trl-internal-testing | "2024-04-23T12:12:07Z" | 1,255,946 | 6 | transformers | [
"transformers",
"pytorch",
"safetensors",
"llama",
"text-generation",
"arxiv:1910.09700",
"autotrain_compatible",
"text-generation-inference",
"endpoints_compatible",
"region:us"
] | text-generation | "2023-03-29T07:11:13Z" | ---
library_name: transformers
tags: []
---
# Model Card for Model ID
<!-- Provide a quick summary of what the model is/does. -->
## Model Details
### Model Description
<!-- Provide a longer summary of what this model is. -->
This is the model card of a 🤗 transformers model that has been pushed on the Hub. This model card has been automatically generated.
- **Developed by:** [More Information Needed]
- **Funded by [optional]:** [More Information Needed]
- **Shared by [optional]:** [More Information Needed]
- **Model type:** [More Information Needed]
- **Language(s) (NLP):** [More Information Needed]
- **License:** [More Information Needed]
- **Finetuned from model [optional]:** [More Information Needed]
### Model Sources [optional]
<!-- Provide the basic links for the model. -->
- **Repository:** [More Information Needed]
- **Paper [optional]:** [More Information Needed]
- **Demo [optional]:** [More Information Needed]
## Uses
<!-- Address questions around how the model is intended to be used, including the foreseeable users of the model and those affected by the model. -->
### Direct Use
<!-- This section is for the model use without fine-tuning or plugging into a larger ecosystem/app. -->
[More Information Needed]
### Downstream Use [optional]
<!-- This section is for the model use when fine-tuned for a task, or when plugged into a larger ecosystem/app -->
[More Information Needed]
### Out-of-Scope Use
<!-- This section addresses misuse, malicious use, and uses that the model will not work well for. -->
[More Information Needed]
## Bias, Risks, and Limitations
<!-- This section is meant to convey both technical and sociotechnical limitations. -->
[More Information Needed]
### Recommendations
<!-- This section is meant to convey recommendations with respect to the bias, risk, and technical limitations. -->
Users (both direct and downstream) should be made aware of the risks, biases and limitations of the model. More information needed for further recommendations.
## How to Get Started with the Model
Use the code below to get started with the model.
[More Information Needed]
## Training Details
### Training Data
<!-- This should link to a Dataset Card, perhaps with a short stub of information on what the training data is all about as well as documentation related to data pre-processing or additional filtering. -->
[More Information Needed]
### Training Procedure
<!-- This relates heavily to the Technical Specifications. Content here should link to that section when it is relevant to the training procedure. -->
#### Preprocessing [optional]
[More Information Needed]
#### Training Hyperparameters
- **Training regime:** [More Information Needed] <!--fp32, fp16 mixed precision, bf16 mixed precision, bf16 non-mixed precision, fp16 non-mixed precision, fp8 mixed precision -->
#### Speeds, Sizes, Times [optional]
<!-- This section provides information about throughput, start/end time, checkpoint size if relevant, etc. -->
[More Information Needed]
## Evaluation
<!-- This section describes the evaluation protocols and provides the results. -->
### Testing Data, Factors & Metrics
#### Testing Data
<!-- This should link to a Dataset Card if possible. -->
[More Information Needed]
#### Factors
<!-- These are the things the evaluation is disaggregating by, e.g., subpopulations or domains. -->
[More Information Needed]
#### Metrics
<!-- These are the evaluation metrics being used, ideally with a description of why. -->
[More Information Needed]
### Results
[More Information Needed]
#### Summary
## Model Examination [optional]
<!-- Relevant interpretability work for the model goes here -->
[More Information Needed]
## Environmental Impact
<!-- Total emissions (in grams of CO2eq) and additional considerations, such as electricity usage, go here. Edit the suggested text below accordingly -->
Carbon emissions can be estimated using the [Machine Learning Impact calculator](https://mlco2.github.io/impact#compute) presented in [Lacoste et al. (2019)](https://arxiv.org/abs/1910.09700).
- **Hardware Type:** [More Information Needed]
- **Hours used:** [More Information Needed]
- **Cloud Provider:** [More Information Needed]
- **Compute Region:** [More Information Needed]
- **Carbon Emitted:** [More Information Needed]
## Technical Specifications [optional]
### Model Architecture and Objective
[More Information Needed]
### Compute Infrastructure
[More Information Needed]
#### Hardware
[More Information Needed]
#### Software
[More Information Needed]
## Citation [optional]
<!-- If there is a paper or blog post introducing the model, the APA and Bibtex information for that should go in this section. -->
**BibTeX:**
[More Information Needed]
**APA:**
[More Information Needed]
## Glossary [optional]
<!-- If relevant, include terms and calculations in this section that can help readers understand the model or model card. -->
[More Information Needed]
## More Information [optional]
[More Information Needed]
## Model Card Authors [optional]
[More Information Needed]
## Model Card Contact
[More Information Needed] |